Students explain evolution by natural selection differently for humans versus nonhuman animals
Abstract
Evolution is foundational to understanding biology, yet learners at all stages have incomplete and incorrect ideas that persist beyond graduation. Contextual features of prompts (e.g., taxon of organism, acquisition vs. loss of traits, etc.) have been shown to influence both the learning process and the ideas students express in explanations of evolutionary processes. In this study, we compare students’ explanations of natural selection for humans versus a nonhuman animal (cheetah) at different times during biology instruction. We found “taxon” to be a significant predictor of the content of students’ explanations. Responses to “cheetah” prompts contained a larger number and diversity of key concepts (e.g., variation, heritability, differential reproduction) and fewer naïve ideas (e.g., need, adapt) when compared with responses to an isomorphic prompt containing “human” as the organism. Overall, instruction increased the prevalence of key concepts, reduced naïve ideas, and caused a modest reduction in differences due to taxon. Our findings suggest that the students are reasoning differently about evolutionary processes in humans as compared with nonhuman animals, and that targeted instruction may both increase students’ facility with key concepts while reducing their susceptibility to contextual influences.
INTRODUCTION
Dobzhansky (1973) famously wrote, “Nothing in biology makes sense except in the light of evolution”. As such, evolutionary theory provides the needed context, underpinnings, and coherence to understand biological complexity (Alters and Alters, 2001; Blackwell et al., 2003). Evolution is to biology what plate tectonics is to geology, relativity is to time, and heliocentrism is to astronomy (Deniz and Borgerding, 2018a). However, despite its importance, it is perhaps one of the most controversial and polarizing science topics (Glaze and Goldston, 2015; Pobiner, 2016). Across many countries and cultures, a significant proportion of people do not accept evolution as the unifying theory that explains the origin and diversity of life (Downie and Barron, 2000; Miller et al., 2006; Nehm and Schonfeld, 2007; Thagard and Findlay, 2010; Smith, 2010a, 2010b; Allmon, 2011; Council of Europe, 2017; Oliveira and Cook, 2018; Deniz and Borgerding, 2018b; Brenan, 2019).
Understanding evolution is a critical component of science literacy and its centrality to the biology curriculum is broadly valued by the scientific community. National and international reports that provide guidance for science teaching at both the K-12 and undergraduate level have stressed inclusion of evolution as a foundational concept (AAAS, 2011; NGSS Lead States, 2013; UK Department of Education, 2015; Deniz and Borgerding, 2018a). However, a significant number of students graduate from college without an understanding of evolution even after rigorous training in science (Alters and Nelson, 2002; Kalinowski et al., 2010; Pobiner et al., 2018).
Students have great difficulty comprehending and explaining evolution, and misconceptions often persist despite explicit instruction (Bishop and Anderson, 1990; Nehm and Reilly, 2007; Sinatra et al., 2008; Bray Speth et al., 2009; Catley and Novick, 2009; Morabito et al., 2010; Smith, 2010a, 2010b; Nehm and Ridgway, 2011). Some studies have suggested that students are also less likely to retain concepts related to evolution than noncontroversial topics such as photosynthesis (Sinatra et al., 2003; Nehm and Schonfeld, 2007; Glaze and Goldston, 2015). Developing conceptual understanding of evolutionary concepts is further inhibited by the presence of lexically ambiguous terms (e.g., pressure, purpose, cause, adapt; Mead and Scott, 2010a, 2010b; Rector et al., 2013).
Theoretical Framework and Research Questions
As with any concept, students’ knowledge and understanding of evolutionary theory is not created in a vacuum but is affected by the setting in which it is constructed (Brown et al., 1989; Hall, 1996; Van Oers, 1998). Context plays a vital role not only in shaping, but also in eliciting and activating this knowledge (Jones et al., 2000; Clark, 2006; Hofer, 2006; Sabella and Redish, 2007). These ideas are central to the theory of situated cognition which posits that learning and problem solving do not happen in abstraction, but rather by contextualizing and reasoning about information and problems using the particular context in which they are presented (Brown et al., 1989; Krish, 2009). According to this theory, knowledge is not directly transferred across contexts but is “dynamically constructed, remembered, reinterpreted” using contextual cues (Clartcey, 2009, p. 17). Therefore, understanding how context both helps and hinders learning and knowledge transfer is of paramount importance if we are to improve science literacy (NASEM, 2016, 2018).
As a construct, “context” can be complex because it can mean many things. For example, social and cultural contexts comprise multiple variables that characterize one’s physical space as well as associated norms, values, and behaviors. We are all likely familiar with the influence of one’s setting in how we approach and solve problems. For example, computing the same fraction may be perceived differently when baking a cake versus solving it as an item on a school math worksheet. Even experts are not immune from contextual influences. Gros et al. (2019) showed that experts struggled to solve simple math problems when contextualized around daily-life scenarios. Discipline imparts its own social and cultural context and has been shown to influence experts’ interpretations of relevant phenomena (Schwarz et al., 2020) and students’ reasoning and approaches to problem solving. For example, students coenrolled in college biology and chemistry courses used different language and reasoning when explaining both protein structure–function relationships (Kohn et al., 2018a) and energy (Kohn et al., 2018b) where differences were linked to the course in which they completed the assessment.
Given the potential for context to bias what knowledge is elicited, contextual features of prompts (i.e., prompt context) must be carefully considered when designing assessments. Prompt context can encompass a broad range of types and/or quantities of information included in a task stem, including personal perspectives, examples provided, activities of objects, amount of background text, etc. (Son and Goldstone, 2009; Urhahne et al., 2011; Krell et al., 2012). Prompts that differ contextually despite testing for the same conceptual ideas have been shown to result in major differences in the content of students’ responses and performance on assessments (Chi et al., 1981; Potari and Spiliotopoulou, 1996; Schurmeier et al., 2010). Even minor changes in phrasing can lead students to interpret a prompt differently than was intended or to focus on irrelevant or superficial features unrelated to the concept being assessed (e.g., diSessa et al., 2004; Ozdemir and Clark, 2009). Often, such variations in task stems and assessment prompts create unintended differences between the items compared (e.g., the nature of examples provided results in differences in numbers of words or quantities of explanatory background) making it impossible to isolate the causal variable accounting for performance differences.
Assessing students’ conceptions of evolution, in particular, has proven especially sensitive to prompt context influences. Evans (2008) lists multiple studies that show major differences in responses to questions about micro- versus macroevolutionary processes. Kampourakis and Zogza (2008, 2009) found the content of students’ responses related to differences in the structure and content of prompts that had been designed to be conceptually similar. However, among the many sources of variation in prompt context, organism (or biological taxon) emerges as a potential factor influencing students’ reasoning about evolution. An early study by Clough and Driver (1986) documented differences in the conceptual frameworks students applied when explaining evolution by natural selection for the origin and prevalence of different colors in caterpillars versus thick fur in Arctic foxes. Since then, numerous studies suggest organism may be a plausible/likely factor related to differences in students’ reasoning about and performance on evolution assessments (e.g., Ha et al., 2006; Nehm and Schonfeld, 2008; Nehm and Ha, 2011; Göransson et al., 2020).
Assessing evolutionary knowledge and understanding is most problematic when considering humans as the evolving organism. Ever since Darwin proposed evolutionary theory, human evolution by natural selection has been controversial. The society he lived in, including his peers (A.R. Wallace included), took objection to the fact that humans were not the exception (Mayr, 1982). Even today, people are more willing to accept natural selection as an explanation for evolution of species other than humans (Miller et al., 2006; Nadelson and Southerland, 2012; Nadelson and Hardy, 2015). Such trends are seen even among college-educated adults (Brenan, 2019). Many studies that have explored students’ acceptance of human evolution have shown that students reason differently in human versus nonhuman animal contexts (Atran, 1998; Atran et al., 2001; Nettle, 2010) and that acceptance of evolution increases when the organism in question is farther in evolutionary distance from humans (Sinatra et al., 2003; Evans, 2008).
While we know that evolution acceptance can be influenced when considering human versus nonhuman organisms (Sbeglia and Nehm, 2019), we know less about whether differences extend to the content of students’ explanations about evolution by natural selection. Beggrow and Sbeglia (2019) showed that disciplinary context (anthropology vs. biology) was more important than prompt context (human vs. nonhuman) when explaining differences in student responses to questions about human and nonhuman evolution.
In this study, we aim to contribute to a growing understanding about the role of context in influencing students’ reasoning about evolution by asking: 1) How do contextual features influence the content of student responses to prompts about evolution by natural selection? In particular, we explore the influence of “humans” as a contextual feature in a prompt in comparison with nonhuman animals. Hereafter, and for the purposes of this study, we constrain our use of “context” to refer to a specific type of prompt context that explores item features of a prompt or task stem used in assessment (i.e., item-feature context). Our use of “context” is consistent with definitions offered by Krell et al. (2012, 2015) and Nehm and Ha (2011), where “context” refers to specific item features of an assessment prompt (e.g., the organism or trait that is the subject of the prompt) and influences of context are evaluated by comparing otherwise equivalent (i.e., isomorphic) prompts. And 2) How does a semester of active, learner-centered instruction influence the content of student responses to the same prompts? Evolution learning has long been known to be fraught with difficulty, including numerous misconceptions that are notoriously difficult to dislodge (Bishop and Anderson, 1990; Nehm and Reilly, 2007; Sinatra et al., 2008; Bray Speth et al., 2009; Catley and Novick, 2009; Gregory, 2009; Morabito et al., 2010; Smith, 2010a, 2010b; Nehm and Ridgway, 2011). However, there is evidence that “active learning” approaches can be particularly effective in promoting more normative ideas about evolutionary processes (Andrews et al., 2011; Nehm et al., 2022; Sbeglia and Nehm, 2022).
METHODS
Setting, Participants, and Course Structure
This study was conducted at a large, public university in the Midwest in the United States with highest research activity (The Carnegie Classification of Institutions of Higher Education, n.d.). Data for these analyses came from student responses in a large introductory biology course for majors (N = 194 students enrolled) that focused on content domains of genetics, evolution, and ecology. The course is targeted toward sophomores (59% of students in study) but also includes a significant number of juniors (31%) and few freshmen (3%) or seniors (7%). The course is the second in a two-course sequence required for life science majors; the first course focuses on cell and molecular biology. Of the enrolled students, 160 completed all required tasks and were included in the analysis. The study population was 61% female, 21% first-generation college students, and 21% non-White, with an average GPA of 3.2 (4.0 scale). In 2008–2009, the course was transformed to be active, collaborative, learner-centered, and focused on science practices, such as modeling, arguing from evidence, and analyzing and interpreting data. It is important to note, however, that the course was not designed nor modified in any way for the purposes of testing hypotheses related to this study.
Classes met twice weekly for 80-min per class meeting. A survey administered through CATME.org was used at the start of the semester to organize students into teams of four. Grouping criteria privileged diversity in students’ self-reported skills and leadership preferences, and homogeneity in their study habits and schedules. Instruction emphasized engaging students in practices, such as representing and interpreting data, reasoning from evidence, explaining phenomena through explanations and model-based assessments, and modeling biological processes and systems. Course-level learning goals were communicated in the syllabus and daily learning objectives were shared and discussed at the beginning of each class meeting.
Overall, the course was organized into three modules corresponding to primary content (genetics, evolution, and ecology) and linked by the theme of “biological variation”. For each module, overarching questions framed the content relative to the course theme and progressed as follows: 1) How does biological variation arise? How is it expressed and passed on to future generations of cells or organisms? 2) Why is biological variation important within a species? Why do populations differ over time and space? and 3) How does biological variation interact with the environment? Throughout, considerable emphasis was placed on connecting concepts learned in previous class periods to new content. For example, using one’s understanding of genes and alleles (from the genetics module) to explain natural selection (in the evolution module). In addition, case studies were used to explicitly link content across modules and emphasize a “common storyline” that cohered content (e.g., the genetics of the melanin system in dogs was linked to the evolution of dogs from wolves and to the ecology of wolves on Isle Royale).
Typical class periods consisted of short (5–20 min) bouts of instruction followed by questions or problem sets during which students worked in teams to test their ability to apply concepts, link new ideas to existing knowledge, and explore connections among related concepts and principles. In-class activities and homework were designed as low-stakes opportunities for practice and feedback and intentionally aligned with higher-stakes assessments (i.e., exams and quizzes) that aimed to test students’ ability to transfer their knowledge and skills. All assessments were designed using diverse cases and biological contexts to illuminate the transferability and foundational nature of core principles (e.g., central dogma, natural selection, matter transformation, etc.). This strategy was regularly and transparently communicated to students and students were frequently asked to model, explain, and compare instances of the same biological phenomenon across multiple cases (e.g., constructing models of evolution of antibiotic resistance in bacteria and comparing them directly to their models of evolution of fur color in mice). In this way, we aimed to promote students’ abilities to consistently elicit coherent mental models that linked canonical understandings of biological concepts and processes despite case-specific contextual variability.
Assessment Design
We designed four isomorphic prompts based on the ACORNS instrument (Nehm et al., 2012) to assess students’ explanations about natural selection in human and nonhuman animals. Prompts were designed as open-ended questions because prior research has shown they provide better insights into students’ thought processes and subject knowledge (Foddy, 1993).
Each prompt contained the following basal structure: “(Taxon) has (trait). How would biologists explain how a (taxon) with (trait) evolved from an ancestral (taxon) without (trait)?” Contextual features of prompts varied in taxon (human vs. cheetah) and type of trait (structural vs. functional). Cheetahs were chosen as the organism to contrast with humans for several reasons. Cheetahs are broadly recognizable, and therefore a familiar context for students. In evolutionary terms, humans and cheetahs are not very distant (diverged approximately 96 MYA [Kumar et al., 2017] ), and therefore less likely to trigger perceptions about differences attributed to more distantly related species (e.g., snails or salamanders). In addition, prior studies have examined how students reason when the taxon is “cheetah” (Bishop and Anderson, 1990; Nehm and Reilly, 2007; Nehm and Ha, 2011; Göransson et al., 2020) so our prompt aligns well with prior studies that have examined students’ explanations of natural selection using the same context. “Structural traits” in this study refer to morphological traits that affect fitness, specifically “heel bones” in humans and “leg bones” in cheetahs. “Functional traits” are behavioral traits or abilities that similarly affect fitness, such as “walking upright” in humans and “running fast” in cheetahs. Because trait gain and trait loss have been shown to elicit different reasoning in students (Nehm and Ha, 2011), we explicitly designed our prompts to only address trait gain.
From the four prompts, we created two forms of the assessment, hereafter “Human/Cheetah Assessment” (or, HCA, Figure 1A). Each form contained two prompts that differed in taxon (one prompt with humans; one with cheetah) but only one trait type. In other words, forms controlled for trait type while testing for effects due to taxon. Form 1 assessed students’ reasoning about humans versus cheetahs with respect to structural traits, while Form 2 assessed reasoning about humans versus cheetahs for functional traits (Figure 1A). Each student therefore responded to prompts about both taxa (i.e., cheetah and human), but only one trait type (i.e., either structural traits or functional traits; not both; Figure 1B). This design allowed us to fully distinguish differences in reasoning owing to organismal context from differences due to trait type.
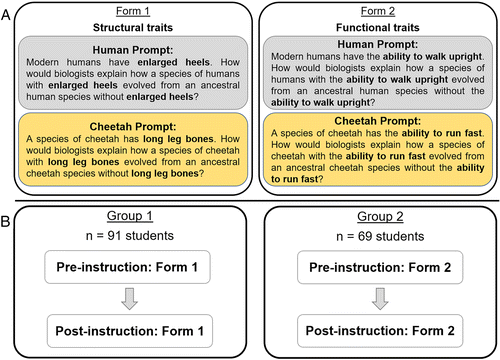
FIGURE 1. Prompts used in the HCA . (A) Two forms of an assessment were developed that differed in trait type (structural vs. functional). (B) Each form prompted students (n = 91, Form 1; n = 69, Form 2) to explain evolution by natural selection for both human and nonhuman animals. Students responded to the same form at the beginning and at the end of the semester.
To control for potential influences of order (Schuman and Presser, 1996; Federer et al., 2015), each form of the HCA was further divided into subforms that differed in the order of appearance of each taxon (i.e., half of the copies of each form had cheetah first and half had human first). Prior research has shown that student performance on assessment tasks can be affected by the sequence in which the assessment items are presented (Monk and Stallings, 1970; Hambleton and Traub, 1974; Gray, 2004; Federer et al., 2015; Carter and Prevost, 2018), and general recommendations are to take task order into consideration when designing assessments (Schuman and Presser, 1996).
Students completed the HCA individually during class time for credit. Formative assessments were administered regularly in class and awarded credit for participation/effort. A study using the same ACORNS instrument, explored the effect of providing credit (extra credit vs. regular credit), and showed no differences in the patterns in student responses (Sbeglia and Nehm, 2022).
In our study, each student provided responses to the same form of the HCA at the beginning and end of the semester (i.e., form was held constant, Figure 1B). Only students who completed the HCA at both times were included in analyses (N of students = 160). The two taxa (human and cheetah) were not referenced during instruction or assessment at any point in the course.
Coding Responses
Students’ explanations were coded using the online assessment tool EvoGrader (Moharreri et al., 2014). EvoGrader codes for the presence of six key evolution concepts (KCs; Variation, Heritability, Competition, Limited Resources, Differential Survival, and Nonadaptive) and three naïve ideas (NIs; Adapt, Need, and Use/Disuse; Table 1). EvoGrader’s reliability and validity have been established in previous studies (see Moharreri et al., 2014) and demonstrated comparable to that of trained human raters (>0.81 Kappa) despite requiring 99% less time for scoring. It is important to note that EvoGrader evaluates presence/absence of concepts; additional analyses and coding approaches are necessary in order to make inferences about correct applications of concepts.
Concept type | Concept name | Concept description |
---|---|---|
Key Concepts | Variation | The presence and causes of variation (mutation/recombination/sex) |
Heritability | The heritability of variation (The degree to which a trait is transmitted from parents to the offspring) | |
Competition | A situation in which two or more individuals struggle to get resources that are not available to everyone | |
Limited Resources | Limited resources related to survival/reproduction, such as food and predators, and reproduction (such as pollinators) | |
Differential Survival/Reproduction | The differential reproduction and/or survival of individuals | |
Nonadaptive Idea | Genetic drift and related nonadaptive factors contributing to evolutionary change | |
Naïve Ideas | Adapt/Acclimation | Adjustment or acclimation to circumstances (which may subsequently be inherited) |
Need/Goal | Goal-directed change; needs as a direct cause of evolutionary change | |
Use/Disuse | The use (or lack of use) of traits directly causes their evolutionary increase or decrease |
Data Analysis
A total of 640 student responses were included in the analyses (N = 160 students; four responses per student). We used three quantitative approaches to determine the influence of context and instruction on student responses.
Abundance and diversity of KCs and NIs. In ecology, abundance indices measure the relative frequencies of organisms in a community, while diversity indices (e.g., Shannon, Simpson) measure variation in the types of organisms (e.g., species) across different communities. In our analyses, we considered the sum of all students’ responses as analogous to a community, and subsets of them representing discrete populations (e.g., the population of responses to a cheetah prompt pre-instruction). We then explored both the diversity (KCs and NIs) and relative abundances of ideas (number of times each KC or NI appears) in the respective populations and in the community in general.
Regression analyses for total number of KCs and NIs. We fitted regressions to understand variation in the total number of KCs and NIs. Because the data (the number of KCs [or NIs] in a response) is discrete, we used a mixed-effects Poisson regression. Specifically, we modeled variation in the number of KCs (and NIs, as part of a separate model) as a function of five predictors of interest (prompt order, taxa, trait, task order, and pre/post) as well as one random intercept term (student ID). The random intercept term allows us to account for nonindependence in the data caused by having multiple measurements from the same student. The nonindependence is because responses from the same student are more similar on average than any two randomly selected responses.
We calculated the 95% confidence intervals on all parameter estimates based on the model standard errors (SEs). The models showed signs of underdispersion, so we refit the models to account for this in two different ways: 1) using a mixed-effects zero-inflated Poisson regression, and 2) using a mixed-effects Conway Maxwell Poisson regression. In both cases, the estimated coefficient values and p values were almost identical, indicating that dispersion was not a major problem. Therefore, we present the results from the mixed-effects Poisson here:
Multiple logistic regression analysis. Students’ responses were sorted into four groups based on the EvoGrader output (See Table 2 for examples of student responses with accompanying EvoGrader codes and corresponding group assignments):
KC only: These responses had only key concepts. The maximum number of key concepts measurable by EvoGrader is six.
Mixed: These responses had both key concepts as well as naïve ideas.
NI only: These responses had only naïve ideas. The maximum number of naïve ideas measurable by EvoGrader is three.
None: These responses had no key concepts or naïve ideas.
Student response | Coded by EvoGrader | Group |
---|---|---|
![]() | Three KCs: Variation, Limited Resources, and Differential Reproduction | KC only |
![]() | One KC: Limited ResourcesOne NI: Need and Adapt | Mixed |
![]() | Two NIs: Use and Need | NI Only |
![]() | None |
For every pair of groups, we modeled the probability of a response belonging to each group as a function of five predictors of interest (prompt order, taxa, trait, task order, and pre/post) as well as one random intercept term (student ID). This approach of fitting several logistic regression models for all pairs of groups is equivalent to fitting one multinomial logistic regression predicting the probability of belonging to any of the four groups. We did not use the (conceptually simpler) multinomial approach in this case because of numerical instabilities producing unreliable output.
Software
All statistical analyses were done using the R statistical environment v 3.6.3 (R Core Team, 2020). We made use of the dplyr (Wickham et al., 2020) and tidyr (Wickham and Henry, 2020) packages for data processing, lme4 (Bates et al., 2015) for mixed-effects logistic regressions, effects (Fox, 2003) for computing and plotting marginal effects, DHARMa (Hartig, 2018) to checking residuals of mixed-effects models for patterns of overdispersion and underdispersion and glmmTMB (Brooks et al., 2017) to fit mixed-effects Poisson regression.
RESULTS
Our results showed that students’ responses were influenced by both prompt context and instruction. Results of our specific analyses are presented with respect to each of our original research questions.
1) How do contextual features influence the content of student responses to prompts about evolution by natural selection?
Results of all three analytic approaches indicated that students’ responses (N = 640 responses) were significantly influenced by both taxon and trait.
Both before and after instruction, responses to questions about cheetahs had more KCs and fewer NIs than questions about humans (with the exception of Variation). Figure 2 shows the percentage of responses that contained each of the six KCs and three NIs for each taxon. Limited Resources was the KC most sensitive to the effect of taxon both before and after instruction. Pre-instruction, only 24% (n = 38) of responses to the human prompt mentioned Limited Resources compared with 62% (n = 99) of responses to cheetah. This was virtually unchanged with instruction, with 29% (n = 46) and 63% (n = 101) of responses to human and cheetah, respectively, mentioning Limited Resources. In contrast, Variation increased significantly with instruction, but there was almost no difference due to taxon. Interestingly, post-instruction Variation is the only instance in which we saw a higher frequency of a KC in the human prompt (approximately 6% n = 10 more) compared with cheetah.

FIGURE 2. Percentage of responses that contain each of the six key concepts and three naïve ideas pre- and post-instruction. KCs occurred more frequently in cheetah responses and responses written at the end of the semester. NIs occurred less frequently at the end of the semester. For numeric data equivalents refer to Supplemental Table S1.
The mixed-effects Poisson regression (Supplemental Figures S1 and S2) and the mixed-effects logistic regression (Supplemental Figures S3–S8) both show that taxa and trait influence the content of student explanations. Two of the other predictors of interest (prompt order, task order) did not show any effects. The results relating to the final predictor of interest (instruction, i.e., pre/post) are described in the next section.
Overall, responses had an average of 1.7 KCs and 0.4 NIs. Number of KCs differed between taxa, with a mean of 1.8 KCs for cheetah versus 1.4 KCs for human (p < 0.001; Figure 3). Most of the responses did not have any NIs, and the number of NIs differed based on the type of trait. Responses had an average of 0.3 NIs when the prompt was about a functional trait and 0.2 NIs when the prompt was about a structural trait (p < 0.05; Figure 4).
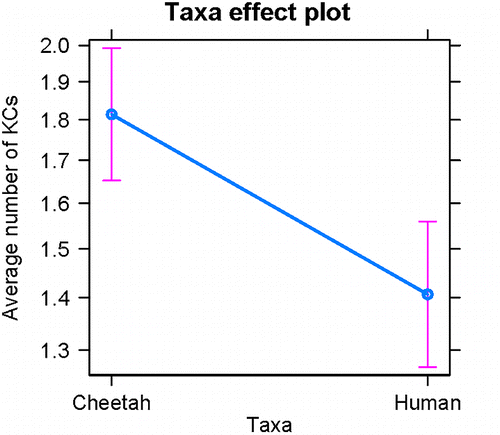
FIGURE 3. Average number of KCs in responses for each of the two taxa, estimated by the fitted model.

FIGURE 4. Average number of NIs in responses for each of the two traits, estimated by the fitted model.
Students’ responses were even less likely to have either KCs only or a mixture of KCs and NIs, than no KCs or no ideas (KCs nor NIs) in their responses to the human prompt (relative to the cheetah prompt, p < 0.001, Table 3).
NI only versus None | Mixed versus None | KC only versus None | Mixed versus NI only | KC only versus NI only | KC only versus Mixed | |
---|---|---|---|---|---|---|
Taxon“Human” | 0.87(0.17, 3.02) | 0.06***(0.01, 0.22) | 0.19***(0.08, 0.41) | 0.04***(0.00, 0.19) | 0.17***(0.06, 0.40) | 1.14(0.66, 1.99) |
Trait“Structural” | 0.89(0.23, 3.57) | 0.27(0.04, 1.29) | 1.21(0.45, 3.34) | 0.31(0.03, 1.56) | 1.06(0.35, 3.15) | 3.97**(1.51, 11.65) |
When responding to the human prompt (relative to the cheetah prompt) students were:
50% less likely to include a mixture of KCs and NIs in their responses, as opposed to only NIs or no ideas at all (Supplemental Figures S4 and S6)
6% less likely to include only KCs than only NIs (Supplemental Figure S7)
10% less likely to include only KCs than no ideas (Supplemental Figure S5).
Students’ responses were more likely to have only KCs, than a mixture of KCs and NIs when they were responding to prompts about a structural trait (relative to a functional trait, p < 0.01, Table 3). Students included only KCs ∼15% more frequently than they included a mixture of KCs and NIs when writing about structural traits (Supplemental Figure S8).
2) How does a semester of active, learner-centered instruction influence the content of student responses to the same prompts?
Results of the abundance and diversity of KCs and NIs in students’ responses (pre- and post-instruction) are shown in Figure 5, A and B, respectively (N = 640 responses for both). Although six KCs are possible, we observed no more than four within any response (n = 47) and a majority contained at least two (n = 398). No KCs were present in 96 student responses. For NIs, the maximum of three naïve ideas were present in only a single response and a majority had 0 naïve ideas (n = 466).

FIGURE 5. Frequencies of (A) KCs and (B) NIs in student responses pre- and post-instruction.
Overall, our results show that instruction increases the number of KCs in responses and decreases the number of responses containing no KCs. Similarly, instruction decreases the number of NIs per response and increases the frequency of responses with no NIs.
Differential Survival was the most frequently applied KC; it was present in more than 50% (n = 167) of the responses pre-instruction and more than 63% (n = 207) responses post, irrespective of prompt context. The least used KC was Non-Adaptive, which appeared in only 1.5% (n = 5) of the responses post-instruction (Figure 2). Variation was the KC most responsive to instruction, with 33% (n = 105) and 47% (n = 150) responses including its pre- and post-instruction, respectively. Post-instruction, >93% (n = 140) of the responses that mentioned Variation were in the KC Only group; only 6.6% (n = 10) of those responses had any NIs at the end of the semester, compared with 14% (n = 15) at the beginning of the semester (Figure 2). Taxon-specific differences in KCs decreased moderately with instruction, with the greatest reductions observed for Heritability (4.4%) and Limited Resources (3.7%; Figure 2).
The above trends are further corroborated by regression analyses (Figure 6, A and B) that show the results of our mixed effects Poisson regressions for significant fixed effects (pre/post-instruction) for KCs and NIs, respectively. Table 4 gives the odds ratios of multiple logistic regressions that show the relative odds of belonging to one of the four previously mentioned groups (Table 2) based on pre/post-instruction (post-instruction as the reference value).

FIGURE 6. Average number of (A) KCs and (B) NIs in responses for pre- and post-instruction, estimated by the fitted model.
NI only versus None | Mixed versus None | KC only versus None | Mixed versus NI only | KC only versus NI only | KC only versus Mixed | |
---|---|---|---|---|---|---|
Pre/post-instruction“post” | 0.49(0.14, 1.47) | 1.13(0.36, 3.76) | 2.06*(1.03, 4.27) | 3.35λ(0.99, 17.29) | 4.62***(2.01, 12.47) | 3.06***(1.75, 5.54) |
Overall, students’ responses contained more KCs following instruction, regardless of taxon or trait type. Post-instruction had 30% more KCs compared with pre-instruction (1.9 vs. 1.4, respectively; p ≤ 0.001; Figure 6A). Additionally, responses had 40% fewer NIs at the end of the semester (p ≤ 0.001; Figure 6B).
Students’ responses were even more likely to have KCs only, than a mixture of KCs and NIs, or only NIs, or no ideas (KCs nor NIs) in their responses pre-instruction (relative to pre-instruction, p ranging from <0.001 to <0.05; Table 4).
Post-instruction (relative to pre-instruction) students were:
13% more likely to include only KCs in their responses in their responses, as opposed to a mixture of KCs and NIs (Supplemental Figure S8)
3% more likely to include only KCs than only NIs (Supplemental Figure S7)
3% more likely to include only KCs than no ideas (Supplemental Figure S5).
Similar patterns were seen even when the responses were separated by taxa (Figure 7, A and B). Postinstruction, the number of responses that included only KCs increased and most of the students’ responses included only KCs. Additionally, the number of responses that included a mixture of KCs and NIs, only NIs, and no ideas, decreased at the end of the semester.

FIGURE 7. Changes in the contents of the responses for students’ responses to the (A) cheetah prompt and (B) human prompt. A total of 160 students provided four responses each: one to the cheetah prompt and one to the human prompt at the start of the semester (pre), and the same at the end of the semester (post). Plots created using SankeyMATIC.
DISCUSSION
Our results are consistent with predictions that emerge from theories of situated cognition—students’ explanations about evolution by natural selection were influenced by both contextual features of prompts (taxon and trait type) and by instruction. Here, we explore our findings in view of previous studies and offer some possible explanations for the patterns we see. Additionally, we will discuss implications for instruction and assessment.
Contextual effects of the prompt
The isomorphic prompts in our study share a common underlying structure and are intended to assess equivalent knowledge despite minor variations in an item feature unrelated to the construct of interest. Because they share the same prompt stem (except for the specific item feature that was intentionally varied) they are designed to go beyond defined standards of equivalency in difficulty and complexity (Kjolsing and Van Den Einde, 2016) to test for students’ ability to transfer concepts across contexts. Terms such as “explanatory coherence” (Kampourakis and Zogza, 2009), “knowledge coherence” (Nehm and Ha, 2011), and “causal flexibility” (Evans, 2008) refer to one’s ability to produce similar responses to isomorphic prompts and identify a relevant concept despite irrelevant or peripheral details. For example, Weston et al. (2015), found that changing a species on questions about photosynthesis did not influence students’ responses. They state that students did not consider the species in the prompt to be a relevant detail and therefore, did not consider it when formulating their response. In contrast, many studies, including our own, have shown that students’ explanations about evolution by natural selection are highly susceptible to contextual features of question prompts (Kampourakis and Zogza, 2008; Schurmeier et al., 2010; Prevost et al., 2013). In particular, our findings are consistent with others that suggest taxon may be particularly influential in shaping students’ responses (Nehm and Ha, 2011; Beggrow and Sbeglia, 2019; Göransson et al., 2020).
In each of our analyses, we found that “taxon” was the most important variable influencing the number and type of KCs in a response and the group to which the response belonged. Responses to prompts about human evolution had fewer KCs and were more likely to have NIs despite instruction. This suggests that students are reasoning differently about humans compared with nonhuman animals. Beggrow and Sbeglia (2019) found that even students who study humans as a focal organism (e.g., anthropology majors) responded with fewer KCs and more NIs in responses to questions about evolution in humans as compared with nonhuman animals. Similar results were obtained by Ha et al. (2006) who found that students were less likely to use “natural selection after mutation” as an explanation in response to questions about human evolution as compared with questions about plants and other animals. It is possible that because students consider humans taxonomically unique (Coley, 2007) and not part of the evolutionary tree (Coley and Tanner, 2015; AAAS, 2018) that they are willing to reason differently about humans in evolution contexts.
Effects of instruction
Increased use of KCs and decreased sensitivity to prompt contexts can be important indicators of students’ understanding of evolution and acceptable measures of instructional efficacy. Our results show that patterns of KCs and NIs changed following instruction. Specifically, we observed an increase in the number of KCs and decrease in the number of NIs per response, as well as a modest reduction in response differences due to taxon. There is abundant literature documenting the difficulties of evolution learning and its resistance to instruction (Bishop and Anderson, 1990; Nehm and Reilly, 2007; Sinatra et al., 2008; Bray Speth et al., 2009; Catley and Novick, 2009; Morabito et al., 2010; Smith, 2010a, 2010b; Nehm and Ridgway, 2011). However, active learning approaches have been shown promising in improving student outcomes. In the specific context of evolution instruction, active learning appears to be effective in producing improved conceptual knowledge as measured by performance on ACORNS instruments. For example, ACORNS assessments were used to document positive learning gains: in introductory biology where differing intensities of active learning were paired with misconception-focused instruction (Nehm et al., 2022) and in an intensive practice-based professional development program for teachers (Cofré et al., 2017). At the undergraduate level, Andrews et al. (2011) showed that practices such as purposefully eliciting and challenging naïve conceptions and emphasizing conceptual frameworks were effective in improving students’ understanding of natural selection.
In our study, we are unable to speculate about causal mechanisms that could explain our outcomes because we did not manipulate nor quantify either our “active learning” approach nor any of the specific pedagogical practices comprised within it. However, we note that our pedagogy did include opportunities for students to explicitly confront misconceptions and apply conceptual frameworks (e.g., central dogma, natural selection, etc.) across diverse cases during in-class, collaborative activities and on homeworks and exams. Overall, our findings are consistent with research that shows that although instruction can increase the accuracy of students’ explanations of evolution (Halldén, 1988; Nehm and Reilly, 2007; Bray Speth et al., 2009, 2014; Andrews et al., 2011; Pobiner et al., 2018; Nehm et al., 2022), influences of context often persist (Ha et al., 2015; Aptyka et al., 2022).
Of the KCs assessed, Variation was most responsive to instruction. Students’ use of Variation increased by 10.6% and 17.5% in cheetah and human contexts, respectively. In the course that was the target of this study, variation was a central theme. Course content was organized around the central questions of: 1) how does biological variation originate at the molecular level? 2) How is molecular-level variation expressed at the organismal level? And, 3) what are the consequences of organismal variation for evolution of populations and ecosystem function? Our data revealed that students gained an appreciation of variation during the semester (14% more inclusion of Variation on average in the post-semester responses). Our findings are consistent with those of Bray Speth et al. (2014) that observed improvement in students’ representations of origin of variation using a similar instructional approach. Additionally, in our study, variation was elicited to a greater extent by the human prompt post-instruction. This could be an artifact of student’s general tendency to categorize by species (not recognize individual-level variation) when asked about nonhuman animals as compared with humans (Nettle, 2010), rather than a direct consequence of instruction causing them to appreciate Variation differentially between the species.
An appreciation of the causes, consequences, and extent of Variation is central to understanding evolution (Halldén, 1988; Shtulman, 2006; Gregory, 2009; Emmons and Kelemen, 2015). Darwin himself recognized the importance of Variation (Darwin, 1868, p. 192) and lamented the lack of understanding of its origin (Darwin, 1859, p. 167). In our study, few of the responses that included Variation had any naïve ideas (9.8% of n = 255 responses across all taxa and at both time points). This is consistent with the findings of Shtulman and Schulz (2008), who showed that students who have a better understanding of within-species variation also have an accurate and mechanistic understanding of natural selection.
We observed that although the presence of naïve ideas decreased post-instruction, they still persisted in students’ responses. At the start of the semester, 34% of responses had naïve ideas compared with the 20% of responses at the end of the semester. Our results are consistent with many studies that have shown that naïve ideas, a form of intuitive thinking, are remarkably resistant to change and frequently coexist with correct scientific conceptions that are fundamentally mutually exclusive (Bishop and Anderson, 1990; Nehm and Reilly, 2007; Sinatra et al., 2008; Bray Speth et al., 2009; Smith, 2010a, 2010b; Nehm and Ridgway, 2011; Shtulman and Valcarcel, 2012).
Linking findings with existing theory
Literature offers several insights that could account for the difficulties associated with teaching and learning evolution. Here, we discuss three hypotheses that may inform our understanding of the patterns we observed: worldview and intuitive thinking, prior knowledge and experience, and scientific expertise.
Students’ worldview and intuitive thinking.
A worldview is a set of deeply entrenched beliefs and expectations that form the framework of a person’s individuality and define how they see the world around them (Glaze and Goldston, 2015). A worldview that is composed of seemingly coherent ideas can also have inconsistencies (Gabora, 1998). These inconsistencies occur as a result of trying to generalize or create an abstraction based on new ideas and concepts, some of which fit into the existing worldview, and some of which need to be “stretched”. There are multiple theories that explain what happens when these new ideas conflict with or threaten an existing worldview (Proulx et al., 2012). One potential strategy is preventing the idea from being assimilated into the worldview and thereby holding conflicting views simultaneously (Gabora, 1998; Taber et al., 2011).
Worldviews regarding evolution often do not change after instruction (Blackwell et al., 2003; Cavallo and McCall, 2008) and can hinder understanding and acceptance of evolutionary theory (Alters and Nelson, 2002; Nehm, 2006; Evans, 2008). Smith (2010a) proposes that such barriers due to worldview can be overcome through education and exposure to empirical evidence. Ingram and Nelson (2006) showed that after instruction about evolution students’ positive views toward evolution increased and students who showed the greatest gains were those who were initially undecided about evolution. Dunk et al (2019) argue that instruction about the nature of science and consideration of students’ social and religious identities can help to increase not only evolutionary knowledge but also acceptance. Cofré et al., (2018) showed that evolution education that included explicit instruction on the nature of science increased acceptance of evolution. Regardless of the specific mechanism, inconsistencies between students’ worldviews and tenets of evolutionary theory (especially with respect to human evolution) could make students more susceptible to contextual influences.
Intuitive ways of thinking can also pose barriers to evolutionary understanding by promoting contextual susceptibility. Smith (2010b) describes these predictable ways of thinking as “rules of thumb” or default approaches that are ingrained into the brain. If one’s intuitions have proven useful in some contexts or gone unquestioned, they are more likely to be used in new situations where there is a general lack of knowledge. Researchers have documented such expected patterns when students reason about biological entities, processes, and phenomena (Inagaki and Hatano, 2006; Coley and Tanner, 2015). Coley and Tanner (2015) categorized biological-intuitive thinking into three different types that they called “construals” namely: teleological thinking, essentialist thinking, and anthropocentric thinking. These patterns of reasoning are powerful and can pose incredible barriers to learning because students do not understand that their reasoning itself is erroneous (Sinatra et al., 2008). Among the most prevalent of these intuitive patterns is teleology, which is attributing a purpose to all events and their cause to intentional agency (Coley and Tanner, 2015). For example, it is common in human discourse to explain an unexpected observation, phenomenon, or pattern by simply stating, “there must be a reason for it.” Such intuitive thinking that everything must have a “reason” or was guided by an overarching force aligns with naïve ideas like need and adapt, where new variation is perceived to arise within a species because it is needed and perpetuates notions that all members of a species are the same. The tendency to consider members of a species as “all the same” can deter students from appreciating the variation that is necessary for evolutionary change.
Students’ prior evolutionary knowledge and education.
Students arrive at every course with previous knowledge and prior conceptions about evolution that they have gained through their formal education and lived experiences. This knowledge often includes evolutionary misconceptions which have been well-documented in the literature (e.g., Gregory, 2009; West et al., 2011). Alters and Nelson (2002), listed several factors such as inconsistent language usage and contradictory learning that can contribute to misconceptions. For example, colloquial terms such as “fitness” and “adaptation” that have distinct meanings in and out of evolution contexts or seeing humans and dinosaurs coexisting in various media.
By the time students reach the undergraduate classroom, their knowledge about evolution has also been influenced by their formal education, the quantity and quality of which is not consistent. Although evolution is now a part of the required curriculum in many countries, it is not required in some and banned outright in others. Even in countries that require evolution to be taught, the grades at which it is introduced, the perspective from which it is taught, and the focus of evolution education varies widely (Deniz and Borgerding, 2018b). In the United States, 20 states have adopted the Next Generation Science Standards (NGSS), which are generally more comprehensive than other state standards with respect to evolution (Gross et al., 2013). However, in their review of the NGSS, Gross et al. (2013) stated that while these standards were better than many state standards with respect to evolution, they too had some important weaknesses including the way they dealt with heredity and the links between DNA and evolutionary relationships. In terms of our study, the major drawback we noticed with the NGSS is that they do not even mention human evolution.
Among the states that have not adopted the NGSS, some do not even mention the word “evolution” in their standards and others make superficial references to it (Lerner, 2000; Vazquez, 2017). Additionally, adopting standards for evolution education does not guarantee they are actually being implemented (Glaze and Goldston, 2015) or that they are being implemented consistently. In some cases, students continue to be taught alternative theories in addition to, or at times instead of evolution (Bowman, 2008). Multiple studies have documented troublesome issues with teachers responsible for evolution education, ranging from inadequate preparation for teaching evolution (Smith, 2010b) to de-emphasizing or avoiding teaching it (Glaze and Goldston, 2015) to purposefully teaching students that “evolution is wrong” (BouJaoude et al., 2011). Such variability and inconsistency in students’ instruction about evolution make it difficult to make any sort of assumptions about their prior knowledge before entering the undergraduate classroom.
Even at the undergraduate level, evolution is rarely presented as a unifying theme for understanding biology, despite its pervasiveness as an explanatory construct across biological research. Instead, evolution is generally taught as a distinct topic without explicitly making it clear how it plays a role in other biological concepts and processes. This is reflected in the structure of textbooks frequently used in undergraduate biology instruction and in the syllabi derived from them (Nehm et al., 2009). Additionally, in the context of this particular study, while “Evolution” is one of the core concepts in Vision and Change (AAAS, 2011), the report does not refer to human evolution either (to be fair – it does not use any other taxa as a reference either).
Such differences in the quantity and quality of students’ prior evolutionary knowledge at both the K-12 and undergraduate levels could explain students’ susceptibility to contextual influences as well as the difficulty associated with changing students’ mental models of evolution that have been shaped by years of exposure and experiences.
Students’ scientific expertise.
As novice science learners, students may be more sensitive to contextual influences when learning complex concepts, such as evolution. There are major differences between the way experts and novices approach problem solving in any field. Experts have a deeper conceptual understanding of their subject matter which enables them to be flexible in identifying and retrieving bits of relevant knowledge. This leads experts to intuitively see patterns that novices are unable to discern (NRC, 2000). Additionally, experts are more able to identify and focus on the abstract principles that underlie a problem’s structure while novices tend to focus on more superficial features (Chi et al., 1981; Hmelo-Silver and Pfeffer, 2004; Nehm and Ridgway, 2011). As novices, it is not surprising that students are influenced by prompt contexts. However, our data suggest that instruction can decrease students’ sensitivity to context, perhaps indicating they are making progress in their transition from novice to expert.
Implications for instruction and assessment
Multiple studies that have looked at different instructional strategies and contexts and have shown varying levels of improvements in students’ understanding of evolution in general (Bray Speth et al., 2009, 2014; Kampourakis and Zogza, 2009; Aptyka et al., 2022; Nehm et al., 2022; Sbeglia and Nehm, 2022), and human evolution in particular (Alters and Nelson, 2002; Kalinowski et al., 2010; Bravo and Cofré, 2016; Pobiner, 2016; Pobiner et al., 2018).
Many researchers have called for evolution to be taught using humans as a focal organism. Nettle (2010) showed gains in student understanding of evolution in general after students were taught evolution in the context of humans. Pobiner et al. (2018) and Pobiner (2016) propose teaching about human evolution as a direct and effective way to decrease barriers to accepting and subsequently understanding evolution. However, Beggrow and Sbeglia (2019) did not find any particular affordances offered by teaching evolution in a human context. Deeper learning can result when the learner identifies with the subject matter and finds it relevant (NRC, 2009). Therefore, because students are highly likely to find themselves and their development interesting (Pobiner et al., 2018), teaching evolution in the human context could mean that students find it relevant and identify with it. We would like to offer the suggestion that while teaching evolution in a solely human context is not optimal, including humans as one of the contexts could be very important.
At various institutions and at the national level, numerous efforts are underway to renovate and align the biology core curriculum. In particular, there is considerable interest in increasing the prominence of science practices as an explicit objective at all levels to teach science as it is practiced and to encourage students to think and reason about science similar to practitioners (AAAS, 2011; Cooper et al., 2015). A lack of scientific accuracy in students’ reasoning is often not because of a lack of knowledge of the scientific principles, but due to inadequate activation, recruitment, or transfer of those scientific principles across contexts (Brown et al., 1989; Clark, 2006; Clartcey, 2009; Nehm and Ha, 2011; diSessa, 2013; Aptyka et al., 2022). Multiple recent meta-analyses have shown that instructional models that incorporate active learning improve student learning gains broadly (Freeman et al., 2014; Shi et al., 2020; Theobald et al., 2020; Bredow et al., 2021). Additionally, studies that have used the ACORNS instrument to measure learning gains of active learning strategies in evolution classrooms have shown similar results (Cofré et al., 2017, 2018; Nehm et al., 2022). Our study, as well as that of Bray Speth et al. (2014), showed gains in student performance in understanding variation after a semester of active learning that incorporated modeling-based practices. Perhaps by using scientific practices such as data analysis, modeling, and argumentation during active learning instruction, and including humans as an instructional context, will lead to a deeper, more conceptual understanding of evolution, an increased ability to transfer relevant concepts, and thereby decrease susceptibility to contextual influences.
Finally, our findings have clear implications for assessment. A common strategy in university classrooms is to design parallel, or alternative, versions of assessments for the purpose of creating multiple exam forms or assessing concepts using contexts that are different from those used during instruction. It is generally assumed that these minor variations are of little significance when measuring learning outcomes. The assumption is that students will identify the underlying concepts being assessed, recruit the relevant knowledge, and transfer it to the new context. However, it has been established that ensuring parallel prompts are equivalent in terms of difficulty is both important and challenging to accomplish (Hamp-Lyons and Mathias, 1994; Lee and Anderson, 2007; Sydorenko, 2011; Li, 2018). The prompts used in our study were designed not just to test for equivalent content but were truly isomorphic, in that they utilized the same prompt stem. The words that varied were also remarkably similar in that both taxa were mammals, close in evolutionary terms (Kumar et al., 2017), and familiar to students (Nehm et al., 2012). However, despite the high degree of prompt similarity, we still saw differences in student responses based on seemingly minor variations in context. Our data, as well as the broader corpus of findings about contextual influences, suggest that assumptions about equivalence among assessments that vary in context are frequently unwarranted. Care must be taken to ensure assessments are fair and unbiased − particularly in high-stakes situations, such as exams or when the assessments are linked to rewards or inclusion in programs or other educational opportunities. To buffer against contextual effects, instruction should feature multiple contexts of the same concept or phenomenon and instructors should provide explicit guidance that distinguishes the underlying principles that transfer across contexts.
Limitations and Future Directions
Due to the constraints of the study design, the degree to which the findings of this study can be generalized is difficult to predict. We compared student responses to prompts about trait gain in two taxa (cheetah and human) and are therefore unable to say how our findings might apply to other taxa that are at different evolutionary distances from humans, or whether students would respond similarly about trait loss. Such systematic comparisons could be explored through the design of multiple prompts varying in evolutionary distance and administered across a large sample of students.
Students’ responses were coded by an online automated response tool (EvoGrader) that only identified the presence/absence of six key concepts and three naïve ideas with respect to evolution. Additional evolutionary concepts (including threshold concepts) or other biologically relevant information (e.g., the level of biological organization at which variation occurs) may have been present in student responses but were not considered in our assessment of outcomes. Similarly, students’ use of language and consistency in their application of KCs and NIs at different points in their narratives were not considered and could potentially influence interpretation of students’ meaning.
This study did not explore whether there are patterns in susceptibility to contextual influences based on demographic characteristics (e.g., sex, race, grades, etc.). Such information could be useful when designing assessments that aim to be fair, accessible, and unbiased in their potential to measure student learning.
Finally, our study reports findings from one introductory biology course taught by one instructor. Although the course had been transformed to be active, learner-centered, and used evidence-based pedagogies, such as cooperative learning, high-frequency low-stakes assessment, and an emphasis on science practices, it was not designed to explicitly test any particular hypothesis about contextual susceptibility. As such, we can neither claim that our findings are broadly generalizable across other course contexts or instructors, nor can we point to specific causal mechanisms underlying the patterns we observed. Identifying the mechanisms that explain how and why students do or do not succumb to minor variations in context will be a significant and impactful contribution to literature, but will require multiple systematically designed studies to do so.
ACKNOWLEDGMENTS
We thank Mitch Distin for his contributions to conceptualizing and designing the study and for help with data collection; Etiowo Usoro and Patrycja Zdziarska for logistical and technical support throughout the project; Mridul Thomas for help with data analysis; and Melanie Cooper, Amelia Gotwals, and Katherine Gross for comments on earlier versions of the manuscript. We also thank Francesco Pomatti and Anita Narwani at the Department of Aquatic Ecology at Eawag, Switzerland for providing space and resources while writing this manuscript. We gratefully acknowledge all the students whose anonymous assessments were used in this study. Finally, we would like to point out that it is not “tea” unless it is made with the leaves of Camellia sinensis.
This is Kellogg Biological Station Contribution No. 2365. This material is based in part upon research supported by the National Science Foundation under grant numbers DRL 1420492, DRL 0910278, DUE 2012933, and DBI-0939454. Any opinions, findings, and conclusions or recommendations expressed in this material are those of the author(s) and do not necessarily reflect the views of the National Science Foundation.