Metacognitive Exam Preparation Assignments in an Introductory Biology Course Improve Exam Scores for Lower ACT Students Compared with Assignments that Focus on Terms
Abstract
Preparing for exams in introductory biology classrooms is a complex metacognitive task. Focusing on lower achieving students (those with entering ACT scores below the median at our institution), we compared the effect of two different assignments distributed ahead of exams by dividing classes in half to receive either terms to define or open-ended metacognitive questions. Completing metacognitive assignments resulted in moderately higher exam scores for students on the second and third exams. Metacognitive assignments also improved accuracy (difference between predicted and actual exam scores) for the second and third exam in lower ACT students, but that improvement was driven largely by higher exam scores in the metacognitive group. Thus, despite the fact that the metacognitive assignments specifically asked students to reflect on their previous exam performance, their previous estimates and predict how well they expected to perform on the exam they were preparing for, there was little evidence that these assignments influenced lower achieving students’ confidence levels any more than assignments where students defined terms. While understanding relevant terms was certainly important in this course, these results highlight that open-ended metacognitive prompts may improve exam scores in some students in introductory biology classrooms.
INTRODUCTION
Metacognition, or the awareness, understanding and monitoring of one’s own learning processes is considered essential for effective learning (Donovan and Bransford, 2005; Flavell, 1979; Tobias and Everson, 2002) and is thought to play a critical role in the academic success of college students (Everson and Tobias, 1998; Isaacson and Fujita, 2006; Young and Fry, 2008). Successful college students are able to distinguish what they know from what they don’t yet know and strategize about how best to master the material they have not yet learned. Metacognitive exercises that ask students to plan, monitor and evaluate regularly have been highlighted as valuable ways to facilitate learning in college biology classes (Stanton et al., 2021; Tanner, 2012). As students engage in metacognition, work to identify gaps in their knowledge and repeatedly reflect on their efforts, they likely learn more about their metacognitive skills and develop more efficient study habits (Donovan and Bransford, 2005). A metacognitive approach is considered particularly important for teaching the application, analysis, and evaluation skills important in science (Zohar and Barzilai, 2013, 2015) and may be critical in supporting students as they develop the lifelong learning skills needed to succeed at higher levels of science (Schraw et al., 2006). In fact, many consider metacognition, critical thinking, and reflection as fundamental components of scientific literacy (Ford and Yore, 2012).
Strong metacognitive skills are likely to be important as students navigate the academic challenges they face transitioning from high school to college classrooms (Everson and Tobias, 1998; Isaacson and Fujita, 2006). We know that students frequently find their introductory science courses require a different kind of learning than high school (Jensen and Moore, 2008a; Nordell, 2009) and that introductory biology students focus more on surface learning rather than the deep learning expected of them in college (Stanger-Hall, 2012; Tomanek and Montplaisir, 2004). While mastery of biology terms is critical, college courses also expect students to move beyond knowing and understanding concepts and require students to be able to apply, analyze, and synthesize material. Such synthesis and integration abilities are likely to require metacognitive skills, since students must be aware of their factual knowledge to access and retrieve that knowledge in order to connect and evaluate new concepts (Tanner, 2012). Students facing more difficult or more complex material may also fail to recognize what they don’t know and are thus more likely to be mis-calibrated or less accurate in their self-assessments (Isaacson and Fujita, 2006; Nietfeld et al., 2005; Schraw and Roedel, 1994; Zell and Krizan, 2014).
Students arriving in our introductory biology courses seem to struggle with these greater demands on their learning and capable but underprepared students may leave the sciences especially as they move through introductory course work (Freeman et al., 2014; Haak et al., 2011; Rath et al., 2007; Tracy et al., 2022). It seems clear that introductory biology students have poor metacognitive skills (Jensen and Moore, 2008) and struggle to actually use those skills (Stanton et al., 2015). We also know that those not using metacognitive strategies such as self-testing and goal setting had lower grades or were less likely to improve their grades than their peers that used these strategies more frequently (Rodriguez et al., 2018; Sebesta and Bray Speth, 2017). In fact, Gregg-Jolly et al. (2016) highlighted the potential role of metacognitive skills in the retention of first-generation students in STEM.
Difficulty in introductory college science courses often revolves around exams and preparing for an exam is a complex task that requires sophisticated metacognitive skills (Haak et al., 2011; Hill et al., 2014; Stanton et al., 2021). Beginning students often focus on exam successes and failures (Lizzio and Wilson, 2013). When they receive low exam scores, they often express frustration, and fail to understand what went wrong and why. They are also uncertain of how to move forward and may have limited knowledge of effective strategies (Stanton et al., 2015; Dye and Stanton, 2017). Some students may not have the ability cope productively with failure, a skill important to success in the STEM fields (Henry et al., 2019). Despite the importance of exams, students seem unsure about how to approach preparing to take an exam, have limited knowledge of the strategies necessary for high achievement (Sebesta and Bray Speth, 2017), and tend to repeatedly adopt less effective strategies (Dye and Stanton, 2017; Rodriguez et al., 2018).
Metacognitive Interventions—Workshops and Exam Wrappers
Given the importance of student metacognition in STEM fields, research has focused on ways of improving those skills, an effort that is likely to be especially important for lower achieving students since they have the most to gain. Metacognitive interventions in college biology classrooms have been shown to improve academic performance and increase the use of metacognitive study strategies. While some research has focused on the addition of activities or workshops emphasizing some aspect of metacognition, other research has focused on the addition of metacognitive-oriented reflective assignments completed after exams. In the former category are studies like those of Osterhage et al. (2019) where students explicitly taught self-evaluation strategies improved relative to those in other sections of the same course. Stanger-Hall et al. (2011) found that students scored higher on exam questions that were reviewed in a workshop with an emphasis on self-testing (a metacognitive approach). In other research, biology students that chose to attend metacognitive sessions performed better on exams than students that did not choose to attend those sessions (Chaplin, 2007; Nordell, 2009). Finally, students themselves perceive metacognitive activities as useful to their learning (Sandall et al., 2014) and reported an increase in metacognitive awareness in a course where active reading skills were emphasized (Hill et al., 2014).
A number of other interventions in biology classes have focused on metacognitive assignments such as exam wrappers, that ask students to analyze and correct exams (Lovett, 2013). These assignments emphasize the use of exams as part of a self-regulated learning cycle, which may help students to focus on the value of learning and understanding where they have gone wrong previously. Dang et al. (2018) found students showed qualitative gains in their metacognition during the semester in a course where all were assigned post exam reviews and Sabel et al. (2017) found that when students chose to use enhanced answer keys and reflection questions those materials helped students engage in metacognition. Performance was also improved in biology classes using such post exam assignments (Mynlieff et al., 2014; Williams et al., 2011). However, in a course where students completed post exam reflections and predicted their grade, students as a group did not become more accurate as they predicted their exam scores across a semester and the metacognitive reflection score the authors used had limited predictive value when it came to performance (Knight et al., 2022).
Lin and Lehman (1999) focused on biology labs rather than exams, and found that students completing an assignment with a metacognitive emphasis after labs had a deeper understanding of scientific experiments and were better able to apply their knowledge on a novel problem relative to students completing other kinds of assignments. In fact, asking open-ended metacognitive questions during a lab module led to increased complexity of responses on a final exam question about scientific research (Dahlberg et al., 2019). However other research in psychology classrooms has not found any effect of these assignments on exam scores or metacognitive ability when controlling for time on task (Soicher and Gurung, 2017).
Metacognitive Interventions—Accurate Self-Assessment
Other metacognitive-related research has focused more explicitly on the ability to self-assess and this skill has also been the focus of extensive research especially in psychology courses. Students that can accurately self-evaluate and monitor their learning are said to have strong calibration skills. Accurate self-evaluation is considered a metacognitive skill necessary for high achievement since students must be able to identify what they don’t know in order to fill in any gaps in their knowledge as they study. As a result, accurate self-assessment is often considered a necessary first step towards improved performance (Everson and Tobias, 1998; Nietfeld et al., 2005; Schraw and Dennison, 1994). Self-monitoring skills have been assessed in many kinds of courses by asking students to estimate how well they will do (predict) or have done (postdict) on exams. Research consistently shows that lower achieving students are chronically overconfident, imagining they know and understand the material they will be tested on when in fact they do not, while higher achieving students tend to be very accurate. In their classic study, Kruger and Dunning (1999) found students in the lowest quartile grossly overestimated their test performance and ability. They argue that incompetence not only causes poor performance but also the inability to recognize that one’s performance is poor. Dunlosky and Rawson (2012) have pointed out that such overconfidence perpetuates underachievement because students will terminate studying before they have mastered the material they will be tested on. Such overconfidence in lower achieving students has been extensively documented in a number of college-level courses (Hacker et al., 2000; Bol and Hacker, 2001; Bol et al., 2005; Nietfeld et al., 2005; Isaacson and Fujita, 2006; Nevid et al., 2015). Such overconfidence has also been confirmed in college biology courses (Jensen and Moore, 2008), being more frequent among the students receiving the lowest grades in biology and chemistry courses where students estimated their grade right before taking an exam (Osterhage et al., 2019; Osterhage, 2021), right after taking an exam (Chaplin, 2007; Dang et al., 2018; Knight et al., 2022) and in an upper level biology course (Ziegler and Montplaisir, 2014). It is also common in college chemistry courses (Karatjas, 2013; Hawker et al., 2016).
Given the overconfidence of lower achieving students, interventions have been developed to address overconfidence by trying to make students more realistic about how they will perform. Students able to accurately predict their performance may put more effort into studying. A number of studies have explored interventions specifically focused on improving such self-monitoring skills with somewhat mixed results. In biology classrooms, several different interventions have focused on improving accuracy. Students were more accurate or better calibrated when the difficulty of self-evaluation was emphasized (Osterhage et al., 2019) and when they completed practice tests (Osterhage, 2021). However, students did not increase their accuracy across a semester when completing reflections after exams (Knight et al., 2022). Research in psychology classrooms has shown that the addition of monitoring exercises and feedback on exams increased both the accuracy of students’ predictions and their achievement (Nietfeld et al., 2006), but improved accuracy does not always lead to improved exam scores (Miller and Geraci, 2011). In some cases, accuracy improved with extra credit incentives (Hacker et al., 2008), but in other cases incentives and training improved the accuracy of exam score predictions and achievement only when students also received feedback on exams (Callender et al., 2016). Other interventions, such as regular practice making predictions (Bol and Hacker, 2001), and overt sharing of predictions (Bol et al., 2005) have not necessarily improved the ability of students to predict their exam scores or improved their exam scores.
Our Goals
Faculty discussions as part of an Accredited Colleges of the Midwest (ACM) Teagle Collegium on Metacognition (Ottenhoff, 2011) highlighted that these somewhat different approaches (metacognitive workshops, exam reflections, and interventions focused on improving accuracy) are all broadly connected and have the potential to be blended in a classroom setting. In addition, these discussions highlighted that the relationship between a student’s exam performance and their ability to accurately self-assess by predicting their grade is complex. Callender et al. (2016) have also suggested that dissecting the relationship between these three (exam performance, predictions of performance and the accuracy of those predictions) has theoretical importance and noted that this approach is often overlooked in the literature. Lastly, these discussions highlighted that examining the relationship between these three across a semester with a particular focus on lower achieving students would be most productive, since lower achieving students have the most to gain from an intervention. Higher achieving students are more likely to already have strong metacognitive skills and are thus less likely to show improvements in performance.
Our research focused explicitly on lower achieving students as we explored the relationship between exam performance, predictions of performance, and the accuracy of those predictions by introducing a metacognitive assignment ahead of exams and compared that with an assignment that required students to define terms. In this study, students were separated into higher and lower ACT achievement groups based on their incoming ACT score (higher achieving students were defined as those at or above the median incoming ACT at our institution and lower achieving were defined as those below the median incoming ACT). This approach is unlike other research that has used the exam scores students were receiving in the courses being studied to sort students into achievement groups. We selected ACT scores in order to categorize students independent of their grades in the course and viewed ACT as an objective and generalizable measure of achievement.
We relied on a framework that divides metacognition into metacognitive knowledge and metacognitive regulation (Schraw and Moshman, 1995; Stanton et al., 2021). Metacognitive knowledge includes knowledge of one’s thinking as well as knowledge of when and how to use different learning strategies. Metacognitive regulation includes one’s ability to plan, monitor, and evaluate learning. In the context of this metacognitive framework, improved performance (higher exam scores) may be a function of students’ being more aware of what material in the course they know and don’t know, which then could help them identify and fill gaps in their knowledge as they study. The ability to accurately predict what you know is often considered a metacognitive knowledge skill, but also likely includes some metacognitive regulation, since students must also monitor and evaluate changes in what they know. Students with these abilities are likely to be more accurate when asked to predict their performance since they know what they don’t know. But this metacognitive skill (knowing what you know) is only one aspect of metacognition. Improved performance may also be a function of a students’ ability to plan (a different metacognitive regulation skill), to understand their own thinking processes (a metacognitive knowledge skill) and to understand when and how to use different learning strategies (also a metacognitive knowledge skill). Thus, it is unclear the degree to which we should consider the ability to predict performance as a general measure of a students’ metacognitive ability or whether we should focus on developing this skill in students.
To explore the relationship between metacognitive related assignments, exam performance and accurate self-assessment, we integrated previous approaches to improving student metacognition (metacognitive workshops, exam reflections and interventions focused on improving accuracy), by dividing each class in half to receive a different type of assignment as they prepared for three exams. One assignment included open-ended metacognitive knowledge and regulation prompts that asked students about what material they were confident of and what material they still needed to study and asked them about the strategies they were planning to use or were currently using to study. Ahead of the second and third exams, the metacognitive assignment also asked students to review their previous exam and explain where they went wrong, compare how the grade they received compared with their estimate and how they would modify their study practices. These questions were based on questions we found ourselves asking students during office hours ahead of exams. We contrasted the effects of these metacognitive exam preparation assignments with a different but also potentially valuable assignment that asked students to define or identify biology terms relevant to the exam they were about to take. Both assignments asked students to predict the grade they expected to receive on the exam they were preparing for.
Collecting exam scores and exam score predictions across the semester allowed us to look closely at the relationship between these two variables, an approach that has recently been used by several other researchers in biology classrooms (Dang et al., 2018; Knight et al., 2022; Osterhage, 2021; Osterhage et al., 2019). Exam scores and exam score predictions enabled us to calculate accuracy, by subtracting the actual from the predicted score. While we use the term accuracy, Schraw (2009) refers to this as bias and others use the term discrepancy score (Osterhage et al., 2019). Recent research also refers to this more specifically as prediction accuracy (Knight et al., 2022). Students that are overconfident or over predict will have a positive score, while those that are under confident or under predict will have a negative score. In addition, the magnitude of the distance from zero provides information about the severity of judgment error.
Our first research question (RQ1) examined the effect of our metacognitive and term definition assignments on exam scores. We then examined the effect of our assignments on the ability of students to predict their exam scores (RQ2) and on their accuracy (predicted-actual score) (RQ3). We used the median ACT score for incoming students at our institution to separate students into two groups, higher ACT (at or above the median) and lower ACT (below the median).
RQ1 How Do Metacognitive Assignments Affect Exam Scores?
We expected metacognitive assignments to improve exam scores relative to students assigned to define terms. Since higher ACT students are likely to already have strong metacognitive skills, we expected metacognitive assignments would disproportionately improve the exam scores of lower ACT students.
RQ2 How Do Metacognitive Assignments Affect Exam Score Predictions?
We expected metacognitive assignments would result in lowered exam score predictions relative to students assigned to define terms. Since higher ACT students are likely to already have strong metacognitive skills, we expected metacognitive assignments would disproportionately improve the predictions of lower ACT students.
RQ3 How Do Metacognitive Assignments Affect Accuracy?
We expected metacognitive assignments would result in increased accuracy relative to students assigned to define terms. Since higher ACT students are likely to already have strong metacognitive skills, we expected metacognitive assignments would disproportionately improve the accuracy of lower ACT students.
The decision to directly contrast the effects of two different kinds of assignments in a classroom setting was in response to a call for more rigorous controlled studies on the effects of using metacognitive assignments in classrooms (Zohar and Barzilai, 2013; Callender et al., 2016). Moreover, direct comparisons of two assignments provides faculty with an authentic comparison they can use as they decide which of many different kinds of assignments are meaningful in busy introductory biology courses, where understanding terms is critical. Our approach was an attempt to control for time on task (Mynlieff et al., 2014) since both assignments required students to spend time completing an assignment related to the material they were learning. Unlike exam wrappers, which are typically assigned after an exam, questions about the previous exam were asked of students as they studied for the next exam in order to capitalize on students’ attention and motivation as they prepared for the exam they were about to take. Our choice to have several assignments distributed across the semester was also in response to concerns that students need more than a one-time metacognitive workshop or assignment (Nietfeld et al., 2006). We did not evaluate the responses to the metacognitive assignments, students were simply asked to respond to metacognitive knowledge and regulation reflection questions and exam scores, predictions and accuracy were monitored in our two assignment groups.
METHODS
Participants and Context
St. Olaf is a liberal arts college of almost 3000 students and biology tends to be one of the largest majors on campus with almost half of incoming students declaring some interest in being a biology major. Participants were students in five sections of the same class taught by three instructors. This course is an Evolution and Diversity course which is the second of a two-semester introductory sequence for biology majors generally taken in either their first or second year. Sections ranged in size from 49 to 70 students and classes took place between 2010 and 2013. Instructor 1 taught one section (enrolled students = 70), Instructor 2 taught one section (enrolled students = 68), and Instructor 3 taught three sections enrolled students = 50, 70, 53). Only data from the 233 students that fully completed the three assignments during the semester and agreed to be part of the study out of a total of 311 enrolled students were included in analyses. Within each class there were 1−3 students that chose not to be involved in the study. For a summary, please see Supplemental Tables S3 and S4.
We used ACT scores to separate higher and lower achieving students and used the median for incoming first-year students to divide the two groups. Students scoring at or above the median for entering students at St. Olaf were defined as higher ACT students (29 and above, N = 151). Those that scored below the median were lower ACT students (28 and below, N = 82). Students without any standardized testing scores were dropped from the data and those that took the SAT were adjusted using concordance tables published by the College Board. While ACT scores only explain part of the variation in student achievement in college, the scores were chosen here because these data enabled us to sort students in a way that was independent from their performance in the course itself and stable over time. Both the decision to use ACT scores and the decision to define a higher and a lower achieving group were made in the planning stages of the study to align with the questions faculty were posing during discussions as part of an ACM Teagle Collegium on Metacognition (Ottenhoff, 2011) and have been used in previous research (Mynlieff et al., 2014). In addition, we chose to use median ACT scores to define these two groups because ACT scores were perceived by faculty at our institution as more objective and generalizable to other institutions than some other grouping measures and, at our institution, that median was stable over time. In addition, there were concerns that first exams in each course (a different way to group students into higher and lower achievement groups) may vary in difficulty from one faculty member to another, making comparisons across instructors difficult and that grouping students by college GPA would be incomplete since it might only be based on a small number of previous courses since this was an introductory course.
The three faculty that taught the course each had a minimum of 15 years of teaching experience, used the same text, covered the same chapters, shared laboratory exercises, had the same number of exams and roughly similar writing assignments. All exams were valued at 100 points and included both questions geared toward remembering and understanding material as well as the ability to apply, analyze, and evaluate. While exams may have had some multiple-choice questions, most questions were short or longer essay questions. All five sections of the course taught by the three faculty generally included a diversity of approaches to teaching including lecture, some active learning and discussion as well as at least some small group work during class. The first of three exams in the course covers natural selection and evolution, including the evolution of populations, species and speciation and the history of life on earth, the second exam covers phylogenies, bacteria, archaea, protists, and plant and fungal diversity. The third and final exam covers animal diversity with a focus on invertebrates and vertebrates. The third exam includes some cumulative material on themes in the course.
Students were informed about the research during class and were given the option to decline participation in the study although the assignments were required whether or not students agreed to be part of the study. Other than an initial introduction of the study in the classroom where students were provided with a consent form to sign if they chose to participate, all communication was through email unless students brought up the assignments during office visits. The St. Olaf College Institutional Review Board approved this study (IRB 0910-04).
Exam Preparation Assignments
Two different exam preparation assignments were emailed to students before each of the three exams (two midterms and a final). Half of the students were emailed an assignment that required them to respond to a series of open-ended metacognitive oriented questions or prompts (Supplemental Table S1) and half were required to define terms related to material that was likely to be on the exam (Supplemental Table S2). The decision to divide each section into two groups was based on the fact that at our institution sections of the same course can differ with the kinds of students enrolled. For example, the section students enroll in is often a function of the timing of other classes they need to take (i.e., if one section overlaps with organic chemistry all organic students will enroll in one of the two sections) and may be related to their level of motivation (students that enroll in an 8 am section may be differently motivated than students that enroll in a 10 am section). This approach also avoids student’s perceptions that one section might be doing something different and interesting and therefore having either positive or negative preconceptions. Each section was divided in half by randomly choosing the first student in alphabetical order to be in one treatment group and then alternating placement.
Students were emailed their assignments several days ahead of the exam and received one reminder to respond before taking the exam. For example, assignments sent out on Wednesday were expected to be completed by Friday if the exam was on a Monday, assignments sent out on Friday were expected to be completed by Monday for an exam scheduled on a Wednesday and assignments sent out on Monday were due on Wednesday for a Friday exam. We expected both assignments would take ∼ 20−30 min each to complete based on observing students completing such surveys during class before the start of this study, but we did not measure the actual time students spent completing the two assignments. Students were assigned a small number of points (equivalent to 3−5% of each exam) for each assignment as long as they responded thoroughly. At the end of each assignment, all students were also asked to estimate the grade (%) they expected they would receive on the exam they were preparing to take. Although all students were asked to estimate their grade, only the metacognition group was asked to reflect on their previous exam if they had taken one, before estimating the grade they would receive on the exam they were preparing for. Assignments that included biology terms to define focused on terms likely to be included on the material that students were to be tested on and were selected from class or chapter readings. Assignments that included the open-ended metacognitive questions varied from exam to exam across the semester in order to keep students engaged (Soicher and Gurung, 2017) but included questions that focused on both metacognitive knowledge and regulation. For example, students were asked about the study strategies they used in the past and the effectiveness of those strategies and what techniques they were using or planning to use to master material in the text and in the classroom. They were asked about concepts they were struggling with, why they thought they were having trouble with those concepts and how they were planning to come to an understanding of that material. Once students had taken their first exam, they were also asked to review their predictions of their performance, analyze where they had gone wrong, and address how they were going to change their studying if they had not done as well as they expected.
Because each section had students receiving both types of assignments, we did not present any information on the details of the two different assignments during class. In other words, there was no presentation during class describing either what metacognition is, why it is important or describing why knowing terms might be important in biology. In two of the five classes, the faculty member teaching the course was blind to which students were receiving metacognitive assignments or term-defining assignments since a faculty member other than the individual teaching the course was sending and receiving the email assignments. In the remaining three sections, the faculty member was the individual sending and receiving the emails.
Data Analysis
All analyses were conducted in R (4.2.2) (R Core Team, 2020). Mixed-effects models were conducted using the lmer function from the package lme4. Plots were generated with the ggplot2 package. We used linear mixed-effects modeling to determine the impact of metacognitive assignments on exam scores, predicted scores, and accuracy when accounting for other factors likely to influence those values such as Achievement and Section. In each case, our reference was the first exam, higher ACT students, and the terms assignment. Following best practices outlined we determined the best fixed-effects structure first without including random effects, and subsequently determined the best random-effects structure while holding the fixed effects constant (E. Theobald, 2018). To account for the fact that students took three exams across the semester, Student ID was included as a random effect. Instructor was also included as a random effect since the clustering of students within each course section means students within a section clearly share attributes that are not shared by other sections. The best-fit model was selected using Akaike’s information criterion (AIC).
RESULTS
RQ1 How Do Metacognitive Assignments Affect Exam Scores?
For higher ACT students (those that scored at or above the college median on the ACT), exam scores for those receiving the metacognitive assignments were similar for each of the three exams relative to those required to define terms ahead of exams (Figure 1A). Lower ACT students (those that scored below the college median on the ACT) scored higher on two of three exams when receiving the metacognitive assignment as compared with the assignment that required them to define terms (Figure 1B). When considering raw means and standard deviations, exam scores for those lower ACT students receiving the metacognitive assignment on both the second and third exams were just over 5 points higher on both 100-point exams (Second Exam Meta group = 80.2 ± 11.1 SD, Term group = 75.0 ± 10.7 SD; Third Exam Meta group = 82.8 ± 8.3 SD, Term group = 77.3 ± 9.1 SD). These differences can be considered small to moderate (Effect Size Second Exam; Cohen’s d = 0.48) and moderate to large (Effect Size Third Exam; Cohen’s d = 0.63).
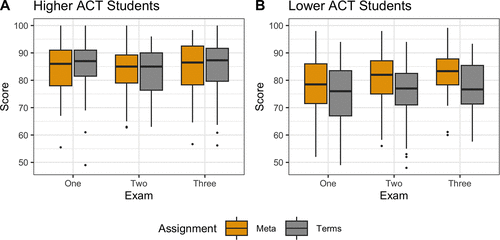
FIGURE 1. Exam scores across the semester for students receiving either metacognitive assignments (Meta) or terms to define (Terms). Higher ACT students (A) scored similarly regardless of the assignment they received. Lower ACT students (B) receiving open-ended metacognitive assignments scored higher than those receiving terms to define for exams two and three. Higher ACT (n = 151) and lower ACT (n = 82) students are those that scored at or above the median or below the median incoming ACT score for our institution. (The line in the box represents the median, the box represents the interquartile range (IQR) and the whiskers represent the lowest and highest data points no more than 1.5 times the IQR above and below the box. Data points not included in this range are represented as circles.)
The best-fit model for exam scores was determined by identifying the model with the lowest AIC (Table S5). The best-fit model for exam scores included Achievement (Hi/Lo ACT), Exam (First, Second and Third), Assignment type (Terms, Metacognitive) and an interaction between Achievement and Assignment Type as fixed effects. Instructor and Student ID were included as random effects. The best-fitting model included Student ID as a random effect to account for the fact that students took three exams across the semester.
The inclusion of an interaction effect between Achievement and Assignment in this model indicates that, all else being equal, it is only lower ACT students receiving the metacognitive assignment that improved their exam scores (Supplemental Table S8). Students who received the metacognitive assignments performed, on average, 0.51 points lower on their exams relative to those defining terms (β = −0.51, SE = 1.25, p = 0.68). Adding the interaction term to account for achievement indicates that students completing the metacognitive assignments scored, on average, 5.3 points higher on exams (β = 5.31, SE = 2.12, p = 0.01). Not surprisingly achievement itself has a large effect with lower achieving students as a group scoring more than 9 points lower than higher achieving students (β = −9.01, SE = 1.39, p < 0.01). There was no clear pattern for students as a whole (combined higher and lower ACT students) as they took each exam, given that for the second exam β = −0.57 (SE = 0.58, p = 0.32) and for the third exam, β = 1.06 (SE = 0.58, p = 0.07).
RQ2 How do Metacognitive Assignments Affect Exam Score Predictions?
When higher ACT students (those that scored at or above the median) were asked to predict the grade they expected to receive on the exam ahead of time, both those completing a metacognitive assignment and those defining terms ahead of the three exams across the semester had approximately similar predictions (Figure 2A). Lower ACT students receiving the metacognitive assignments also did not differ in their predicted scores relative to lower ACT peers that defined terms on any of the three exams (Figure 2B). Most of the adjustment in predicted scores for lower ACT students seems to have come between the first and second exams, where as a group these students dropped their average predicted scores from an average of 87.2 to 83.6 out of a total of 100 points for those defining terms and from 88.7 to 84.6 for those completing the metacognitive assignment, with virtually no further adjustments made ahead of the third exam.

FIGURE 2. Predicted exam scores across the semester for students receiving either metacognitive assignments (Meta) or terms to define (Terms). Higher ACT students (A) predictions were similar regardless of the assignment they received. Lower ACT students (B) receiving open ended metacognitive assignments did not clearly adjust their predictions relative to those receiving terms to define for any of the exams. Higher ACT (n = 151) and lower ACT (n = 82) students are those that scored above or below the median incoming ACT score for our institution. (The line in the box represents the median, the box represents the interquartile range (IQR) and the whiskers represent the lowest and highest data points no more than 1.5 times the IQR above and below the box. Data points not included in this range are represented as circles.)
The best-fit model for predicted exam scores was determined by identifying the model with the lowest AIC (Table S6). The best-fit model for exam scores included Achievement (Hi/Lo ACT), Exam (First, Second and Third), Assignment type (Terms, Metacognitive) and an interaction between Achievement and Assignment Type as fixed effect. Instructor and Student ID were included as random effects. The best-fitting model included Student ID as a random effect to account for the fact that students took three exams across the semester.
The inclusion of an interaction effect between Achievement and Assignment in this model indicates that it improves the model, all else being equal, however the effects are small (Supplemental Table S9). Predicted scores for students who received the metacognitive assignments were similar to those receiving terms, being lower on average by 0.08 points (β = −0.08, SE = 0.77, p = 0.91). In contrast to exam scores, adding the interaction term had no clear effect since lower ACT students receiving the metacognitive assignments adjusted their predicted scores by only 1.53 points (β = 1.53, SE = 1.31, p = 0.24). Not surprisingly, achievement itself affected predicted scores, with lower ACT students predicting scores 2.96 points lower than higher ACT students (β = −2.96, SE = 0.94, p < 0.01). Relative to the first exam students as a whole (higher and lower ACT students) adjusted their predicted scores, lowering their predictions for their second and third exams (by 3.05 points and 3.18 points, respectively). This pattern in predicted scores is indicated because β = −3.05 (SE = 0.36, p < 0.01) for the second exam and β = −3.18 (SE = 0.58, p < 0.01) for the third exam.
RQ3 How Do Metacognitive Assignments Affect Accuracy?
When accuracy (predicted-actual) was calculated for higher achieving students the assignments had little effect (Figure 3A). Higher ACT students were relatively accurate, having small positive values for accuracy. When raw means and standard deviations for accuracy were calculated for lower ACT students, the difference between those receiving the metacognitive assignment and those defining terms on both the second and third exam was ∼ 4 points on both 100-point exams (Figure 3B) (Second Exam Meta group = 4.4 ± 10.5 SD, Term group = 8.6 ± 8.3 SD; Third Exam Meta group = 2.0 ± 7.0 SD, Term group = 5.9 ± 9.5 SD). The larger positive scores of the terms group indicates that these students were more overconfident. These differences can be considered small to moderate (Effect Size Second Exam; Cohen’s d = 0.44, Effect Size Third Exam; Cohen’s d = 0.47). Overall, lower ACT students as a group were less accurate than higher ACT students since they had larger positive values for accuracy.

FIGURE 3. Accuracy (predicted−actual exam scores) across the semester for students receiving either metacognitive assignments (Meta) or terms to define (Terms). Higher ACT students (A) accuracy was similar regardless of the assignment they received. Lower ACT students (B) receiving open-ended metacognitive assignments were less biased on exams two and three relative to those receiving terms to define. Higher ACT (n = 151) and lower ACT (n = 82) students are those that scored above or below the median incoming ACT score for our institution. (The line in the box represents the median, the box represents the interquartile range (IQR) and the whiskers represent the lowest and highest data points no more than 1.5 times the IQR above and below the box. Data points not included in this range are represented as circles.)
The best-fit model for accuracy was once again determined by identifying the model with the lowest AIC (Supplemental Table S7). The best-fit model for accuracy included Achievement (Hi/Lo ACT), Exam (First, Second and Third), Assignment type (Terms, Metacognitive), and an interaction between Achievement and Assignment Type as fixed effects and Student ID only as random effects. The inclusion of Instructor did not improve the model.
The inclusion of an interaction effect between Achievement and Assignment in this model indicates that it improves the model, all else being equal (Supplemental Table S10). Once again, it is important to remember that a lower value indicates greater accuracy, meaning students are less mis-calibrated. Before accounting for achievement level, students who received the metacognitive assignments showed very small differences in accuracy compared with those defining terms (β = −0.39, SE = 1.02, p = 0.70). Adding the interaction term to account for achievement indicates that students completing the metacognitive assignments improved their accuracy by 3.68 points becoming less mis-calibrated (β = −3.68, SE = 1.71, p = 0.03). Achievement itself has a large effect with lower ACT students as a group being less accurate and thus more mis-calibrated (by 6.04 points). In other words, lower ACT students had lower accuracy (predicted-actual was higher) than higher ACT students (β = 6.04, SE = 1.23, p < 0.01). Relative to the first exam all students improved their accuracy for their second and third exams (by 2.48 points and 4.24 points, respectively). In this case β = −2.48 (SE = 0.69, p = < 0.01) for the second exam and β = -4.26 (SE = 0.69, p < 0.01) for the third exam. These values are negative because an initial large value for accuracy was reduced to a lower value, indicating greater accuracy.
While adding the interaction term to account for achievement indicates that students completing the metacognitive assignments improved their accuracy, we need to remember that accuracy is simply a function of the difference between exam scores and predicted exam scores. As previously noted, the inclusion of the interaction between Achievement and Assignment for predicted scores improved the model, but the effects were small and not significant. Given this lack of significance for predicted scores, greater accuracy may be simply a function of improved exam scores in lower ACT students, rather than large adjustments in predicted scores (Figure 4).

FIGURE 4. Exam scores (solid lines) and predicted exam scores (dashed lines) plotted side by side to highlight patterns across the semester for students in both achievement categories and students receiving both types of assignments (Meta = Metacognition Assignment, Terms = Terms Assignment). Included are both higher ACT students (A) and lower ACT students (B). Higher ACT (n = 151) and lower ACT (n = 82) students are those that scored above or below the median incoming ACT score for our institution. Lines connect median values for each assignment group and each exam. (The line in the box represents the median, the box represents the interquartile range (IQR) and the whiskers represent the lowest and highest data points no more than 1.5 times the IQR above and below the box. Data points not included in this range are represented as circles.)
DISCUSSION
Our results connect with two different but related areas of study. We first focus closely on the effect of our metacognitive and term definition assignments on exam scores and describe how our findings fit into research that considers the effects of metacognitive interventions on exam performance in college science classrooms. We then describe the effect of our assignments on the ability of students to predict their exam scores and on their accuracy, connecting these findings broadly to research on the ability of students to self-assess. While we share results for our higher ACT students, given our research focus was on improving the performance of students with lower incoming ACT scores, this discussion focuses mostly on that group.
RQ1 How Do Metacognitive Assignments Affect Exam Scores?
As expected, we found higher ACT students (those at or above the median ACT level at our college) performed equally well whether they were asked to respond to metacognitive questions ahead of their exams or to define terms and showed no clear shift in exam scores across the semester. Higher ACT students started the semester strong and finished strong. Since high achieving students are likely to have sophisticated metacognitive skills, it is not surprising that prompting more metacognition had little effect. Higher achieving students are also quick to reach a performance ceiling and are thus less likely to show an effect unless given challenging exams (Zohar and David, 2008).
Lower ACT students (those with ACT scores below the median at our college) receiving our metacognitive assignments scored higher on exams than those receiving terms to define, even though the terms they were assigned to define were sometimes used in questions on the exam (Figure 1). While there were no clear differences in scores on the first exam, ahead of which students had completed a single metacognitive or term definition assignment, by the second exam and third exams the lower ACT students receiving the metacognitive assignment showed moderate increases in exam scores compared with those asked to define terms. We should note that the metacognitive assignment before the second and third exams were different from the assignment before the first exam because by that point in the semester students had graded exams in hand to reflect upon. In addition to asking students about what material they were finding difficult and what strategies they might use to master material on the exam they were preparing to take, many of the open-ended questions asked students to reflect on the exam or exams they had already taken. Students in the metacognitive group were assigned to look over and review their graded exams, consider where they had lost points and whether they had done as well as they had expected. Although the differences we saw were moderate (an average difference of just over 5% on the second and third exams) the fact that we found lower ACT students showed improvements in their exam scores after completing the metacognitive assignments on the last two exams adds to the growing body of evidence suggesting that metacognitive approaches may better prepare students to take exams and thus can have positive effects on academic performance in college biology courses.
While our approach of assigning reflective assignments ahead of exams as students are studying was unique, previous research has found that introducing a metacognitive perspective has value in biology courses. For example, the addition of metacognitive related workshops or study sessions to biology courses seems to increase academic performance when those choosing to attend workshops are compared with those not attending workshops (Chaplin, 2007; Nordell, 2009) although such self-selection may leave open the possibility that students electing to participate were different from those that chose not to participate in terms of motivation. Osterhage (2019) avoided this self-selection bias by comparing two sections, one with an emphasis on self-evaluation and found that students performed better on the first exam in the section where self-evaluation was emphasized. Zhao et al. (2014) also avoided this self-selection bias by comparing two chemistry courses, one with a metacognitive workshop and found some evidence that students performed better on exams. Again, our study was slightly different because we compared two different assignments within each of several courses, an open-ended metacognitive assignment with an assignment specifically focused on course content (terms to define).
Other research on the role of metacognition in college biology classrooms has focused on assignments that asked students to reflect on their exams after they are handed back (exam wrappers). Two of these studies avoided self-selection bias by taking a more experimental approach. These studies show that students who are asked to reflect on where they have gone wrong are able to refine their response when asked questions on related material later (Mynlieff et al., 2014; Williams et al., 2011). However as Mynlieff et al. (2014) point out, it is often still the case that an assignment with a metacognitive component (reflecting on exam responses) is compared with the absence of an assignment. Thus, although both avoid self-selection bias, it may not be clear that increases in performance are due to the metacognitive aspect of the assignment (reflecting on previous answers and why they were wrong), or simply the added time students were spending looking over material.
Two studies worked toward controlling for time on task by assigning students to receive one of several different kinds of assignments completed after an exam or, in one case, a lab exercise. Lin and Lehman (1999) found students receiving a more metacognitive oriented assignment resulted in students being better able to apply the knowledge gained during a biology lab in a different context than students receiving other kinds of assignments. However, when Soicher and Gurung (2017) in a college psychology class controlled for time on task by assigning some students to simply review their exams after receiving them back while assigning others to complete a metacognitive exam wrapper, they found no effect of the metacognitive exam wrapper on exam grades. One difference between the exam wrappers used by Soicher and Gurung (2017) and our exam preparation assignments was that our assignments asked students to both review their previous exams and reflect on their study strategies as they were preparing for their next exam. This timing may make a difference in terms of improving exam scores, helping students make more explicit connections between how they studied for their previous exam and how they are currently preparing for their next exam.
RQ2 How do Metacognitive Assignments Affect Exam Score Predictions?
RQ3 How do Metacognitive Assignments Affect Accuracy?
In addition to monitoring students’ exam scores in the two assignment groups, we also had them predict their score ahead of each exam as they were completing their assignment and then calculated their accuracy by subtracting their actual score from their predicted score. We considered both exam score predictions and accuracy along with actual exam scores, because Callender et al. (2016) have highlighted that dissecting the relationship between these three has theoretical importance. For example, if students’ predictions of performance exactly track their actual performance, their accuracy will not change if both exam scores and predictions of exam scores shift to the same degree in the same direction.
Because our metacognitive assignments specifically asked students to reflect on how much they had studied, as well as whether they had done as well as expected on their previous exam, we expected lower achieving students but not higher achieving students completing these assignments would adjust their exam score predictions more than those assigned to define terms. As expected, we found higher ACT students (those at or above the median ACT level at our college) exam score predictions were not influenced by the assignment they completed (Figure 2). In contrast to our expectations, metacognitive assignments did not clearly affect exam score predictions in lower ACT students (those below the median ACT level at our college) relative to those defining terms for any of the three exams during the semester.
Therefore, despite being asked to reflect on their previous exam, whether they had done as well as expected on the exam, and to consider whether they had mastered the material they were about to be tested on, there was no evidence that the metacognitive assignments resulted in students lowering their predictions or being more realistic about the grade they would receive on the exam they were about to take. It is possible that our decision to contrast the metacognitive assignment with a term defining assignment affected our results. The term definition assignment may incidentally have been just as effective as the metacognitive assignment in helping students realize they did not know as much as they thought they did. This would have resulted in both groups adjusting their predictions equally.
We found small to moderate effects of the metacognitive assignments on accuracy, but only in lower ACT students (Figure 3). Lower achieving students receiving the metacognitive assignments were more accurate (predicted score – actual score) on the second and third exams. However, because there were no differences in predicted scores between those receiving the metacognitive assignments and those receiving terms to define, as highlighted above, the improvements in accuracy on the second and third exams are likely driven by improved exam scores (Figure 4). In other words, the shifts in accuracy we observed in lower achieving students receiving our metacognitive assignment do not seem to be a function of students becoming more realistic since they did not adjust their predictions relative to those defining terms. Instead, they may have been more directly a result of the improved performance of lower achieving students receiving our metacognitive assignment. This is also illustrated by the fact that the difference in exam scores between the metacognitive and terms groups in lower achieving students (∼ 5 points on the 100-point exam) closely corresponds to the difference in accuracy (∼ 4 points).
A similar pattern has been seen in other introductory biology and psychology classrooms where accuracy increases were largely a function of students’ performance improvements rather than students adjusting their predicted scores (Miller and Geraci, 2011; Osterhage et al., 2019; Osterhage, 2021). Others have found improving accuracy improves performance (Nietfeld et al., 2006), but that relationship may not be simple. For example, while research has found a general association in individual students between reductions in overconfidence and improved performance (Knight et al., 2022), these researchers also found that as a group, students did not become more accurate across a semester when they estimated their performance after taking exams and completed metacognitive reflections after receiving their exams back. The timing of assignments was different in our study since performance estimates and metacognitive questions took place as students prepared for an exam. These researchers also point out that little is required of a student when predicting a grade, so that it is possible that some students guess their grades without engaging in metacognitive awareness. Correcting the overconfidence of lower achieving students has been considered important, since students seem unlikely to put the appropriate amount of effort into learning content if they believe they already have an understanding of that material (Pintrich, 2002). Dunlosky and Rawson (2012) point out that overconfidence is likely to perpetuate underachievement because students will terminate studying before they have mastered the material they will be tested on.
The overconfidence in lower achieving students we observed here is strikingly common and a diversity of studies have consistently found this pattern (Bol et al., 2005; Bol and Hacker, 2001; Hacker et al., 2000; Isaacson and Fujita, 2006; Kruger and Dunning, 1999; Nietfeld et al., 2005). Overconfidence in lower achieving students is also common in introductory biology courses, whether students were estimating their grade right before taking an exam (Osterhage, 2021; Osterhage et al., 2019) or after taking an exam (Chaplin, 2007; Dang et al., 2018; Knight et al., 2022) or filling out knowledge surveys in an upper level biology course (Ziegler and Montplaisir, 2014). Similar to previous research we found that some individual higher achieving students were in fact slightly underconfident, predicting they were going to perform less well than they actually did (Hacker et al., 2000; Bol and Hacker, 2001; Dunning et al., 2003).
While our models were not structured to specifically examine patterns of change across the semester, graphing exam scores and predictions of exam scores suggests taking exams across a semester may have provided all lower ACT students the practice and feedback needed to improve their scores (Figure 4). In fact, it may only be when lower achieving students are surprised by a low grade on their first exam that they begin to adjust their expectations for studying (Knight et al., 2022). Increases in accuracy or calibration across a semester have been shown in several studies of introductory biology students. For example, Dang et al. (2018) found that lower performing students became more accurate by the end of the semester. Osterhage (2019) found that for the third and fourth exam during the semester, students predicted and actual scores were more strongly correlated suggesting that students as a group became more accurate over time. While Knight et al. (2022) in a genetics course found that students did not become more accurate across the semester when completing postexam reflection assignments and predicting their grade after taking their exam, but they did find that individuals that shifted their confidence levels from being over to underconfident showed improved achievement. Accuracy has been well researched in psychology courses. Hacker et al. (2008) found that high achieving students hit an accuracy ceiling, that the addition of incentives improved the accuracy of lower achieving students, but that reflection had no effect on accuracy. Miller and Geraci (2011) found that incentives of extra credit points were needed to improve the accuracy of lower achieving students over time, but only if they received additional feedback. These diverse results point to the need for more research on the factors that affect the complex relationships between student performance, predictions of performance and accuracy over time.
Our Approach in Context
Our approach of combining metacognitive questions with exam analysis and reflection questions into a single assignment distributed ahead of exams across the semester was quite different from previous research. Contrasting two kinds of assignments (metacognitive, defining terms) was an attempt to control for time on task since both assignments required students to complete an assignment related to the material they were learning, an approach that others have called for (Mynlieff et al., 2014). Because both assignments had potential value, we chose to assign students to one or the other treatments, dividing each class into two groups. This also assured that any incidental differences in the kinds of students that chose to sign up for one section over another would not bias the results (R. Theobald and Freeman, 2014). This approach also avoided any possibility of one section being viewed by students as the section doing something new or different. Although students clearly arrive at college differently prepared, all students had approximately equivalent college biology experience because this is the second biology course in an introductory sequence. Unlike some previous studies, we used ACT scores to divide students into lower and higher achievement groups, rather than using grades received on exams in the course. We chose this measure since it was perceived by faculty at our institution as more objective and generalizable than relying on other measures.
Finally, unlike some recent studies (Knight et al., 2022), we chose to distribute assignments ahead of the three exams since this is a time students often stop by our offices asking us what and how to study. The fact that students were also asked to predict their score before rather than after having taken the exam may take advantage of a student’s heightened attention as they are in the midst of preparing to take an exam. It may also be a time when students are better able to honestly reflect on their previous exam performance, consider where they went wrong and why and be motivated to build on that understanding to better prepare for the exam they are about to take. Our choice to have several assignments distributed across the semester was also based on concerns that students need more than a one-time metacognitive workshop or assignment (Nietfeld et al., 2006). The faculty involved also varied in the details of their classroom approaches, although most included some combination of lecture and active small group work. Moreover, our assignment required students to take a metacognitive approach, but the assignments were not prefaced with information on metacognition itself or what we know about its role in improving student learning. Faculty during an ACM Teagle Collegium on Metacognition (Ottenhoff, 2011) informally referred to this as stealth metacognition. We would expect that introducing the concept of metacognition in class, sharing with students research that has shown its effectiveness and explicitly teaching or modeling metacognitive skills would be valuable (Zohar and David, 2008; Sandall et al., 2014; Sebesta and Bray Speth, 2017; Soicher and Gurung, 2017).
Implications for Instructors, Limitations, and Future Directions
We expect these results may help faculty teaching busy introductory science courses as they face the decision of whether to replace assignments which may facilitate the mastery of practical and relevant knowledge (terms), with more open-ended metacognitive assignments. A metacognitive approach also has the advantage of putting students in charge of their learning and may prompt a shift towards a growth mindset since the assignments asked students to generate ideas as to how they could make changes to their study strategies to become more effective learners (Dweck, 2000). In addition, as Rodriguez et al. (2018) has pointed out, we need to systematically support students as they develop beneficial study practices, especially those that have historically been underserved in STEM disciplines.
Since our data were collected from students at a single small liberal arts institution, our results may not be applicable to other types of institutions enrolling students with fundamentally different academic backgrounds and with much larger course enrollments. In addition, improvements in exam scores for lower achieving students receiving the metacognitive assignments were moderate compared with students asked to define terms and our sample sizes are small relative to research often conducted at larger institutions. Because our approach combined exam reflection, with a reflection of study practices and reflection on previous performance estimates, we cannot know which of these was more important in influencing student exam scores or if all three together played a role. It is also important to note that we did not evaluate the responses to the metacognitive assignments, students were simply asked to respond to questions that we expected to require metacognitive skills. Examining students’ responses to our metacognitive assignments and exploring the quality of their responses would be valuable in the future. We also cannot know how much effort students put into each assignment, so while we expected both the metacognitive assignment and the assignment to define terms to take approximately the same amount of time, it is possible that the open-ended responses on the metacognitive assignment were more time intensive. Moreover, the fact that we asked students to predict their exam grade as they were completing their exam preparation assignments means it is not clear whether those predictions would have been different if they had made them just before they started their exam or immediately after finishing their exam. In fact, we cannot know the precise point in their study cycle students completed their assignments, since students had several days to complete them and they were due approximately a day and a half before each exam. Finally, while we chose to group students using ACT scores, these scores are clearly only one measure of achievement. Since many institutions no longer require such standardized testing, other measures may be more appropriate to use.
In the future it may be productive to consider how the addition of such metacognitive assignments affects students’ perceptions of faculty and faculty’s perceptions of students. Assigning open-ended exploratory questions may have signaled to students that faculty valued them as individuals. As Gasiewski et al. (2012) have pointed out, it is important that students not see faculty in introductory science courses as gatekeepers. We also found students wrote surprisingly honestly about their study habits and challenges, a perspective that may help instructors to become more aware of students’ voices and their students’ agency in learning (Dewsbury and Brame, 2019).
CONCLUSION
We found that the addition of short metacognitive assignments completed ahead of each exam in an introductory biology course resulted in moderate increases in the exam scores of lower achieving students (those with below the median incoming ACT score) relative to students assigned to define terms relevant to the exam they were preparing to take. Despite the fact that the metacognitive assignments asked students to reflect on their previous predicted scores, lower achieving students did not adjust their predictions relative to those that simply defined terms. The fact that lower achieving students receiving metacognitive assignments showed moderate improvements in exam scores relative to those asked to define terms without that metacognitive assignment affecting their predictions, indicates we may want to reconsider the emphasis we place on improving the accuracy or reducing the overconfidence of student predictions. While our metacognitive assignments did not affect predictions any more than assignments that asked students to define terms, lower achieving students as a group did adjust their predictions on their second and third exams after taking their first exam and receiving feedback.
Others have questioned the relationship between the ability to predict performance and achievement. While it is often suggested that students need to adjust their expectations in order to be motivated to study more and perform at a higher level, it may be that the connection is more complex. Isaacson and Fujita (2006) highlight that it is not clear whether the ability to predict exam performance facilitates student learning and point out that accurate predictions of knowledge may only exist after one has mastered a body of knowledge. Calendar et al. (2016) suggested that we may need to shift our perspective, since it may be more likely that improvements in performance precede or co-occur with changes in judgements about performance. While the lack of an ability to predict performance in our students frustrates many, efforts that focus on correcting students’ predictions and improving accuracy may be less important than we expected, especially for beginning science students facing the transition from high school.
These results may serve as a reminder that a metacognitive framework highlights the importance of diverse metacognitive knowledge and regulation skills (Schraw and Moshman, 1995; Stanton et al., 2021). While our metacognitive assignments did not lead lower achieving students to adjust their predictions (a metacognitive knowledge skill), predicting performance or knowing what you know is only one aspect of metacognition. Improved performance is also likely to be a function of a student’s ability to plan (a metacognitive regulation skill), to monitor (a different metacognitive regulation skill), to understand their own thinking processes (a metacognitive knowledge skill) and to understand when and how to use different learning strategies (also a metacognitive knowledge skill). Given that predictions of performance did not change relative to those defining terms in lower achieving students, it would be valuable to explore whether the open-ended metacognitive prompts led students to shifts in these other aspects of metacognition, something we did not focus on in this study. These findings alongside other recent research (Knight et al., 2022) indicate the need for more research tracking actual exam scores, predicted exam scores and accuracy simultaneously across a semester. Such research may continue to guide the kinds of assignments busy introductory biology faculty choose to implement in their courses as their students face the challenging transition to college exams.
ACKNOWLEDGMENTS
This research was originally inspired by discussions during the Teagle-funded Collegium on Student Learning led by the Accredited Colleges of the Midwest and by work with students as part of an NSF S-STEM Biologists for the Future grant to A. W., D. A., and K. G. (NSF Award # 0727556). This work would also not have been possible without the participation of introductory biology faculty at St. Olaf College as well as a team of Student Fellows (Kelly Hennessey and Sam Bailey-Seiler) within the Center for Interdisciplinary Research at St. Olaf (NSF Program to Enhance Mathematical Science for the 21st Century DMS-1045015 and DMS-0354308). We are grateful to Mary Walczak for providing valuable feedback on this paper.