“I don’t Know what I Would do Without it” How Life Science Graduate Students Describe Resource Value
Abstract
Graduate students often face choices about which resources to use to help them succeed in their programs. These choices likely differ among students, in part, due to different perceptions of resource value. However, little is known about why particular resources might be considered highly valuable to students, thus driving choice. Utilizing expectancy-value theory for help sources as our theoretical framework, this qualitative study explored life science (LS) graduate students’ top three resource choices, their explanations about why they made those choices, and whether students’ perceptions of value differed among resources and across demographic groups. We addressed two research questions: 1) What resources do LS graduate students consider to be the most important? 2) What drives LS graduate students’ perceptions of resource value? Many participants indicated that ‘advisor’ and ‘academic stipend’ were most important. Student perceptions of value were driven by their perceptions of which needs resources fulfilled, such as basic needs, academic help, or support. Participants’ top resource choices and underlying values of those resources did not differ among demographic groups. We propose a model for understanding graduate student resource choice that may inform future work on student outcomes.
INTRODUCTION
Graduate students have access to myriad resources within their programs (e.g., faculty members, university research facilities, and online forums) that help them achieve success. A resource can be any item, person, or place that facilitates an outcome, and the use of resources underlies many of the integral moments of graduate study. For example, a student may use an advisor to develop a project, research equipment to collect and analyze data, or presentation software to give a thesis defense. Because students make individual choices about what resources they think they need, each student is likely to use a different set of resources throughout their programs (Weatherton and Schussler, 2022). Previous work in undergraduate populations has shown that differential use of single resources, like advisor or family members, lead to different academic (Gisemba Bagaka’s et al., 2015; Sverdlik et al., 2018) and affective outcomes (Breitenbach et al., 2019; Blanchard and Haccoun, 2020). Knowing that overall resource choice varies across students and that differential resource choice leads to differential outcomes in undergraduates (e.g., Stites et al., 2021), it is likely that graduate students’ resource choices may be similarly related to variation in outcomes like academic success and programmatic persistence. To understand relationships between resource use and differential outcomes, we first need to know why students select the resources that they use and the extent to which these resource choices vary among students.
Across domains of study, researchers have been fascinated by the question of what drives individual choice; different theories have posited that aspects of expectations, perceptions, needs, and beliefs all may impact decisions (e.g., expected utility theory, Allais and Hagen, 1979; optimal foraging theory, Pyke, 1984; prospect theory, Mercer, 2005). This study draws on one of these theories, the expectancy-value theory for help sources (EVTHS). EVTHS is an adaptation of expectancy-value theory and was created specifically to explain why students utilize help sources (e.g., resources; Makara and Karabenick, 2013), making it well-suited as a framework for research on students’ perception of resources. EVTHS posits that students’ choice of resources is impacted by their perceptions related to each resource; these perceptions can be broken into two categories: 1) expectations and 2) values. For example, as students see a resource more favorably (i.e., students viewing a resource as higher quality [value], or more accessible [expectation]), they’re more likely to choose to use that resource (Makara and Karabenick, 2013). Previous research in undergraduate populations supports this relationship (e.g., Meece et al., 1990; Yu et al., 2018; Scoulas and De Groote, 2022) and our quantitative analyses showed preliminary support for a relationship between graduate students’ perceptions of value and their resource choices (Weatherton and Schussler, 2022). However, because “value” includes many distinct components, our previous work was unable to identify the underlying reasons for students’ perceived value.
Understanding what drives student perceptions of value, and consequently resource choice, is important not just in theory, but also in practice, because of how resource choice might be influenced by others and the potential outcomes of these choices for individual students. For example, many mentors within science, engineering, technology, and mathematics (STEM) domains rely on their own experiences when recommending resources to students. While these assumptions are founded on experience within academia, previous research has shown that graduate students’ perceptions and choice of resources is related to demographic variables, like their racial and gender identity (Weatherton and Schussler, 2022). As such, assumptions made by leaders within STEM, who are majorly white or Asian males (National Center for Science and Engineering Statistics, 2021), may not align with the different needs of the STEM student populations, which are increasingly diversifying in terms of racial and gender identity (National Center for Science and Engineering Statistics, 2021). Furthermore, given extant issues with retention in STEM graduate programs, it is critical to understand the potential relationship between resource choice and graduate student outcomes, like persistence (Okahana et al., 2018). However, before we can explore potential misalignments or outcomes, we first need to know basic information about which resources are most valuable to students and why they value them. Thus, this exploratory, qualitative study examined life science (LS) graduate students’ perceptions of value about their top resource choices.
Expectancy-Value Theory for Help Sources
This study utilized EVTHS as its theoretical framework to inform research questions, methodology, and interpretation of results (Luft et al., 2022). Proposed by Makara and Karabenick (2013), EVTHS states that students’ help-seeking outcomes (i.e., resource choice) are driven by students’ expectations and values for any given resource. Specifically, the model includes two components of prospective expectations: 1) perception that the resource will be available and accessible, and 2) perception that the resource will provide help. Value, as outlined by EVTHS, has four components: 1) the type of help provided, 2) the relevance of help provided, 3) the quality of help provided, and 4) the accuracy of the help provided (Figure 1). Within EVTHS and its theoretical predecessor, expectancy-value theory, expectations and values are hypothesized to be positively correlated, and empirical work supports this claim (Wigfield and Eccles, 2020). Previous work has argued that expectations and values may interact in a multiplicative way (Nagengast et al., 2011; Trautwein et al., 2012), though other studies have refuted this claim and many in the field continue to use an additive model of expectations and values (Barron and Hulleman, 2015; Eccles & Wigfield, 2020; Schnettler et al., 2020). These conflicting results may suggest that perceptions and values are conceptually very strongly linked and may even overlap in participants’ minds; though, regardless of their conceptual overlap, both expectations and values have been repeatedly shown to impact students’ achievement, persistence, and other academic outcomes (see Wigfield et al., 2009 for review).

FIGURE 1. Adapted model of Makara and Karabenick's EVTHS (2013). Model components outlined in red are the focus of this study.
The theoretical backing of EVTHS has been repeatedly tested within populations of undergraduate students and has demonstrated evidence of validity. For example, Evenhouse and colleagues (2020) indicated that undergraduate students’ expectations of resource availability were important drivers of resource preferences; students in this study preferred to use resources which were readily accessible, such as online discussion forums and search engines, as opposed to homework solutions posted after corresponding assignment due dates or office hours, highlighting the impact of expectations of availability on student resource choice. Similarly, Tinsley and colleagues (1982) demonstrated a pattern of resource usage in undergraduate students based on the type of help they were seeking; for example, students were more likely to turn to close friends for personal help, while they would more likely ask instructors for help with career problems. These studies highlight the empirical support for the relationship between expectations, values, and resource choice in undergraduate students. Within graduate students, our previous work has shown preliminary support for EVTHS, where LS graduate students’ perceptions of resource usefulness (i.e., value) and frequency of use were positively correlated (Weatherton and Schussler, 2022).
The present study sought to further examine the applications of EVTHS within a graduate student population by qualitatively exploring student perceptions of value. Our previous work quantitatively examined one aspect of value, students’ perception of resource usefulness, using Likert-scale survey responses (i.e., ‘how useful is this resource from 1–5?’; see Weatherton and Schussler, 2022) across a list of 34 resources. To more deeply understand the nuances of student perception of value, this study asked students to choose their top three resources and explain why they felt each was valuable. While expectations and values have been shown to be strongly correlated and may potentially interact multiplicatively (see Trautwein et al., 2012; Wigfield and Eccles, 2020), we chose to focus solely on value to attempt to elucidate the individual impact of this component on students’ resource choice.
Previous Studies of Graduate-Student Resource Choice
For decades the field of graduate education has underscored the importance of a handful of resources: students’ advisors, departmental structures, and financial aid (Girves and Wemmerus, 1988; Tinto, 1993; Lovitts, 2002; Sverdlik et al., 2018). Much of this work has focused on the relationship between the use of certain resources and student success (e.g., the use of “thesis supervisors” resulted in greater academic success; Garcia et al., 1988). However, relatively few studies have examined graduate students’ perceptions and choices among the resources available to them beyond academic advisors. In this brief review of the literature, we will highlight research on graduate students’ use and perceptions of social and material resources.
Social Resources.
Social resources are integral to student success and well-being and as such, have been central to studies of graduate education for decades (Tinto, 1993; Lovitts, 2002; Sverdlik et al., 2018; Griffin et al., 2020; Wofford et al., 2021). Resources that fall into this category are diverse and include both academic and nonacademic relationships, like advisors, labmates, significant others, and family members, among others. In particular, the student–advisor relationship is often heralded as the most important factor in the graduate school experience and thus has been extensively studied (Sverdlik et al., 2018). A students’ choice of advisor is driven, in part, by their values related to the advisor (e.g., quality of advisor’s reputation, type of help provided by advisor), validating the relationship between students’ perceptions and their resource choice (Zhao et al., 2007). Students’ primary advisors facilitate many goals, including academic, personal, affective, and career goals (Lovitts, 2002; Zhao et al., 2007; Blanchard and Gisemba Bagaka’s et al., 2015; Haccoun, 2020; Tuma et al., 2021). However, for many students, including minoritized students (i.e., those historically excluded from higher education on the basis of gender identity, sexual orientation, race, or ethnicity), perceptions of support from peers or family members are often greater than or equal to the support provided by primary advisors (González, 2006; Allen and Joseph, 2018; Holloway-Friesen, 2021). For example, a study of minoritized graduate students’ social networks found that their social support came primarily from minoritized peers (e.g., lab mates, other graduate students; Tullis and Kowalske, 2021). Furthermore, graduate students have previously cited the importance of family members and significant others for providing psychosocial (e.g., emotional support, motivation) and material (e.g., financial support, childcare) support (Breitenbach et al., 2019). These findings highlight the importance of social resources beyond advisors, as well as evidence for potential misalignments between a theoretical understanding of graduate student resource choice (i.e., the student–advisor relationship is one of the most important sources of support in academia), and the lived reality of many graduate students, who may perceive other resources as equally or more valuable.
Material Resources.
Material resources, such as lab equipment or grant funding, provide some of the most basic support for graduate students’ progress through their degrees, though these resources are often overlooked in empirical examinations of student success (Knight et al., 2018; Sverdlik et al., 2018; Wollast et al., 2018). Our recent work within a population of LS graduate students found that students reported material and financial resources – like academic stipend, online academic journals, electronic resources, and grants – to be highly useful and frequently used (Weatherton and Schussler, 2022). However, many students report that the material resources they receive to be inadequate; in a recent survey of chemistry graduate students, almost half of respondents (46%) reported the need for greater support, both financial (e.g., greater stipends) and physical (e.g., updating lab equipment or infrastructure; Stockard et al., 2022). While it is clear that material resources are important to students, it is unclear whether all material resources are equally valuable and how students choose between the many material resources available to them.
Current Study
Clearly, there are a number of resources which graduate students choose to use and perceive to be highly valuable, but it is unclear what drives student perceptions of resource value and whether perceptions of value may vary across resources. The present study utilized the same national sample of LS graduate students as our previous work, which examined which resources students used in their programs (Weatherton and Schussler, 2022). The previous study asked students to rate the usefulness of each of up to 34 resources using Likert-scale survey responses. The present study asked about students’ three most important resources and collected data about perceptions of value using free-response answers and rich, qualitative data. By doing so, we aimed to better understand drivers of student resource choice, which may ultimately impact graduate student outcomes. This study had two research questions: 1) What resources do LS graduate students consider to be the most important? 2) What drives LS graduate students’ perceptions of resource value?
METHODS
Data Collection
Data for this study were collected during the Fall of 2021 as part of a national survey of LS graduate students. For more details about the survey and recruitment information, see Weatherton and Schussler (2022). This study was approved by the Institutional Review Board before data collection (IRB #20-05870-XP).
The survey collected data about what resources students used in their graduate program, how often they used these resources, and how they perceived these resources. Data for this study came from two survey questions, which asked students to choose the three resources that were the most important to them (“Of the resources that you use, choose the three most important resources to you”), and then asked, for each of the three resources, why it was selected (“Please describe why you feel that this resource is important”). Students did not rank these resources in terms of importance, they simply chose the three resources most important to them.
These two free-response questions underwent two rounds of pilot testing (N = 32 students) to ensure that the questions were interpreted similarly across students and that the wording of the questions was not confusing (Weatherton and Schussler, 2022). Pilot testing showed that “importance” was a more understandable word than “value” for students in our population, so we used “most important” in our survey questions to refer to the concept of high-value resources. This study sought to collect qualitative data focused on students’ perception of resource value, not their expectations, and thus, we only pilot tested whether “importance” was an adequate proxy for the concept of resource value, though it may have captured some aspect of students’ expectations of the resource as well (see Limitations). Given this, we were attentive to all student responses in this study, including those that may not have fallen under the strict definition of value.
At the end of the survey, participants were asked a suite of demographic questions, which they could choose to skip. The questions gathered the following data: participants’ gender identity, race, year in their graduate program, and college generation status (i.e., whether they identified as first-generation college students or not).
Data Analysis
Data Cleaning.
We received 668 responses from LS graduate students across the United States. One hundred and thirty five responses were removed because they did not complete the survey. We also removed 18 survey responses that did not answer the free-response questions and five responses from participants who selected more than three important resources. Eighteen uncodeable responses were removed during the process of data analysis. Thus, the final sample size for this study was 492. We analyzed and visualized our results in R Studio (version 1.2.5) using the base (Rstudio team, 2020), ggplot2 (Wickham, 2016), and ggpubr (Kassambara, 2022) packages.
Qualitative Analysis.
Two researchers (M.W. and B.V.) conducted qualitative analysis on participant explanations about why they chose their three most important resources (1533 free responses). We followed a basic qualitative analysis procedure, in which responses were first inductively coded (open coding), similar codes were combined (axial coding), and then codes were sorted into broader categories (i.e., themes; Merriam and Tisdell, 2015; Elliott, 2018; Skjott Linneberg and Korsgaard, 2019). Throughout the process, both researchers made a conscious effort to “bracket,” or set aside, their preconceptions of what may drive student perception of value and the study’s theoretical framework, in order to examine the responses as impartially as possible (Patton, 2014).
Each researcher independently read all student responses to conduct their initial open coding, and researchers used descriptive coding methods to note code(s) that summarized main ideas from students’ responses. After initial open coding, the researchers met to discuss their codes and develop a codebook based on codes they agreed were represented in the data. While the original intent of the study was to capture students’ perceptions of resource value, during analysis we noticed that participants also often discussed their expectations of resources. In accordance with a qualitative, constructivist epistemology (i.e., that there is no one “correct” answer to survey questions; Merriam, 2009) and our inductive coding process, we decided to keep all student responses, regardless of whether they discussed resource value or not. The initial codebook was then tested independently by each researcher on the same set of 100 randomly selected responses; the codes for these responses were then compared to ensure an adequate level of interrater agreement (i.e., the degree to which raters consistently distinguish between different responses; Hruschka et al., 2004; Gisev et al., 2013; Patton, 2014). Interrater agreement is a critical component of qualitative coding as it enhances the consistency and reliability of qualitative work, which is inherently liable to coders’ subjectivity. Interrater agreement was calculated by dividing the percent of responses that the coders agreed on by the total number of responses. While responses could receive multiple codes, agreement was only met when all assigned codes for that response matched between the two coders. After a round of testing, the codebook was then modified based on researchers’ discussions. This process was repeated until interrater agreement was consistently above 70% on sets of 100 responses, after which both researchers independently coded the entire dataset (Hruschka et al., 2004).
Researchers did not code responses under three words (e.g., “useful”, “my income”), or responses that were too vague or missing too many words to understand. Researchers did not code responses that described the resource itself as opposed to answering the question (e.g., [Research collaborators] “collaborations/publications”; [University library] “Covers the majority [sic] of papers from past to present”). After removing these uncodeable responses, the final dataset included 1262 student responses from the 492 participants.
After both researchers independently coded the entire data set, the primary author (M.W.) identified any disagreements in the two sets of codes. The final agreement percentage was 73%. The researchers then reconciled all coding conflicts via discussion, so that codes were 100% consistent between the two coders (Hruschka et al., 2004; Gisev et al., 2013). After this stage of coding was completed, the primary author (M.W.) sorted codes into broader themes, and these themes were discussed with a group of education researchers. The final themes and codes, along with descriptions and examples of each code from participant comments, are shown in Table 2.
Post hoc Demographic Analyses.
Given previous work showing that students’ resource choices and perceptions of resources may be impacted by their demographic backgrounds (e.g., Wigfield and Eccles, 2000; Tullis and Kowalske, 2021; Weatherton and Schussler, 2022), we analyzed whether there were differences in which resources were chosen, as well prevalence of qualitative codes across the following demographic groups: race (i.e., nonwhite and white), gender identity (i.e., men, women, and gender nonbinary individuals), year in program (i.e., first two years and year three plus), and college generation status (i.e., first- and continuing-generation students).
Code prevalence was calculated for each resource by taking the number of times a code was present in the responses of a certain demographic subgroup divided by the total number of times a code was present in all responses (e.g., number of times white participants mentioned advisor using the “academic” code divided by the total number of times advisor was mentioned using the “academic” code). Prevalence was then compared within demographic subgroups (e.g., comparing the prevalence of each code between white and nonwhite students) using chi-squared tests (Turhan, 2020). Because the number of participants selecting each resource as their most important varied considerably (see Supplemental Table S3), we chose to run chi-squared tests only for the three most prevalent resources in the data set: academic stipend, advisor, and significant other.
Validity
As opposed to quantitative research, qualitative research uses nonnumerical information, such as individual perceptions, experiences, and cognition, as data. The topics are inherently subjective – they are influenced by the personal feelings or opinions of the research participants – and the process of interpreting participants’ experiences via a research team adds another layer of subjectivity and potential bias. Participant-based subjectivity is an essential and inevitable aspect of qualitative work, while researcher-based subjectivity can add important context and nuance to research findings (Leung, 2015). Our goal as qualitative researchers is to orient readers to this subjectivity and reduce our own biases as much as possible (Leung, 2015; Merriam and Tisdell, 2015). Thus, we undertook several precautions to reduce our biases and ensure the reliability and rigor of our work.
First, we used a method of constant comparison when creating the codebook to ensure that the codebook accurately reflected students’ free-response answers, thereby working to ensure the consistency and accuracy of our results (Leech and Onwuegbuzie, 2007; Patton, 2014). Throughout the process of analyzing and interpreting the data, we aimed to understand and bracket the biases that may influence our interpretation of the data, and this was done through a combination of memo writing and peer debriefing (Patton, 2014). We aimed for a high interrater score when conducting our analyses to establish the reliability of our results. We did not conduct a full analysis of the data until the two coders (M.W. and B.V.) consistently agreed more than 70% of the time, the standard for the field (Hruschka et al., 2004; DeCuir-Gunby et al., 2011). Ensuring that the interrater agreement was both consistent and high provides assurance that individual researchers’ biases have a minimal impact on data analysis and that the codebook was functional for our data. Finally, we used a noncoding expert (E.S.) to conduct a critical review of the codebook and interpretation of the data, which further increases the rigor of analysis and interpretation.
RESULTS
Participants
Participant demographics are shown in Supplemental Table S1 (N = 492). A majority of participants were in the first three years of their LS graduate program (65%). A majority of participants identified as women (70.7%) and white (71%). Our sample was approximately split between participants who identified as first-generation college students (51%) and continuing-generation students (47.6%).
RQ 1: What Resources do LS Generation Students Consider to be the Most Important?
Of the 34 resources available for participants to choose from (Weatherton and Schussler, 2022, Supplemental Table S2), 31 resources were selected at least once by participants as one of their top three most important resources. Three resources – international student center, publishing funds, and university sponsored events – were not chosen by any participants.
“Advisor” was selected as a top three choice by the most participants (67.68%), followed by “academic stipend” (56.71%), and “significant other” (24.60%). Resources chosen least often by students included “alumni network”, “departmental graduate-student association”, “departmental seminars”, “social media”, “university career center”, and “university-sponsored workshops”, which were all chosen once (Table 1). These trends stayed relatively consistent when broken down by demographic variables (Table 2). As shown in Table 2, “advisor” was the most important resource for participants regardless of their demographic characteristics, followed by “academic stipend”. “Significant other” was the third most-often chosen resource for all demographic groups except men, whose third most chosen resource was “online academic journals”. Also of note, for participants who identified as gender nonbinary (n = 20), “significant other” and “labmates” were tied for third most frequently chosen resource.
Resource | Frequency | Percentage |
---|---|---|
Advisor | 333 | 67.68% |
Academic stipend | 279 | 56.71% |
Significant other | 121 | 24.59% |
Online academic journals | 100 | 20.33% |
Lab mates | 96 | 19.51% |
Friends | 82 | 16.67% |
Other graduate students | 70 | 14.23% |
Electronic resources | 68 | 13.82% |
Family members | 45 | 9.15% |
Therapist or other mental health counselling | 45 | 9.15% |
University courses | 39 | 7.93% |
Research collaborators | 36 | 7.32% |
University-provided research facilities | 34 | 6.91% |
Grants | 27 | 5.49% |
Departmental faculty | 21 | 4.27% |
University health center | 20 | 4.07% |
University gym | 10 | 2.03% |
University library | 10 | 2.03% |
Previous mentors | 8 | 1.63% |
Conferences | 6 | 1.22% |
University transit system | 5 | 1.02% |
Travel funds | 4 | 0.81% |
Special interest student organizations | 3 | 0.61% |
Departmental administrators | 3 | 0.61% |
University writing center | 2 | 0.41% |
Alumni network | 1 | 0.20% |
Departmental graduate student association | 1 | 0.20% |
Departmental seminars | 1 | 0.20% |
Social media | 1 | 0.20% |
University career center | 1 | 0.20% |
University sponsored workshops | 1 | 0.20% |
Resource | Demographic characteristic | Relative percent within demographic category | Number of participants (N) |
---|---|---|---|
Advisor | Nonwhite | 66.20% | 94 |
White | 68.29% | 239 | |
Continuing-generation | 69.66% | 163 | |
First-generation | 64.94% | 163 | |
Woman | 67.53% | 235 | |
Man | 66.94% | 83 | |
Years 1–2 | 67.54% | 154 | |
Years 3+ | 67.80% | 179 | |
Academic stipend | Nonwhite | 52.11% | 74 |
White | 58.57% | 205 | |
Continuing-generation | 55.13% | 129 | |
First-generation | 58.17% | 146 | |
Woman | 58.05% | 202 | |
Man | 49.19% | 61 | |
Years 1–2 | 55.26% | 126 | |
Years 3+ | 57.95% | 153 | |
Significant other | Nonwhite | 26.76% | 38 |
White | 23.71% | 83 | |
Continuing-generation | 22.65% | 53 | |
First-generation | 25.90% | 65 | |
Woman | 27.59% | 96 | |
Years 1–2 | 24.12% | 55 | |
Years 3+ | 25.00% | 66 | |
Online academic journals | Man | 20.16% | 25 |
RQ 2: What Drives LS Graduate Students’ Perception of Value?
When asked to describe why their three selected resources were important, student free-response answers were grouped into nine codes, and these codes fell into two themes, “resource attributes” and “help provided” (Table 3). These theme names were guided by the nature of the codes and not EVTHS constructs; we also note that the codes that emerged from student responses encompassed both value and expectations.
Theme | Code | Description | Example | Frequency (N = 1816 codes) | Percentage |
---|---|---|---|---|---|
Resource attributes | Availability | Participants’ expectation of the resources’ convenience, ease of use, or accessibility | [Advisor] …She is always available when I need her | 43 | 2.36% |
Validity | Participants’ expectation that the resource is helpful, knowledgeable, trustworthy, or correct in terms of information or advice | [Departmental faculty] Faculty are the experts in our department so they are a valuable resource of knowledge. | 81 | 4.46% | |
Essential | Participant describes their perception that the resource is a necessity, or otherwise provides a prerequisite to some aspect of their graduate program. | [Academic stipend] Would not be feasible to complete my degree without this. | 59 | 3.25% | |
Help Provided | Basic need | Resources fulfills some aspect of participants’ requirements of living or survival, including food, shelter, security, healthcare, etc. | [Academic stipend] To survive day-to-day and pay my bills. I need to pay rent and eat. | 236 | 13.0% |
Connection | Resource links the participant to other resources | [Advisor] My advisor can connect me with the resources I need but don't know about | 77 | 4.24% | |
Academic | Resource helps student with some aspect of their academic endeavors – including research or degree progress and career pursuits | [Electronic resources (e.g., specific research software, R, mendeley)] Could not explore and analyze my data without them. | 585 | 32.21% | |
Persistence | Resource motivates or otherwise provides affective assistance in student retention in their program | [Other graduate students] My motivation to finish comes from my peers | 78 | 4.30% | |
Support | Resource provides student with some sort of mentoring, guidance, or help with problem solving | [Advisor] Overall guidance through the process of my graduate education. | 459 | 25.28% | |
Wellbeing | Resource supports students’ emotional health by reducing stress, offering community, or providing emotional support | [Friends] Friends help to maintain work-life balance and have a life outside of work | 267 | 14.70% |
Resource attributes included any codes where students described their perceptions of the characteristics or nature of the resource that made it valuable to them. Responses that were coded under the “resource attributes” theme discussed the resource itself, as opposed to the outcomes produced by the resource (as in the help provided theme). Within resource attributes, there were three codes, “availability,” “validity,” and “essential,” which were coded 43, 81, and 59 times, respectively (out of 1816 total codes assigned).
The theme “help provided” included six codes where participants described what they used a resource for or what the resource provided for them, these were “basic needs,” “connection,” “academic,” “persistence,” “support,” and “wellbeing.” Overall, academic and support codes were the most frequently used, with academic being coded 585 times (out of 1816 codes) and support being coded 459 times. Following these codes, “wellbeing” (267) and “basic needs” (236) were the next most common, with all other codes in the “help provided” theme being assigned less than 100 times (Table 3). Resources often provided many different types of help and thus, many participant responses were assigned multiple codes.
Participant responses often included codes from both themes (Figure 2). For example, when describing why previous mentors were an important resource, one participant said, “(previous mentors are) invaluable resource for research questions; often more accessible or responsive than advisor or committee members.” This quote includes both a code from the “resource attribute” theme (availability) and describes the “help provided” by the resource (academic). However, 10 resources were solely described in terms of the help they provided to students (Figure 2). Figure 2 shows each of the resources that participants discussed and the proportion of codes representing the resource attributes or help provided themes. Below we will discuss each theme in depth and examine how codes within these themes differed across resources.

FIGURE 2. Prevalence of “resource attribute” and “help provided” themes across resources.
THEME 1: Resource Attributes.
The code “availability” was used 43 times and referred to participants’ perceptions of a resources’ convenience or accessibility. For example, participants often discussed that a resource was important to them because it was always available to help, “My advisor…is consistently available to help answer my questions or concerns.” Both social and material resources (e.g., advisor, electronic resources, family members, university library) were often described using availability, when students described that they interacted with these resources often, or they could rely on them to be available for help (Figure 3). The resource most often associated with this code was advisor, which participants described as being important because they were often available when they needed them, or they met frequently.
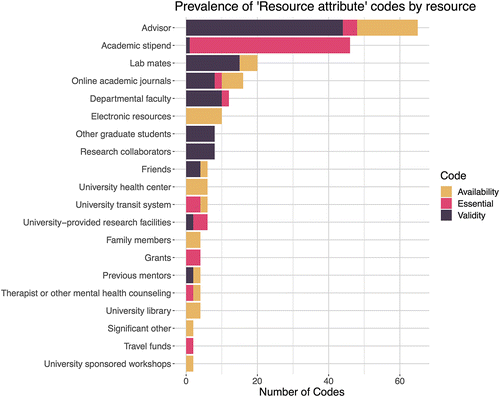
FIGURE 3. Prevalence of each code (by frequency) within the “resource attributes” theme for each resource.
The code “validity” was coded 81 times and referred to participants’ expectation that a resource would give out correct, helpful, or trustworthy information. For example, a participant discussed the validity of online academic journals, “I can find the answer to my questions from this reliable source.” Advisor, department faculty, research collaborators, and labmates were the resources most frequently described using the validity code, meaning that students felt these resources were important because of their ability to give them helpful or trustworthy information. Of those, advisor was most often associated with the code of validity (Figure 3).
The code “essential” referred to participants’ expectation that they could not complete some aspect of their program without the resource (i.e., it was essential to their graduate program or success generally) and was coded 59 times. This was often coded when participants discussed that without a certain resource, they wouldn’t be able to continue in their program, for example, many participants discussed the necessity of their academic stipend, “Without the stipend I would not be in school,” “Without it, a PhD was not affordable in my case.” As opposed to the “basic need” code, essential was used when participants described that they needed a resource but did not specify that it fulfills a basic need (e.g., food, shelter, paying bills). Monetary resources (e.g., travel funds, academic stipend, grants) were often described by the “essential” code, as well as university transit system, indicating that students perceived that these resources were integral to their persistence and success in their graduate program (Figure 3). By far, the resource most associated with the code of need was academic stipend, which participants often discussed in terms of this resource allowing them to focus on their academic work or attend their program.
THEME 2: Help Provided.
The code “basic need” was used whenever participants described that a resource provided some form of food, shelter, security (physical or positional), or similar. “Basic need” was coded 236 times. Researchers used the list of basic needs used by the Human Needs Index to identify what needs constituted a basic need (Salvation Army, 2020). Resources that provided basic needs were often monetary resources that afforded students the ability to pay for rent or buy food. For example, a student described the importance of their academic stipend in this way: “My stipend is important because being a graduate student is my job. Therefore, I need financial resources to be able to stay afloat by paying rent, for food, etc.” By far the resource that was most often mentioned in relation to basic needs was academic stipend, followed by mental health counseling and the university health center (Figure 4).
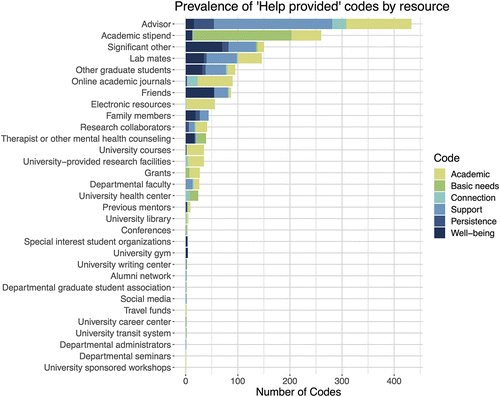
FIGURE 4. Prevalence of each code (by frequency) within the “help provided” theme for each resource.
The code “connection” was used whenever participants described that a resource connected them to other resources or provided them with access to other resources. “Connection” was coded 77 times. Students described that some resources were valuable because they allowed them to access other resources, as this participant described, “(University library) Allows me to search for and read relevant journals that would otherwise be inaccessible.” Furthermore, advisors or faculty members were valuable to students as they often connected them to resources that they were otherwise unaware of, “My advisor provides opportunities that cover many of the items that are important. So with a good advisor, it won’t matter what the institution provides, they’ll help you find the resources you need.” “Advisor” and “online academic journals” were the resources most often coded with connection (Figure 4).
The code “academic” was the most prevalent code in the data set and was identified 585 times; this code was used whenever participants described that a resource was important to them because it helped them accomplish an academic- or career-related task (e.g., analyzing their data, learning a new procedure, or brainstorming dissertation ideas). For example, when describing the importance of university research facilities, a student said: “These resources enable my data generation and my research would not be possible without them.” In the same way that material resources, such as research facilities, help to accomplish academic goals, participants described that social resources, such as labmates, were equally helpful in accomplishing academic tasks or learning new skills, “I have a very experienced laboratory scientist in the lab who has been instrumental in my lab skill development and thesis.” Many of the resources surveyed provided academic help to participants, and “advisor” was the resource most often coded using the “academic” code (Figure 4).
The code “persistence” was coded 78 times and was used whenever participants described a resource helping them fulfill an affective need related to completing their degree. As opposed to the “academic” code, wherein resources helped to complete material tasks related to degree acquisition, the code “persistence” was used when resources helped participants mentally to continue toward degree acquisition. This was often in the form of the resource motivating or encouraging students; for example, two students wrote similar sentiments about their advisors: “Keeps me going,” “Keeps me on track.” Family members, too, often provided essential motivation for participants, “My family consistently encourages me to keep going even when times get tough.” Social resources, like “advisor,” “significant other,” “research collaborators,” and “family members” were all frequently coded with “persistence”, and of these, “advisor” was coded most often (Figure 4).
The code “support” was the second most prevalent code in the data set and used 459 times; this code was used whenever participants described that a resource was important to them because it provided them with support, guidance, or mentorship. This included providing feedback, general guidance, or space for participants to express their feelings. For example, in describing the importance of their advisor, a student wrote: “My advisor helps me with everything and has given me incredibly useful guidance throughout my graduate degree.” Support often came from nonacademic resources as well, like therapists or other mental health counseling, which often supported students by providing a place to vent and a listening ear: “The rollercoasters of grad school, although felt by most, can be immensely heavy at times. My therapist helps me productively work through these feelings and thoughts.” Social resources, like advisor, significant other, labmates, and other graduate students were all frequently coded with support, and of these, advisor was coded most often (Figure 4).
The code “well-being” was used whenever participants described that a resource was important to them because it helped with their mental well-being by reducing stress or improving mental health and was coded 267 times. Resources that helped with participants’ well-being often did so by making participants feel loved, providing emotional or social outlets, or a sense of community. For example, a participant described why friends were one of their most important resources, “Seeing friends allows me to blow of(f) steam, escape from the stress of grad school for hours at a time, try new things in my city, etc.” In this example, friends were an important resource because they allowed the participant to destress and maintain a healthy work–life balance. Similarly, significant others were often mentioned as important resources for maintaining students’ well-being: “Grad school is inherently stressful- having support at home that isn’t academic really helps destress.” “Significant others” and “friends” were most often coded as fulfilling participants’ “well-being” needs (Figure 4).
Code Prevalence Across Demographic Characteristics.
Table 4 shows how codes in participants’ responses for the top three most frequently chosen resources varied across demographic characteristics. Percentages in this table represent relative frequency of code prevalence. Regardless of participants’ demographic characteristics, we found no significant differences in code prevalence (i.e., how participants described a resources’ importance). For example, student responses about their advisor were coded using the “academic” code approximately a quarter of the time, regardless of student demographic characteristics (e.g., 25% code prevalence for first-generation students vs. 23% for continuing-generation students; and 24% of code prevalence for women compared with 24% for men). For a full comparison of how code prevalence differed across demographic characteristics for all resources, see Supplemental Table S3.
Code | First/Continuing generation | Nonwhite/White | Woman/Man | Years 1–2/Years 3+ |
---|---|---|---|---|
Advisor | ||||
Academic | 25.88/23.61 | 28.28/23.80 | 24.65/24.32 | 26.81/23.57 |
Availability | 3.53/3.43 | 3.45/3.40 | 3.36/2.70 | 2.98/3.80 |
Basic need | 0.39/0 | 0/0.28 | 0.28/0 | 0/0.38 |
Connection | 5.49/4.72 | 4.14/5.67 | 5.60/4.50 | 3.40/6.84 |
Support | 43.92/48.50 | 42.07/47.03 | 44.54/52.25 | 45.96/45.52 |
Essential | 0.78/0.86 | 0.69/0.85 | 0.56/0 | 0/1.52 |
Persistence | 8.24/7.30 | 8.97/7.08 | 8.68/4.50 | 7.23/7.98 |
Validity | 7.84/9.01 | 8.28/9.07 | 8.96/9.91 | 8.51/9.13 |
Well-being | 3.92/2.58 | 4.14/2.83 | 3.36/1.8 | 5.11/1.52 |
Stipend | ||||
Academic | 20.25/16.67 | 13.51/20.26 | 15.86/27.87 | 15.83/20.96 |
Availability | 0 | 0 | 0 | 0 |
Basic need | 60.74/63.04 | 71.62/58.62 | 63/59.02 | 62.59/61.08 |
Connection | 0.61/0 | 0/0.43 | 0.44/0 | 0.72 /0 |
Support | 0 | 0 | 0 | 0 |
Essential | 12.27/17.39 | 13.51/15.09 | 15.86/11.48 | 15.83/13.77 |
Persistence | 0.61/0 | 0/0.43 | 0.44/0 | 0.72 /0 |
Validity | 0.61 /0 | 0/0.43 | 0.44/0 | 0.72/0 |
Well-being | 4.91/2.90 | 1.35/4.74 | 3.96/1.64 | 3.60/4.19 |
Significant other | ||||
Academic | 6.58/9.59 | 8.16/7.84 | *Women only 6.96 | 5.97/9.52 |
Availability | 1.32/0 | 2.04/0 | *Women only 0 | 0/ 1.19 |
Basic need | 0/4.11 | 0/2.94 | *Women only 2.61 | 1.49/2.38 |
Connection | 0 | 0 | *Women only 0 | 0 |
Support | 31.58/38.36 | 30.61/37.25 | *Women only 34.78 | 31.34/38.10 |
Essential | 0 | 0 | *Women only 0 | 0 |
Persistence | 9.21/6.85 | 10.2/6.86 | *Women only 8.70 | 10.45/5.95 |
Validity | 0 | 0 | *Women only 0 | 0 |
Well-being | 51.32/41.10 | 48.98/45.10 | *Women only 46.96 | 50.75/42.86 |
Online academic journals | ||||
Academic | *Men only 72.73 | |||
Availability | *Men only 0 | |||
Basic need | *Men only 0 | |||
Connection | *Men only 18.18 | |||
Support | *Men only 4.55 | |||
Essential | *Men only 4.55 | |||
Persistence | *Men only 0 | |||
Validity | *Men only 0 | |||
Well-being | *Men only 0 |
DISCUSSION
This study addressed two research questions: 1) What resources do LS graduate students consider to be the most important? 2) What drives LS graduate student perception of resource value? Regardless of their demographic characteristics, a majority of our respondents considered two resources as the most important – advisor and academic stipend. However, many other resources were selected as important by participants, albeit at a much lower frequency than advisor and academic stipend, suggesting that considerable diversity exists within student perceptions of resource importance. When asked why their chosen resources were important to them, participants’ free-response answers supported all four components of value outlined by EVTHS, though notably participants often described their expectations of the resource as well. Explanations of resource value did not differ significantly across demographic characteristics for the top three resources in the data set. We were surprised by the preponderance of responses focused on the type of help provided by the resource; this may indicate that some components of value (i.e., the type of help provided by resources) are more salient than others in students’ resource choice decisions. Finally, student responses revealed that the same resource can be perceived as having multiple values, suggesting a diversity of ways that individuals may perceive, and accordingly choose resources. Below, we discuss the implications of these results for policy, practice, and research in higher education, including how an adaptation of Maslow’s theory of human motivation (1943) could be used to visually conceptualize the unique needs of graduate students.
Expectations and Values: Theoretically Confounding Variables
While our study set out to investigate one component of EVTHS, resource value, we found that graduate student responses also aligned with aspects of EVTHS’ concept of “expectations.” For example, “availability” is a component of expectations as outlined by EVTHS. These results indicate that our study likely did not exclusively capture students’ perceptions of resource value, but instead captured both expectations and values. These results could indicate that expectations and values are strongly linked, as supported by current literature (e.g., Wigfield and Eccles, 2020). While previous work has found that expectations and values may be multiplicatively linked (e.g., Trautwein et al., 2012), our study was not able to investigate this type of interaction, and this may have further contributed to the conceptual overlap present in students’ responses (see Limitations). It may also be that expectations and values were linked for resources that are used most frequently by graduate students because the concepts of immediate use (i.e., value) and prospective use (i.e., expectations) are also confounded; an advisor is seen as useful in the future because they have already demonstrated utility in the present, for example. Future research should investigate whether expectations and values can be disentangled and, if so, develop methods to evaluate each component separately. While expectations and values were confounded in our results, many of our codes closely aligned with the four components of value hypothesized by our theoretical framework. For example, our codes “type of help provided” and “validity” are analogous to theoretical components of value “expected type of help” and “quality of help,” respectively. These results indicate support for the use of EVTHS in LS graduate student populations, though additional theoretical clarity is needed.
Similarity in Resource Perception Across Demographic Groups
Based on our previous work, which found significant differences in how graduate students perceived resources across certain demographic characteristics (Weatherton and Schussler, 2022), we disambiguated the data by demographic characteristics to examine whether demographic factors were related to which resources participants felt were most important, or how participants described resource importance. In regard to our first research question, we qualitatively examined differences in which resources students chose as most important and found no differences we felt rose to the level of further examination. While there were some resources chosen by certain demographic groups of participants that were never chosen by others (e.g., “university career center” was chosen as important by one continuing-generation student, but never by first-generation students), the number of students choosing these resources was too small to draw conclusions about the relationship between resource perception and student demographics broadly. Similarly, in terms of our second research question, we found that student perception of resource value (i.e., prevalence of response codes across student responses) did not differ significantly across demographic characteristics (Supplemental Table S3).
As opposed to existing literature on student perception of value (e.g., Eccles et al., 1993; Ruble et al., 2006; Wigfield et al., 2015), our results did not find a significant relationship between resource perception and demographics. We believe the most likely explanation for our findings is that this study examined students’ most important resources instead of student perception of all resources. These top resources may be so generically useful to graduate school that students value them in similar ways, versus how less important resources may be valued. This hypothesis is supported by our previous work (Weatherton and Schussler, 2022), which used data from the same participants and found significant differences in participants’ frequency of resource use and perception of resource usefulness across demographic characteristics for certain resources; importantly, the resources which showed significant differences were not those which were frequently chosen as “most important” in this study. Thus, it may be that student perceptions of resource value do relate to demographic characteristics, but only for resources that are not as critically valuable as those top “universally important” resources (see Limitations).
Another potential explanation for this null effect is a filtering effect of higher education; most studies on resource perception are conducted at the K–16 level (e.g., Eccles et al., 1993; Guthrie et al., 2007; Chiang et al., 2011). If the use and perception of resources are linked to academic success, students at the graduate level may represent the outcome of a filtering process. Thus, students at the graduate level would have similar perceptions of resources, regardless of demographic characteristics. Future work should continue to examine differences in resource perception across demographic groups, potentially using qualitative methods to dive deeper into perceptions that may impact students’ resource use.
The Majority of Participants Considered Advisor, Academic Stipends to be Important, Regardless of Demographic Characteristics
The finding that advisor and academic stipend were chosen most frequently as an important resource is consistent with previous work showing the overwhelming importance of social and financial resources for graduate students’ well-being and academic success (Tinto, 1993; Lovitts, 2002; Sverdlik et al., 2018; Blanchard and Haccoun, 2020). Our results indicate that this seems to be generally true regardless of the demographic background of the participant. Although advisor and academic stipend were chosen frequently by participants in our study, there was an astonishing diversity of other resource choices among the participants. Indeed, 23 of the 31 resources that were selected as important were selected by fewer than 50 participants, or 10% of our sample. These findings validate the notion that university leaders and mentors cannot make assumptions about what resources are important to students. Resources that were chosen less frequently, like university transit system, departmental seminars, or university-provided research facilities were clearly important to some students, however, relatively little research has been done to explore these resources and their impacts on student outcomes (as opposed to resources like advisor or labmates). Thus, our results provide multiple avenues for future research into the resources that LS graduate students feel are important, and which may be integral to improving student outcomes. Overall, our results indicate that advisors, mentors, and institutions should continue to foster supportive social relationships and facilitate opportunities for graduate students to secure funding. Mentors and department leaders should also be encouraged to engage in conversations with students about their resource needs and perceptions of value, to collect individual-level data upon which to make mentoring and policy decisions.
Graduate Students’ Perceptions of Importance are Driven by What Kind of Help the Resource Provides
While participants mentioned both expectations and values, a majority of responses centered around one component of value: “expected type of help” (i.e., codes in our help provided theme). Student perceptions of this aspect of value are not often evaluated and many expectancy-value scales lack items pertaining to this component (Wigfield and Eccles, 2000; Flake et al., 2015). Thus, our results indicate that student expectations of the type of help a resource will provide may be an integral but understudied aspect of student resource choice.
Within the theme of “help provided,” “academic” and “support codes” were most prevalent, indicating a priority among this population for resources that provided academic help or supported them in some way. This finding aligns with previous work that indicates academic and mentoring supports are integral to the success and well-being of graduate students (Sverdlik et al., 2018; Tullis and Kowalske, 2021). However, much of the extant literature related to graduate student academic support focuses on single resources, such as advisors, while our participants described using a myriad of resources to receive academic help. Indeed, participants described that they received academic help from 22 of the 31 resources surveyed (Figure 4). The variety of sources from which our participants sought academic help further validates the notion that the field of graduate education has not fully explored graduate-student resource choice and more empirical data are needed to understand graduate students’ complex behaviors and perceptions as they relate to resource choice.
Beyond “academic help” and “support”, our results highlighted the importance of resources that fulfilled students’ basic needs, which are often overlooked by graduate programs and institutional policy (Zahneis and June, 2020; Oh et al., 2022). Participants in our study overwhelmingly described the importance of their academic stipend, with 57% of participants choosing it as one of their top three most important resources, and of these participants, 95% mentioning that academic stipend was important to them, in part, because it fulfilled their basic needs. Academic stipend conferred participants the ability to pay their bills, afford housing, and generally worry less about struggling financially. Although the current extent of food and housing insecurity in graduate student populations is unclear (Nazmi et al., 2019), our results suggest that a large proportion of graduate students are concerned about being able to meet their basic needs. Maslow’s theory of human motivation (1943) suggests that an inability to fulfill basic needs impedes individuals’ ability to fulfill other needs, like safety, belonging, or self-esteem. In the context of graduate education, this may suggest that students unable to fulfill their basic needs may be less able to achieve academic success or maintain their mental health. Indeed, this notion is supported by previous work in undergraduate students, which has revealed that that food and housing insecurity is related to poor mental health and lower academic persistence (Phillips et al., 2018; Oh et al., 2022). Future research should investigate the extent to which resources offered by graduate programs, and specifically academic stipends, are able to fulfill students’ basic needs. Furthermore, future work should explore the effects of unmet needs on student outcomes within graduate student populations.
Components of Resource Importance Differ Across Resources
Our results revealed substantial variation in the codes that made up participants’ perceptions of resource importance across resources in our study, with some resources having multiple codes associated with them, while others were only seen as important due to the help they provided in one area (i.e., were only described using one “help provided” code). For example, labmates and friends both provided five different types of help – 1) academic, 2) connection, 3) support, 4) persistence, and 5) well-being (Figure 4). On the other hand, resources like university gym and departmental administrators only provided one type of help, well-being, or support, respectively (Figure 4). We found no identifiable pattern across resources in terms of the diversity of types of help they provided (Supplemental Table S3). For example, social resources (e.g., advisor, significant other) generally were perceived to provide many different types of help, but other social resources like alumni network did not show the same trend. Thus, it’s unclear what aspects or characteristics of a resource may lead to multiple perceptions of types of help, or more broadly, what aspects of a resource inform student perceptions of importance and value. However, the demonstrated variation among resources and the components of importance described by participants reveals interesting avenues for future research, and this variation should be further investigated in LS graduate students and in other populations (e.g., undergraduate students, graduate students within other domains). Future work could investigate whether perceptions of resource importance are stable across time and if so, how components of importance align with certain resources (e.g., examine whether certain resources are preferentially valued for their validity, while others are valued for their availability) and how that informs overall resource choice. A more nuanced understanding of how perceptions of importance differ across resources could inform institutions and mentors about highly impactful resources or which resources to recommend to students based on the type of help students are seeking.
An Academic Hierarchy of Needs
Our results indicated that LS graduate students’ perceptions of their most important resources are driven, in part, by their attempts to fulfill needs. Furthermore, our results show that while graduate students are likely to have similar needs, each student will have unique perceptions of these needs and unique ways of fulfilling them. Therefore, an important takeaway of these results is that institutions, departments, and mentors must gather similar qualitative data about their own students to understand how to best support them. Below, we use Maslow’s theory of human motivation (1943) to further understand these results and how to apply them to current thinking and practice within higher education.
The theory of human motivation states that individuals have an increasingly complex set of needs that they strive to meet, including physiological and psychosocial needs, and that the goal of fulfilling these needs motivates individual behavioral choices. This theory is often visualized by a pyramid of needs, with more basal needs at the bottom (e.g., physiological and safety needs) moving towards more complex needs (e.g., self-esteem and self-actualization needs) at the top (De Bruyckere et al., 2015; Bridgman et al., 2019). However, this visualization, and the coinciding idea that needs must be met in a hierarchical, stepwise order was not proposed by Maslow; instead, the theory indicated that while needs move from basal to complex, individuals may aim to meet multiple needs at once (Maslow, 1943). We see similar trends in our results, where participants identified that some resources, like their academic stipend, help them to fulfill both basic and academic needs (e.g., “I would not be able to focus on my graduate education, or have access to education without this stipend to pay rent and buy food.”). Participants also discussed resources that helped them to fulfill more complex needs, like self-esteem and self-actualization. For example, realizing one’s talent and potential are key aspects of self-actualization, and participants often cited their advisor as helping them to realize their scientific potential, as these three participants said similarly: “(my advisor is) central to developing as scientist,” “propels me in my academic development,” and “helps me development as a scientist.” Maslow’s theory provides a useful framework to identify the holistic needs of graduate students; combining this framework with the results of this study, we propose an adapted model of academic needs that may be used in future work to explain the needs and resource choices of graduate students. This model, like the original theory proposed by Maslow, is organized from basal to more complex needs and includes physiological, academic, social, esteem, and self-actualization needs (Figure 5). Also included in the model are examples of resources that may help students to achieve those needs, though as previously mentioned, resources may fill multiple needs, needs may be fulfilled by multiple resources, and different students are likely to use different sets of resources to fulfill the same needs. This model recognizes that needs overlap considerably and that the process of fulfilling these needs does not happen linearly, thus the reciprocal arrows between needs.
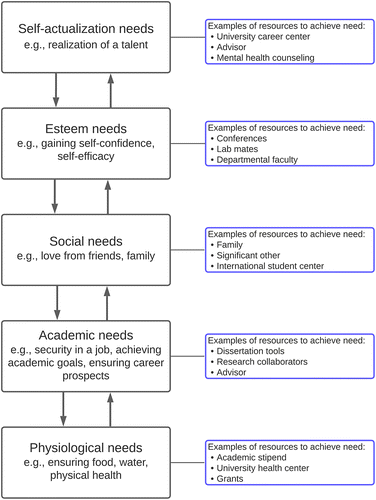
FIGURE 5. Our proposed academic hierarchy of needs. Black boxes indicate needs, arrows indicate the possibility of fulfilling multiple needs at once, and blue boxes represent example resources that students may use to fulfill need.
This model adds to the literature by providing nuance that is specific to higher education settings in the form of academic needs and mention of resources specific to graduate students, and thus is a useful adaptation for future studies of student needs, value perceptions, or resource choice. Utilization of this model will allow researchers to consider graduate students and their needs more holistically, which in turn may allow for the advancement of policy which considers all aspects of graduate students’ needs. This model may also be used as a tool for those in higher education. For example, institutions may use it to evaluate the extent to which graduate students’ needs are being fulfilled by certain policies, or mentors and graduate students may use it to identify current and future needs and how to fulfill them. For example, a mentor and graduate student could talk about the students’ unique academic needs and what resources they might draw on to fulfill them, identifying gaps and potential professional development opportunities. We believe that utilizing this model to facilitate these discussions represents important mentoring opportunity, as identifying student needs and resources to fulfill them may be, for some students, part of the academic “hidden curriculum” of aspects of graduate school that are not explicitly discussed. As previous literature has shown, students struggle to achieve success when components of their needs are not met (Phillips et al., 2018; González-Betancor and Dorta-González, 2020; Oh et al., 2022); thus, we believe that a greater focus on striving to fulfill all of students’ needs will lead to an increase in students’ well-being and academic success.
Limitations
We are mindful of several limitations that limit the scope and generalizability of our work. Specifically, we recognize that our participant sample is not fully representative of LS graduate students broadly, and thus conclusions drawn from our work may be missing important perspectives. As with our previous work drawn from the same survey, our participant pool was overrepresentative in terms of white students (71% of our participants, compared with 47% of LS graduate students broadly; National Science Board 2020) and female students (70.8% in our sample compared with 55.8% in LS graduate programs broadly; National Center for Science and Engineering Statistics, 2021). These participant-level limitations limit the generalizability of our findings beyond our sample. Future research should work to validate the patterns found in our results in more diverse population pools (in terms of racial and gender diversity, as well as in terms of study domains) to further explore the nuance within student perceptions of value.
Furthermore, survey-level limitations included resources missing from the survey which students may have found important and issues with overlap between the concepts of expectations and values. As with our previous work drawn from the same survey, participants wrote in 20 unique resources that were missed during survey development and were not included in our list. These “missing” resources may have been highly important to students, though because they were not included in our survey list, we were unable to collect data related to them. Thus, our results do not represent a fully comprehensive examination of the resources that are important to students. Future work may circumvent this limitation by allowing students to write in resources that are not included in the survey.
As previously mentioned, although this study set out to solely examine students’ perception of resource value, our results included aspects of expectations (as outlined by EVTHS). This may indicate that our survey question was not clear enough to exclusively collect students’ perceptions of value, or because surveys about resource choice are an inherently retrospective endeavor (i.e., we are asking about choices that have already happened), values and expectations are inextricably linked concepts. Future research should work to more fully understand students’ definitions of resource value and resource expectation so that more refined survey questions can be created to separate the two concepts.
Finally, we recognize that qualitative work is inherently limited by our own experiences and biases. We took many steps to address the validity and trustworthiness of our methods (see Validity). While we have done our due diligence to explore, understand, and bracket our internal biases as they relate to interpreting data, there will always be limitations inherent to a qualitative study that stem from who is interpreting the data, and thus our results and conclusions should be interpreted in light of this fact.
CONCLUSION
This study asked questions about LS graduate students’ resource choices and the values associated with those choices. Using EVTHS and qualitative methods we found that LS graduate students perceive a variety of resources to be important to them, and that these choices were driven largely by the type of help participants felt that the resources provided. Although we asked specifically about value, participants often discussed their expectations for a resource, as well as their values, indicating that expectations and values may be fundamentally linked. Based on our results, we have proposed a novel way to understand graduate-student resource choice: an academic hierarchy of needs. While this study provides important insights about why graduate students use certain resources, future work is needed to understand how resource choice is related to student outcomes (e.g., academic success, well-being, persistence) and how student perception of resources may change over time. Resource utilization is a ubiquitous and essential aspect of the graduate student experience, though much diversity exists within this process. Further research examining the complexity of this process will allow mentors, advisors, and leaders of institutional change to tailor resources for graduate students and ultimately help improve their experience as graduate students.
ACKNOWLEDGMENTS
The authors would like to thank Dr. Caroline Wienhold and Hope Ferguson for their thoughtful comments and feedback on versions of this paper. We would like to thank the editor and reviewers for their useful feedback, which has helped strengthen and clarify our work.