Variations in Student Approaches to Problem Solving in Undergraduate Biology Education
Abstract
Existing research has investigated student problem-solving strategies across science, technology, engineering, and mathematics; however, there is limited work in undergraduate biology education on how various aspects that influence learning combine to generate holistic approaches to problem solving. Through the lens of situated cognition, we consider problem solving as a learning phenomenon that involves the interactions between internal cognition of the learner and the external learning environment. Using phenomenography as a methodology, we investigated undergraduate student approaches to problem solving in biology through interviews. We identified five aspects of problem solving (including knowledge, strategy, intention, metacognition, and mindset) that define three qualitatively different approaches to problem solving; each approach is distinguishable by variations across the aspects. Variations in the knowledge and strategy aspects largely aligned with previous work on how the use or avoidance of biological knowledge informed both concept-based and nonconcept-based strategies. Variations in the other aspects revealed intentions spanning complete disengagement to deep interest with the course material, different degrees of metacognitive reflections, and a continuum of fixed to growth mindsets. We discuss implications for how these characterizations can improve instruction and efforts to support development of problem-solving skills.
INTRODUCTION
Creating learning opportunities for students to engage with complex, real-world problems is a major goal for undergraduate science, technology, engineering, and math (STEM) education (Harper, 2006; Klegeris and Hurren, 2011; Hoskinson et al., 2013; Conana et al., 2020; Avena et al., 2021; Frey et al., 2022). There have been multiple calls to align learning and teaching with real-world problems (Jacob, 2004; American Association for the Advancement of Science, 2011). One important component is problem solving, where students apply concepts and think through a series of decisions that allow them to define, interpret, and solve a problem (Martinez, 1998; Carlson and Bloom, 2005; Price et al., 2021).
Multiple studies have characterized the knowledge and strategies that students use when solving problems in STEM (Carlson and Bloom, 2005; Jones, 2009; Fredlund et al., 2015; Price et al., 2021; Frey et al., 2022). Both domain-general knowledge (which can be applied across any discipline) and domain-specific knowledge (which are skills unique to a given discipline) can influence problem solving, suggesting the existence of discipline-related variations (Alexander et al., 1989; Jones, 2009; Fredlund et al., 2015; Prevost and Lemons, 2016). In the context of undergraduate biology education, students utilize a variety of conceptual strategies (that rely on biological reasoning) and nonconceptual strategies (that are algorithmic or heuristic in nature but without connecting to biological principles) to solve problems (Brumby, 1982; Hoskinson et al., 2013; Avena and Knight, 2019; Avena et al., 2021; Sung et al., 2022). Similarly, a previous case study on problem solving identified one conceptual strategy grounded in biological understanding and two nonconceptual strategies based on algorithms or patterns (Sung et al., 2022). While our previous work aligns well with other existing literature, its focus on knowledge and strategies alone limits our understanding of how other aspects of student learning can impact problem solving.
A number of aspects that influence learning are correlated to how students solve problems in STEM. For example, students’ intentions for choosing particular problem-solving strategies inform the specific actions that they perform in engineering (Case and Marshall, 2004). Similarly, the level of metacognitive reflection has been found to correlate with problem-solving outcomes in mathematics and medical sciences (Safari and Meskini, 2016; Izzati and Mahmudi, 2018). Students’ mindsets, or their beliefs about their ability to improve, may also influence their problem-solving potential in mathematics (Callejo and Vila, 2009). However, little work has been done to investigate how these aspects of intention, metacognition, and mindset interact with strategies to inform students’ problem-solving approaches, particularly in undergraduate biology education.
To complement existing work in the literature, we identify and characterize student approaches to problem solving in undergraduate biology education, while accounting for multiple aspects that influence learning. This current study significantly extends previous work (Sung et al., 2022) beyond considering only knowledge and strategies in problem solving, providing an opportunity to develop a more comprehensive model of how multiple aspects of problem solving are integrated in a particular approach. Specifically, our research question is: What are the qualitatively different approaches that undergraduate students use to solve problems in biology?
THEORETICAL FRAMEWORKS
In discipline-based education research across STEM, the term framework or theoretical framework is used inconsistently to convey a combination of conceptual perspectives that inform the overall interpretation of the results, methodological rationale that guides the research process from data collection to analysis, and existing literature on the research topic that situates the novel contributions of the conclusions (Bussey et al., 2020; Luft et al., 2022). To distinguish these overlapping elements for our current study, we articulate situated cognition as a conceptual framework for understanding problem solving as a learning phenomenon, explain the utility of phenomenography as the methodology, and describe relevant literature on problem solving in STEM.
Situated cognition
Research on problem solving in STEM included cognitive, metacognitive, affective, and contextual dimensions (Lee et al., 1996; Taconis et al., 2001; Shin et al., 2003; Reigosa and Jiménez‐Aleixandre, 2007; Taasoobshirazi and Glynn, 2009; Jonassen, 2010; Löffler et al., 2018; Akben, 2020). To account for these intersecting dimensions, we use situated cognition as the conceptual framework for this study (Table 1). Situated cognition is a sociocultural learning theory positing that knowledge is not simply a product of cognition but is further situated in the activities, contexts, and cultures in which the knowledge is produced and used (Brown et al., 1989; Cakmakci et al., 2020). Correspondingly, learning is viewed not just as constructing an understanding of disciplinary knowledge but rather more expansively as the interactions between the learner and the environment as learning occurs (Lave and Wenger, 1991; Cakmakci et al., 2020). Past work examining problem solving through the lens of situated cognition has identified that contextual and situational factors can impact students’ problem-solving approaches (Kirsh, 2008; Roth and Jornet, 2013). By considering problem solving as a learning phenomenon from the perspective of situated cognition, we expect to identify a coherent way to understand student approaches to problem solving that can likely accommodate the different aspects in the existing literature, as well as additional dimensions related to course and disciplinary contexts that may emerge from the data as we observe students interacting with the problem-solving process.
Term | Definition | Study context |
---|---|---|
Situated cognition | Posits that knowledge is situated in the activities, contexts, and cultures in which it is produced and used. | We used situated cognition as the conceptual framework by considering problem solving as a learning phenomenon. |
Cognitive apprenticeship | Articulates six processes for instructors and learners that can facilitate learning based on situated cognition. | We used cognitive apprenticeship in relation to our results to inform implications for teaching. |
Phenomenography | Aims to identify and characterize the qualitatively different ways that individuals experience a phenomenon. | We used phenomenography as methodology to identify the different student approaches to problem solving. |
Aspect | Characterizes specific features of a phenomenon that are experienced or noticed by individuals. | We identified aspects or specific features of problem solving that study participants attend to in the interviews. |
Variation | Describes differences in a given aspect and thus the phenomenon that are experienced by individuals. | We identified variations or differences in each aspect of problem solving that study participants describe. |
Outcome space | Represents a set of logically related descriptions that define the qualitatively different experiences. | We used the outcome space to describe variations within each aspect of approaches to problem solving. |
Variation theory | Posits that variations in how aspects are experienced by individuals can lead to different understanding. | We used variation theory as part of the methodology to construct an outcome space of different approaches. |
Approach | Is defined classically to include strategy (plans for doing something) and intention (motivation to do it) | We identified approaches to problem solving based on variations observed in different aspects. |
Intention | Encompasses a combination of motivation and interest, to be consistent with literature in phenomenography. | We identified intention as one of the aspects from the data and characterized its variations. |
Metacognition | Describes one’s awareness of their own learning and utilization of specific reflective skills to support learning. | We identified metacognition as one of the aspects from the data and characterized its variations. |
Mindset | Describes one’s beliefs about their ability to improve on a continuum between fixed and growth mindsets. | We identified mindset as one of the aspects from the data and characterized its variations. |
Developed from situated cognition as a learning theory, cognitive apprenticeship describes six processes that can facilitate learning: modeling, coaching, scaffolding, articulation, reflection, and exploration (Table 1; Hennessy, 1993). Modeling, coaching, and scaffolding are processes that involve an expert making explicit their tacit knowledge and thought processes, providing feedback as learners engage in a task, and designing increasingly complex tasks that successively expands on the understanding of a concept or development of a skill, respectively (Hennessy, 1993; Cakmakci et al., 2020). Articulation, reflection, and exploration are processes that involve learners describing their knowledge and reasoning, comparing their own thought processes with those of other people or a model, and entering a mode of learning on their own, respectively (Hennessy, 1993; Cakmakci et al., 2020), all of which relate to metacognition or a student’s ability to reflect on their own thinking (Tanner, 2012). Because cognitive apprenticeship is a pedagogical articulation of situated cognition, fundamental insights about student approaches to problem solving emerging from this study can likely be translated into tangible teaching implications.
Phenomenography
We use phenomenography as the methodological framework to guide our research process (Table 1). Phenomenography is the empirical examination of the variations in how people think about the world, with the goal of identifying and characterizing the qualitatively different ways of experiencing a specific phenomenon (Figure 1; Marton, 1981, 1986; Hajar, 2021). Variation theory in phenomenography further organizes the results of a research study into a two-dimensional outcome space, which articulates the aspects or specific features of a phenomenon that individuals are aware of and pay attention to, as well as the variations or distinctions in how each of these aspects are experienced by different individuals (Table 1; Marton and Tsui, 2004; Åkerlind, 2018). Together across multiple aspects, the outcome space represents a hypothesis based on a set of logically related descriptions that define the qualitatively different ways of experiencing the phenomenon by different individuals (Marton and Booth, 1997), that is, distinct approaches to problem solving.

FIGURE 1. Phenomenography as methodology. We use phenomenography to investigate the qualitatively different ways that undergraduate students approach problem solving in biology education. Instead of directly studying problem solving as a phenomenon, we are examining how different study participants experience and understand problem solving. The research team interacts empirically with study participants in terms of data collection and conceptually with the phenomenon of problem solving situated as positionality.
Variation theory can be further applied to explain how instructors and students may experience the same learning phenomenon differently (Bussey et al., 2013). Previously, this application of variation theory was used to articulate why it is important to examine instructors’ definition of understanding in biology education (Hsu et al., 2021) and students’ strategies in solving biology problems (Sung et al., 2022). Furthermore, variation theory can be used as a framework to examine how individual students may experience the same learning phenomenon differently. For example, when presented with an exam problem, students could attend to the features of the problem itself, patterns of the problem that are similar to other practice problems, or biological concepts embedded within the problem, thus resulting in a variety of conceptual and nonconceptual problem-solving strategies (Sung et al., 2022).
Phenomenography is a research methodology developed within higher education research by higher education researchers (Tight, 2016) and has been used extensively to study learning and teaching across disciplines (Akerlind, 2005; Booth, 1997; Entwistle, 1997), including STEM as well as medical and nursing education (Swarat et al., 2011; Stenfors-Hayes et al., 2013; Barry et al., 2017; Han and Ellis, 2019). Within this literature, an approach to learning was initially defined as having two aspects, including the strategy (plans for doing something) and the intention (motivation for doing it) (Table 1; Marton, 1988; Case and Marshall, 2004). Three general student approaches to learning have been identified: (1) a surface approach that utilizes rote memorization to reproduce details of the course, (2) a procedural approach that relies on study strategies to achieve course outcomes, and (3) a deep approach that internalizes and assimilates meaning to understand disciplinary content (Biggs, 1979; Entwistle et al., 1979; Case and Marshall, 2009). Subsequent research highlighted the importance of affect and metacognition in student approaches to learning (Case and Gunstone, 2002; Pintrich, 2004; Case, 2008). More recently, a few studies have specifically examined approaches to problem solving in biology, engineering, physics, and physiotherapy using phenomenography, further highlighting the potential connections between different aspects in problem solving across STEM (Walsh et al., 2007; Lönngren et al., 2017; Dringenberg and Purzer, 2018; Dahlgren et al., 2021; Sung et al., 2022).
Problem solving in STEM
Existing literature on problem solving in undergraduate biology education primarily focus on knowledge and strategies. In addition to domain-general knowledge that can be applied across disciplines, students need to utilize declarative knowledge such as disciplinary concepts, procedural knowledge such as problem-solving strategies specific to the discipline, and conditional knowledge such as metacognitive awareness on when to use certain strategies (Prevost and Lemons, 2016). Problem-solving strategies can be algorithmic, when students memorize a pattern or formula and utilize it without recognizing the underlying biological concepts (Avena et al., 2021; Sung et al., 2022), or can include more complex sets of actions, where students organize biological concepts into a mental framework to solve the problem (Nehm, 2010; Prevost and Lemons, 2016). Furthermore, students often rely on domain-general knowledge and nonbiological reasoning, particularly when approaching problems with higher-order cognitive demands in biology (Prevost and Lemons, 2016; Sung et al., 2022).
Beyond knowledge and strategies, approaches to learning and problem solving include intention, or motivation to use a certain strategy (Table 1; Marton, 1988; Case and Marshall, 2004). Motivation is a complex term encompassing distinct and related constructs from multiple theoretical traditions (Conradi et al., 2014; Murphy et al., 2019; Richardson et al., 2020). One motivational factor that can influence student learning and persistence across STEM is interest (Schiefele, 1991; Wang, 2013), a term that has been applied in many contexts in biology education research (Rowland et al., 2019). Development of interest as motivation for learning can be serendipitous (e.g., trigged by an unplanned event), promoted by other people (e.g., in response to external demands as imposed by course structure), or self-generated by students (e.g., internally recognizing connections among different concepts; Renninger and Hidi, 2022). Interest can drive student learning, leading to more efforts and reflections (Zimmerman, 2002). While we acknowledge that there are entire bodies of research focused on motivation and interest, we use the term intention in this paper, operationalized as a combination of motivation and interest, to be consistent with prior literature in phenomenography that has studied student approaches to learning and problem solving.
Metacognition is another aspect that can influence problem solving and learning in undergraduate biology education (Table 1; Tanner, 2012). There are multiple definitions of metacognition (Schraw and Moshman, 1995; Pintrich, 2004; Veenman et al., 2006; Dinsmore et al., 2008). Here, we refer to metacognition based on self-regulated learning (SRL), where metacognition in conjunction with motivational and behavioral processes can influence the ability of learners to regulate their own learning (Zimmerman, 2002; Sebasta and Bray Speth, 2017). In this definition, the term metacognition encompasses metacognitive knowledge (understanding and awareness of one’s own thinking and learning) and metacognitive processes or regulation (activation and utilization of specific reflective skills such as planning, monitoring, and evaluating to support learning; Pintrich, 2004; Dinsmore et al., 2008; Stanton et al., 2021). Past work has identified that increased metacognitive knowledge and processes can drive regulation of learning and improve problem solving (Swanson, 1990; Antonietti et al., 2000; Aşık and Erktin, 2019); thus, we hypothesize that different problem-solving approaches may be associated with specific forms of metacognition.
Mindset can refer to several distinct and related constructs that shape student beliefs about the nature of their abilities, which can impact learning; most work in this area has focused specifically on student beliefs about their ability to improve, which is the definition that we use in this study (Table 1; Dweck and Yeager, 2019). Students who possess a growth mindset and believe that they are able to improve their skills tend to have more success at developing those skills, compared with students who possess a fixed mindset and believe that abilities are static and unmalleable (Limeri et al., 2020; Miller and Srougi, 2021). The impact of such mindsets on student learning is further influenced the instructor’s mindset as well as the sociocultural and institutional contexts of the learning environment (Muenks et al., 2020; Muenks et al., 2021; Canning and Limeri, 2023). Because of these complex interactions among mindset, student learning, and the learning environment, we believe that this study with situated cognition as the conceptual framework can provide insights into how mindsets may impact student approaches to problem solving.
Taken together, the existing literature suggests that multiple aspects can influence student learning and problem solving. In a previous study, case studies were developed to describe the knowledge and strategies used by three participants in solving problems, with a focus on implications for assessments, and the results were largely aligned with those in the existing literature (Sung et al., 2022). However, there was additional richness to these participants’ experiences in other aspects such as intention, metacognition, and mindset that were left unexplored. More broadly, there is a need in the literature for studies that examine how these various aspects integrate together to form different approaches to problem solving, specifically in biology. Consequently, the goal of this study is to develop a comprehensive model for how students approach problem solving in undergraduate biology education.
METHODS
Context and participants
This study took place at a 4-y, private not-for-profit, doctoral university with very high research activity in the United States, with an undergraduate profile described as 4-y, full-time, more selective, lower transfer-in, large, and primarily residential, according to the Carnegie Classification of Institutions of Higher Education (McCormick and Zhao, 2005). We recruited 22 study participants over three academic years from an introductory course on genetics and molecular biology using a purposeful stratified sampling plan that included exam performance and gender (Sung et al., 2022). The sampling variables were included to maximize the potential number of aspects and variations in the outcome space for student approaches to problem solving (Han and Ellis, 2019) but not to determine whether these variables correlated with the different approaches identified in the study. Additional descriptions on the study context and participants were reported previously (Sung et al., 2022).
We chose genetics as the disciplinary context because genetics has been identified as a critical component of undergraduate biology education (Smith and Wood, 2016). Furthermore, there has been a long history of other work examining a variety of issues related to problem solving in genetics (Tolman, 1982; Smith and Good, 1984; Stewart and Kirk, 1990; Cavallo, 1996; Avena et al., 2021), providing an existing literature to build upon. Finally, a variety of qualitative and quantitative problems are available in genetics coursework that can be used as interview tasks (Sung et al., 2022).
Data collection and analysis
We used clinical interview originating from Piagetian constructivist traditions as a method (Piaget, 2007) to explore students’ approaches to problem solving in biology. Additional descriptions on this method were reported previously (Sung et al., 2022). Here, we highlight that the interview protocol was semistructured, a common feature for phenomenography as a methodology (Han and Ellis, 2019). Participants were asked to explain, elaborate, or confirm their problem solving approaches by verbalizing their thought processes and drawing diagrams (diSessa, 2007) in interview tasks with problems that involved nondisjunction and genetic recombination, with possible follow-up prompts that further explore ideas brought up by participants (Supplemental Material). Participants were also asked if and how the course could be structured differently to support their learning, an interview question that was not analyzed in the previous study (Sung et al., 2022). The interviewers (S.L.S. and S.M.L.) had no prior contacts with the participants and were not involved with the courses that served as the recruitment site for this study. Interviews were audio-recorded and transcribed semiverbatim to remove verbal nods such as “um” and “ah” by a professional service, and Transcripts were spot-checked. Drawings also were collected as artifacts. Additional descriptions on the data collection process were reported previously (Sung et al., 2022).
Transcripts were analyzed using qualitative methodologies in three stages (Saldana, 2021) as described previously (Zuckerman and Lo, 2022). First, preliminary codes were developed through iterative close reading of the transcripts to provide a large corpus of thought processes emerging from the data (S.L.S., A.J.G., S.K., and S.M.L.). Second, codes were condensed to identify different aspects of approaches to problem solving, leading to the creation of a preliminary outcome space (J.L.H., R.J.S., and S.M.L.). Using constant comparative method (Glaser, 1965), excerpts that describe each aspect and variations across the different approaches were contrasted with previously analyzed transcripts, allowing for the confirmation or disconfirmation of working conjectures. Third, data for each intersection in the preliminary outcome space were revisited to guard against biases, maintain consistency, and further refine specific variations from one approach to the next within each aspect, resulting in the final outcome space (J.L.H., R.J.S., and S.M.L.). We note that as the outcome space is emergent from the data, the interview protocol was not constructed around the aspects identified through this analysis process, nor were the participants asked to discuss them in the follow-up prompts. Finally, results are reported as excerpts associated with pseudonyms for study participants.
The data analyses in this current study were expanded from the set of interviews conducted previously (Sung et al., 2022). Revisiting prior data in what is called secondary analysis has emerged as common practice in qualitative methodologies in the past two decades (Bishop and Kuula-Luumi, 2017). In general, data sources for such secondary analyses include formal sharing through public repositories, informal sharing among collaborators, and reuse of previously self-collected data to investigate additional research questions (Heaton, 2008). This study falls under the third category of reusing our own data that were collected previously. Furthermore, there are five purposes to secondary analyses of qualitative data; specifically, this study falls under the category of supra analysis, where the focus of the new study transcends that of the previous work to address novel research questions and to reach new theoretical, empirical, and/or methodological insights (Heaton, 2008).
Compared to the previous study (Sung et al., 2022), the results reported in this study represent a novel analyses of: (1) study participants who were not previously included, (2) additional data with previously unreported excerpts from the three study participants in the prior study, and (3) additional data from an interview question that had not been analyzed. We are also investigating a supra research question that asks how the previously identified strategies (Sung et al., 2022) are more comprehensively situated in an outcome space with other aspects of problem solving. Given that the goals of this study are to extend our previous work, we believe that it was of value to reexamine the previous interview data as well. Consequently, the excerpts reported in this study for the previous three case-study participants (Sung et al., 2022) represent an extension of existing work specific to the new research question outlined in this study.
Reliability, validity, and trustworthiness
In phenomenography, reliability focuses on dialogic agreement “through discussion and mutual critique of the data and of each researcher’s interpretive hypotheses” (Åkerlind, 2005). The research team met regularly to examine the preliminary codes, the emergent aspects and their variations, and iterations of the outcome space, providing checks against personal biases and supporting reliability throughout the process. All disagreements were resolved through dialogic discussions in the form of argumentation (Schoenfeld, 1992) to reach consensus on the structural relationships between the various aspects and approaches of the final outcome space.
Research in phenomenography is also expected to yield an outcome space considered to be appropriate and useful by relevant communities (Entwistle, 1997; Åkerlind, 2005; van Rossum and Hamer, 2010; Hajar, 2021). Throughout data analyses, our results were presented to various communities of biology education researchers and practitioners, including faculty, staff, postdoctoral scholars, and students, for feedback. Validity stems from these repeated cycles of critique and refinement as well as the perceived usefulness of the outcome space, resulting in potentially new understanding of student learning and educational interventions (Åkerlind, 2005).
Theoretical saturation was achieved by collecting and analyzing data through the constant comparative method over a sufficient length of time (Aldiabat and Le Navenec, 2018) as described previously (Sung et al., 2022). In short, preliminary descriptions were developed from data in the first year and were confirmed by data in the second year. While refinement of the outcome space was supported by data in the third year, the overall structure of the outcome space did not change. Furthermore, the number of interviews conducted (n = 22) are within the estimated range that would typically result in theoretical saturation (Hennink et al., 2017).
We recognize that our own experiences can influence the way we collected and analyzed the data, and we include a statement of positionality to situate our identities as researchers and educators in the context of this study to enhance the trustworthiness of our findings (Bourke, 2014). J.L.H. and R.J.S. are pretenure faculty in biology at a comprehensive university and a primarily undergraduate institution, respectively, and both have programs in biology education research. A.J.G. and S.K. were undergraduate students majoring in quantitative methods in social sciences and biological sciences, respectively, when they contributed to the data analyses. S.L.S. has an M.S. in evolutionary biology and Ph.D. in learning sciences and was a senior research associate focused on STEM education at a teaching and learning center at the time of the study. S.M.L. is tenured teaching faculty in biology who primarily works in discipline-based education research at a research-intensive university. Together, our collective professional experiences with complementary expertise and career stages provide enriched interpretations of the data and guard against potential biases.
RESULTS
We identified the following five aspects with variations that distinguish different approaches to problem solving: (1) Knowledge: What is the knowledge base that students are drawing from as they work on problems? (2) Strategies: How are students connecting and applying their knowledge to reach an answer to the problem? (3) Intention: Why are students engaging with problems from this course and/or the discipline? (4) Metacognition: How do students reflect on their experiences when solving problems in biology? (5) Mindset: What are the beliefs that students have in their abilities to solve the problems?
Variations in each of these aspects revealed three hypothesized student approaches to problem solving in biology that aligned with approaches to learning previously identified in higher education (Biggs, 1979; Entwistle et al., 1979; Case and Marshall, 2009). To maintain continuity with this existing work, we have named our three approaches as the surface approach, procedural approach, and deep approach. Here, the surface approach to problem solving is primarily characterized by the use of nonbiological concepts in knowledge and strategy, a desire to complete the course as the primary intention, minimal metacognitive reflection, and a fixed mindset about biology. In the procedural approach, students develop pattern-based processes from prior experience with course-specific material but do not rely on a conceptual understanding of biology; the metacognition, intention, and mindset aspects of this approach reflect complex dynamics between interest in biology as a discipline but a fixed mindset with regards to the specific genetics course in the study. The deep approach is characterized by a strong emphasis on biological concepts and pronounced interest in the intellectual challenges afforded by participation in biology and/or genetics.
In the sections below, we detail the variations in each aspect along with the three hypothesized approaches to problem solving in undergraduate biology education. Although most participants displayed variations in all aspects (i.e., knowledge, strategies, intention, metacognition, and mindset) that correlated within one approach (i.e., surface, procedural, or deep), we also identified some participants who displayed hybrid combinations of variations related to distinct approaches in different aspects. Such inconsistencies within an outcome space can occur when individuals are transitioning from one approach to another or are at a liminal state in the learning process (Cousin, 2006; Land et al., 2014). Therefore, as we will further elaborate in the Discussion section, we do not necessarily view these approaches as fixed for a given participant in time and, in fact, propose that the variations we identified may be opportunities for fluidity in supporting how students can shift between approaches and towards the deep approach in the classroom.
Knowledge
Existing studies generally distinguished the knowledge underlying different problem-solving strategies as being conceptual (grounded in disciplinary concepts) or nonconceptual (not utilizing concepts in the discipline; Walsh et al., 2007; Lönngren et al., 2017; Sung et al., 2022). In this study, we find that the binary distinction to be insufficient to capture the observed variations. While the surface and deep approaches are aligned with nonconceptual and conceptual cognitive knowledge, respectively, participants using the procedural approach cannot be fully characterized by either variation and instead demonstrate elements from both conceptual and nonconceptual knowledge. We can only fully capture the range of variations and describe all three approaches by considering two dimensions to knowledge emerging from the data: whether the knowledge involves biological concepts and what the participants consider as their source of the knowledge.
Key features of the surface approach in the knowledge aspect are a lack of biology, both in concept and in the source of the knowledge. For example, William described:
Usually what I do whenever I start a problem [is to] try to look at which ones are bogus answers. I look for the ones that don’t really make much sense and then from there I try to think about it. If I don’t necessarily know it, like with one of the questions, I kind of try to logically figure it out.
The knowledge used in William’s approach is devoid of biological concepts, and the primary source of knowledge is prior experience with the format and/or style of the question. In the excerpt, William focused on identifying self-described “bogus” answers in the question without mentioning any biological concepts for his initial attempts to solve the problem. William also hinted at a shift in his approach to use logic in figuring out the answer, and we will further explore this type of shift in the Discussion section.
The knowledge aspect in the procedural approach, similarly to that in the surface approach, does not rely on conceptual understanding of biology. However, participants characterized by the procedural approach drew on a different source of knowledge. Whereas in the surface approach, participants used knowledge based on the question itself irrespective of the course from which those experiences are drawn, in the procedural approach, students used their prior experiences with information specifically from this genetics course. For example, Martin described:
I worked enough problems that I saw, and also like in the solutions manual, I remember it stated that like a simple way you can tell the order of the genes was if there is only one type of like recombination that occurs, you can look at whatever occurs together.
Although Martin was clearly relying on knowledge sourced from other genetics problems in the course, he made no attempt to meaningfully connect this information to biological concepts. Therefore, even though participants using both the surface and procedural approaches did not incorporate biological concepts in their knowledge base when solving problems, superficial connections to biology may make an appearance in the procedural approach in the form of specific references to the course material. These examples highlight the variation in the knowledge aspect that distinguishes the surface approach from the procedural approach: Participants can draw on knowledge that is unrelated to biology (surface approach) or draw on information related to course material but use it in a way unconnected to biological concepts (procedural approach).
The knowledge aspect in the deep approach is characterized by its grounding in biological concepts, in contrast to that in the surface approach and the procedural approach. For example, Evan described:
Translocation is when a chunk from one chromosome breaks off and joins another chromosome and then the opposite chunk joins. So it’s just when chromosomes switch sections of DNA. I don’t how it’d affect map distance. It gets smaller, so it can’t be it. Yeah, that makes sense. It can’t be a translocation because it should do the opposite. It should be bigger. And there would be a new phenotype. Or usually there’s a new phenotype in translocation, when translocation happens because where the chromosome breaks, a new phenotype is created because the protein that’s encoded for at that breakage point gets messed up. So that’s why it’s not a translocation. It’s an inversion.
Evan used biological concepts and his internal conceptualization of this knowledge. Based on Evan’s logical reasoning, we argue that the source of knowledge went beyond simple recall of concepts from course material, as Evan was drawing inferences and making connections using his conceptual understanding.
Participants using the deep approach similarly drew on knowledge that originated from the course material and was grounded in their own understanding of biological concepts, reflecting a deep engagement between the participants as learners in the course and the course material as part of the learning environment. In contrast, while participants in the procedural approach also draw on knowledge that originated from the course material, this knowledge was grounded in processes that allowed the participants to bypass biological concepts as they solve the problem.
Strategy
Variations in the knowledge aspect across the three distinct approaches provide a valuable lens with which to situate our current and prior work related to the strategy aspect. Contextualizing the strategy aspect with variations in the knowledge aspect allows us to identify connections between these two aspects within each of the three distinct approaches. Below, we apply this analysis to data from new participants specific to the current study as well as unreported data for Samuel, Kylie, and Michael (2022) from the previous case-study paper.
In the surface approach, the absence of biological concepts in the knowledge aspect likely reinforces a strategy that relies on information from a nonbiological source, such as the format, style, and/or language of the question itself. For example, William shared:
Generally, I don’t usually go with the nonpossible, so I pretty much cross that out from the beginning, because logically, I think there is something that goes on between one of the first couple of choices.
William’s strategy is based on the logic that it is necessary to eliminate nonpossible answers first, and from previous experience, those are typically the first few choices. Consequently, this strategy could potentially be applied to questions of a similar style in different contexts, not necessarily just in the genetics course. Similarly, Samuel explained how he eliminated one of the options in a multiple-choice question based on the structure of the options:
You realize that out of these four [options], like three of the four, like “describe four progeny being created”, so this one is two and two, this one is two and two, and this one is one, one, one, one, one, and this one is only one. So that’s another reason why [Option] A seems wrong, because it’s the odd one out in that sense, which again is not like super good related to biology skills.
The strategy aspect in both the surface and procedural approaches is characterized by patterns of process based on prior experience with similar problems. However, the knowledge base for the procedural approach relies on course-specific information such as practice problems, examples from class, and even (unintentional) instructor cues. Nonetheless, participants characterized by the procedural approach still focused on the nonconceptual features to support a strategy that allowed them to reason through the problems without conceptual understanding. For example, Martin shared:
I just looked at the numbers to see which was a double recombination and which one was a single recombination, and that is the way it was set up, the largest one was the parental and then smallest one was like the most recombinations. I think the most [the instructor] did was two. So I look at these numbers, and the smallest number is usually the double, and the largest is the parental. Again, I don’t really know why. I just know that the higher the frequency, the further away they are from each other, or the more like normal they are.
Martin acknowledged an explicit awareness of how to do the problem without any understanding of the concepts. Similarly, as seen below, Kylie explained her elaborate process for how to solve a recombination problem by identifying pair-wise additions that arithmetically summed to an even round number while also gauging the complexity of the problem based on the point value assigned to it. She further implied toward the end of this excerpt that other students in the course also used this type of strategy:
Also, it really helps because [the instructor] makes the numbers add up really nicely together, and you know if you’re doing it right, if you get an even number. So like here, you get 100. (…) So I knew that like you add 26 and 24, and you add 27 and 23, you get 50 each. So like that helped too. I mean, he said that out loud to us once. He was like, I’m not going to ask you to, it’s not a math test. It’s not like an understanding, but it’s a clue. And also, the point values tell you how difficult the problem is going to, like how difficult the explanation is. So like, sometimes he asks if, are these genes linked, and it’s like two points, then you know that it’s yeah they’re all linked, or they’re not linked. And if it’s more than that, then you know there’s something else going on. And it’s true every time. Don’t tell [the instructor] that, ’cause all the [other students] will hate me!
As opposed to processes based solely on the question itself, and absent any course context as seen in the surface approach, participants using the procedural approach were reliant on information related to the specific course material. The processes ranged in complexity from a simple single-step recall from course material to connecting multiple pieces of information and/or steps to form a more complex set of associations, as respectively exemplified by Martin’s and Kylie’s descriptions above.
The deep approach is also characterized by a range in complexity, from a single-step conceptual connection to an elaborate process involving multiple concepts. Instead of connecting course-based information and perceived rules to generate essentially a heuristic strategy as seen in the procedural approach, the strategy in the deep approach relies on connecting conceptual ideas grounded in biological understanding. For example, in a single-step conceptual connection, Evan described nondisjunction:
[Nondisjunction is] when the, in meiosis whenever all the chromosomes line up, and then the spindles attach, and pull them apart to separate them, haploid gametes. If for some reason, it doesn’t do that correctly, and it doesn’t split the two homologues up then, and say one gamete gets both, and one gets none, that’s a nondisjunction.
While it is possible that Evan could have memorized such a definition from a textbook, there was a certain fluency in how he described the idea using language grounded in biological concepts. In contrast, in the procedural approach, Cara described homologous chromosomes explicitly through references to visualizations provided in class: “In lecture, the visual [the instructor] put up made it seem like they, they’re similar, and so I’m like, and they look according in the visual [the instructor] put up in lecture, it looked like they were similar in size.” Evan further elaborated on how he solved a recombination problem, connecting multiple concepts and processes:
First, what you’d do is just draw the two chromosomes next to each other, and then you have to figure out which genes in what order. You knew from these numbers that these are the two wild-type phenotypes, and so in that worm, you know that the two chromosomes have these alleles, and you know the order, and you know which ones are on it, and it’s asking you what crossover would give rise to a wild-type progeny. You know that you have to get all, you have to get big C, big W, and big D. So the only way that happens is a crossover right there, which would give you all dominant alleles. So the hardest part is just figuring out what the gene order is, and which alleles are on which chromosome. And the way you get that information is from these two parental classes.
Even though Evan seemingly used what appears to be a heuristic process with sequential steps, it was also evident that this strategy involved connecting each step together using his conceptual understanding of the biological system. This aligns with the knowledge base identified for the deep approach, which is centered on the participant’s own understanding of biological concepts. It is interesting to note that Evan shifted to a formula-based strategy only when he did not understand or remember the meaning of a concept, i.e., interference in the excerpt below. We will further explore this type of shift in the Discussion section.
The last part is where applicable calculate a value for interference. And that’s just an equation. It’s one minus d, number of double recombinants, divided by the expected number of double recombinants. (…) That’s just the equation for interference. (…) Just whenever the number of double recombinants you get deviates from what you expect. Then interference is happening. I don’t know exactly why, or I don’t remember.
As we consider the connections between the two cognitive aspects of knowledge and strategy through the lens of situated cognition, we see that each approach begins to capture distinct interactions between the learner and the environment as learning occurs. For example, the near absolute avoidance of conceptual understanding and even in relation to the course material seen in the surface approach is suggestive of a lack of interaction, or disengagement, between the learner and the learning environment. The procedural approach is characterized by an almost transactional interaction; the selective knowledge that is observed and retained from the course is specific information such as formulas and patterns in previous questions that allows the learner to solve the problem without application of concepts. In the deep approach, the strong conceptual connections within both the knowledge and the strategy aspects suggests a deeper engagement in the interaction, in which the learner is internalizing and integrating concepts seen in the learning environment into their own understanding.
Intention
We characterize the intention aspect as the self-identified reasons why participants were interested and motivated to engage with problems from this course and/or the discipline. To describe the intention aspect, we consider two dimensions emerging from the data: desire and demand. We describe desire as being representative of the participant’s interest in being intellectually challenged and/or connected to the subject matter, likely correlating with interest in biological concepts and/or problem solving. On the other hand, demand captures the participant’s underlying motivation in the educational enterprise at hand, either specifically in the genetics course from which these problems originated or in the discipline of biology as a whole. Similar to our analysis of the knowledge aspect, the use of two dimensions allows us to more fully describe the variations in the intention aspect among the three distinct approaches.
Broadly, the intention aspect in the surface approach shows a disinterested perspective towards biology as a discipline, suggesting low interest in the underlying biological concepts and/or problem solving. There is a lack of desire to connect with the course material; consistently, the primary demand is motivation to complete the course and move past the content, external structures set forth by other people such as requirements for future careers or the instructor. For example, Samuel described:
I wish I was learning biology, but at some point, I have to square with the fact that I’m not. I hate this class, and I don’t think I like these kinds of premed sciences that much. You just have to get through it and then, ‘cause when you’re a doctor, I’m not going to be dealing with biology. So you just have to get through it, which is why I’m not giving it up just yet.
Similarly, William said:
I think I have never been like a cheat-for-the-test kind of person. I think learning it is probably better, but sometimes I don’t see things coming at all, and if I could be exposed to that during a review session or during class sometimes. We get taught how it works, but I don’t exactly see how that translates to problems at times, so I think getting exposed to problems more would probably help. I was trying to focus on what [the instructor] told us to study on.
In both examples, there was a lack of desire to connect to the material: Samuel did not consider the course learning biology and consequently hated the course, and for William, the perceived disconnection between what was taught and what was tested in the course frustrated him so much that he focused his efforts on what he was told to study. For both Samuel and William, this lack of connection to the course material reinforced the motivation to just finish the course.
The procedural approach is characterized by a combination of desire that is different from that of the surface approach and demand that is similar to that of the surface approach. For example, the demand of focusing on motivation to complete the course was also reflected in Kylie’s experience:
I thought it was kind of ridiculous that I knew all the information, yet I couldn’t apply it to anything, and I just think it’s a really bad way to test. [The instructor] did go through practice problems, but they were always straightforward. Then they threw you a curveball at you on the test. I’m used to tests being challenging and not presenting the information as you see it when you study, and that’s fine, but I feel like this had to do a lot with like logic and just like. I don’t know, I just, it’s not a logic class, you know. It’s biology. You just memorize how it’s done. I’m a little bit bitter about it. I kind of felt really frustrated, and the only reason why I would’ve bothered to play the game is because I’m competing with everyone else to do well, so I knew everyone else would.
Kylie’s response demonstrated her frustration that the genetics course material included on the exam did not reflect her preconceived idea of what biology should be, instead consisting of questions that were more akin to logic questions to her. This frustration underscored her need to play the game, with her primary demand also focused on just finishing the course. However, what distinguishes Kylie’s response from those of Samuel and William is her desire to be challenged on the test and/or see things presented differently in the course, suggesting interest in the underlying biological concepts and/or problem solving. This distinction is particularly striking given that William cited those exact reasons for not intellectually engaging with the class. This variation in desire, in this case a demonstrated interest to be intellectually challenged, is what distinguishes the procedural approach from the surface approach.
Similarly, Martin described:
Genetics was not my favorite. I was just like, I have to get through it to be honest. I just like really tried to cover as many problems as I could until I found a trend in the problems. And I don’t like that it is not really a way of learning, I don’t feel like. In molecular biology, because there were not so many problems per se, you really had to get an understanding of the concepts and like all the basics to really understand that, and I like that a lot better than just working problems to find an answer. Even in math the trend was always there, but you actually had to understand the basics. You couldn’t just memorize a typical pattern in the problems, because you know that wouldn’t help you all the time. These [genetics problems], if you found a pattern nine times out of ten, it would be the same, which is okay, but if the basic understanding of the concept isn’t there, I don’t feel like it is very helpful. I like learning a lot. That is why I am in college, I don’t like just doing problems and finishing it up there. I memorized how to do it, and applied it on the tests, and then pretty much forgot about it, because I wasn’t very interested in it.
Here, Martin expresses their feelings about genetics through comparisons to other courses he has taken, highlighting features that simultaneously underscored his sense of learning in those spaces and his sense of not learning in genetics. Similar to Kylie’s experience, Martin had a strong desire to be intellectually challenged. However, the seeming mismatch between how he expected those intellectual challenges to manifest in the course and how he actually did on the exam resulted in focusing the demand on his motivation to just move past the course.
Both procedural and deep approaches share the desire to be intellectually challenged; what distinguishes between the two is the variation in demand, where the deep approach is characterized by a self-generated motivation to learn both for themselves and in the course. For example, Evan shared:
I mean the test wasn’t, [the instructor] didn’t make it as hard as he could’ve, could’ve made it a lot trickier. But it’s just, there’s a lot of different things that could happen, and you have to really understand what causes those things to happen so that you, ’cause you can’t just memorize every scenario. You just have to logically work your way through it. It’s just a long process, and if you do that, you can get the right answer, but a lot of people struggled with that ’cause they don’t get that initial foundation to build off of.
Similarly, Sean explained:
I also appreciate the fact that there are some more difficult questions, such as these ones, that actually require you to, you know, work out a calculation or think of something in a new way that actually involves some sort of thought process, not just simple memorization of the notes, and then go to the test, and recognize the pattern, and just circle it. So, I appreciate the challenge. The bio class doesn’t really have that rule that you have to get a certain percentage right, it just encourages you, not just to, you know, focus on getting the right answer, and stress about that. It doesn’t matter if I get it right or wrong, as long as I learn.
These responses from Evan and Sean demonstrate that both participants appreciated intellectual challenges, having scenarios that were possibly beyond what had already been shown and citing the questions that required some sort of thought process compared with memorization of the notes. In turn, these participants indicated that their intention supported a demand that is largely driven by an internal motivation to deeply engage with course material. Moreover, the mechanism of this deeper engagement aligns with both the knowledge and strategy aspects already identified for the deep approach, where participants drew on their own conceptual understanding and form connections between these concepts to deduce a solution to the problem.
Similar to how we observed interactions between the knowledge and strategy aspects, it is possible that the intention aspect also aligns with other aspects within each approach. For example, avoiding the use of biological concepts in both knowledge and strategy in the surface approach could reinforce the notion that the participants were not learning biology, and the lack of desire or demand in their intention could in turn reinforce a deliberate choice to avoid the use of biological concepts to solve problems. Participants described above in the procedural approach commented on intellectual challenges when working through the problems; however, these challenges were sources of frustration that ultimately led to intellectual disengagement, reinforcing the use of biological concepts in strategies that supported completing course requirements rather than deeper conceptual learning.
Metacognition
Based on ideas emerging from the data, we focused this aspect on how participants reflected on their experience solving problems based on metacognitive knowledge and metacognitive process or regulation. Specifically, we examined how much participants were aware of their own knowledge and strategies in relation to the problems (metacognitive knowledge) and how the features of the course may influence connections between such awareness and problem solving by participants (metacognitive process or regulation).
The surface approach captures a largely passive engagement with the reflective process used by participants, with limited metacognitive knowledge and metacognitive process in their reflections. For example, Samuel described: “I think you’re gonna expose how superficial my understanding is. I’m not sure if that’s right. So I guess again, in explaining it, I’m realizing that I don’t know it as well as I thought I knew it.” Similarly, William states: “I know the thought process behind it. I think my biggest issue with genetics so far has been like I know what is going on, [but] I don’t know how it [is] going on.”
Both participants were clearly metacognitively aware of the limitations of their biological knowledge, recognizing that they had significant gaps in their conceptual understanding. Samuel named his understanding as superficial, whereas William identified his lack of understanding by distinguishing between his familiarity with the topic of what was going on versus his lack of the conceptual knowledge needed to solve a problem and explain how it was going on. In Samuel’s case, beyond identifying a sense that the course was not biology, there was limited reflection on metacognitive processes, with very little reflection on the additional factors that contributed to his experience. Similarly, as described earlier, William proffered only a superficial reflection on factors that would shape his metacognitive processes: “We get taught how it works, but I don’t exactly see how that translates to problems at times, so I think getting exposed to problems more would probably help.” In both cases, metacognition in the surface approach reflects some level of metacognitive knowledge and almost no metacognitive process, reflection that connects the knowledge and strategy aspects with broader contextual factors that may influence problem solving.
The procedural approach is characterized by a degree of reflection that captures a greater amount of metacognitive knowledge though with still a limited amount of metacognitive process, resulting in disconnections or incompatibilities between their increased metacognitive knowledge and their limited recognition of metacognitive processes. Specifically, participants using the strategic approach can recognize their own content knowledge and a set of expectations of what is necessary to achieve success in problem solving. While participants reflected on expectations of how the course should support them as learners, the contextual factors that shape the limited metacognitive processes that emerged as part of this reflection were often viewed as oppositions to their success. For example, Kylie indicated:
I have a friend that took this over the summer and is really good at logic problems and puzzles. Like he’s the best and he got like 95 [percent] on all his exams because of it. Even though I know for a fact I studied way harder than he did, and I probably knew the information better. It’s just like, you shouldn’t have a test that people do well because they’re good at taking it. It should be because you know the information better, you should do well, better than others, I guess.
Here, Kylie implicitly recognized that she was not as proficient as her friend in logic problems, conveying limited metacognitive processes. She explicitly laid out contextual reasons in her reflection for this limited metacognitive process, suggesting that her performance was due to the tests being a measure of logic rather than knowledge. In doing so, the reflection provided a more complex lens into her experience of problem solving than participants using the surface approach. Similarly, Bailey described:
I couldn’t get some of [the concepts]. I’m not sure. Some of these really screwed me up. I think my problem was that I never actually learned what was happening or like, why it would happen. It was like, okay, I see these numbers and so [the instructor] kinda asked the same questions, so I would try to figure out how to solve them instead of actually learning it. It definitely put me in a robot mode a little bit, maybe practice problems in class or explanation, like step-by-step what conceptually I guess is happening. I know [the instructor] did a little bit of that, but like I feel like a lot of people struggle with this concept.
Here, Bailey demonstrated her metacognitive knowledge by explicitly recognizing that she never learned some of the concepts and instead developed algorithmic strategies to solve the problems. Although she was less direct than Kylie, Bailey also highlighted limited ability for metacognitive processes, instead focusing on contextual reasons that would explain her lack of content knowledge and her reliance on nonconceptual problem-solving strategies. For instance, she cited how being asked similar questions and the lack of opportunity to review the conceptual basis for problems contributed to her not learning the biological concepts.
In contrast, the deep approach is characterized by reflections that recognize and connect metacognitive knowledge related to the expectations necessary to achieve success and a high degree of metacognitive processes that are essential for problem solving. These participants were able to distinguish specific patterns and processes that they used to improve their conceptual understanding and problem-solving capacities. For example, as described earlier, Evan noted that on the tests there were “a lot of different things that could happen, and you have to really understand what causes those things to happen” and as a result “you can’t just memorize every scenario.” Evan reflected on a difference in lower-order cognitive problems that required recall and higher-order problems that required application, and then he provided a strategy for how to solve the latter type of problems, stating that “[y]ou have to break it down, and you have to start from the beginning in terms of like segregation and stuff like that.” Similarly, Sean noted that:
There were questions on the test that I found to be very fair, very straightforward. If you had studied the notes and gone over the material and done the reading you would be in a good position to breeze through them, but I also appreciate the fact that there are some more difficult questions that actually require you to work out a calculation or think of something in a new way that actually involves some sort of thought process, not just simple memorization of the notes and then go to the test and recognize the pattern and just circle it.
Similar to Evan, Sean distinguished between cognitive levels of problems and reflected on his own metacognitive processes on what he needed to do to succeed, such as going over the notes and reading. Both participants indicated that the primary expectation of the course was to provide opportunities for developing problem-solving processes, typically in the form of higher-order cognitive problems. The alignment of metacognitive knowledge and metacognitive processes in these participants reflects a more cooperative relationship between the participants and the learning environment, whereas the reflections in the procedural approach highlight a more combative relationship or tension between these factors. In contrast, the minimal reflection seen in the surface approach represents a more passive engagement with metacognitive knowledge and metacognitive processes for problem solving.
Variations in the metacognition aspect also correlate with those in the aspects described earlier. The surface approach suggests a lack of connections to biological concepts and also a passive relationship to the course, both in intention and metacognition. The procedural approach focuses on a transactional view that uses course-based knowledge and strategies to complete the course requirements, correlating with greater metacognitive knowledge but still limited metacognitive processes. The deep approach emphasizes biological concepts in the knowledge and strategy aspects, an intention to be intellectually challenged and to engage with course material, and a connection between both metacognitive knowledge and processes.
Mindset
Students can operate on a continuum of attitudes and beliefs between fixed mindset, believing that their intelligence and ability to do well cannot be improved, and growth mindset, believing that such qualities are malleable (Yeager and Dweck, 2020). However, our data indicate that this nearly binary distinction of either a fixed or growth mindset as the two ends of the spectrum is not sufficient to describe the variations within this aspect for the three approaches. Instead, we articulate an additional dimension that further defines an intermediate point in the continuum between fixed and growth mindsets, i.e., whether participants viewed their learning from a deficit-based or asset-based perspective (Denton et al., 2020; Denton and Borrego, 2021).
The surface approach is characterized by attitudes and beliefs that learning cannot be improved as predetermined by certain factors, classic features of a fixed mindset. This variation also adopts a deficit-based perspective. For example, Michael shares:
I think people who have a natural aptitude towards biology maybe can figure [this problem] out. I have a friend who does really well on the tests, because she literally goes to the test and figures out the test. Like, she can read this and understand, think, and figure out what a homologous chromosome is. But I don’t have that kind of natural aptitude towards biology.
Michael then compares himself to another student and explicitly states that he believes that he will not be able to develop the needed aptitude for problem solving in biology, showing a fixed mindset toward his abilities to solve biological problems. He implies from a deficit perspective that he does not have an aptitude for biology, consistent with a fixed mindset that he will not be able to acquire the skills needed to solve the problem. He further characterizes this natural aptitude (or lack thereof) as something that is relevant for the entire field of biology. Michael’s reflections correlate with the idea of brilliance, that success requires innate, natural talent that cannot be taught (Rattan et al., 2012, 2018).
Whereas participants using the surface approach show an alignment with fixed mindset inherently consistent with a deficit-based perspective, we found that the procedural approach can be described by a growth mindset but also coming from a deficit-based perspective. For example, Bailey explains:
I didn’t have the background to understand it in class. I took AP Bio sophomore year in high school, so it wasn’t recent to me anymore. I could’ve done this [problem] five years ago, easily. Trying to learn it in the short time I had, I like, you know, I just didn’t have it down.
Bailey viewed her lack of success as due to her missing some foundational background or ability in terms of a deficit. However, what distinguishes the procedural from the surface approach is the attitude and belief that she could have been able to succeed if she had had more time to learn and/or if the knowledge from her AP Biology experience had been more recent. Bailey also situated this deficit as specific to this genetics course, not necessarily to biology as a discipline, unlike Michael as illustrated in the surface approach. Consequently, this view that there are some specific deficits particular for this one course preventing success implies that these participants believe that they would be able to improve if they were able to rectify the deficit. Bailey further ascribed the path to success and thus improvement as one influenced by external factors, such as time because the prerequisite course was taken, rather than how her work and effort in the course could influence her ability to improve and successfully solve genetics problems. Therefore, participants using the procedural approach share elements of a growth mindset but from a deficit-based perspective.
The deep approach is similar to the procedural approach in that both are described by a growth mindset; however, the deep approach focuses on an asset-based perspective instead of a deficit-based perspective. Participants using the deep approach conveyed the attitudes and beliefs that they and their peers could take specific steps to gain skills and become better at solving problems in biology, focusing on internal factors on what they could do to improve rather than external constraints that limit their success. For example, Evan shares that “[y]ou just have to logically work your way through [problems], and it’s just a long process, and if you do that, you can get the right answer.” Similarly, Sean mentioned that he enjoyed doing practice problems for the following reason: “[i]t just encourages you, not just to focus on getting the right answer and stress about that, and instead it doesn’t matter if I get it right or wrong, as long as, like, I learn.” Both Evan and Sean described that working through problems will aid them in learning, improving, and gaining the ability to become better, aligning with a growth mindset from an asset-based perspective.
As described earlier, the connections between the cognitive aspects of knowledge and strategy suggest that each approach encapsulates distinct degrees of interactions with the learning environment on the basis of what knowledge was used in each strategy. Here, by considering multiple other aspects beyond knowledge and strategy, we can begin to formulate a more coherent understanding of each approach. For example, we can see that the lack of connections to biological concepts in knowledge and strategy correlated with a passive relationship to the course, both in intention and metacognition, and subsequently with a fixed mindset of not being able to improve in learning biology. In contrast, a transactional view as described by the knowledge and strategy aspects for the procedural approach fails to capture the sense of internal conflict between the student and the course environment as reflected in the intention, metacognition, and mindset aspects. On the other extreme, the depth of interactions between the learner and the learning environment seen in the knowledge and strategy aspects for the deep approach also correlate with the depth of engagement and cooperative relationship between internal factors of the participants and contextual factors in the course. By considering multiple dimensions of a learner’s experience with their environment through the lens of situated cognition, we see that individuals characterized by each of the approaches can have complex relationships with the course and course material. As we will discuss later, this creates potential opportunities for the instructor to acknowledge and address features of this interaction to potentially shift individuals’ problem-solving from one approach to another.
DISCUSSION
Our work provides one of the first examples to examine student approaches to problem solving in a broader and more holistic perspective that can potentially integrate multiple different aspects. The outcome space emerging from our data (Figure 2) represents a hypothesis for how variations in each of the five aspects (i.e., knowledge, strategies, intention, metacognition, and mindset) are connected to one of the three approaches (i.e., surface, procedural, or deep). As we considered problem solving as a learning phenomenon through the lens of situated cognition, it is perhaps not surprising that we identified three approaches that are aligned with the previously characterized student approaches to learning (Biggs, 1979; Entwistle et al., 1979; Case and Marshall, 2009), even though we did not set out to constrain ourselves to a specific number of approaches in the outcome space. Classically, approaches as defined in phenomenography consisted only of strategies and intentions (Marton, 1988; Case and Marshall, 2004). Here, our outcome space expands the number of aspects to include knowledge, metacognition, and mindset in addition to strategies and intentions.
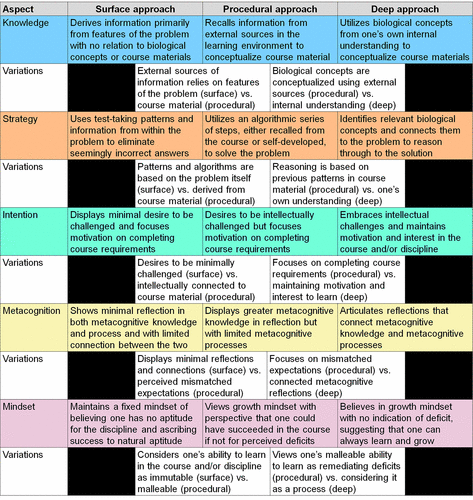
FIGURE 2. Outcome space for different student approaches to problem solving. This outcome space articulates hypothesized relationships among the three approaches illustrated by specific variations across five aspects: the aspects of knowledge (blue), strategy (red), intention (green), metacognition (yellow), and mindset (purple). Under each aspect, variations are described to distinguish between two neighboring approaches, either surface versus procedural or procedural versus deep.
The three approaches to problem solving span a continuum from deliberate avoidance of using conceptual information to intentional application of biological concepts. The knowledge and strategy aspects align with other studies that have observed both biological and nonbiological strategies for problem solving (Schoenfeld, 2016; Sung et al., 2022). Moreover, we show that the knowledge and strategy aspects may be connected with other aspects including intention, metacognition, and mindset. These results provide an expanded view of factors that may impact how students solve problems beyond considering the domain-specific factors of declarative, procedural, and conditional knowledge (Prevost and Lemons, 2016; Avena et al., 2021).
Previous phenomenographic studies
Our current work is further situated in other phenomenographic studies characterizing problem solving across STEM disciplines (Walsh et al., 2007; Lönngren et al., 2017; Dringenberg and Purzer, 2018; Dahlgren et al., 2021; Sung et al., 2022), which previously identified variations in problem solving in a limited number of aspects (Figure 3). Therefore, our results extend these previous findings by providing an outcome space that incorporates a larger number of aspects and synthesizes the different aspects into a coherent hypothesis as a model for student approaches to problem solving.

FIGURE 3. Outcome space with mapping of prior work characterizing approaches to problem solving in STEM. Our outcome space is shown encompassing different approaches identified in previous phenomenographic studies, which are highlighted by different colors: sustainability in engineering education (Lönngren et al., 2017) in blue, physics education (Walsh et al., 2007) in red, collaborative problem solving in engineering education (Dringenberg and Purzer, 2018) in green, physiology education (Dahlgren et al., 2021) in yellow, and biology education (Sung et al., 2022) in purple. The highlighted text represents the approaches as named and described in each of the original studies. Some of these approaches span multiple aspects. For example, the cognitive-based clinical reasoning approach in yellow aligns with the knowledge, strategy, and mindset aspects but not the intention and metacognition aspects.
In engineering education focusing on sustainability development, four problem-solving approaches were identified (Lönngren et al., 2017) that overlap with the variations in the strategy aspect in our outcome space (Figure 3, blue). In the simplify-and-avoid approach, students worked on the problems without structure or meaning; in the divide-and-control approach, students did not consider the context of the problem or draw upon knowledge of the field (Lönngren et al., 2017). This lack of conceptual application in both approaches parallels the strategies seen in the surface approach in our current study. In the isolate-and-succumb approach, students show some understanding of the concepts needed to solve the problem, but their responses remain superficial, disjointed, and lack recognition of the true complexity of engineering systems (Lönngren et al., 2017). This superficial and limited understanding of the system, despite drawing on information from the course, aligns with strategies in the procedural approach. In the integrate-and-balance approach, students show the most thorough understanding of the problem and apply appropriate engineering concepts to solve the problem (Lönngren et al., 2017), aligning with strategies in the deep approach.
In physics education, approaches to problem solving were identified (Walsh et al., 2007) with variations corresponding to the knowledge and strategy aspects in our outcome space (Figure 3, red). In the approach named no clear approach, students relied on features of the problem with no conceptual knowledge from the course to solve the problem (Walsh et al., 2007), aligning with knowledge and strategies in the surface approach in our current study. In two plug-and-chug approaches, students used heuristics that were either devoid of meaning in physics (memory based) or incorporated some conceptual understanding (structured), but this conceptual understanding was applied in a formulaic manner with limited meaning of the complexity of the system (Walsh et al., 2007). These features correspond to the knowledge and strategies in the procedural approach. In the scientific approach, students drew upon their own understanding and knowledge of physics principles to address each problem, aligning with knowledge and strategies in the deep approach.
Similarly, the previous study on student problem solving in biology (Sung et al., 2022) identified variations only in the knowledge and strategies aspects in the new outcome space presented in this paper (Figure 3, purple). In the nonconceptual approach, students utilized clues identified in the formatting of the question itself or in the language of the question to arrive at their final answer through a process of eliminating seemingly incorrect answers (Sung et al., 2022). These features correspond to the knowledge and strategies of the surface approach in our current study. In another nonconceptual approach, students relied on algorithmic processes that lacked conceptual understanding and were instead based on patterns identified from either instructor cues or previous problems (Sung et al., 2022). These features correspond to the knowledge and strategies of the procedural approach in our current study. Lastly, in the conceptual approach, students drew upon their own conceptual knowledge and understanding of biology in order to address the problem (Sung et al., 2022), aligning with the knowledge and strategy aspects of the deep approach identified in our current study.
Collaborative problem-solving approaches in engineering centered on two aspects (Dringenberg and Purzer, 2018) aligned with variations in the intention and metacognition aspects in our outcome space (Figure 3, green). In one extreme, students using a completion approach focused primarily on completing the problem as quickly as possible and did not view their work as authentic engineering (Dringenberg and Purzer, 2018), aligning with our surface approach characterized by a motivation to just complete the course (intention) and limited self-reflection that viewed the coursework as not biology (metacognition). In the middle, students using a transition approach recognized problem solving as a needed and fulfilling process that could lead them to success in future engineering classes but resisted working through the ambiguity inherent in complex engineering systems (Dringenberg and Purzer, 2018), aligning with the conflicting desire and demand (intention) we observed in our procedural approach. In the other extreme, students using a growth approach viewed problem solving as an integral part of personal growth for becoming an engineer (Dringenberg and Purzer, 2018), aligning with our deep approach characterized by sustained interest and motivation in the discipline (intention) and the ability to reflect on the specific concepts and steps needed to solve a problem (metacognition).
Two approaches to problem solving identified in physiotherapy education (Dahlgren et al., 2021) span the knowledge, strategy, and mindset aspects in our outcome space (Figure 3, yellow). In one approach called cognitive-based clinical reasoning, students relied on a step-by-step problem-solving process that was algorithmic in nature and only depended on a superficial understanding of physiotherapy; these students also centered their own experiences rather than the patient experience in physiotherapy, thus limiting how they adapt their learning to respond to new situations (Dahlgren et al., 2021). These features share similarities with the variations in the knowledge, strategy, and mindset aspects characterized by the procedural approach in our outcome space. In the second approach called relational understanding of clinical reasoning, students used understanding and reasoning in physiotherapy, which provided a more complex view that connected the student and patient; these students also indicated learning was an ongoing process and acknowledged the need to continually adapt to patient needs (Dahlgren et al., 2021). These features all align with the variations in knowledge, strategy, and mindset in the deep approach.
Our study expands the current literature on student problem-solving approaches by providing a more comprehensive outcome space that include many different aspects of problem solving. The other outcome spaces for problem solving in biology, engineering, physics, and physiotherapy education can be largely correlated within the one from our study, with some minor exceptions only in the strategy aspect (Figure 3). In previous studies, strategies such as simplify-and-avoid versus divide-and-control (Lönngren et al., 2017) or memory-based versus structured plug-and-chug (Walsh et al., 2007) were separately identified within those outcome spaces; here, our data do not allow us to distinguish among these different strategies. Nonetheless, the similarities identified across these disciplines and in our work, which focuses on biology, illustrates that the outcome space emerging from this study may be applicable to other disciplines. Finally, by considering problem solving through the lens of situated cognition, our results allowed for an integration of various aspects that influence problem-solving, thus supporting the importance of sociocultural learning theories beyond cognition.
Implications for teaching
We observed some instances of participants switching approaches even within the same problem, such as Evan and William in this study and Michael in the previous study (Sung et al., 2022). Therefore, we posit that approaches to problem solving are malleable and that students can learn to adopt the deep approach. Prior work has indicated that changes in how a question is framed or how knowledge of biological concepts is activated can lead to differences in problem-solving strategies used by students (Weston et al., 2015; Avena and Knight, 2019). Similarly, student metacognition and mindset can shift based on the use of specific prompts (Case and Gunstone, 2002; Berthold et al., 2007; Tanner, 2012; Limeri et al., 2020); However, much of this work has focused within a limited number of aspects, with little to no exploration on how shifts in one aspect may impact other aspects.
By identifying variations in each of the five aspects (i.e., knowledge, strategies, intention, metacognition, and mindset), our results provide additional context for considering interventions that could potentially shift students between approaches (i.e., surface, procedural, or deep), for example, by targeting transitions from one variation to another within one aspect. As demonstrated by other studies in phenomenography on learning in higher education (Åkerlind, 2005, 2018), we hypothesize that interventions shifting students from one variation to another within some aspects could also lead to changes in other aspects. In biology education research, interventions promoting metacognition can shift students’ intention from the surface approach towards the variations seen in the procedural or deep approaches while improving cognitive learning outcomes (Conner, 2007; Tanner, 2012; Avargil et al., 2018; Dang et al., 2018). Across STEM disciplines, interventions that guide students away from fixed mindset toward growth mindset can positively influence intention and also cognitive learning outcomes (Fink et al., 2018; Muenks et al., 2020, 2021). Similarly, we speculate that providing students with structured guidance on how to become more aware of and to shape the thinking of others (Halmo et al., 2022) may spark changes in students’ intention from surface to procedural or deep approaches. For example, instructors who rely on small-group activities in class may wish to provide instructions, so that students have guidance on how to evaluate, question, and challenge their group members’ thinking or to elicit greater explanations from their peers (Halmo et al., 2022). Instructors may also wish to refer to recent evidence-based teaching guides on metacognition (Stanton et al., 2021) and problem-solving in biology (Frey et al., 2022).
To further consider implications for teaching, we draw upon the framework of cognitive apprenticeship (Hennessy, 1993). Instructors could utilize interventions based on modeling and coaching that encourage intended and/or actual adoption of knowledge and strategies based on biological concepts and scaffolding in higher-order cognitive questions that promote understanding of biological concepts (Tanner and Allen, 2005; Jensen et al., 2014; Cleveland et al., 2021), all of which are aligned with variations in the knowledge and strategy aspects of the deep approach. For example, when teaching problem solving, instructors can first link various concepts relating to the problem by explaining their connections and then demonstrate the conceptual steps needed to solve the problem (modeling). Next, instructors can provide time for students to work on similar problems and provide feedback during this process (coaching), before iterating through small-group activities with complex problems designed to expand students’ thinking (scaffolding). By directly modeling, coaching, and scaffolding the thinking process, instructors can encourage students to utilize conceptual strategies and draw upon biological knowledge when solving problems.
Similarly, instructors can take steps to promote student learning and metacognition through articulation, reflection, and exploration. Students who are able to internalize concepts and produce a mental model of the new information are more successful at learning (Bierema et al., 2017). As an example, instructors teaching problem solving in genetics can consider implementing a variant of the jigsaw intervention (Aronson, 1978; Premo et al., 2018; Baken et al., 2022). For example, instructors can provide several scenarios that are related but require distinct solutions; students can work in small groups on one scenario, with different groups solving different scenarios. Next, the class forms new groups that merge students who worked on different scenarios. In these new groups, students can work together to share their solutions and tackle new problems that synthesize ideas from these different scenarios. In such a manner, students describe their knowledge and reasoning to each other (articulation), compare different approaches to related scenarios (reflection), and solve broader problems that require the integration of different concepts and strategies (exploration). Throughout this time, students develop their conceptual understanding of the relevant biological concepts and familiarity with different approaches to problem solving. In addition, structured reflection prompts from instructors throughout this process can be used to spark metacognition.
The relationship among different aspects influencing each other as well as impacting student learning has been empirically demonstrated in other contexts (Case and Marshall, 2004; Limeri et al., 2020). Theoretically, within phenomenography, the outcome space represents descriptions that coherently define the different ways of experiencing a phenomenon across the identified aspects (Marton and Booth, 1997). Therefore, it is reasonable to hypothesize that by developing student metacognition or fostering an asset-based growth mindset in the classroom, we may be able to shift students toward the deep approach to problem solving. Together, we further speculate that there may be a complex, linked relationship among the five aspects of approaches to problem solving, and more work is needed to investigate the interaction among these different aspects.
LIMITATIONS AND FUTURE DIRECTIONS
We acknowledge that our results may not be broadly generalizable to biology education. Our study population includes students at a single institution who were enrolled in one specific course, albeit across several years. Furthermore, we chose genetics to be the disciplinary context for specific methodological reasons, and there may be differences in how students approach problem solving in other subdisciplines of biology. Nonetheless, the contribution of this study is the novel phenomenographic outcome space on student approaches to problem solving that incorporates many aspects previously observed in the education research literature across STEM disciplines.
Within our study sample, only a small number of participants described all five aspects of the outcome space, making it more challenging to draw inferences about the intersections between aspects and approaches. This limitation may be due to the variability in how loquacious participants were when reflecting upon one or more of the aspects. The interview protocol did not directly ask about each aspect, as they emerged from data analysis as part of the methodology in phenomenography (Åkerlind, 2005, 2018), Instead, our results illustrate how participants organically provided insights into these aspects when discussing their thought processes. Future work will be able to build upon this study by directly exploring the different aspects identified in the outcome space of approaches to solving problems.
We also did not explore what specific steps or sequential actions that students took to solve problems, as others have studied such heuristics (Prevost and Lemons, 2016; Price et al., 2021). Instead, this study focused on the aspects and variations in how students approach problem solving to generate complementary insights in the existing literature. Future work can explore how variations in these aspects intersect to influence each step of the problem-solving process.
ACKNOWLEDGMENTS
We are grateful to the study participants for their time, and we thank the course instructors for their support in providing the exam problems. We also thank R. Holmgren and G. Light for thoughtful discussions in the early phases of the study. This project was initiated with support by an institutional award from the Howard Hughes Medical Institute for undergraduate biology education under award number 52006934 and the Hewlett Fund for Curricular Innovation from the Weinberg College of Arts and Sciences at Northwestern University. S.K. and A.J.G. were supported by the Undergraduate Research Assistant Program in the Office of Undergraduate Research at Northwestern University. S.M.L. was supported in part by the Faculty Career Development Program at the University of California San Diego.