The Role of Scientific Communication Skills in Trainees’ Intention to Pursue Biomedical Research Careers: A Social Cognitive Analysis
Abstract
Scientific communication (SciComm) skills are indispensable for success in biomedical research, but many trainees may not have fully considered the necessity of regular writing and speaking for research career progression. Our purpose was to investigate the relationship between SciComm skill acquisition and research trainees’ intentions to remain in research careers. We used social cognitive career theory (SCCT) to test a model of the relationship of SciComm skills to SciComm-related cognitive variables in explaining career intentions. A sample of 510 graduate students and postdoctoral fellows at major academic health science centers in the Texas Medical Center, Houston, Texas, were surveyed online. Results suggested that interest in performing SciComm tasks, SciComm outcome expectations (SCOEs), and SciComm productivity predicted intention to remain in a research career, while SciComm self-efficacy did not directly predict career intention. SCOEs also predicted interest in performing SciComm tasks. As in other SCCT studies, SciComm self-efficacy predicted SCOEs. We conclude that social cognitive factors of SciComm skill acquisition and SciComm productivity significantly predict biomedical trainees’ intentions to pursue research careers whether within or outside academia. While further studies are needed, these findings may lead to evidence-based interventions to help trainees remain in their chosen career paths.
Growing the scientific workforce has been the focus of considerable effort, with particular emphasis on retaining emerging scientists who are already in the science, technology, engineering, and mathematics (STEM) pipeline. The National Institute of General Medical Science (NIGMS) annually channels major funding to didactic research training programs to develop a highly trained biomedical/behavioral research workforce. In 2012, $637 million of the total NIGMS budget was dedicated to training and to workforce- and diversity-development programs at the undergraduate, graduate, doctoral, postdoctoral, and faculty levels (Hall, 2013). Many NIGMS-sponsored program activities focus on increasing opportunities for mentoring and broadening scientific training opportunities, but these are largely focused on the undergraduate or early graduate levels, and few, if any, have evaluated the role of trainees’ scientific communication (SciComm) skill development in influencing career intentions.
SciComm skills are a critical driver of success in biomedical and behavioral research (BBR) careers. Without peer-reviewed publications and oral conference presentations, it is virtually impossible for aspiring academic researchers to compete for junior faculty positions or obtain grants. As trainees progress through the doctoral and postdoctoral stages of their training, solidifying their professional identities as scientists, the need to acquire professional-level SciComm skills becomes increasingly apparent (Johnson and Huwe, 2003; Nettles and Millet, 2006). At the undergraduate and predoctoral levels, students are focused most intently on learning scientific content and acquiring research skills, but as they begin to produce data independently, they must turn their attention to disseminating their findings. Postdoctoral fellows and, increasingly, graduate students must gain experience and confidence in publishing research articles, presenting at conferences, and participating in professional conversations with colleagues and mentors as part of the socialization process for entering the profession. Yet the prospect of academic writing and public speaking is typically not what attracts students to BBR to begin with, and many may not have fully considered that their career progression will depend on regular writing and speaking. Thus, a discrepancy may exist between the primary, discipline-related skill set that trainees are interested in using (e.g., laboratory experiments, study design) and the ancillary (extradisciplinary) one that they are obliged to use (SciComm skills) to succeed in their desired careers. It is as if one has spent many months planning (and paying for) a dream trip to a foreign land, only to discover on the way to the airport that one’s passport is expired and a visa is required.
Coming to understand the critical and continuous role of advanced communication skills in a scientific career can be sobering for trainees. By the time they reach the doctoral and postdoctoral stages, substantial human, financial, and educational resources have been invested to prepare them for careers as independent investigators. Minimizing late attrition is critically important. Identifying whether and how SciComm skills contribute to career intentions can inform the development of evidence-based interventions to support and sustain trainees’ academic career pursuits and guide their mentors’ efforts in building their SciComm skills.
The purpose of this study was to use social cognitive career theory (SCCT; Lent et al.,1994) to investigate the process of SciComm skill development and its relation to trainees’ intentions to continue pursuit of academic careers. In the following literature review, we first discuss the relevant linguistic background on the role of speaking and writing skills on professional development in the sciences. We then summarize SCCT (Lent et al., 1994) and discuss its utility and implications for guiding our investigation of the contribution of SciComm skills to development of a BBR career in academia.
REVIEW OF RELEVANT LITERATURE
Linguistic Background
The difficulty of developing professional-level SciComm skills is a widely acknowledged source of frustration for both trainees and faculty mentors (Eyres et al., 2001; Wang and Bakken, 2004; Aitchison et al., 2006, 2012; Cargill and O’Connor, 2006; Kamler and Thompson, 2006; Cameron et al., 2009, 2011, 2013; Kranov, 2009; Shah et al., 2009; Parker, 2012). One linguistic concept that helps to explain some of the difficulty is that of register, which refers to a special style of language an individual uses on particular occasions or in particular settings. Examples of register include formal and ceremonial language; intimate language spoken among family members and close friends; street slang; lay academic language that may be widely used by students; and highly specialized, disciplinary academic language used by doctoral students, postdoctoral fellows, and faculty (Prior, 1994). The academic scientific register is thought of by many as a more intellectually demanding level of standard mainstream English. But thinking of scientific register as a reflection of cognitive ability or innate intelligence can lead us to misjudge those who have not (yet) mastered it. It is more helpful to understand scientific register as a variety of language, or “sociolect,”1 acquired through extended exposure and socialization (Labov, 1966; Halliday and Martin, 1993; Bekker et al., 2001; Snow, 2010). For some trainees who may have had limited exposure to the academic scientific register of English and few opportunities to acquire it earlier in their careers, achieving professional-level proficiency in SciComm may require additional effort. This is relevant both for trainees who are nonnative speakers of English (L2s) as well as for native speakers of English (L1s) who grow up without exposure to this register.
The development of identity as a scientist has been shown to be a major contributor to student intentions to pursue STEM fields (Summers and Hrabowski, 2006; Chemers et al., 2011; Estrada et al., 2011). Language and all its components (accent, intonation, grammar, vocabulary, discourse style, and register) are potent markers of identity and belonging (Ryan and Giles, 1982; Valdes, 1988; Tannen, 1994; Lippi-Green, 1997; Preston, 1997; Baugh, 2000; Lev-Ari and Keysar, 2010), and pejorative judgments of linguistic differences can have real consequences for emerging scientists as they seek to define their professional identities. Research in social psychology indicates that speaking differently from the dominant group results in individuals being judged as less credible or knowledgeable, regardless of the content of their speech (Lev-Ari and Keysar, 2010). In an informal survey of 41 research trainees with primary languages other than English, conducted by C.C. in 2009 at the University of Texas MD Anderson Cancer Center, 81% agreed or strongly agreed that their language status had led to “missed professional opportunities,” such as being selected to first-author a paper, give a platform presentation, or have their verbal contributions recognized in meetings.
As all trainees, regardless of linguistic background, work to acquire a discipline-specific professional register, they must do so in three primary modes: free-form speech in conversation; scripted speech in presentations; and formal writing for manuscripts, abstracts, and other written products. In the following discussion, we will briefly review the unique challenges of each mode.
Speaking Skills
Mastery of professional oral communication requires two sets of skills, fluency in spontaneous conversation and a command of planned speech. One cannot “rehearse” for spontaneous communication; presentations, on the other hand, are often scripted and practiced. In the research setting, trainees must learn to speak convincingly at lab meetings and conferences in spontaneous conversation and also to excel in oral presentations that require planned and rehearsed performance. Both types of oral communication must navigate around potential pitfalls. In spontaneous conversation, one cannot be sure of avoiding mistakes, since conversation is emergent. In presentations, “mistakes” can be edited out and avoided, but the audience’s expectations of eloquence and organization are higher, and the pressure to appear competent in front of the public may limit the speaker’s willingness to fully engage with the audience, by answering questions, for example. Although some trainees are comfortable with both types of oral skills, many are challenged by one or both, especially if they are not adept at use of standard English or the register of scientific English. Trainees who aspire to speak professionally in academic environments may contend with uncertainty about personal speaking style or dialect (as in the case of first-generation graduates). If L2s, they may also feel uncertain about foreign accent, limited vocabulary, and grammar errors (Labov, 1966; Ryan and Giles, 1982; Valdes, 1988; Fought, 1994; Tannen, 1994; Lippi-Green, 1997; Preston, 1997; Baugh, 2000; Phinney et al., 2001; Lev-Ari and Keysar, 2010).
Writing Skills
While universities have begun to recognize the importance of scientific writing ability, scientific writing study is not a standard part of the research training curriculum. It is common for students to enter and exit graduate or medical school (Fischer and Zigmond, 2001) with no formal training in scientific writing. Many of the available scientific writing books or courses focus on the analysis of ideal forms (model research articles and grants) rather than on the generative process of creating and revising documents and are very rarely specialized for particular disciplines. Nevertheless, research trainees are expected to write in a professional academic register, characterized by a high level of density and abstraction, long and complex sentences, elevated and impersonal rhetoric, discipline-specific vocabulary and phraseology, and perfect grammar (Halliday and Martin, 1993; Martin and Veel, 1998; Fang, 2004; Snow, 2010). Most learn to write by imitating existing articles and receiving feedback from mentors (Aitchison et al., 2012). Mentors, for their part, are trained as researchers, not writing or grammar instructors, and indicate that they find it burdensome and difficult to teach about writing and provide feedback on documents (Pagel et al., 2002; Kranov, 2009; Aitchison et al., 2012; Cameron et al., 2013). Added to these barriers are affective obstacles, such as perfectionism, procrastination, and writer’s block.
In sum, the challenge of acquiring SciComm skills may present a potent deterrent, at least for some trainees, to sustaining a career goal of becoming an academic biomedical or behavioral researcher. The realization that one’s intended career entails a lifetime of writing and public speaking may, for some, overshadow the initial enthusiasm for designing and conducting experiments and making scientific discoveries. While structural reasons for leaving research careers (Fuhrmann et al., 2011; National Research Council, 2011), such as the lack of faculty positions and grant funding are undoubtedly important, no research at the graduate or postdoctoral level, to our knowledge, has been reported that explores the critical role of linguistic skills in the education of research trainees and how related challenges may affect trainees’ intentions to remain in research careers.
Theoretical Model: SCCT
Using a theoretical model grounded in SCCT, we investigated the role that acquisition of SciComm skills plays in the expressed intentions of BBR trainees to remain in research careers. SCCT was originally proposed by Lent et al. (1994), based on Bandura’s (1986) and Betz and Hackett’s (1983) work in social cognitive theory. SCCT theory has been elaborated by Byars-Winston et al. (2010) for ethnic and contextual variables and by Bakken et al. (2006) for gender. Because SCCT examines how academic and career-related behavior and decision making are related to individuals’ learning experiences and environment, it is a useful model for understanding the relationship of SciComm skill development to career intentions.
SCCT emphasizes the role of social cognitive variables—particularly self-efficacy beliefs, outcome expectations, interest, and goals—in enabling people to assert personal control through their actions. Self-efficacy (SE) is the individual’s personal beliefs about whether he or she can perform a defined task or set of tasks effectively; thus, it is distinct from self-confidence, which is a more global sense of ability or worth. Outcome expectations (OEs) are the individual’s beliefs about what would happen if he or she pursued that set of tasks: “What are the consequences if I do this?” Interest and goals reflect, respectively, the individual’s sense of engagement with and commitment to performing the tasks that lead to the desired outcome. Previous studies (Betz and Hackett, 1983; Fouad and Smith, 1996; Bakken et al., 2006) testing the SCCT model included such outcome measures as persistence (i.e., choice stability), goal (of achieving a degree), and intended academic-major choice.
Much empirical evidence supports the utility of SCCT in understanding the academic and career choices of undergraduate and graduate students in STEM fields. For instance, strong SE beliefs consistently figure prominently in predicting choice of and persistence in STEM majors, interest in STEM careers, and perceived STEM career options (Gainor and Lent, 1998; Kahn and Nauta, 2001; Byars-Winston et al., 2010). Lent et al. (2003) found that math and science SE and interests were significant predictors of goal intentions to pursue an engineering degree, and goals fully mediated the influence of these two variables on persistence in the chosen major across three academic semesters. More recently, theoretically based research into career choice and persistence in the biomedical sciences has shown that social cognitive variables play a demonstrable and significant role in shaping undergraduate and graduate students’ intentions to pursue STEM careers. For instance, longitudinal data have revealed that science SE (i.e., belief in one’s ability to conduct science-related activities) influences the building of identity as a scientist, which is related to commitment to a science career (Chemers et al., 2011; Estrada et al., 2011).
Because the SCCT model is well developed theoretically and empirically, we chose to build on it to elucidate aspects of career development in the biomedical sciences related to SciComm skills. Our model includes specific social cognitive variables of SCCT, such as SE, interest, and OEs. Consistent with our current study’s focus on individuals who have already selected and invested in advanced training for a specific career path, our proposed model defines the outcome variable as “intention to remain in a research career.”
A novel aspect of our model is the inclusion of SciComm productivity. We define SciComm productivity as those observable past and recent past behaviors related to producing SciComm products: writing manuscripts and abstracts, participating actively in research-related conversations, and delivering presentations to scientific and lay audiences. In accord with Bandura’s (1986) tenet that past performance is a source of SE, some previous SCCT studies have examined historical indicators of the perceived quality of the student’s performance (e.g., standardized test scores; Lent et al., 1993) or student self-report of understanding classroom material (Garriott et al., 2014) as precursors to the development of strong SE beliefs before career entry. In our model, “SciComm productivity” denotes trainees’ self-reported quantity of SciComm activity during the current training period. We did not attempt to evaluate the quality or acceptability of the communication products (Bandura, 1986). Our model for SciComm skills specifies doctoral and postdoctoral students rather than undergraduate students and focuses on discretionary, self-motivated production rather than on perceived quality of an externally imposed activity. Unlike undergraduates, who are assigned obligatory term papers and may receive comments or grades on the quality of their writing, doctoral and postdoctoral trainees are writing and speaking, at least in part, to meet their own self-defined goals of dissemination of results and participation in the research community and may prioritize quantity over quality. Thus, consistent with much previous research confirming the role of past behavior in predicting intention (Ouellette and Wood, 1998) and task-specific SE (Vrugt and Koenis, 2002), SciComm productivity seems likely to be an important factor in understanding their career intentions.
To summarize, we hypothesized a career model in which trainees’ intentions to remain in a research career are predicted directly by SciComm SE (SCSE), interest, outcome expectations (SCOEs) and productivity. Moreover, we hypothesized that SciComm productivity predicts SCSE as well. The hypothesized model is shown in Figure 1.

Figure 1. Hypothesized structural equation model of intention to remain in a research career.
METHODS
We assembled a multidisciplinary team including a biomedical scientist (S.C.), an educational (H.Y.L.) and a social (C.A.) psychologist, a linguist (C.C.), a writing instructor (C.D.B.) and a counseling psychologist (A.B.W.), each of whom has expertise and experience in conducting research, mentoring, teaching, writing, presenting, and working with a wide variety of research trainees and faculty. This team designed the study, developed or selected existing study instruments and analytical methods, and analyzed and interpreted the results.
Invited participants included graduate students and postdoctoral fellows in various BBR departments primarily at the University of Texas MD Anderson Cancer Center, Houston, Texas, but also at other tertiary academic institutions in the Texas Medical Center. Survey participants were recruited via email messages or invitation cards sent by our Scientific Communication Skills Study project coordinator. The survey was administered online and took 20–30 min to complete. Respondents received a $20 gift card to compensate them for time expended.
The protocol for this study (#2009-0409) was approved by the University of Texas MD Anderson Cancer Center Institutional Review Board on September 17, 2009.
PARTICIPANTS
Initially, 673 research trainees began the online survey. Six-hundred thirty (630) met the study criteria of being a doctoral student or postdoctoral fellow more than 18 years of age and completed the informed consent form. One-hundred twenty (120) quit the survey after completing only a few initial questions, yielding a final sample of 510 trainees. Respondents were 38% male and 62% female, 52.4% doctoral and 47.6% postdoctoral, and 22–60 yr of age (mean = 31.1 yr). The sample characteristics are shown in Table 1.
Variable | Category | Frequency | Percentage |
---|---|---|---|
Gender | Female | 316 | 62.0 |
Male | 194 | 38.0 | |
Citizenship | U.S. citizen | 280 | 54.9 |
Visa holder | 230 | 45.1 | |
Ethnicity | Hispanic | 58 | 11.4 |
Non-Hispanic | 452 | 88.6 | |
Race | Asian or Asian American | 222 | 43.5 |
Black or African American | 37 | 7.3 | |
White | 204 | 40.0 | |
Other | 30 | 5.9 | |
More than one race | 17 | 3.3 | |
Primary language | English | 255 | 50.0 |
Other languages | 255 | 50.0 | |
Academic ranka | Doctoral student | 257 | 50.4 |
Postdoctoral fellow | 233 | 45.7 | |
Other/missing | 20 | 3.9 |
DATA ANALYSIS
Measures
SCSE.
Using a 24-item, three-factor scale (Anderson et al., 2015), we measured SCSE for writing, presenting, and speaking, even if the participants had not yet performed the task (e.g., “Write and submit an abstract to a scientific meeting”; “Use the expected scientific style when speaking”). The coefficient alphas of the SE subscales for this study were 0.91 for writing, 0.89 for presenting, and 0.89 for speaking.
SciComm Interest.
Using a 12-item, three-factor scale (Anderson et al., 2015), we measured SciComm interest in performing for writing, presenting, and speaking tasks, even if the participants had not yet performed the task (e.g., “Giving an impressive oral presentation at a national scientific meeting”; “Asking questions of a presenter during a meeting”). The coefficient alphas of the interest subscales for this study were 0.85 for writing, 0.87 for presenting, and 0.85 for speaking.
SCSEs.
Using a six-item, one-factor scale (Anderson et al., 2015), we measured positive SCSEs for excelling in SciComm tasks (e.g., “Allow me to obtain a highly desirable academic faculty position”; “Be necessary for me to be recognized as an expert in my research area”). The coefficient alpha for the OEs subscale for this study was 0.83.
SciComm Productivity.
Trainees were asked to write in the number of SciComm tasks they had completed during their current training period. These included three types of writing tasks (“Prepared by myself a full first draft of a first-author manuscript [does not have to have been submitted]”; “Prepared by myself an abstract for a scientific meeting [does not have to have been submitted]”; “Written a portion of a manuscript with other authors [does not have to have been submitted]”); two presentation-task types (e.g., “Given a presentation at a national scientific meeting”; “Given a presentation within my department”); and three speaking-task types (e.g., “Asked a speaker a question during their presentation at scientific meeting”; “Explained my research to a group of experts”; “Explained my poster informally at a scientific meeting”). Because SciComm productivity had not been previously examined, we performed confirmatory factor analysis (CFA) to assure validity and reliability of our proposed eight-item, three-factor scale measuring the number of SciComm tasks completed during the current training period. CFA results are reported in Preliminary Analysis.
Intention to Remain in a Research Career.
Trainees’ intentions to remain in a research career were assessed with four five-point, Likert-type scale items ranging from 1 (strongly disagree) to 5 (strongly agree). These items elicited trainees’ intentions to 1) pursue the next-step position in research (e.g., postdoctoral fellowship, junior faculty position); 2) stay in a research career as a principal investigator (PI); 3) stay in a research career, but not as a PI; or 4) pursue a research career outside of academics (e.g., in industry). We performed CFA to assure validity and reliability of our proposed four-item scale. CFA results are reported in Result.
Approach
We conducted structural equation modeling (SEM) using Mplus, version 7, a multivariate statistical technique, to model the hypothesized relationships between multiple independent and dependent variables simultaneously. We proposed a model based on theory and previous studies, drew a path diagram to reflect the relations between the elements of the model, and analyzed the data to evaluate how well our hypothesized model described what was observed.
Before the hypothesized structural model was evaluated (Figure 1), the measurement model was evaluated (Figure 2) to test how well the study variables were measured. The hypothesized structural model featured one exogenous variable (SciComm productivity) and four endogenous variables (SciComm SE, interest, OEs, and intention to remain in a research career). To assess the individual relations between the measured variables (i.e., the individual items comprising each questionnaire) and latent variables or factors (i.e., the “topic” or underlying construct that each group of items measures), we used a measurement model before testing the structural model.
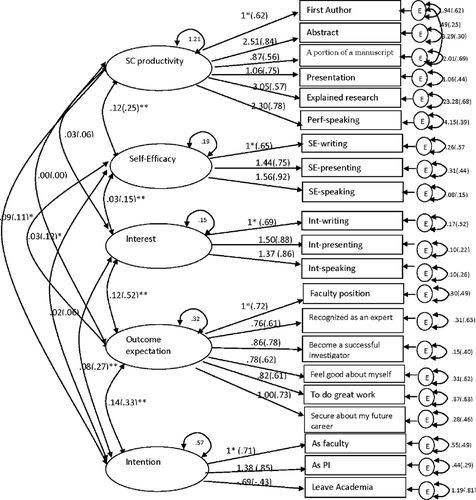
Figure 2. Measurement model of intention to remain in a research career. Unstandardized and standardized (in parentheses) parameter estimates.
To assess model fit, we used several indices: the comparative fit index (CFI), the Tucker-Lewis index, the root-mean-square error of approximation (RMSEA), and the standardized root-mean-square residual (SRMR; Hu and Bentler, 1999). Hu and Bentler (1999) have provided joint criteria to determine the goodness of fit of a model: the model is considered to have a good fit when the CFI is ≥0.96 and the SRMR is ≤0.10, or when the RMSEA is <0.06 and SRMR is <0.10. Muthén and Muthén (2002) indicate that minimum sample size for a complex structural equation model that includes missing data is 175 to achieve a power of 0.81. Thus, the sample size for this study was adequate to run SEM methods.
RESULTS
Missing data
Approximately 8% (n = 38) of the data were missing. To determine the missing data mechanism, we conducted a missing completely at random (MCAR) test (Little, 1988), which indicated these data were missing at random, χ2(2719) = 2583.17, p > 0.05. Therefore, we used the multiple imputation option in SPSS, considered to be one of the best solutions for handling missing data missing at random (Schafer and Graham, 2002), to impute missing data (10 imputed data sets; IBM SPSS Statistics, version 21), using an expected maximization algorithm.
CFA for SciComm Productivity
Before examining the construct validity for SciComm productivity, we observed that the distribution of the productivity data was not normal, and that the data were positively skewed (i.e., the bulk of the data were low values with a few outliers reflecting unreasonably high values). To control for this, we capped values on the eight tasks at the 95th percentile of the frequency distribution for each task variable (e.g., someone who said he or she had 50 departmental oral presentations in the current training period was given the 95th percentile value of 9). Means, SDs, and variable correlations are shown in Table 2. Next, beginning with an eight-item, three-factor scale, we conducted chi-square difference tests to compare one-, two-, and three-factor models. The one-factor model for the scale provided the best fit to the data, χ2(20) = 219.41, p < 0.001; CFI = 0.87; Tucker-Lewis Index (TLI) = 0.82 (Tucker and Lewis, 1973); RMSEA = 0.14; SRMR = 0.07. The model fit was further improved by deleting two low-loading items one by one and by adding one residual covariance. The final model, with six items and one residual covariance provided a good fit to the data, χ2(8) = 33.36, p < 0.001; CFI = 0.98; TLI = 0.96; RMSEA = 0.08.
Variable | 1 | 2 | 3 | 4 | 5 | 6 | 7 | 8 | 9 | 10 | 11 |
---|---|---|---|---|---|---|---|---|---|---|---|
SCSE in writing | 1 | ||||||||||
SCSE in presenting | 0.47** | 1 | |||||||||
SCSE in speaking | 0.60** | 0.69** | 1 | ||||||||
Interest in writing | 0.13** | 0.08 | 0.08 | 1 | |||||||
Interest in presenting | 0.03 | 0.19** | 0.10* | 0.59** | 1 | ||||||
Interest in speaking | 0.06 | 0.15** | 0.13** | 0.56** | 0.76** | 1 | |||||
Highly desirable academic faculty position | 0.12** | 0.04 | 0.11* | 0.33** | 0.26** | 0.26** | 1 | ||||
Recognized as an expert | 0.12* | 0.06 | 0.08 | 0.27** | 0.19** | 0.22** | 0.41** | 1 | |||
Become a successful investigator | 0.13** | 0.12** | 0.15** | 0.34** | 0.32** | 0.36** | 0.55** | 0.56** | 1 | ||
Feel good about myself | 0.08 | 0.09 | 0.07 | 0.25** | 0.28** | 0.32** | 0.47** | 0.36** | 0.42** | 1 | |
To do great work | 0.09* | 0.11** | 0.14** | 0.27** | 0.31** | 0.32** | 0.39** | 0.36** | 0.46** | 0.41** | 1 |
Secure about my future career | –0.03 | –0.01 | –0.04 | 0.30** | 0.30** | 0.29** | 0.55** | 0.42** | 0.56** | 0.47** | 0.45** |
Productivity in writing (first author) | 0.19** | 0.20** | 0.19** | 0.05 | 0.02 | 0.00 | 0.12* | 0.09 | 0.10* | 0.01 | 0.04 |
Productivity in writing (abstract) | 0.17** | 0.27** | 0.18** | 0.11* | 0.08 | 0.07 | 0.02 | 0.12* | 0.03 | −0.03 | 0.04 |
Productivity in writing (a portion of a manuscript) | 0.20** | 0.18** | 0.20** | 0.08 | 0.02 | −0.01 | 0.00 | 0.03 | −0.02 | −0.05 | 0.05 |
Productivity in presenting (given a presentation at a national meeting) | 0.12** | 0.20** | 0.14** | 0.07 | 0.08 | 0.05 | 0.03 | 0.08 | −0.01 | −0.00 | 0.04 |
Productivity in speaking (explained my research to a group of experts) | 0.14** | 0.31** | 0.33** | 0.05 | 0.04 | 0.05 | 0.07 | 0.05 | 0.03 | 0.06 | 0.07 |
Productivity in speaking (explained my poster…) | 0.14** | 0.21** | 0.17** | 0.15** | 0.16* | 0.09 | 0.03 | 0.06 | −0.00 | −0.02 | 0.07 |
Remain in academia as faculty | −0.03 | 0.06 | 0.04 | 0.27** | 0.22** | 0.23** | 0.32** | 0.16** | 0.20** | 0.05 | 0.17** |
Remain in academia as a PI | 0.11* | 0.02 | 0.05 | 0.26** | 0.21** | 0.14** | 0.36** | 0.12* | 0.19** | 0.08 | 0.20** |
Leave academia | −0.03 | −0.01 | 0.03 | −0.04 | 0.05 | 0.09 | −0.12** | −0.04 | −0.07 | 0.00 | 0.03 |
Mean | 3.77 | 3.67 | 3.60 | 4.55 | 4.34 | 4.37 | 4.27 | 4.40 | 4.48 | 4.34 | 4.06 |
SD | 0.68 | 0.85 | 0.74 | 0.56 | 0.66 | 0.62 | 0.79 | 0.71 | 0.62 | 0.71 | 0.76 |
Variable | 12 | 13 | 14 | 15 | 16 | 17 | 18 | 19 | 20 | 21 | |
SCSE in writing | |||||||||||
SCSE in presenting | |||||||||||
SCSE in speaking | |||||||||||
Interest in writing | |||||||||||
Interest in presenting | |||||||||||
Interest in speaking | |||||||||||
Highly desirable academic faculty position | |||||||||||
Recognized as an expert | |||||||||||
Become a successful investigator | |||||||||||
Feel good about myself | |||||||||||
To do great work | |||||||||||
Secure about my future career | 1 | ||||||||||
Productivity in writing (first author) | 0.05 | 1 | |||||||||
Productivity in writing (abstract) | –0.00 | 0.51** | 1 | ||||||||
Productivity in writing (a portion of a manuscript) | –0.09 | 0.50** | 0.43** | 1 | |||||||
Productivity in presenting (given a presentation at a national meeting) | –0.01 | 0.44** | 0.60** | 0.45** | 1 | ||||||
Productivity in speaking (explained my research to a group of experts) | 0.00 | 0.32** | 0.40** | 0.34** | 0.36** | 1 | |||||
Productivity in speaking (explained my poster…) | −0.01 | 0.37** | 0.67** | 0.37** | 0.54** | 0.43** | 1 | ||||
Remain in academia as faculty | 0.16** | 0.19** | 0.14* | 0.09 | 0.16** | 0.11* | 0.13* | 1 | |||
Remain in academia as a PI | 0.17** | 0.21** | 0.05 | 0.10* | 0.09 | 0.06 | 0.09 | 0.61** | 1 | ||
Leave academia | −0.03 | −0.20* | −0.05 | −0.10* | −0.05 | −0.07 | −0.06 | −0.31** | −0.40** | 1 | |
Mean | 4.25 | 1.68 | 3.34 | 1.64 | 1.10 | 4.49 | 2.66 | 4.16 | 3.70 | 3.29 | |
SD | 0.77 | 1.66 | 3.10 | 1.65 | 1.46 | 5.35 | 2.98 | 1.08 | 1.27 | 1.24 |
CFA for Intention
The one-factor CFA conducted on the four intention variables did not reveal good fit to the model due to one low-loading item (“Intention to pursue a research career, not as a PI,” loaded at −0.09), χ2(2) = 44.31, p < 0.001; CFI = 0.88, TLI = 0.64, SRMR = 0.08. The item was dropped, and the remaining three items had acceptable standardized loadings (see Figure 2) and were used in the measurement model.
Measurement Model
As shown in Figure 2, each of the five latent variables or factors of our model (enclosed in ellipses) is represented by a group of measured variables (enclosed in rectangles). The latent variables of SciComm SE and interest were each represented by “item parcels” (i.e., the mean of the items) for writing, presenting, and speaking. OEs were represented by six items, productivity by six items, and intention by three items.
The test of this five-factor measurement model in Mplus yielded a good-fitting model, χ2(178) = 446.16, p < 0.01; CFI = 0.94; TLI = 0.92; RMSEA = 0.05; SRMR = 0.05. In addition, most indicators had relatively high standardized factor loadings (e.g., >0.60), showing that these indicators had good psychometric characteristics. Figure 2 shows the unstandardized and standardized item loadings for each factor, and the factor correlations.
Structural Model
Because the measurement model had a good fit, showing that the measures were adequate, we next evaluated the hypothesized structural model. The test of the initial structural model, in which all hypothesized structural paths (Figure 1) were included, yielded an acceptable fit, χ2(179) = 446.89, p < 0.001; CFI = 0.94; TLI = 0.93; RMSEA = 0.05; SRMR = 0.05. All of the hypothesized paths were significant, except two: SE → intention and productivity → OEs. A series of models excluding the nonsignificant paths one at a time did not significantly differ from the initial model. Therefore, we chose the more parsimonious model (Figure 3) as the final structural model, χ2(181) = 447.23, p < 0.001; CFI = 0.94; TLI = 0.93; RMSEA = 0.05; SRMR = 0.05. The data supported that the amount of SciComm productivity significantly predicted SCSE (standardized path a = 0.25, p < 0.01) as well as intention to stay in a research career (standardized path b = 0.10, p < 0.05). SCSE significantly predicted SCOEs (standardized path c = 0.12, p < 0.05) and marginally predicted interest in performing SciComm tasks (path g = 0.09, p = 0.06). As expected, SCOEs significantly predicted interest in performing SciComm tasks (path e = 0.51, p < 0.001) and was also significantly predictive of intention to stay in a research career (path d = 0.26, p < 0.01). In addition, interest in performing SciComm tasks significantly predicted intention to stay in a research career (path f = 0.13, p < 0.05).

Figure 3. Final structural model of intention to remain in a research career. Standardized (unstandardized) coefficient: +, 0.06; *, <0.05; **, <0.01.
DISCUSSION
We conclude that biomedical and behavioral science trainees’ acquisition and thoughts about SciComm skills play a significant and independent role in their intentions to remain in research careers. Our model of the relationships among social cognitive variables related to SciComm and the career intentions of this group fit the data well, and our findings identified influences on career intent relevant to researchers nearing completion of their training that have not been reported in previous STEM career studies. These include the significant role of ancillary but essential professional skills and the importance of productivity and OEs relating to those skills. Also notable was the indirect (through SCOE) rather than direct relationship of SCSE to career intention. These findings have implications for both theory and practice.
Career decision making should not be taken for granted, even at the most advanced stages of training. Recent research suggests that many pre- and postdoctoral students enter their programs without clearly defined career goals and that some are likely to pursue postdoctoral training to clarify their career goals or to avoid leaving school, because they are unsure of what they should do next (National Academy of Science, 2000; Gibbs and Griffin, 2013). Given these personal factors, a clear understanding of drivers of BBR career intention through the postdoctoral stage will be useful in designing thoughtful educational policies, practices, and resources.
Significance and Implications for Theory
A major conclusion of this study that has implications for SCCT is that the need to master ancillary skills for a desired career may play an important role in an individual’s choice to remain in the career path. At the bridging point between completing professional training on the one hand and career initiation on the other, the realization that certain instrumental skills of less intrinsic interest will play a more salient role in one’s life than previously imagined can potentially affect perceived future satisfaction and create doubt in the career path. To our knowledge, no other SCCT studies have investigated the effect on cognitive variables of ancillary rather than discipline-specific skills.
The results of our study were in some ways consistent with previous STEM-focused SCCT models examining variables explicitly related to discipline-specific performance (Lent et al., 1994; Byars-Winston et al., 2010). For example, our SciComm-specific variable of SCSE related to SCOEs, and SCOEs related to both interest in performing SciComm tasks and intention to remain in an academic research career (similar to the outcome measure of goals in previous models). SciComm interest also related to intention. Nevertheless, our study identified influences on career intent novel to SCCT. First, because we assumed that many trainees working at the doctoral or postdoctoral level already have some SciComm products, past productivity was included as a construct in our model. As do previous studies (Bandura, 1986; Lent et al., 1993, 1994), our results show that greater SciComm past performance predicts greater SE, but examining the relationship between productivity and intention to remain in research careers within the SCCT does not, although it has been reported in other research not based on SCCT (Vrugt and Koenis, 2002). Our study population is research trainees at the doctoral and postdoctoral levels, and “productivity” in our model is not measured by scores or self-reports of quality of performance on externally imposed exams and structured assignments, but by the amount of largely self-motivated production of SciComm products.
In much of the SCCT literature on STEM careers, SE is posited as being a predictor of interest. Lent et al. (2003, 2011) found in their studies of undergraduates that interest was primarily influenced by SE rather than OEs. In other words, for undergraduates, interest in choosing a major depends more on how well students believe they can perform toward the major than on the perceived benefits a degree in that major might bring them. In our study, SCOEs, like productivity, played a significant role in trainees’ career intentions, but SCSE did not. This occurred in two ways. First, SCOEs were linked to their interest in performing SciComm tasks. Having more positive expectations about the benefits of producing SciComm was a strong influence on interest. Lent et al. (1994, p. 84) acknowledge that, in some situations, in which outcomes are “only loosely tied to the quality of performance,” OEs may be the stronger influence. Conversely, SE is likely to be the stronger influence on interest when desired outcomes are dependent on quality of performance. As discussed previously, postgraduate trainees typically are evaluated not on the quality of their writing and speaking skills per se but on their productivity as communicators; this may help explain the relationship between SCOEs and career intention. Byars-Winston et al. (2010), in their study of undergraduates pursuing both biological sciences and engineering, found that, for biosciences majors, there was a significant relationship of SE to goals and, as in the studies of Lent and colleagues, a significant indirect relationship of SE through interest. Engineering students, on the other hand, exhibited a pattern of OEs influencing goals both directly and indirectly through interest, with SE exerting an influence on OEs. This pattern for engineering undergraduates, also reported by Flores et al. (2014), is similar to ours for BBR postgraduates. Byars-Winston et al. (2010) suggest that the OEs → interest → goals path and OEs → goals path “may … reflect participants’ pragmatic orientation toward STEM pursuits” (p. 213). Our study population was composed of trainees nearing entry to professional positions and pursuing a necessary activity not directly related to research activity. Thus, a “pragmatic orientation” toward SciComm activity may shed light on the variation in the OEs → interest → intention and the OEs → intention path among engineering undergraduates and BBR postgraduates as opposed to BBR undergraduates. Taken together, these results highlight the importance of considering both stage of training and the role of ancillary skills in influencing career intentions.
Significance and Implications for Practice
Several implications for practice can be drawn from these findings. One is that evidence-based educational and training interventions designed to stimulate early, vigorous, and rewarded productivity may help to keep research trainees on their career tracks. Simultaneously, mentors and advisors may be prompted to educate themselves about the importance and dynamics of the process and to protect trainees’ time to develop SciComm skills. Examples of strategies for stimulating productivity include increasing frequency of engaging in SciComm tasks, such as multiple intermediate- and advanced-level writing assignments not centered specifically on reporting research results; ample, encouraged opportunities for formal presentation in a variety of venues; and structured assignments for participating in spontaneous conversations in lab discussions and seminars, such as assigning a trainee to lead the question-and-answer session after a presentation. These activities can be thoughtfully designed to gradually scaffold students’ skill development. Although several interventions that focus on raising SE have been published (Bakken et al., 2010; Byars-Winston et al., 2011; Komarraju et al., 2014), very little has been published within the SCCT framework about possible or tested methods for changing OEs (Fouad and Guillen, 2006; Metheny and McWhirter, 2013). We speculate that potential interventions to increase positive SCOEs might involve creating a more “short-term” along-the-way sense of intrinsic or extrinsic reward or acknowledgment upon completion of an activity. This might be as simple (and as complex) as teaching mentors how to give high-quality, constructive, and encouraging feedback on trainee speaking and writing. Or it could include organizing writing and speaking symposia for trainees, with certificates and prizes awarded and suitable for entry on trainees’ curricula vitae. Interventions to influence long-term SCOEs might include providing career presentations by researchers at various ranks focusing on success stories and “lessons learned,” attending conferences where junior researchers are giving platform presentations, attending informal scientific colloquia, and others. While many of these activities may already be offered to some degree during research training, providing them more intentionally and systematically could increase their reach and depth.
Finally, given the paramount importance of SciComm skills and productivity for obtaining academic research faculty positions and given the fact that trainees rarely arrive at graduate school with adequate SciComm skills, institutional culture and practices at the local and national levels should promote SciComm skill development during research training. Academic research institutions should encourage and support faculty educators in the development of SciComm curricula by promoting and rewarding their efforts, either at the individual level or programmatically, through the final stages of trainee professional development. Research mentors, as well, can further develop their skills through structured mentor-training programs (Pfund et al., 2014), which can include specialized units on SciComm skill development. Federal, state, and private funding sources can specify that SciComm skill development be included as an element of research training–program applications or individual fellowship applications, and academic policy makers can recommend or require that research-training programs or institutions receiving funds for training include SciComm skill development as part of their curricula.
Limitations
A limitation of this study is that it was conducted among trainees primarily at a limited number of research-intensive academic healthcare institutions in the same region and thus may not be representative of graduate schools and biomedical research institutions in general, including those outside the United States. Moreover, the modest numbers of certain demographic categories, particularly racial/ethnic groups underrepresented in the sciences, and linguistic groups such as bilinguals and speakers of nonstandard dialects were insufficient to conduct a complete and meaningful analysis of effects across multiple groups. Although we included items to determine dialect status of English speakers, the response patterns were not clear, suggesting that revision of the question and its response choices might bear improving. The cross-sectional nature of the current study cannot be used to evaluate causal relationships between SciComm SE, interest, productivity, and OEs on the one hand and career intention on the other.
These limitations are addressed together with future research directions in the next section.
Future Research Directions
Several directions for future research are suggested by this study. First, to gain greater insight into the dynamic relationship of SCSE to SciComm productivity over time, longitudinal studies of research trainees over the course of their training periods are needed. Singley et al.’s study (2010), which examined goal SE and goal progress, reported a reciprocal relationship between the two over time, creating a “feedback loop that can either promote or diminish performance” (p. 141). While the constructs in this study, SCSE and SciComm productivity, are distinct from goal SE, performance, and goal progress, it is possible that a similar dynamic is at work. This knowledge could inform the timing and staging of skill interventions across the training period. Future studies will address this aspect of the model by assessing participants at several time points.
Second, determining the role of person factors, such as gender, race/ethnicity, English-language status, dialect status (standard vs. nonstandard), level of education completed by parents, economic background, and other related factors such as trainee academic rank, may provide further insights on the findings presented here. Further investigation using multiple group analyses is essential to evaluate whether various populations exhibit similar cognitive frameworks to given measures.
Finally, examination of the role of contextual barriers and supports, in particular the role of mentoring, would be a useful extension of this study. Because of the particular influence of mentors in guiding trainees’ development of SciComm skills and their oversight of trainee publications and presentations, the dynamics of the mentoring relationship may play an important role in both the learning process and the outcome, Institutions as well may play key roles in creating environmental supports, such as how many and what types of SciComm classes and workshops (including faculty mentor development) are offered, whether the formation of SciComm peer support and coaching groups are encouraged and supported, and whether and how mentors are recognized or rewarded for excelling in teaching SciComm skills.
CONCLUSION
The pressures affecting doctoral and postdoctoral trainees’ selection of careers in the biomedical sciences are manifold, including widespread awareness of the difficulty of obtaining research funding, the personal and professional demands on research faculty and how these demands affect family life, the possible misalignment of trainees’ personal values with the realities of academic research, and the recent rise in the availability of private-sector research opportunities. The development of SciComm skills is one potentially critical yet often disregarded component of career preparation for trainees. With a theoretically based understanding of the influences on the acquisition of these skills, we can develop evidence-based, effective, and feasible approaches that will sustain trainees during their final steps in achieving their desired research careers. This study represents an initial step in that direction.
FOOTNOTES
1 A dialect is a variation of a single language typically associated with a geographical region, while a sociolect is a variation of a single language typically associated with social class or {AQ: Please define “SES.”}SES.
ACKNOWLEDGMENTS
The authors acknowledge the valuable contributions of Christine Pfund, PhD, for expertise in study design and Nikita Robinson, Alicia Bibbs, and Shailesh Advani for research support. The study described in this article was supported by NIGMS grant R01 GM085600-01A1. The content is solely the responsibility of the authors and does not necessarily reflect the views of the funding agency.