Evidence-Based Medicine as a Tool for Undergraduate Probability and Statistics Education
Abstract
Most students have difficulty reasoning about chance events, and misconceptions regarding probability can persist or even strengthen following traditional instruction. Many biostatistics classes sidestep this problem by prioritizing exploratory data analysis over probability. However, probability itself, in addition to statistics, is essential both to the biology curriculum and to informed decision making in daily life. One area in which probability is particularly important is medicine. Given the preponderance of pre health students, in addition to more general interest in medicine, we capitalized on students’ intrinsic motivation in this area to teach both probability and statistics. We use the randomized controlled trial as the centerpiece of the course, because it exemplifies the most salient features of the scientific method, and the application of critical thinking to medicine. The other two pillars of the course are biomedical applications of Bayes’ theorem and science and society content. Backward design from these three overarching aims was used to select appropriate probability and statistics content, with a focus on eliciting and countering previously documented misconceptions in their medical context. Pretest/posttest assessments using the Quantitative Reasoning Quotient and Attitudes Toward Statistics instruments are positive, bucking several negative trends previously reported in statistics education.
INTRODUCTION
Most students have difficulty reasoning about chance events (Shaughnessy, 1977, 1992). Students arrive in the classroom with theories or intuitions about probability that are at odds with conventional thinking (see examples in Table 1) and can even hold multiple mutually contradictory misconceptions about the same situation (Konold, 1995). Unfortunately, misconceptions generally persist and can even become stronger after instruction (Sundre, 2003; Delmas et al., 2007). This can occur not only for traditional instruction, but also for more innovative, hands-on approaches (Hodgson, 1996; Pfaff and Weinberg, 2009). The stakes are high, because overcoming these obstacles is essential for achieving numeracy to the level necessary for informed decision making in modern society (Gigerenzer, 2002; Gaissmaier and Gigerenzer, 2011; Reyna and Brainerd, 2007).
Misconception | Reference | Example of reasoning according to the misconception |
---|---|---|
Outcome orientation | Konold, 1989 | Considering the next 6 rolls of a dice with 5 black sides and one white, the most likely outcome is 6 rolls of black. |
Representativeness heuristic | Kahneman et al., 1982 | The sequence of births G B G B B G (G = girl; B = boy) is more likely than the sequence B G B B B B. |
Equiprobability bias | Lecoutre, 1992 | When rolling two dice, rolling a 5 and a 6 is equally as likely as rolling two 5s. |
Because both probability and statistics are difficult to teach, some have advocated bypassing formal probability in favor of early exploratory data analysis (Moore, 1997). A risk of this approach is that many students never get up to probability at all. This is a problem, because probability is not merely the foundation for statistics but is also directly relevant to medical and other decisions that we all must make (Gaissmaier and Gigerenzer, 2011). Probability is also important to the biology curriculum via genetics (Masel, 2012), and so minimizing probability in a statistics class shifts instructional burden to the biology faculty. Given the central importance of understanding probability in becoming an informed citizen in general, as well as to the life sciences in particular, we believe that the effort to counter probability misconceptions warrants more than the brief treatment it often gets as rapid “background” in a genetics course. For students whose curriculum stresses the exploratory data analysis approach, probability has become an upper-division mathematics elective, such that even the few biology students who take it are unlikely to do so before exposure in genetics.
COURSE DESCRIPTION AND DESIGN
Students are intrinsically motivated to learn about medicine, providing a great opening to teach probability and statistics in a medical context starting earlier in the curriculum. We therefore developed an undergraduate course in evidence-based medicine at the University of Arizona as a substitute for traditional 200-level biostatistics. It doubles as a substitute for either a traditional bioethics course or a science and society elective and meets both institutional requirements for a “writing-emphasis” course and the minimum quantity of reading and writing shown to be associated with gains on the Collegiate Learning Assessment (Arum and Roksa, 2011).
The primary tool of evidence-based medicine is the randomized controlled trial (RCT). We therefore made this the centerpiece of the class, making the class as much an exercise in the scientific method as it was a course in probability and statistics. Instead of teaching a broad diversity of scientific methods, we focused on gold-standard RCTs as an ideal paradigm for teaching the application of the scientific method not just to medicine but also to all messy data, that is, to everyday life. To reinforce the link to normal life, students read an engrossing history of RCTs (Burch, 2009), and all students wrote a proposal to perform an RCT. As a capstone, students carried out a handful of the proposed RCTs as class projects, for example, testing whether texting increases the likelihood that volunteers follow through on their commitment to give blood (Littin, 2012), whether the digital removal of a Nike logo changes the desirability of an article of clothing, or whether men can bench-press more when a woman sits on their hips (Huynh, 2014, 2015; Innes, 2015). Teaching the scientific method through RCTs is both a goal in and of itself, as well as a contextual tool that we hope may help make learning gains about probability stick.
Hypothesis testing was introduced early in the course, starting with two previously developed case studies, slightly modified by us for this course. The first, on Ignaz Semmelweis and hand-washing (Colyer, 1999), introduced hypothesis testing and the scientific method in a nonquantitative setting and prepared the way for contemporary discussions of hand-washing and checklists (Gawande, 2007). The second, based on Fisher’s original essay on the lady tasting tea (Maynard et al., 2009), extended this to bring in more formal hypothesis-testing concepts, including the null hypothesis, p-values, and the binomial distribution.
Motivated by the goal of understanding RCTs, we used backward-design principles to guide our choice of probability and statistics content. Discrete data in a 2 × 2 contingency table (treatment vs. control, live vs. die) is the obvious way to approach a clinical trial. Rather than the traditional Pearson’s version of the chi-square test (comparing Σ(O − E)2/E with χ2), we taught the likelihood-ratio version (comparing G = 2ln [L(data|H1)/L(data|H0)] with χ2) (Howell, 2014), both to reinforce learning of probability, and also because, should students continue in science, likelihoods appear in most statistical settings, whereas Pearson’s approach is used only for contingency tables. To avoid the trap of a canned technique, as Pearson’s test so easily becomes, our teaching of the derivation of the likelihood values required understanding the binomial distribution. Understanding of binomial coefficients is in any case needed to understand Fisher’s argument involving eight-choose-four equally likely options in the lady tasting tea. A less mathematically intensive version of the course than ours might omit the full binomial distribution and use Pearson’s test instead. In either case, p-values and type I and type II error rates are central topics, and working backward from what was needed, it was clear that a basic but firm grounding in probability is key.
To achieve this, we focused on eliciting and then combating known student misconceptions about probability (Table 1). We were particularly concerned about the total failure to grasp stochasticity known as the “outcome orientation” (Konold, 1989), an especially strong danger in the medical context (Humphrey and Masel, 2014). The goal of students with an outcome orientation “in dealing with uncertainty is to predict the outcome of a single next trial” (Konold, 1989, p. 61). When guessing the outcome of the roll of an irregular die, they are happy to call their estimate as right or wrong based on a single roll and are remarkably uninterested in gathering data on multiple rolls (Konold, 1989). If students treat every patient outcome as a unique event, rather than as members of a statistical group, they will not be able to grasp the power of RCTs (Humphrey and Masel, 2014).
Probability, in its modern philosophical interpretations, can mean very different things (Hájek, 2012). Frequentism refers to “forward probability”: the probability of seeing particular data given a state of the world. For example, p-values give the probability of seeing data so at odds with the null hypothesis, given that the null hypothesis is true. The most accessible, classical cases of forward probability focus on randomization devices such as dice and cards, for which each of a set of outcomes is equally likely. In contrast, Bayesianism focuses on “backward probability”; it is epistemic in nature, with “probability” describing our degree of confidence in an inference about the state of the world. Rather than promoting a single interpretation of probability or confusing students by presenting multiple interpretations simultaneously, we introduced notions of probability one at a time throughout the semester, in historical order. First, we worked with dice and playing cards to reinforce classical probability, trying to counter the outcome orientation by forcing students to consider dice rolls as a group. Then we did exercises with irregular dice (Bramald, 1994) to combat equiprobability bias during the transition from classical to frequentist probability. Some students had already encountered this distinction during K–12 as “theoretical” versus “experimental” or “empirical” probability. Here, we addressed outcome orientation again, stressing that however rare an event is, it can still happen, and that frequencies are the only way to put a number on this. We used combinatorics for both classical and frequentist probabilities, connected via the binomial distribution.
We introduced Bayesian probability much later in the semester, out of fear that content on subjective probability would accidentally reinforce the outcome orientation. Bayes’ theorem was taught in the context of medical-screening programs such as mammography (Gigerenzer, 2002) and Ioannidis’ argument that “most published research findings are false” (Ioannidis, 2005). The latter required a strong grounding in type I versus type II errors, built up during work on the likelihood ratio test. Conditional probability was introduced using real data on breast cancer incidence, with students exploring tables of data themselves before receiving formal instruction designed to distinguish between prob(A|B) and prob(B|A), in this case, prob(die of breast cancer|die young) ≠ prob(die young|die of breast cancer). Building on this foundation, Bayes’ theorem was then taught using dot diagrams and natural frequency trees (Sedlmeier and Gigerenzer, 2001; Figure 1) rather than via the equation.
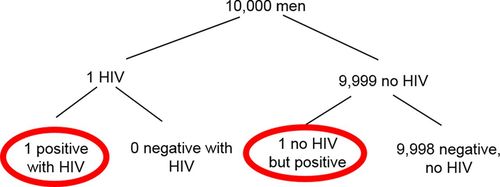
FIGURE 1. Use of a natural frequency tree to implement Bayes’ theorem. For this problem, the information given is “About 0.01% of men with no known risk factors have HIV. HIV+ men test positive 99.9% of the time. HIV− men test negative 99.99% of the time. A man with no known risk factors tests positive. What is the probability that he has HIV?” The two individuals meeting the condition of testing positive are circled; one of them has HIV, making the probability 0.5.
We left out many traditional biostatistics topics, including observational statistics. We taught mean, SD, variance, and SEM as background to the insight that the effect size that a study has adequate power to detect is proportional to one divided by the square root of the number of patients. But we did not teach correlation as a formal mathematical concept, although we did mention it informally when we stressed the importance of a randomized intervention as the only way to sort out association versus causation. For example, we contrasted early observational results that women undergoing hormone replacement therapy have better health (Grodstein et al., 1996) with later contradictory results from randomized trials (Women’s Health Initiative Steering Committee, 2004), drawing attention to how socioeconomic factors confound the former result but not the latter. Incoming students were all too keen to assert that it is impossible to reach conclusions without “controlling for” every conceivable confounding factor; omitting correlation almost until the end of the course allowed us to stress the power of randomization to remove the need to do this and hence distinguish causation from correlation alone.
The basics of randomization turned out to be surprisingly hard to teach and required substantial time. We used a previously developed active-learning exercise in which students assign playing cards randomly into two groups (Enders et al., 2006) and extended this exercise to have students physically implement a matched-pair design using playing cards. This was later reinforced by an exploration of alternative study designs, in particular comparing parallel groups with crossover design and with N of 1 designs.
The third pillar of the course, after RCTs and Bayes’ theorem, was science and society. Indeed, topics such as placebo effects naturally combine statistical material (regression to the mean) with the human aspects of doctors’ and patients’ desires “to please.” Students left the class with the useful take-home skill of being able to place studies, such as those cited above on hormone replacement therapy, on an evidence pyramid (Figure 2), knowing how to locate the highest quality evidence, for example, Cochrane Reviews, and knowing that not treating a patient can be a valid medical option for providers. Interestingly, the most disturbing content for many students came not from fiercely partisan issues such as healthcare system design or even from the troubling influence of money on medical decision making (Angell, 2005; Fugh-Berman and Ahari, 2007), but from challenges to the role of reductionism in biomedical science (Horrobin, 2003; Scannell et al., 2012). Table 2 outlines the topics covered by our course, and Table 3 gives the complete list of learning objectives.

FIGURE 2. Evidence pyramid. Near the end of the course, students are exposed to alternatives to RCTs and learn to identify the level to which a research article belongs and to choose the highest level of evidence available for a given question. The value of meta-analyses vs. large single trials is discussed, with publication bias raising a question mark over the order shown here.
Basic probability | Statistics/randomized controlled trials | Science and society | Bayes’ theorem/medical screening |
---|---|---|---|
|
|
|
|
|
Active-learning techniques were used as much as possible, including dice-rolling whenever possible. In addition to the previously published activities cited and otherwise described above, we made liberal use of think–pair–share interspersed within the 75-min classes. The outlines of these active-learning techniques can be followed via the staggered presentation of material in the slides in the Supplemental Material. Complete course materials are also available upon request. For example, think–pair–share was used for numerical questions such as applications of Bayes’ theorem via natural frequency trees, for guessing how things work in the real world for questions such as which categories of medical professionals are most and least likely to adhere to hand-washing and checklist regimes, and for open-ended experimental design questions such as what are the most important factors to control for/match. Role-playing exercises included one in which students decide on the ratio of type I: type II errors that they consider a reasonable trade-off, both for drug main effects and for serious side effects. Students then act out the roles of a desperate patient, a drug company rep, and an insurance company as each attempts to persuade the doctor as to the appropriate ratio.
ASSESSMENT METHODS
To assess our success in improving not only context-specific qualitative understanding, but also more generalized numeracy, we compared precourse versus postcourse results for each student using the Quantitative Reasoning Quotient (QRQ) instrument (Sundre, 2003), a refinement of the earlier Statistical Reasoning Assessment instrument (Garfield, 1998, 2003). While many later instruments focus on statistics alone, we chose the QRQ, because it also covers probability in a multiple-choice format that assesses many conceptions and misconceptions simultaneously. Note that, in previous studies, instruction does not have a good track record of improving QRQ scores. For example, sophomores who have completed their 10–12 credit-hour requirement in mathematics and sciences do not perform better on the QRQ than those who have not (Sundre, 2003). Indeed, it is not uncommon for some misconceptions to increase postcourse versus precourse (Delmas et al., 2007).
We simultaneously surveyed students’ Attitudes Towards Statistics (ATS; Wise, 1985), precourse and postcourse. Previous research with this and related instruments has found that students’ positive attitudes coming into a statistics course predicts their eventual performance in such a course and that attitudes improve only marginally following instruction (Elmore, 1993; Shultz and Koshino, 1998) or can even deteriorate (Schau and Emmioglu, 2012).
We compared overall correct score and individual QRQ subscores precourse versus postcourse using repeated-measures analysis of variance (ANOVA). For the ATS, we summed total positive attitude scores from the 29 ATS Likert items and compared these overall scores precourse versus postcourse using repeated-measures ANOVA, but given the coarse-grained ordinal nature of the individual Likert items, we analyzed these with the nonparametric Wilcoxon signed-rank test. Nonetheless, we display mean rather than median changes across students in ATS individual item scores precourse versus postcourse; otherwise the changes are sometimes invisible, even for statistically significant items. Raw anonymized data and scripts for QRQ and ATS analyses are available upon request.
RESULTS AND DISCUSSION
After several years of development, the latest iteration of our evidence-based medicine course was taught in Spring 2014 to 40 students (22 women, at least 15 members of underrepresented minority groups). The only prerequisite to the course was a “C” or higher in college algebra or placement directly into calculus. In practice, our enrollment consisted of one freshman, eight sophomores, 15 juniors, and 15 seniors, most of whom had some prior exposure via an introductory biostatistics course, genetics course, and/or social science research methods course. We were delighted that, from pretest to posttest, QRQ increased by 0.63 pretest SDs (p < 0.001; Table 4), and the ATS increased by 0.32 pretest SDs (p = 0.002; Table 5). Figure 3 shows those QRQ subscores and ATS items showing improvement with p < 0.05 and 0.5 < p < 0.1; none deteriorated at this level. QRQ subscores that improved included distinguishing correlation and causation, a task for which statistically significant deteriorations have previously been observed (Delmas et al., 2007).

FIGURE 3. We observed postcourse vs. precourse (a) overall improvements and improvements in some (b) QRQ subscores and (c) ATS item scores for our Spring 2014 course offering. The ATS is a 1–5 Likert scale, and QRQ scores are arbitrarily scaled to match. Negatively phrased ATS questions are shown with scores in reverse direction such that higher scores indicate more positive attitudes across all items; an attitude score of 3 is “neutral.” Because analysis is of paired measures, 95% confidence intervals (red) are shown once for the precourse vs. postcourse differences rather than separately for precourse and for postcourse scores.
Category | Category text | Pretest meana | Pretest SD | Posttest meana | Posttest SD | Mean difference | p |
---|---|---|---|---|---|---|---|
Overall | — | 58.95 | 9.9 | 65.15 | 12.28 | 6.2 (± 2.93) | <0.001 |
Competencies | Correctly interprets probabilities | 2.55 | 1.4 | 2.85 | 1.46 | 0.30 (± 0.44) | 0.183 |
Correctly interprets measures of central tendency | 3.74 | 0.79 | 3.89 | 0.75 | 0.14 (± 0.25) | 0.263 | |
Understands how to select an appropriate average | 2.4 | 0.9 | 2.67 | 0.99 | 0.27 (± 0.35) | 0.132 | |
Correctly computes probability | 2.78 | 1.1 | 2.48 | 1.13 | −0.30 (± 0.5) | 0.239 | |
Understands independence | 3.83 | 1.21 | 4.23 | 1.02 | 0.40 (± 0.43) | 0.073 | |
Understands sampling variability | 2.56 | 0.79 | 3.33 | 0.83 | 0.77 (± 0.28) | <0.001 | |
Distinguishes between correlation and causation | 3.57 | 1.22 | 4.03 | 1.31 | 0.47 (± 0.38) | 0.018 | |
Correctly interprets two-way tables | 3.1 | 1.81 | 3.2 | 1.8 | 0.10 (± 0.82) | 0.809 | |
Understands importance of large samples | 3.15 | 1.66 | 3.85 | 1.49 | 0.70 (± 0.68) | 0.046 | |
Understands sources of bias and error | 3.94 | 0.86 | 4.26 | 0.84 | 0.32 (± 0.31) | 0.044 | |
Recognizes features of good experimental design | 3.73 | 1.11 | 3.58 | 1.24 | −0.15 (± 0.41) | 0.467 | |
Misconceptions | Misconceptions involving averages | 2.54 | 0.7 | 2.2 | 0.66 | −0.34 (± 0.28) | 0.018 |
Outcome orientation misconception | 1.53 | 0.37 | 1.39 | 0.35 | −0.14 (± 0.14) | 0.048 | |
Good samples have to represent a high percentage of the population | 2.5 | 1.28 | 2.43 | 1.34 | −0.08 (± 0.47) | 0.752 | |
Law of small numbers | 2.05 | 1.2 | 1.6 | 0.93 | −0.45 (± 0.49) | 0.071 | |
Representativeness misconception | 1.93 | 0.89 | 1.6 | 0.77 | −0.33 (± 0.29) | 0.025 | |
Correlation implies causation | 2.43 | 1.22 | 1.97 | 1.31 | −0.47 (± 0.38) | 0.018 | |
Equiprobability bias | 3.25 | 1.39 | 3.43 | 1.34 | 0.18 (± 0.6) | 0.562 | |
Groups can only be compared if they are the same size | 2.1 | 1.8 | 2.5 | 1.96 | 0.40 (± 0.75) | 0.291 | |
Failure to distinguish the difference between a sample and a population | 2 | 1.01 | 1.75 | 0.98 | −0.25 (± 0.38) | 0.200 | |
Failure to consider and evaluate all of the data | 1.25 | 0.5 | 1.23 | 0.53 | −0.03 (± 0.18) | 0.785 | |
Inability to create and evaluate fractions or percents | 1.4 | 0.41 | 1.5 | 0.51 | 0.10 (± 0.19) | 0.291 | |
Only large effects can be considered meaningful | 2 | 1.76 | 1.7 | 1.54 | −0.30 (± 0.66) | 0.372 | |
Failure to recognize potential sources of bias and error | 1.97 | 0.81 | 1.63 | 0.72 | −0.33 (± 0.27) | 0.018 | |
Assumes more decimal places indicate greater accuracy | 1.1 | 0.64 | 1.2 | 0.88 | 0.10 (± 0.35) | 0.570 | |
Inability to interpret probabilities | 1.3 | 0.27 | 1.26 | 0.25 | −0.04 (± 0.09) | 0.418 |
Category | Pretest meana | Pretest SD | Posttest meana | Posttest SD | Mean difference | p |
---|---|---|---|---|---|---|
Overall attitude | 63.71 | 10.35 | 69.50 | 12.57 | 5.78 (± 3.64) | 0.002 |
I feel that statistics will be useful to me in my profession | 3.85 | 0.93 | 4.18 | 0.97 | 0.33 (± 0.34) | 0.037 |
The thought of being enrolled in a statistics course makes me nervous | 3 | 1 | 3.08 | 1.11 | 0.08 (± 0.33) | 0.656 |
A good researcher must have training in statistics | 3.95 | 0.86 | 4.56 | 0.55 | 0.62 (± 0.3) | 0.001 |
Statistics seems very mysterious to me | 3.31 | 0.92 | 3.54 | 0.85 | 0.23 (± 0.33) | 0.214 |
Most people would benefit from taking a statistics course | 3.64 | 0.71 | 4 | 0.69 | 0.36 (± 0.23) | 0.005 |
I have difficulty seeing how statistics relates to my field of study | 3.79 | 0.92 | 4.05 | 1 | 0.26 (± 0.32) | 0.106 |
I see being enrolled in a statistics course as a very unpleasant experience | 3.05 | 0.96 | 3.55 | 0.92 | 0.50 (± 0.38) | 0.014 |
I would like to continue my statistical training in an advanced course | 2.56 | 0.72 | 2.92 | 1.09 | 0.36 (± 0.32) | 0.031 |
Statistics will be useful to me in comparing the relative merits of different objects, methods, programs, etc. | 3.82 | 0.6 | 3.95 | 0.79 | 0.13 (± 0.3) | 0.414 |
Statistics is not really very useful, because it tells us what we already know anyway | 4.03 | 0.67 | 4.31 | 0.69 | 0.28 (± 0.24) | 0.029 |
Statistical training is relevant to my performance in my field of study | 3.66 | 0.88 | 4.05 | 0.8 | 0.39 (± 0.23) | 0.003 |
I wish that I could have avoided taking my statistics course | 3.13 | 1.08 | 3.59 | 1.14 | 0.46 (± 0.38) | 0.023 |
Statistics is a worthwhile part of my professional training | 3.66 | 0.71 | 3.92 | 0.78 | 0.26 (± 0.24) | 0.043 |
Statistics is too math oriented to be of much use to me in the future | 3.97 | 0.74 | 4.1 | 0.94 | 0.13 (± 0.28) | 0.386 |
I get upset at the thought of enrolling in another statistics course | 3.08 | 1.02 | 3.32 | 1.16 | 0.24 (± 0.4) | 0.238 |
Statistical analysis is best left to the “experts” and should not be part of a lay professional's job | 3.76 | 0.85 | 3.82 | 0.69 | 0.05 (± 0.29) | 0.721 |
Statistics is an inseparable aspect of scientific research | 4.03 | 0.79 | 4.08 | 0.91 | 0.05 (± 0.26) | 0.721 |
I feel intimidated when I have to deal with mathematical formulas | 3.13 | 1.04 | 3.42 | 1.15 | 0.29 (± 0.32) | 0.100 |
I am excited at the prospect of actually using statistics in my job | 2.76 | 0.86 | 3.14 | 1.03 | 0.38 (± 0.39) | 0.072 |
Studying statistics is a waste of time | 4.16 | 0.68 | 4.24 | 0.88 | 0.08 (± 0.27) | 0.607 |
My statistical training will help me better understand the research being done in my field of study | 3.92 | 0.78 | 4.05 | 0.84 | 0.13 (± 0.2) | 0.212 |
One becomes a more effective “consumer” of research findings if one has some training in statistics | 3.84 | 0.59 | 4.08 | 0.82 | 0.24 (± 0.32) | 0.166 |
Training in statistics makes for a more well-rounded professional experience | 3.92 | 0.43 | 4.05 | 0.77 | 0.13 (± 0.27) | 0.374 |
Statistical thinking can play a useful role in everyday life | 3.68 | 0.62 | 3.92 | 0.85 | 0.24 (± 0.27) | 0.100 |
Dealing with numbers makes me uneasy | 3.58 | 1 | 3.74 | 1.06 | 0.16 (± 0.25) | 0.228 |
I feel that statistics should be required early in one's professional training | 3.26 | 0.6 | 3.63 | 0.79 | 0.37 (± 0.27) | 0.014 |
Statistics is too complicated for me to use effectively | 3.63 | 0.82 | 3.74 | 1 | 0.11 (± 0.32) | 0.597 |
Statistical training is not really useful for most professionals | 3.68 | 0.77 | 3.89 | 0.8 | 0.21 (± 0.26) | 0.120 |
Statistical thinking will one day be as necessary for efficient citizenship as the ability to read and write | 2.68 | 0.84 | 2.53 | 0.89 | –0.16 (± 0.3) | 0.313 |
Previous research suggests that QRQ-like scores correlate negatively with effort-based course grades (explaining previously noted gender biases) and only weakly positively with other graded items (Tempelaar et al., 2006). Results for our course were different: posttest QRQ correlations (Pearson’s r) with both course grades as a whole and with our final closed-book exam (included in the Supplemental Materials) were high at 0.5, and even correlations on more effort-based items such as homework problem sets (as found in the Supplemental Materials) were 0.38. Pretest QRQ correlations with final course grade, final exam, and effort-based content were similarly high. This demonstrates that our course assessments are well aligned with the widely endorsed learning objectives of the QRQ (Sundre, 2003). This is despite the fact that course assessments, for example, the two final exams included as Supplemental Materials, differ substantially in content from the QRQ, testing course-specific information in addition to general quantitative reasoning skills. ATS pre- and posttests also predicted course performance, in line with previous studies on attitudes using both the ATS (Waters et al., 1988; Vanhoof et al., 2006) and similar instruments (Emmioglu and Capa-Aydin, 2012) in other statistics classes. Changes in attitudes and quantitative reasoning reflected in the ATS and QRQ were not significantly correlated with pretest scores (Pearson’s r = –0.18 for ATS; –0.19 for QRQ; both p > 0.35). This indicates despite the diversity in ability and attitudes present in a class with as few prerequisites as ours, initially strong or positive students were not systematically more or less likely to benefit from instruction than weaker or more negative students.
Despite the small class size, the assessment evidence suggests that the course was a spectacular success, especially relative to the somewhat dismal history of probability and statistics education. Note that it aligns well with many calls for change (Table 6). We believe it to be far superior to the standard biostatistics curriculum in preparing students for real-world decision making, which benefits from a critical evaluation of (and perhaps even generation of) evidence. Indeed, we have heard a number of promising anecdotes about former students applying knowledge from their class, both as patients and as medical workers, in ways that affected medical care choices.
Challenge addressed | Document | Reference | Specific competencies covered by our course |
---|---|---|---|
Four out of six core competencies | Vision and Change in Undergraduate Biology Education: A Call to Action | American Association for the Advancement of Science, 2011 | Ability to apply the process of science Ability to use quantitative reasoning Ability to tap into the interdisciplinary nature of science Ability to understand the relationship between science and society |
Two of the eight competencies | Scientific Foundations for Future Physicians | Association of American Medical Colleges–Howard Hughes Medical Institute, 2009 | Apply quantitative reasoning and appropriate mathematics to describe or explain phenomena in the natural world Demonstrate understanding of the process of scientific inquiry and explain how scientific knowledge is discovered and validated |
New MCAT requirements | — | Schwartzstein et al., 2013 | Psychological, Social, and Biological Foundations of Behavior Critical Analysis and Reasoning Skills |
Integrate ethics with scientific content | — | Cech, 2014 | — |
We have begun developing a new hybrid (50% online 50% face-to-face) version of the course, taught for the first time in Spring 2015 to 29 students. This move was motivated primarily by pedagogical concerns; our quantitative material is highly cumulative in nature, inevitably leaving some students behind in face-to-face classes. When material is give online, students have more ability to set their own pace, and interspersing content with frequent autograded quizzes can provide additional help through greater formative assessment and learning through testing (Brown et al., 2014). We have developed two new online apps as part of the online materials, one on confirmation bias (http://bias.oia.arizona.edu/index.html) and one on the mathematics of power (http://power.oia.arizona.edu/index.html). The power app was designed to be used to illustrate how the effect size that a study has power to detect depends on the SD among patients divided by the square root of the number of patients. Customizable options (at http://bias.oia.arizona.edu/options.html and http://power.oia.arizona.edu/options.html for confirmation bias and power, respectively) allow the staged introduction of elements of the apps.
We hope these changes will lead to learning gains in a higher proportion of the class. QRQ and ATS scores for our first offering of the hybrid version (Spring 2015) are shown in Supplemental Tables 1 and 2, and pooled data across both semesters is shown in Supplemental Tables 3 and 4. QRQ and ATS scores each showed improvements of 0.28 pretest SDs (p = 0.046 for QRQ; p = 0.077 for ATS; Supplemental Tables 1 and 2). These effect sizes are on the whole (nonstatistically significantly) smaller, around half the size of the fully face-to-face class discussed at length above. When data from both years were combined, effect sizes for both QRQ and ATS overall scores were intermediate and remained statistically significant (0.46 and 0.42 pretest SDs for QRQ and ATS, respectively; both p < 0.001; Supplemental Tables 3 and 4).
Note that while there is a strong correlation between subscore effect sizes across the two semesters for QRQ (Pearson’s r = 0.55, p < 0.001), the best- and worst-performing subscores in Table 4 nevertheless regress to the mean in Supplemental Tables 1 and 2, a fact that acts as a caution against the overinterpretation of outlier subscores. Nevertheless, the added power afforded by combining results from both years increased the number of individual subscore items showing a change with p < 0.05 (Supplemental Table 3). A consistent underperformer across both semesters was equiprobability bias, which we intend to target more actively next time. Similarly, while overall ATS improvements were seen in each year, when both were combined, the effect sizes of individual ATS items were entirely uncorrelated between years (Pearson’s r = 10−5). This reinforces the caution that individual attitude items are likely uninformative, even though the overall effect sizes may indicate a more general and positive shift in attitudes.
While not definitively worse, clearly the hybrid version is not outperforming the face-to-face version at this time. We note that there were the inevitable teething problems associated with the transition to online instruction, and we hope to see learning gains improve over the coming years as the online materials are refined in the light of the abundant data that online instruction generates. If and when the online hybrid version outperforms the original, a second benefit of the new format is to make it easy to disseminate; its writing-intensive nature can be preserved if a high faculty–student ratio is available, or a simplified version should work for larger classes, helping meet high demand. In the meantime, extensive and up-to-date course materials beyond the Supplemental Materials are available on request.
ACKNOWLEDGMENTS
This work was funded in part by a grant to the University of Arizona from the Howard Hughes Medical Institute (52006942). The opinions expressed herein do not necessarily represent those of our funders, who played no role in the preparation of this article. Online course development was funded in part by an Online/Hybrid Course Development Grant as part of the Online Education Project at the University of Arizona. We thank Gretchen Gibbs for guidance in online course development and Gary Carstensen for implementing the two online apps.