What Makes a Good Match? Predictors of Quality Mentorship Among Doctoral Students
Abstract
Quality mentoring promotes graduate student success. Despite an abundance of practical advice, empirical evidence regarding how to match mentees and mentors to form quality mentoring relationships is lacking. Here, we examine the influence of variables theorized to predict mentorship support and quality in a national sample of 565 science doctoral students from 70 universities in 38 states. Our structural equation modeling results indicate that mentor rank, mentee capital, and the relationship matching mechanism (direct admissions, rotations) were not associated with higher-quality relationships. We found no support for the widely held belief that students whose mentors shared their gender, race, or ethnicity experienced greater mentorship quality. Rather, mentees who shared attitudes, beliefs, and values with their mentor, or whose mentors displayed greater cultural awareness experienced more supportive, higher quality mentoring. Furthermore, these patterns were largely consistent across both mentee and mentor demographic groups. These results highlight the potential benefits of pairing mentees and mentors who share personal and intrinsic qualities rather than demographic or surface-level attributes. Our findings also indicate that graduate students from marginalized backgrounds can be effectively mentored by faculty who are demographically dissimilar if their mentors engage in culturally aware mentorship.
INTRODUCTION
The United States (U.S.) National Academies of Sciences, Engineering, and Medicine have called for widespread change to graduate education in Science, Technology, Engineering, and Mathematics (STEM) (National Academies of Sciences, 2018). For decades concerns have been raised regarding the alignment between STEM graduate students’ career interests and the diversity of desirable jobs present in the labor market (National Academies of Sciences, 2018). These challenges have been exacerbated by a graduate population that is not reflective of the demographic diversity in the U.S. (National Center for Science and Engineering Statistics [NCSES], 2023), resulting in a limited talent pool and lost opportunity for innovation. Moreover, STEM graduate programs are plagued with high rates of attrition and deteriorating physical and mental well-being among students (Sowell et al., 2015; Levecque et al., 2017; Evans et al., 2018; Nagy et al., 2019; Zhang et al., 2022). These persistent problems have lead policy makers and university leaders to propose changes aimed at improving graduate student training in general and the quality of mentorship that graduate students experience in particular (National Academies of Sciences, 2018).
Mentorship is commonly described as a working alliance between a more experienced individual (the mentor) and a less experienced individual (mentee; National Academies of Sciences, 2019). High-quality mentorship during research training is thought to be essential for cultivating, developing, and training the next generation of scientists (National Academies of Sciences, 2019). Quality mentorship also has the potential to be an effective mechanism for recruiting talent and retaining professionals who will contribute to the national and global economy in the STEM research enterprise (National Academies of Sciences, 2019). Numerous empirical studies and robust meta-analyses have shown that effective mentorship can lead to desirable attitudinal, behavioral, career, and health related outcomes (Allen et al., 2004; Eby et al., 2008; Kammeyer-Mueller and Judge, 2008; O’Brien et al., 2010; Eby et al., 2013; National Academies of Sciences, 2019). Effective mentorship is thought to be particularly important for women and racially minoritized1 individuals as they navigate the inequities that persist in STEM fields (Ong et al., 2011; Estrada et al., 2018; McGee, 2020). Despite the positive relationships between quality mentorship and desirable mentee outcomes, far less is known about the factors that predict the formation of quality mentoring relationships. The current study aims to examine the influence of key antecedents theorized to predict mentee perceptions of mentoring support and relationship quality.
Overview of Mentoring Processes
A graduate student’s relationship with their research advisor strongly influences the quality and success of their graduate training (Zhao et al., 2007; Sverdlik et al., 2018). This is consistent with the apprenticeship model characteristic of STEM graduation education, which relies on faculty advisors to mentor graduate students in their development as researchers. Mentoring theory suggests that mentors provide at least two core forms of support to mentees (Kram, 1983). The first is career support, which refers to mentor behaviors aimed at facilitating the mentee’s professional development and career advancement, and includes the provision of career guidance, sponsorship, and protection. The second, psychosocial support, refers to mentor behaviors aimed at developing the mentee’s personal and social development and well-being through the provision of counseling, encouragement, acceptance, role modeling, and friendliness. Mentoring theory suggests that the mentoring relationship quality, or the satisfaction, liking, and trust between the mentee and mentor, acts recursively to reinforce mentoring support and promote desirable mentee outcomes. Studies across mentoring contexts (e.g., workplace, youth) indicate that antecedent variables (e.g., personality) and correlates (e.g., relationship length) may influence the quality of mentoring relationships and provision of career and psychosocial support, which in turn are associated with beneficial outcomes (Eby et al., 2013). Despite an abundance of conjecture, empirical studies testing the influence of such antecedent variables within the context of academic mentoring is limited. Furthermore, while mentorship is considered to be a national priority in STEM education (Crisp et al., 2017), research overall on the antecedents of quality mentorship within STEM fields is still nascent.
Predictors of Quality Mentorship
The mentoring of STEM graduate students presents a distinct and compelling opportunity to examine the factors associated with quality mentoring relationships for several reasons. First, different STEM disciplines and subdisciplines afford or constrain the processes through which graduate student mentees are matched to faculty mentors. For instance, some graduate programs require a mentor to be identified before program admissions (i.e., direct admission). Mentoring matches made between mentors and mentees who are not familiar with one another are known in the mentoring literature as “formal” relationships (Ragins and Cotton, 1999). Other programs facilitate a matching process, commonly known as rotations, during which graduate students temporarily join research groups before the mentor and mentee agree on a match. Matches based on some level of mentor–mentee familiarity is described in mentoring literature as “informal” (Ragins and Cotton, 1999). The direct admission process may effectively constrain the information and capital that both mentees and mentors use to make a match (e.g., information available on a student’s curriculum vitae or on a faculty member’s website). In contrast, rotations may afford an opportunity for mentees and mentees to get to know each other, but to also make judgements about fit and compatibility through fairly limited interactions.
Second, graduate student mentees may consider a variety of characteristics of potential faculty mentors in the process of making a match. For instance, mentees might weigh a potential mentors’ availability to provide support, the extent of their experience mentoring graduate students, and their level of prestige, experience, or accomplishments in the field (e.g., mentor capital). Mentees may also weigh whether they perceive that they have things in common with potential mentors, such as shared interests, values, beliefs, or personal characteristics (e.g., gender, race, ethnicity). Mentees who do not share demographic similarities with their mentor may also consider the extent to which a potential mentor respects and recognizes differences and is able to create an inclusive environment that is responsive to these differences. Prior research has examined the effects of these antecedents (formal vs. informal matching, mentor experience, mentor–mentee similarity) on mentoring support and relationship quality, primarily in youth and workplace mentoring. Little, if any, research has examined whether the factors currently used to match STEM graduate mentees with faculty mentors affect the likelihood of developing a quality mentoring relationship. Thus, in this study, we sought to examine the effects of these antecedents on the mentoring support and relationship quality experienced by doctoral students.
Mentee–Mentor Similarity
A number of individual, relational, and organizational level variables are theorized to influence mentorship quality. Among them, similarity between mentees and mentors, including deep-level, surface-level, and experiential similarity, is thought to be impactful (Harrison et al., 1998). The similarity-attraction paradigm posits that individuals are attracted to and develop higher-quality relationships with individuals who are similar to themselves (Byrne, 1961, 1971). Multidisciplinary meta-analyses lend empirical support for the notion that similarity is associated with high-quality mentoring relationships (Eby et al., 2013; Ghosh, 2014). Yet, mentees can resemble their mentors in many different ways, which necessitates a greater understanding of the particular dimensions of similarity that yield effective mentoring relationships.
Deep-Level Similarity.
Among all dimensions of similarity, deep-level similarity–meaning mentees’ perceptions that they share attitudes, perspectives, interests, values, and beliefs with their mentors–is most consistently and positively associated with mentoring quality (Ensher and Murphy, 1997; Harrison et al., 1998; Ensher et al., 2002; Turban et al., 2002; Hernandez et al., 2017, 2023; Pedersen et al., 2022). Evidence from one of the largest meta-analyses integrating 173 studies of mentoring from youth, workplace, and academic settings found that mentee perceptions of mentorship quality were most strongly associated with deep-level similarity (ρ ranging from 0.38 to 0.59; Eby et al., 2013). In addition, moderation analyses indicate that deep-level similarity has a considerably stronger effect in academic settings than in the workplace in terms of both career support (ρ = 0.64 vs. ρ = 0.38) and psychosocial support (ρ = 0.75 vs. ρ = 0.48; Eby et al., 2013). This suggests that deep-level similarity, while positive across all contexts, may have a disproportionate influence on mentorship quality in academic settings. However, less than a quarter of the studies included in this meta-analysis were based on studies from academic settings and few were specific to graduate student-faculty mentoring relationships (Eby et al., 2013).
There is also growing evidence at the undergraduate level indicating that deep-level similarity is moderately associated with higher levels of mentorship support and greater relationship quality (Hernandez et al., 2017; Pedersen et al., 2022; Saw et al., 2023). For instance, the effects of deep-level similarity have been shown to surpass the influence of demographic similarities in fostering quality mentorship and persistence in the field for African American/Black and Hispanic undergraduates in STEM fields (Hernandez et al., 2017; Pedersen et al., 2022). Results from a quasi-experimental, longitudinal study indicated that deep-level similarity promoted the provision of mentorship support and ultimately the development of a high-quality mentoring relationship (Hernandez et al., 2023). In addition, Hernandez and colleagues (2023) determined that structured relationship-building activities afforded opportunities for mentees to identify similarities with their mentor, which in turn lead to greater perceptions of deep-level similarity and the development of more fulfilling and beneficial mentoring relationships. However, research is needed to understand the effect of deep-level similarity on the quality of STEM graduate students’ mentoring relationships.
Surface-Level Similarity.
Research addressing the influence of surface-level similarities (e.g., gender, race, ethnicity, age) on mentorship quality has produced largely inconsistent results. Women and individuals from racially minoritized groups report a somewhat greater preference for a mentor who shares their surface-level attributes compared with men and racial majority group members (Blake-Beard et al., 2011; Bailey et al., 2016; May et al., 2024). Thus, a common assumption is that matching mentees and mentors on the basis of surface-level similarities will yield the greatest benefits. Yet, evidence in support of this claim is limited (Turban et al., 2002; Allen and Eby, 2003; Lankau et al., 2005). Meta-analytic results suggest that surface-level similarity in the form of shared mentee-mentor gender or race/ethnicity is, in general, not meaningfully related to mentorship quality (ρ ranging from 0.00 to 0.09; Eby et al., 2013). However, these results do not account for changing demographics among mentors (i.e., the majority of mentors have been disproportionately White and male), which raises questions about their relevance as STEM faculty and student populations diversify. In addition, at least some research indicates that diversified mentoring relationships (i.e., dyads that differ on the basis of gender, race/ethnicity, sexual orientation, ability status, religion, socioeconomic status, or nationality) may yield distinct benefits (Ragins, 1997). For example, cross-race relationships are a unique form of social contact that can have a transformative impact on individuals. Furthermore, cross-race relationships can lead to the development of antiracist attitudes and reduced prejudice towards individuals of a dissimilar identity (Davies et al., 2011; Ragins and Ehrhardt, 2021). Although diversity in organizations and STEM fields has been a prominent topic for decades (Roberson, 2019), research on diversified mentoring relationships remains quite limited and has focused primarily on exploring the influence of gender and race/ethnicity on mentoring relationships.
Gender Similarity.
Despite persistent debate over how gender2 influences mentorship, current consensus is that neither mentor nor mentee gender influences the provision of mentoring or the quality of mentoring relationships (Ragins, 1999; Ragins and Cotton, 1999; O’Brien et al., 2010). Some evidence indicates that same-gender relationships afford greater mentoring support than cross-gender relationships (Scandura and Williams, 2001; Morales et al., 2021), perhaps because they facilitate greater interpersonal comfort and closeness, as well as more shared experience than cross-gender relationships (Ragins, 1997; Allen et al., 2005). Hispanic and African American/Black undergraduate mentees in STEM with same-gender mentors reported slightly higher mentorship quality than those in cross-gender relationships, but the associations were small (Hernandez et al., 2017; Pedersen et al., 2022). Latina (women) undergraduate students who had men mentors reported higher intentions to pursue a STEM PhD, while gender match or mismatch did not influence Latino (men) students’ graduate school intentions (Morales et al., 2021). Furthermore, mentee gender has not moderated effects of mentor gender on mentorship support in a sample of predominantly Black and Latinx undergraduate students (Kuchynka et al., 2023). Although, collectively, studies of gender similarity have found small or no associations with mentorship quality (O’Brien et al., 2010; Eby et al., 2013), it is plausible that cross-gender relationships may limit the benefits of mentorship if group members do not recognize, respond to, or value the gender difference. For instance, members of cross-gender relationships may intentionally limit social interactions in an attempt to maintain professional boundaries and minimize the potential for misconstrual that the interaction is romantic in nature (Tuma et al., 2021).
Racial and Ethnic Similarity.
Research on racial and ethnic similarity in mentoring dyads has produced ambiguous and even conflicting results. Meta-analytic research indicates that mentor-mentee matching on the basis of demographics is not a requirement for quality mentorship (Eby et al., 2013). However, studies that were included in this meta-analysis were limited by the lack of diversity among mentors (Eby et al., 2013; NASEM, 2018). Results of more recent studies have been mixed. For example, Hispanic undergraduates in STEM with a Hispanic faculty mentor reported greater scholarly coauthoring opportunities, but not greater mentorship support or relationship quality, when compared with Hispanic undergraduates with a mentor of differing ethnicity (Pedersen et al., 2022). In contrast, Morales and colleagues reported that Latinx undergraduates with non-Latinx mentors had greater intentions to enroll in a STEM PhD program compared with those with a Latinx mentor (Morales et al., 2021). For African American/Black and White undergraduate students in STEM, having a mentor of similar race was not related to greater mentorship quality (Hernandez et al., 2017, 2023).
At the graduate level, a study of 220 doctoral student-faculty dyads at a single university concluded that same-race/ethnicity relationships were not meaningfully associated with levels of mentoring support (Turban et al., 2002). However, the majority of the mentees in this study (>65%) were White, raising the question of whether racial/ethnic similarity may be more influential for individuals from racially minoritized backgrounds. Blake-Beard and colleagues conducted one of the largest studies to date of >1000 racially diverse undergraduate and graduate students in STEM, finding that that individuals in same-race relationships perceived receiving greater psychosocial and career support than those in cross-race relationships (Blake-Beard et al., 2011). In addition, mentees preferred to have a mentor who matched their racial background and felt that mentors, regardless of their race/ethnicity, should recognize how a mentee’s background could affect their professional development (Blake-Beard et al., 2011). However, shared mentee-mentor race/ethnicity did not result in improved mentee outcomes (e.g., increased GPA or self-efficacy) even when the sample was constrained to students who perceived that having a same race mentor was important (Blake-Beard et al., 2011). It is worth noting that this study measured racial/ethnic matching by grouping individuals who identified as Black, Native American, Hispanic, Hawaiian, or Pacific Islander in to a “targeted minority” group (Blake-Beard et al., 2011). Consequently, the study evaluated the effects of having a mentor from any racially minoritized group, which leaves open the question of whether having a more precise match in terms of race or ethnicity might yield benefits that were missed.
Culturally Aware Mentoring.
Given the limited diversity among STEM faculty (Matias et al., 2022) and the growing proportion of STEM graduate students from racially minoritized backgrounds (National Center for Science and Engineering Statistics [NCSES], 2023), many mentoring relationships in STEM fields occur between racially well-represented faculty members and racially minoritized mentees. Furthermore, the small proportion of faculty from racially minoritized backgrounds engage in a greater number mentoring relationships and offer a deeper, stronger level of support to racially minoritized students’ (Baez, 2000; Griffin and Reddick, 2011; Griffin, 2013). This creates a form of “cultural taxation” in which faculty of color expend more time and effort on mentoring work that is not incentivized, valued, or rewarded (Padilla, 1994), further magnifying identity-related inequities observed in faculty workloads (O’Meara et al., 2017). Aiming to match mentees and mentors on the basis of demographic similarity has the potential to be troublesome given the limited number of minoritized STEM faculty and the increasing number of graduate students from racially minoritized backgrounds (Griffin, 2012; Fries-Britt and Snider, 2015). Thus, even if the evidence were clear that demographically matched mentoring relationships resulted in more positive mentee outcomes, it is not just or feasible to ask minoritized faculty to serve as mentors to all minoritized mentees.
Thus, to meet the mentoring needs of graduate students of color and avoid overburdening faculty of color, recent mentoring professional development work has focused on equipping mentors of all backgrounds with knowledge, skills, and training to effectively support mentees from different backgrounds – what is referred to as culturally aware mentorship (Byars-Winston et al., 2018). Culturally aware mentorship, where mentors demonstrate interest in and value of students’ cultural knowledge, frames of reference, and identities (e.g., race/ethnicity, sexual orientation, religion, cultural identity), may be important for fostering quality mentoring relationships in dyads who differ in their demographic characteristics (Syed et al., 2011; Merriweather, 2012; Reddick and Pritchett, 2015). Culturally aware mentorship emphasizes mentors’ skills in recognizing and responding to issues relating to race, power, and privilege in their mentoring relationships. Thus, culturally aware mentoring may be a critical factor in ensuring that minoritized mentees can thrive in their academic and professional pursuits. Some evidence suggests that mentorship that addresses cultural diversity is positively associated with mentee science identity (Haeger and Fresquez, 2016; Camacho et al., 2021; Estrada et al., 2022), satisfaction with research (Haeger and Fresquez, 2016), perceived mentoring effectiveness (Black et al., 2022; Pfund et al., 2022), scientific integration (Estrada et al., 2022), and intent to pursue a scientific career (Estrada et al., 2022). While culturally aware mentorship may be particularly beneficial for individuals of racially minoritized backgrounds, it may also be advantageous for individuals of other marginalized backgrounds. For instance, deaf mentees with research mentors who were responsive to their deaf or hard of hearing status but were not deaf themselves reported similar mentoring outcomes to mentees with a deaf mentor (Braun et al., 2017). To date, however, very little research has investigated the influence of culturally aware mentoring on mentoring support and relationship quality.
Mentor Rank.
STEM faculty members who differ in their levels of experience as reflected in their rank may also differ in their knowledge, skills, prestige, and connections in ways that influence their relationships with mentees and subsequent mentoring outcomes. The term “human capital” refers to a person’s cumulative knowledge, competencies, educational, and professional experiences that can enhance their career attainment and success (Schultz, 1961). Mentor human capital is important to consider because mentors with greater human capital may positively influence their mentees. For instance, mentors who are viewed as rising stars and successful in their careers positively influence the subsequent likelihood their mentees will also be successful and high performing (Judge et al., 2004; Tonidandel et al., 2007; Ma et al., 2020). Alternatively, mentors who are more junior in their positions may have fewer competing demands on their time and greater motivation to provide mentorship, and thus may provide greater mentoring support to their mentees (Kammeyer-Mueller and Judge, 2008; Eby et al., 2013). Some studies have documented that mid-career mentors report greater motivation to mentor than more senior and late-career mentors (Allen et al., 1997a; Morales et al., 2017), suggesting that senior career mentors may have reduced capacity and motivation to mentor.
Mentee Capital.
Mentee human capital may also be associated with the amount of mentoring support received. Mentors may select and provide greater mentoring support to mentees who possess higher potential (e.g., greater levels of experience, higher achievements) because they anticipate that high-performing mentees will be more productive and require less effort to support, which will ultimately result in greater outcomes for the mentor (Allen et al., 1997b). Indeed, research suggests that mentors look for indicators of a mentee’s potential for success and evaluate a mentee’s credentials before choosing to engage in a mentoring relationship (Olian et al., 1993; Allen et al., 2000; Joy et al., 2015). For example, graduate students perceived to have greater potential have been shown to receive greater mentoring support from their advisors than students perceived to have less potential (Green and Bauer, 1995). At the undergraduate level, students from lower socioeconomic backgrounds report receiving less mentorship support than students from more affluent socioeconomic backgrounds (Saw et al., 2023). Mentors may be selecting mentees who are already primed for success, either intentionally or unintentionally. Thereby further amplifying inequities in higher education rather than providing mentorship to those who could benefit most from developmental support.
Relationship Initiation.
Finally, relationship initiation, or the mechanism by which a mentoring relationship is established, may also influence mentoring outcomes. In workplace and youth mentoring, formal mentoring relationships are commonly initiated by assigning or matching by a third-party (Ragins and Cotton, 1999). In contrast, informal mentoring relationships develop spontaneously between mentors and mentees and on the basis of mutual liking, attraction, and perceived interpersonal comfort (Kram, 1985; Ragins and Cotton, 1999). Informal relationships have been shown to confer greater benefits than formal relationships (Chao et al., 1992; Ragins and Cotton, 1999; Inzer and Crawford, 2005), but the differences are small in magnitude (Eby et al., 2013). Although research shows that graduate students develop and maintain formal and informal mentoring relationships with faculty members, postdoctoral associates, and peers (Griffin et al., 2018; Feldon et al., 2019), the effects of relationship initiation have not been studied in graduate student–research advisor mentoring relationships.
In some science graduate programs, relationship initiation occurs through laboratory rotations, which allow students to explore research lab environments of potential mentors and mentors to get to know students before both commit to a match (National Academies of Sciences, 2018; Artiles et al., 2023). Matching after developing some familiarity (i.e., following rotations) is akin to informal relationship initiation in organizational and youth settings. Other graduate programs rely on direct admissions, a process through which a student expresses an interest in working with a particular faculty member and the faculty member then agrees to sponsor them for admissions (or not). In this process, the prospective student and faculty member may have limited interaction before the student joins a mentor’s research group, more akin to formal mentoring in organizational and youth settings. These different approaches to relationship initiation may influence a mentee’s receipt of mentoring support. Rotations may provide time and space to develop some level of familiarity, liking, and shared interests, which may foster greater closeness, higher relationship quality, and enhanced provision of support. Alternatively, direct admissions may limit opportunities for students to evaluate their compatibility with a faculty mentor before committing to the relationship. Despite the differences in the initiation of these mentoring relationships, no studies have directly investigated the relationship between laboratory rotations and direct admissions on mentorship quality and support.
The Present Study
Mentorship is touted by scholars (Crisp et al., 2017; National Academies of Sciences, 2019) and the popular literature (Martin and Haar, 2021; Heemstra and Garg, 2022) as a high-impact practice that is key for mentee success. Yet, our understanding of variables associated with high-quality graduate student-faculty mentoring relationships is limited. Despite this, mentorship efforts continue to gain popularity without the benefit of evidence regarding how to match mentees and mentors to achieve maximum effectiveness. In the present study, we examined the influence of theorized antecedents on the formation of high-quality doctoral student–faculty mentoring relationships. Guided by the similarity attraction paradigm, we hypothesized that greater deep-level similarity and demographic similarity in a mentoring relationship will positively predict mentoring support and relationship quality. Furthermore, we extrapolated that cultural aware mentoring will uniquely and positively predict mentoring support and relationship quality. We also hypothesized that mentor capital, but not mentee capital, would demonstrate small associations with mentorship quality. Finally, we hypothesized that mentoring relationships initiated informally (i.e., through rotations rather than direct admissions) would display small, positive associations with mentoring support and relationship quality. To test these hypotheses, we used structural equation modeling (SEM) to examine relationships between mentoring antecedents and the career and psychosocial support and relationship quality that a national sample of science doctoral students reported receiving from their research advisors.
METHODS
The data presented are part of a larger study examining mentoring relationships between science doctoral student mentees and faculty research advisor mentors during graduate education. This study was approved and determined to be exempt by the University of Georgia Institutional Review Board (PROJECT00003604).
Participants
Participants were individuals currently conducting or who had done doctoral-level research in the U.S. for at least 1 year within the past year and were pursuing or had earned a PhD in a life science discipline. We focused on doctoral students in life sciences fields for three reasons. First, the life sciences represent the largest and most demographically diverse subfield within STEM graduate education in the U.S (National Center for Science and Engineering Statistics [NCSES], 2023). Given our interest in examining the influence of demographic similarity on mentorship support, limiting our sample to the life sciences enabled analyses that were not likely to be possible in other STEM disciplines with more the limited demographic diversity. Second, mentoring norms and practices as well as the nature of graduate education differ across STEM disciplines, thereby making it necessary to control for disciplinary differences. Finally, given the diverse subdisciplines (e.g., synthetic biology, ecology, biophysics) and range of research approaches used (e.g., bench, field, computational), the life sciences are, in many ways, a microcosm of the broader natural sciences.
We recruited doctoral students directly to minimize potential biases from graduate coordinators or programs choosing to share the study information with individuals who had exclusively favorable mentoring relationships. We emailed doctoral students at their university-affiliated addresses with a study invitation that included a link to an online survey hosted on Qualtrics™. To ensure the sample was reflective of the diversity of the science doctoral student talent pool, we purposefully recruited from Hispanic-Serving Institutions (HSIs), Historically Black Colleges and Universities (HBCUs), and from graduate fellowship programs aimed at advancing diversity and inclusion in STEM fields (e.g., Howard Hughes Medical Institute Gilliam Fellows, National Academies of Sciences Ford Foundation Fellows). To incentivize study participation, eligible doctoral students who completed the survey accurately (i.e., passed attention checks) and in its entirety received a $15 gift card of their choice to Amazon, Chipotle, REI, or Starbucks. In addition, we utilized a modified tailored panel management recruitment approach (Estrada et al., 2014) that included compensation, personalized emails, and study branding to encourage participation.
We received a total of 601 responses with varying degrees of completion. Sixteen of these respondents completed <25% of the items and were removed due to incomplete responses. Fifteen students failed to pass attention checks and these responses were also removed (DeSimone and Harms, 2018). Our final analytic sample consisted of 565 doctoral students who represented 70 public and private institutions, and 38 states from varied geographic regions across the U.S. An average of eight participants (SD = 6.5, range = 1-39) responded from each institution. Participants represented predominantly R1 institutions (highest research activity; 89.7%) with comparably fewer from R2 institutions (higher research activity; 10.3%) according to the Carnegie Classification of Institutions of Higher Education (Indiana University for Postsecondary Research, n.d). Approximately 69% of participants represented public universities, 31% were from private institutions, and 10% represented HSIs or HBCUs.
Additional demographic information for doctoral student participants is summarized in Table 1. Students were asked to report on their mentors’ demographics (e.g., gender, race/ethnicity) and career stage (e.g., assistant, associate, or full professor), which are summarized in Table 2. We used separate items to measure race and ethnicity on the survey. Participants could identify with multiples races: American Indian or Alaskan Native, Asian, Black or African American, Hawaiian or Native Pacific Islander, North African or Middle Eastern, or White.
Description | n (%) | Description | n (%) |
---|---|---|---|
Gender | Research context1 | ||
Woman | 325 (58%) | Bench | 425 (75%) |
Man | 224 (40%) | Computational | 277 (49%) |
Non-binary | 14 (2%) | Fieldwork | 167 (30%) |
Prefer not to respond | 2 (<1%) | Theoretical | 55 (10%) |
Race | Prefer not to respond | 1 (<1%) | |
American Indian or Alaskan Native | 10 (2%) | Years completed | |
Asian | 131 (23%) | 1 | 96 (17%) |
Black or African American | 52 (8.5%) | 2 | 110 (19%) |
Hawaiian or Native Pacific Islander | 3 (<1%) | 3 | 125 (22%) |
North African or Middle Eastern | 15 (2.5%) | 4 | 89 (16%) |
White | 339 (60%) | 5 | 85 (15%) |
Prefer to self-describe | 21 (3.7%) | 6 | 43 (7%) |
Prefer not to respond | 22 (3.8%) | 7+ | 13 (2%) |
Ethnicity | Prefer not to respond | 4 (<1%) | |
Hispanic or Latinx/Latine | 86 (15%) | Relationship initiation | |
Not Hispanic | 465 (82%) | Direct admission | 235 (42%) |
Prefer not to respond | 14 (3%) | Following laboratory rotations | 304 (52%) |
Degree status | Other scenario | 26 (5%) | |
Pre-Candidacy | 208 (37%) | Subjective socioeconomic status | |
Post-Candidacy | 314 (55%) | 1 (bottom, worst off) | 2 (<1%) |
Currently Postdoctoral | 40 (7%) | 2 | 10 (2%) |
Prefer not to respond | 3 (0.5%) | 3 | 32 (6%) |
International status | 4 | 77 (14%) | |
Yes | 121 (21%) | 5 | 91 (16%) |
No | 443 (78%) | 6 | 109 (19%) |
Prefer not to respond | 1 (<1%) | 7 | 147 (26%) |
English First-Language | 8 | 70 (12%) | |
Yes | 472 (84%) | 9 | 18 (3%) |
No | 89 (16%) | 10 (top, best off) | 2 (<1%) |
Prefer not to respond | 4 (<1%) |
Description | n (%) |
---|---|
Gender | |
Woman | 196 (35%) |
Man | 364 (64%) |
Prefer not to respond | 5 (<1%) |
Race | |
American Indian or Alaskan Native | 3 (<1%) |
Asian | 75 (13%) |
Black or African American | 12 (2%) |
Hawaiian or Native Pacific Islander | 1 (<1%) |
North African or Middle Eastern | 11 (2%) |
White | 430 (76%) |
Prefer to self-describe | 10 (2%) |
Prefer not to respond | 31 (5%) |
Ethnicity | |
Hispanic or Latinx/Latine | 28 (5%) |
Not Hispanic | 517 (92%) |
Prefer not to respond | 20 (3%) |
Rank | |
Assistant Professor | 114 (20%) |
Associate Professor | 138 (24%) |
Full Professor | 292 (52%) |
Other | 14 (2%) |
Prefer not to respond | 7 (1%) |
Measures
We collected data using a cross-sectional study design and established survey measures. Specific details about the measures are described below. A complete list of the measures and items are included in the Supplemental Materials. Participants were instructed to think about their dissertation advisor and this individual’s role as a mentor during their graduate research while responding to the statements. If participants were coadvised or had multiple dissertation advisors, they were asked to pick one individual to respond about. Unless otherwise noted, variables were measured using five-point Likert scales (i.e., 1 = “strongly disagree”; 5 = “strongly agree”) and items were coded so that higher values represent greater amounts of the construct.
Culturally Aware Mentoring.
We used a five-item scale (Byars-Winston and Butz, 2021) to measure the extent to which mentees perceived their advisor’s mentoring behaviors reflected racial and ethnic cultural awareness, such as being aware of and willing to discuss racial and ethnic differences. We modified the response format of this scale from a five-point frequency response (i.e., 1 = “never”; 5 = “all of time”) to an agreeability response (i.e., 1 = “strongly disagree”; 5 = “strongly agree”) format because we were interested in mentees’ perceptions of their mentor’s ability to act with awareness of race and ethnicity in their mentoring relationship. A sample item is “My mentor was willing to discuss race and ethnicity, even if it may have been uncomfortable for them.”
Deep-Level Similarity.
We used an eight-item scale (Ensher et al., 2002; de Janasz and Godshalk, 2013) to measure the degree to which mentees perceived their mentor to be similar to themselves in terms of their attitudes, perspectives, and values. Sample items include “My mentor and I see things in the same way” and “My mentor and I are more similar than dissimilar in important ways.”
Gender Similarity.
We created a dummy-coded, binary measure of gender similarity between mentors and mentees using the gender designations (e.g., man, nonbinary, woman) reported by the mentee for themselves and their mentor. Mentees who reported being in cross-gender (differing gender match within the dyad) relationships were coded as 0 (cross gender) and same-gender (matching gender within the dyad) relationships were coded as 1 (same gender). Participants who selected “prefer not to respond” for their own or their mentor’s gender were omitted from our measure of gender similarity because we could not determine whether they were in a similar or dissimilar dyad. Total sample sizes for gender similarities by dyadic composition are included in Tables S1–S3 of the Supplemental Materials.
Mentor Human Capital.
We operationalized mentor human capital from participant reports of faculty member rank. We used these data to create a mentor human capital score, ranging from less to more human capital where: 1 = Assistant Professor, 2 = Associate Professor, 3 = Professor or Administrator (e.g., department head).
Mentee Capital.
We used the MacArthur Scale of Subjective Social Status (i.e., the MacArthur ladder) as a subjective measure of social and economic status (Adler et al., 2000). We used this single item measure of subjective social status because we predicted that an individual’s subjective social status could be interpreted by a mentor as a general indicator of a mentee’s potential and ability. We presented participants a drawing of a ladder with 10 rungs and the following description: “Think of the ladder as representing where people stand in our society. At the top of the ladder are the people who are the best off, who have the most money, most education, and best jobs. At the bottom are the people who are the worst off, those who have the least money, least education, worst jobs, or no job.” Participants were asked to place themselves on the ladder based on a 10-point scale (1 = bottom, worst off; 10 = top, best off), and the scores were used as indicators of mentee capital.
Race and Ethnicity Similarity.
We measured racial and ethnic similarity in a precise manner by disaggregating racial and ethnic groups. Specifically, we created a dummy-coded, binary measure of racial and ethnic similarity between mentors and mentees using the race and ethnicity designations reported by the mentee for themselves and their mentor. Mentees who reported being racially or ethnically heterogeneous (differing race or ethnicity within the dyad) were coded as 0 (i.e., cross-race and ethnicity), and mentees in racially and ethnically homogeneous relationships (both the same race and the same ethnicity within the dyad) were coded as 1 (same-race and ethnicity). For example, a Hispanic/Latine and White mentee with a Hispanic/Latine and White mentor would be coded as 1, and a Hispanic/Latine and Black/African American mentee with Hispanic/Latine and White mentor would be coded as 0. Although this reduces our statistical power and increases the risk of a Type II statistical error, we elected to use this approach to more precisely test the influence of racial and ethnic similarity on mentoring functions and quality. Participants who selected “prefer not to respond” for their own or their mentor’s race and/or ethnicity were omitted from our measure of racial and ethnical similarity because we could not reasonably determine if they were in a similar or dissimilar dyad. Total sample sizes for racial and ethnic similarities by dyadic composition are included in Tables S1, S4, and S5 of the Supplemental Materials.
Relationship Initiation.
We operationalized relationship initiation by asking participants to indicate whether they joined their graduate research mentor’s lab via direct admission, following rotations, or through another scenario. Mentees who were direct admits were dummy-coded as 0 (i.e., formal relationship initiation), and mentees who joined a laboratory following rotations were coded as 1 (i.e., informal relationship initiation). We reviewed all participant responses who selected “another scenario” and manually coded responses based on text descriptions as being formal or informal relationship initiation when possible. We omitted responses from students whom we were unable to categorize their relationships as being formal or informal.
Career Support.
We used a ten-item scale (Tuma, Adams, Choi, & Dolan, unpublished data) to measure the perceived career-related support received from the mentor (e.g., coaching, sponsorship, protection, networking, visibility, appropriately challenging tasks). Sample items include “My mentor offers useful advice for achieving my career goals” and “My mentor helps me prepare for important milestones in my degree.”
Psychosocial Support.
We used an eight-item scale (Tuma et al., unpublished data) to measure the perceived psychosocial support received from the mentor (e.g., counseling, encouragement, friendship, role modeling, acceptance). Sample items include “My mentor tells me when they think I have done a good job” and “My mentor values me as a person.”
Relationship Quality.
We used a four-item scale (Tuma et al., unpublished data) to measure the perceived quality of the mentoring relationship (i.e., the general satisfaction and overall quality and reciprocity of the relationship). A sample item is “My mentor and I can talk about things other than work tasks.”
Demographic Variables.
Mentee and mentor gender, race, and ethnicity were reported by the mentee and included as variables in the structural models. Mentee gender was recoded in two dummy-coded variables (i.e., woman = 1 or not woman, meaning nonbinary or man = 0; and nonbinary = 1 or not nonbinary, meaning woman or man = 0). Mentor gender was recoded in to one dummy-coded variable with men included as the reference group (e.g., woman = 1, man = 0). Mentee and mentor ethnicity were each recoded into a dummy-coded variable with non-Hispanic/Latine individuals as the reference group (i.e., Hispanic/Latine = 1, non-Hispanic/Latine = 0). Mentee race was recoded in to five dummy-coded variables and mentor race was recoded in to four-dummy coded variables, one for each racial identity (e.g., Asian = 1, non-Asian = 0).
Data Analysis
We followed a two-phase SEM process (i.e., assessment of measurement model followed by evaluation of a structural model specifying how mentoring antecedent variables related to mentoring support and quality) to address our research questions (Kline, 2015; Zyphur et al., 2023). SEM utilizes a set of statistical techniques to model complex relationships between multiple latent variables simultaneously, enabling the testing of multiple hypotheses and competing theoretical models and controlling for measurement error variance. Thus, SEM allows for more robust and accurate estimates of effects (MacCallum and Austin, 2000; Zyphur et al., 2023).
Preliminary Analyses.
We conducted an a priori power analysis for global hypothesis testing to determine the number of observations necessary to yield sufficient power in order to reject the null hypothesis and detect associations among the latent variables. We used the semPower package (Jobst et al., 2021) and the RMSEA fit index to assess model misspecification. The power analysis indicated that, to achieve an 80% chance of correctly rejecting the null hypotheses (ß = 0.20) with an α of 0.05 and a df of 589, n = 63 observations were required to detect model misspecification with a RMSEA of 0.05. Our final sample size of 565 observations is well above this number, indicating sufficient statistical power to assess the overall fit of the model.
Before assessing our structural models, we conducted a series of preliminary analyses to evaluate the plausibility of statistical assumptions for our structural models. We began by determining whether the patterns of missing data were missing completely at random (MCAR), missing at random, or missing not at random by conducting Little’s MCAR test (Little, 1988). The results revealed that the missing data were consistent with MCAR, χ2(1420) = 1395, p = 0.31. We, therefore, used maximum-likelihood estimation without adjustments to address missing data. Most items had a skewness and kurtosis below |1.0| and all items had a skewness below |1.70| and kurtosis below |2.50|, thereby meeting assumptions for multivariate normality. Finally, we examined the degree to which our responses were nested within 70 institutions, thereby violating the assumption of independence between observations in our sample (i.e., students clustered by institution). We calculated intraclass correlation coefficients (ICC) for each outcome variable to determine the clustering at the institutional level using the lme4 linear mixed-effects model package (Bates, 2010). The ICC values (career support = 0.010, psychosocial support = 0.029 and relationship quality = 0.016) were low, suggesting very little variability at the institution level. Therefore, we opted not to pursue a multilevel SEM (Stapleton et al., 2016).
Assessment of Measurement Models.
We evaluated our measurement models (e.g., culturally aware mentoring, deep-level similarity, career support, psychosocial support, and relationship quality) using confirmatory factor analysis (CFA) with robust maximum likelihood estimation to determine how adequately our model reproduced the variance-covariance matrix. We assessed measurement model fit by examining both incremental (e.g., Comparative Fit Index [CFI], Tucker-Lewis Index [TLI]) and absolute (e.g., Root Mean Square of Approximation [RMSEA], Standardized Root Mean Square Residual [SRMR], Chi-Square Test [χ2]) indices of model fit. We further assessed the goodness of model fit with equivalence testing (Marcoulides and Yuan, 2017; Peugh and Feldon, 2020) and adjusted, “T-size” fit statistics (e.g., RMSEAT, CFIT). In general, our analyses revealed good model fit and confirmed unidimensionality of the scales. To provide evidence of reliability, we used coefficient omega (ω) to evaluate the internal consistency of the scales (Dunn et al., 2014). A comprehensive description of our approaches and model fit indices are reported in the Supplemental Materials.
Assessment of Structural Models.
We tested our structural models by specifying the hypothesized relationships between our variables of interest (i.e., path analysis) as depicted in Figure 1. All of the analyses were conducted in R software version 4.1.0 (R Core Team, 2016) using the lavaan package for latent variable modeling (Rosseel, 2012). We used the maximum likelihood robust method to examine the degree to which our conceptual model fit the data, with the theorized antecedents as predictors of our outcome variables of interest. Specifically, we modelled the extent to which mentee reports of culturally aware mentoring, deep-level similarity, mentor human capital, mentee capital, gender similarity, race/ethnic similarity, and relationship initiation predicted mentee perceptions of career support, psychosocial support, and relationship quality.
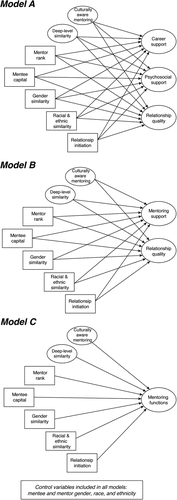
FIGURE 1. Conceptual models of relationships between antecedent variables and indicators of quality mentorship. We examined three structural models to the identify and test the relationships between hypothesized mentoring antecedents and forms of mentoring support (i.e., career and psychosocial support) and relationship quality. Mentee and mentor gender, race, and ethnicity were included as demographic control variables in all models. Solid black lines indicate tested paths examining the influence of exogenous variables on endogenous variables. Rectangles indicate observed indicator variables and ellipses indicate latent variables. Observed indicators for latent variables and covariances are omitted for parsimony.
We tested a series of competing models to identify the structural model that was most parsimonious and representative of the relationships in the data. Our first structural model (Model A) tested the relationships between the theorized antecedent variables and individual mentoring variables (i.e., career support, psychosocial support, and relationship quality). Because all three mentoring variables are highly correlated (Table 3), we tested two additional alternative and nested models (Figure 1). The first nested model (Model B) estimated the relationships between the antecedent variables and relationship quality and a combined construct of mentoring support where all items from the mentoring support scales (e.g., career and psychosocial support) loaded on to one factor. The second nested model (Model C) estimated relationships between the antecedent variables and a single global construct for mentoring processes (e.g., career support, psychosocial support, and relationship quality) where all items from these scales loaded on to one factor. All structural models included mentee and mentor gender, race, and ethnicity as separate variables. We used adjusted p values to account for multiplicity with the Benjamini-Hochberg method to control the false discovery rate (Benjamini and Hochberg, 1995). Specifically, we calculated a critical value for each p value using the formula (i/m)*Q, where i is the rank of the p value from lowest to highest, m is the total number of tests run, and Q is our chosen false discovery rate. Variables were considered significant at an FDR rate of 5%, which corresponded to Padj< 0.005.
Variable | N | M | SD | Ω | Correlations | ||||||||
---|---|---|---|---|---|---|---|---|---|---|---|---|---|
1 | 2 | 3 | 4 | 5 | 6 | 7 | 8 | 9 | |||||
1. Career support | 565 | 3.83 | 0.80 | 0.92 | – | ||||||||
2. Psychosocial support | 565 | 3.76 | 1.04 | 0.96 | 0.79* | – | |||||||
3. Relationship quality | 565 | 4.02 | 0.95 | 0.91 | 0.70* | 0.81* | – | ||||||
4. Culturally aware mentoring | 548 | 3.28 | 0.93 | 0.90 | 0.60* | 0.62* | 0.54* | – | |||||
5. Deep–level similarity | 565 | 3.27 | 0.89 | 0.94 | 0.67* | 0.75* | 0.73* | 0.56* | – | ||||
6. Mentor rank | 557 | 2.34 | 0.82 | – | –0.13* | –0.08 | –0.06 | –0.10* | –0.07 | – | |||
7. Mentee capital | 558 | 5.92 | 1.65 | – | 0.14* | 0.12* | 0.10* | 0.06 | 0.16* | –0.02 | – | ||
8. Gender similarity | 558 | 0.50 | 0.50 | – | 0.08 | 0.01 | 0.02 | 0.03 | 0.04 | 0.01 | 0.06 | – | |
9. Racial & ethnic similarity | 517 | 0.48 | 0.50 | – | 0.04 | –0.04 | –0.03 | 0.05 | 0.07 | 0.04 | 0.17* | 0.01 | – |
10. Relationship initiation | 547 | 0.56 | 0.50 | – | –0.05 | 0.03 | 0.00 | –0.02 | –0.01 | –0.02 | –0.03 | –0.05 | –0.16* |
RESULTS
Descriptive Statistics and Zero-Order Correlations
Means, standard deviations, and zero-order correlations of all variables are reported in Table 3. Doctoral students in our study reported receiving moderate amounts of career (M = 3.83; SD = 0.80) and psychosocial (M = 3.76; SD = 1.04) support from their mentors and indicated that their relationships were of generally high quality (M = 4.02; SD = 0.95; Table 1). As expected, the zero-order correlations showed positive associations between career and psychosocial support and relationship quality. Two antecedent variables (i.e., culturally aware mentoring and deep-level similarity) were strongly positively associated (r = 0.54 – 0.75) with all three mentoring variables. Finally, the association between mentee capital and the mentoring variables and deep-level similarity were small and positive (r = 0.10 – 0.16).
Measurement Model Fit
Complete measurement model fit results for each latent variable are reported in the Supplemental Materials. The CFA results for the culturally aware mentoring and deep-level similarity scales indicated good model fit. However, the model fit for the career and psychosocial support and the relationship quality scales indicated slight model misfit, which was addressed as detailed in the Supplemental Materials. Before proceeding with the structural phase, we fit a multivariate CFA to identify potential issues of multidimensionality and determine the global fit of the five major latent variables included in the structural model (i.e., culturally aware mentoring, deep-level similarity, career support, psychosocial support, and relationship quality). Our model fit the data very well (CFI = 0.95, TLI = 0.94, RMSEA = 0.05, SRMR = 0.04) and all factor loadings (λ) were > 0.50. These results provided sufficient support for our measurement model such that we could proceed with assessing the structural models.
Structural Model Fit
We used our entire analytical sample to test and compare multiple, theoretically plausible structural models to determine the influence of various mentoring antecedents on doctoral students’ reports of mentoring support. Model A (Figure 1), which includes direct effects of predictor variables on doctoral students’ reports of career and psychosocial support and the quality of their mentoring relationships, had good model fit - χ2 (2092) = 1059 (p < 0.001), CFI = 0.94, TLI = 0.92, RMSEA = 0.04, SRMR = 0.04. Our two alternative structural models showed comparatively poorer fit (Model B - χ2 (2472) = 1082 (p < 0.001), CFI = 0.91, TLI = 0.89, RMSEA = 0.05, SRMR = 0.04 and Model C - χ2 (2741) = 1104 (p < 0.001), CFI = 0.89, TLI = 0.87, RMSEA = 0.05, SRMR = 0.04). Equivalence testing for Model A indicated that the CFIT was 0.926 and the RMSEAT was 0.044 with adjusted fit values.3 Both the T-Size CFI and the T-Size RMSEA indicated close model fit suggesting acceptable model fit to the data. Given that Model A was best supported by evidence and indicated adequate fit, we proceeded with our hypothesis testing and interpretation of the paths in this model.
Relationship between Mentee and Mentor Demographics and Mentorship Quality
We first examined whether mentees experienced differences in mentoring support and relationship quality based on their gender, race, and ethnicity. Compared to their non-Asian peers, Asian students reported slightly higher levels of psychosocial support (Table 4). However, students otherwise reported experiencing similar levels of their mentorship support and relationship quality regardless of their gender, race, or ethnicity (Table 4). We also examined whether mentors of different gender, racial, and ethnic backgrounds were perceived as providing different levels of support or as engaging in relationships of varying quality. Again, students reported similar levels of support and relationship quality regardless of their mentors’ gender, racial, and ethnic characteristics (Table 4).
Predictor | Career Support | Psychosocial Support | Relationship Quality | |||||||||
---|---|---|---|---|---|---|---|---|---|---|---|---|
b | SE | β | p value | b | SE | β | p value | b | SE | β | p value | |
Culturally aware mentoring | 0.20 | 0.03 | 0.34 | <0.001 | 0.29 | 0.04 | 0.30 | <0.001 | 0.16 | 0.04 | 0.17 | <0.001 |
Deep–level similarity | 0.32 | 0.03 | 0.52 | <0.001 | 0.64 | 0.04 | 0.64 | <0.001 | 0.68 | 0.05 | 0.68 | <0.001 |
Mentor rank | –0.03 | 0.02 | –0.04 | 0.16 | 0.02 | 0.03 | 0.02 | 0.55 | 0.03 | 0.03 | 0.03 | 0.35 |
Mentee capital | 0.01 | 0.01 | 0.03 | 0.28 | 0.01 | 0.01 | 0.01 | 0.59 | 0.01 | 0.02 | 0.01 | 0.88 |
Gender similarity | 0.04 | 0.03 | 0.04 | 0.21 | –0.05 | 0.05 | –0.02 | 0.36 | –0.03 | 0.06 | –0.01 | 0.66 |
Racial & ethnic similarity | 0.05 | 0.04 | 0.05 | 0.24 | –0.12 | 0.06 | –0.07 | 0.06 | –0.19 | 0.07 | –0.11 | 0.01 |
Relationship initiation | –0.07 | 0.03 | –0.06 | 0.04 | 0.02 | 0.05 | 0.01 | 0.67 | –0.01 | 0.05 | –0.01 | 0.93 |
Mentee gender: woman | –0.02 | 0.04 | –0.02 | 0.64 | 0.01 | 0.05 | 0.00 | 0.86 | 0.02 | 0.06 | 0.01 | 0.71 |
Mentee gender: non–binary | –0.28 | 0.11 | –0.09 | 0.01 | –0.33 | 0.15 | –0.06 | 0.03 | –0.24 | 0.17 | –0.04 | 0.16 |
Mentor gender: woman | 0.00 | 0.03 | –0.00 | 0.83 | –0.04 | 0.05 | –0.02 | 0.40 | –0.08 | 0.06 | –0.04 | 0.15 |
Mentee race: American Indian | 0.14 | 0.12 | 0.03 | 0.26 | 0.28 | 0.17 | 0.05 | 0.11 | –0.02 | 0.21 | 0.00 | 0.94 |
Mentee race: Asian | 0.10 | 0.05 | 0.08 | 0.02 | 0.23 | 0.07 | 0.11 | 0.001 | 0.10 | 0.08 | 0.05 | 0.18 |
Mentee race: Black | 0.15 | 0.06 | 0.09 | 0.01 | 0.10 | 0.09 | 0.03 | 0.28 | 0.06 | 0.11 | 0.02 | 0.55 |
Mentee race: Pacific Islander | –0.14 | 0.22 | –0.02 | 0.50 | –0.16 | 0.31 | –0.01 | 0.61 | –0.28 | 0.36 | –0.02 | 0.43 |
Mentee race: Middle Eastern | 0.03 | 0.10 | 0.01 | 0.79 | –0.37 | 0.15 | –0.07 | 0.01 | –0.33 | 0.17 | –0.06 | 0.06 |
Mentee ethnicity: Hispanic | 0.06 | 0.05 | 0.04 | 0.26 | –0.11 | 0.07 | –0.04 | 0.12 | –0.07 | 0.08 | –0.03 | 0.42 |
Mentor race: American Indian | 0.38 | 0.22 | 0.06 | 0.09 | 0.21 | 0.31 | 0.02 | 0.45 | 0.25 | 0.36 | 0.02 | 0.48 |
Mentor race: Asian | 0.04 | 0.05 | 0.03 | 0.42 | 0.01 | 0.07 | 0.00 | 0.95 | –0.01 | 0.08 | –0.01 | 0.91 |
Mentor race: Black | 0.01 | 0.11 | 0.00 | 0.93 | –0.16 | 0.16 | –0.03 | 0.30 | –0.44 | 0.18 | –0.08 | 0.02 |
Mentor race: Middle Eastern | 0.17 | 0.12 | 0.05 | 0.15 | 0.19 | 0.16 | 0.03 | 0.24 | 0.19 | 0.19 | 0.03 | 0.31 |
Mentor ethnicity: Hispanic | –0.05 | 0.07 | –0.02 | 0.50 | 0.00 | 0.11 | 0.00 | 0.99 | 0.07 | 0.12 | 0.02 | 0.58 |
Variance predicted | R2 = 0.65 | R2 = 0.75 | R2 = 0.65 |
Main Influences of Mentoring Antecedents on Mentorship Support
Deep-level similarity was the strongest predictor of quality mentorship among all antecedent variables tested in this study, as indicated by having largest standardized path coefficients. As hypothesized, the parameter estimates reported in Model A indicated that doctoral students’ perceptions of deep-level similarity with their research advisors exhibited unique and large positive associations with mentoring support and relationship quality, after controlling for demographic characteristics (estimated path coefficients are reported in Table 4). For example, a 1 SD increase in deep-level similarity was associated with a 0.52 SD increase in career support, a 0.64 SD increase in psychosocial support, and a 0.68 SD increase in relationship quality.
Similarly, students’ perceptions that their advisors engaged in culturally aware mentoring had significant, positive effects beyond that of deep-level similarity, although the effects were more modest than for deep-level similarity. For instance, a 1 SD increase in culturally aware mentoring was associated with a 0.34 SD increase in career support, a 0.30 SD increase in psychosocial support, and a 0.17 SD increase in relationship quality. The relationships between all other antecedents (i.e., mentor rank, mentee capital, gender similarity, racial and ethnic similarity, relationship initiation) were not meaningfully associated with mentee perceptions of career support, psychosocial support, and relationship quality. Overall, the proportion of the variance explained (R2) by the antecedent and demographic variables included in the model was 65% for career support, 75% for psychosocial support, and 65% for relationship quality.
Moderation Effects of Sociodemographics
Existing mentoring theory proposes that demographic similarity may be particularly salient for individuals of certain demographic groups (Ragins, 1997; Ragins and Cotton, 1999), yet this notion has largely evaded substantive examination. Through a series of posthoc analyses, we examined whether the influence of surface-level similarity on mentorship quality varied in its impact across gender and racial/ethnic groups. Specifically, we examined whether the relationship between either gender match or racial and ethnic match and mentorship quality (e.g., career support, psychosocial support, relationship quality) was moderated by demographic group membership (e.g., gender or race/ethnicity), such that the effect of demographic similarity on mentorship quality varied for individuals belonging to different demographic groups. We also report descriptive statistics regarding levels of mentoring support and relationship quality disaggregated by mentee–mentor gender, racial, and ethnic dyad composition (i.e., gender matching as matching or not for each gender group, ethnicity matching as Hispanic/Latine matching or not, and race matching as matching or not for each racial group) in the Supplemental Materials (Supplemental Figure S3–5).
To enhance transparency and minimize our potential biases, we preregistered our hypotheses and exploratory posthoc analyses (https://osf.io/5j8dv). Our preregistered analytical approach was designed to utilize a series of multigroup SEMs to assess the invariance of path coefficients across various gender and racial/ethnic groups. However, our sample was underpowered to detect significant differences between all possible comparisons (e.g., Hispanic/Latine vs. non-Hispanic/Latine) using a multigroup SEM model. We opted not to include categorical manifest variables (e.g., particular gender, race, or ethnic groups) as interactions in the SEM because of the limited sample sizes across the demographic groups and in our precise measures of gender and racial and ethnic similarity. Therefore, we opted to explore possible moderation effects on the relationship between demographic similarity and mentorship quality for gender and Asian, racially minoritized, and White students using Kruskal-Wallis tests (i.e., nonparametric ANOVAs). We opted to combine individuals who identified as American Indian or Alaskan Native, Black or African American, Hawaiian, or Native Pacific Islander, and North African or Middle Eastern in to one broad composite variable termed, “racially minoritized” (RM).4 We recognize that these groups are not monolithic in their experiences and this pooling may mask individual patterns specific to each group. Although distinct, individuals of these racially minoritized backgrounds collectively as a group have both historically and currently face the structurally racist systems endemic in STEM higher education. Thus, individuals of these backgrounds may share some similarities in collectively experiencing racial minoritization in STEM fields. Because ethnicity was measured separately from racial identity with a dichotomous variable, we used Wilcoxon Rank Sum Tests (i.e., nonparametric t tests) to test for potential differences for Hispanic/Latine mentee–mentor dyads.
Gender composition of mentoring dyads was unrelated to mentee reports of career support (H (5) = 5.31, p = 0.39), psychosocial support (H (5) = 3.05, p = 0.69), and relationship quality (H (5) = 5.28, p = 0.38; Figure 2). Only Asian students reported varied levels of career support (H (2) = 13.01, p = 0.001, η2 = 0.09) and psychosocial support (H (2) = 8.57, p = 0.01, η2 = 0.05), but not relationship quality (H (2) = 4.21, p = 0.12) based on their dyad composition (Figure 3A). Posthoc tests using Dunn’s multiple comparisons procedure revealed that both Asian mentee-Asian mentee-Asian mentor dyads reported significantly lower levels of career support (p = 0.006) and psychosocial support (p = 0.01), but similar relationship quality (p = 0.14) when compared with Asian mentee-RM mentor dyads. No significant differences in career support (p = 0.99), psychosocial support (p = 0.99), and relationship quality (p = 0.99) were found between Asian mentee-Asian mentor dyads and Asian mentee-White mentor dyads. Hispanic mentee-Hispanic mentor dyads reported statistically similar levels of career support (p = 0.75), psychosocial support (p = 0.67), and relationship quality (p = 0.48) compared with Hispanic mentee-non-Hispanic mentor dyads (Figure 3B). Racially minoritized mentees also reported similar levels of career support (H (2) = 2.72, p = 0.25), psychosocial support (H (2) = 2.53, p = 0.28), and relationship quality (H (2) = 1.38, p = 0.50) regardless of the racial composition of their dyad (Figure 3C). Finally, White mentee perceptions of perceived career support (H (2) = 3.46, p = 0.17), psychosocial support (H (2) = 1.59, p = 0.45), and relationship quality (H (2) = 2.32, p = 0.31) also did not differ based on the racial composition of their dyad (Figure 3D).
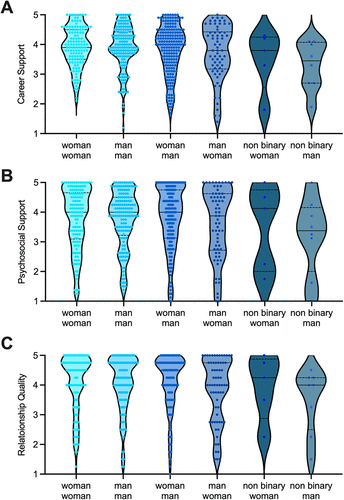
FIGURE 2. Effects of gender composition of the dyad on mentoring support and relationship quality. Mentee reports of received (A) Career support, (B) Psychosocial support, and (C) Relationship quality by mentee–mentor gender composition. Violin plots are labeled with mentee gender on top and mentee-reported gender of the mentor on the bottom. Plots show distribution of data with solid lines indicating the median and the dashed lines representing the first and third quartiles. Significance was derived from Kruskal-Wallis tests; no significant differences were observed.

FIGURE 3. Effects of racial and ethnic composition of the dyad on mentoring support and relationship quality. (A) Asian mentee perceptions of career support, psychosocial support, and relationship quality from Asian, racially minoritized (RM), or White mentors, (B) Hispanic/Latine mentee perceptions of career support, psychosocial support, and relationship quality from Hispanic/Latine or non-Hispanic/Latine mentors, (C) RM mentee perceptions of career support, psychosocial support, and relationship quality from RM, Asian, and White mentors, and (D) White mentee perceptions of career support, psychosocial support, and relationship quality from White, Asian, and RM mentors. Violin plots show distribution of data with solid lines indicating the median and the dashed lines representing the first and third quartiles. Significance was derived from Kruskal-Wallis tests and Dunn’s test for posthoc comparisons (A, C, and D) or Wilcoxon Rank Sum tests (B). p < 0.05.
DISCUSSION
In the present study, we used SEM to examine whether antecedent variables often considered, either explicitly or implicitly, in the process of matching graduate student mentees with research advisor mentors affect the support that mentees experienced and the quality of their mentoring relationships. Consistent with studies of mentoring at the undergraduate level and in the workplace, our results provide some of the most robust evidence to date showing that deep-level similarity is strongly and positively associated with the provision of career and psychosocial support and with relationship quality in doctoral mentoring relationships (Ensher et al., 2002; Turban et al., 2002; Eby et al., 2013; Ghosh, 2014; Hernandez et al., 2017; Pedersen et al., 2022). This finding is encouraging because deep-level similarity is malleable and can be identified and cultivated in mentoring relationships. Mentees can work to identify potential advisors who share their outlook, perspectives, and values before selecting them as a mentor. For instance, students could consider discussing their personal values, experiences, and viewpoints to determine compatibility and identify areas of alignment with a potential faculty advisor before selecting them as a research mentor. Students may also benefit from seeking out feedback from existing lab members who are familiar with the advisor and can provide additional perspectives on their advisor’s values, personality, and attitudes.
Once a mentee is paired with a mentor, the pair can work to cultivate deep-level similarities. The development and continued cultivation of deep-level similarities within the dyad has the potential to enhance the provision of support and ultimately the quality of the relationship. For example, mentees and mentors can work together to find and affirm commonalities (e.g., nonlinear paths into research, areas they care about and value outside of research/work, etc.). The Entering Mentoring curriculum includes a series of questions and talking points that mentors can use to start these conversations, including “things to ask” and “things to tell” (Pfund et al., 2015). These talking points focus on sharing often implicit information about how a lab group operates to help mentees onboard. They also present an avenue for mentors to share an appropriate level of information about their personal lives, such as their hobbies or their weekend plans, to the extent that they feel comfortable doing so. These kinds of self-disclosure can foster closeness in a relationship, which in turn can enhance the quality of the relationship and the provision of support. Experimental research from educational contexts provides evidence indicating that structured activities can promote feelings of deep-level similarity in student–teacher and undergraduate–faculty relationships (Gehlbach et al., 2016; Hernandez et al., 2023). A similar intervention could be developed and tested with graduate students and research advisors for its potential to enhance mentee and mentor perceptions of deep-level similarity, mentoring support, and relationship quality. Such interventions may be particularly well suited for creating stronger perceptions of similarity between mentors of well-represented backgrounds and mentees from marginalized and minoritized backgrounds.
We also found that mentees reported greater support and higher relationship quality with mentors who demonstrated willingness and ability to discuss race and ethnicity in a respectful way. This finding adds to the documented outcomes associated with culturally aware mentoring and mentorship quality (Haeger and Fresquez, 2016; Byars-Winston et al., 2018; Black et al., 2022; Pfund et al., 2022). Emerging culturally aware mentoring professional development efforts, which require only a modest time investment, show some potential for supporting faculty developing their culturally aware mentoring skills (Byars-Winston et al., 2023). However, mentoring professional development is likely not a “magic bullet” for achieving effective mentoring relationships. Participation in mentoring professional development is largely not incentivized or rewarded (Tuma et al., 2021; White-Lewis et al., 2022). In fact, only 7% of faculty report engaging in significant mentor professional development (Stolzenberg et al., 2019). Without reward systems in place for participation in mentoring professional development and provision of quality mentorship, change efforts are likely to be limited (National Academies of Sciences, 2019). Given the decentralized structure of graduate education (i.e., departments, labs, and dyads operate mostly independently), there is also potential for idiosyncrasies in the extent to which mentors feel it is necessary to notice and respond to cultural differences in their research mentoring relationships. This underscores the need for institutions to shift the current systems towards incentivizing, recognizing, supporting, and rewarding quality mentorship (National Academies of Sciences, 2019).
Conventional wisdom emphasizes the importance of demographic matching to promote mentorship quality. Using one of the largest and most demographically diverse samples of science doctoral students to date, we found that shared mentee–mentor demographic characteristics were not associated with greater mentoring support or higher relationship quality. Our results are consistent with a number of studies in academic contexts that have found no or weak effects of the influence of surface-level similarities on mentorship quality (Blake-Beard et al., 2011; Hernandez et al., 2017; Pedersen et al., 2022; Kuchynka et al., 2023). At first, these findings may seem paradoxical to the similarity attraction paradigm, which suggests that individuals will gravitate towards others who are similar (Byrne, 1961). These results may also seem at odds with the powerful influence that both similar and counter-stereotypical role models can exert on minoritized individuals in STEM fields (Herrmann et al., 2016; Gladstone and Cimpian, 2021). It is possible that surface-level similarities may yield negligible effects on mentorship quality because gender and race/ethnicity are only one aspect of an individual’s identity, and individuals from the same gender or racial/ethnic backgrounds can have vastly different life experiences, cultures, and values (Harper and Nichols, 2008). While individuals of similar gender and race/ethnic backgrounds likely have similar experiences, these categorizations may obscure within-group differences of individuals’ lived experiences and self-concepts. In addition, it may be that surface-level similarity is more salient for fleeting or unidirectional influences such as role models, while the enduring nature of mentoring relationships enriches mentees’ insights about their mentors in ways that inform their judgments of similarity.
We also observed that most individuals from varying demographic groups reported similar levels of mentoring support and relationship quality regardless of whether they were in demographically similar or dissimilar dyads. These results are noteworthy because we anticipated that gender or race may have moderated the relationship between demographic match and mentoring quality for certain groups, particularly for those with minoritized identities in STEM fields. However, only Asian mentees reported differing levels of career and psychosocial support based on their mentor’s race. Specifically, Asian mentees with Asian and White mentors reported lower mentoring support than Asian mentees with racially minoritized mentors. While these effects are medium in size, it is important to note that the majority of subsamples for these analyses are limited in size (Supplemental Figure S4). Furthermore, we did not disaggregate students by nationality, and we treated Asian students as a monolith, despite significant cultural and historical differences between countries in Asia. We caution readers from overinterpreting these results given the potential confounds and the fact that our sample sizes between groups are unbalanced and limited. Future research should first determine whether the findings from our moderation analyses are replicable. If so, further research should aim to understand the specific factors underpinning these effects and the mechanisms through which they influence the establishment of quality mentoring relationships. These results also call attention for finer grained analyses aimed at addressing the mentoring experiences of mentees and mentors who represent unique demographic intersections, which has been limited in the mentoring literature to date.
Our results also indicated that two factors that commonly influence how graduate student mentees in the sciences match with their research mentors – namely relationship initiation and mentor rank – are not meaningfully associated with mentee reports of support or relationship quality. These results suggest that, in terms of setting graduate students up for quality mentorship, graduate programs are justified in allowing for both rotations and direct admissions. In addition, mentees can be reassured that the rank of their faculty mentor is not likely to limit their development of a quality mentoring relationship. This result is somewhat surprising; we anticipated that mentors with greater human capital would provide a greater breadth and depth of resources to their mentees, thereby improving their mentees’ perceptions of mentoring support. However, our results complement work from prior meta-analyses which found little support for capital as a predictor of mentorship quality (Kammeyer-Mueller and Judge, 2008; Eby et al., 2013). Rather, human capital may be a better predictor of outcomes of mentorship, such as graduate students’ scholarly productivity or career advancement, rather than mentorship processes directly. Alternatively, capital may have a greater role during different stages of the mentoring relationship (e.g., initiation or termination). Given our relatively simplistic measure of mentor and mentee capital, subsequent research should examine other indicators of mentor human capital (e.g., mentor experience as faculty member or as a research advisor, mentor publication record normalized by discipline) to further explore the influence of mentor human capital on mentoring processes. Future research should also examine more fine-grained indicators of mentee capital, and how these indicators influence both the matching process and subsequent mentoring quality.
Finally, future research should more closely examine predictors of deep-level similarity given its outsized influence on mentorship quality. Our measure of deep-level similarity focused predominantly on mentee–mentor similarity of attitudes, perspectives, and outlook, which is limited. Future research could explore the effects of other forms of deep-level similarities, such as value congruence and similar approaches to interpersonal attachment, both of which have been associated with quality mentoring relationships in workplace settings (Mitchell et al., 2015; Illies and Reiter-Palmon, 2018). Longitudinal and quasiexperimental study designs may be productive for understanding when and how doctoral student mentees come to recognize deep-level similarity with their research advisor mentors, including whether perceptions of deep-level similarity fluctuate across stages of doctoral training. Longitudinal studies should also be fruitful for understanding the direction of the association between deep-level similarity and mentoring support and quality (i.e., potential for reverse causality) in a way that is not possible based on the cross-sectional design of the current study. For example, it is plausible that mentees who receive more mentoring support may be more likely to view their mentor as a role model and develop a greater sense of identification, which may result in a greater perception of similarity. Finally, research is needed to understand how mentors effectively engage in culturally aware mentoring and how culturally aware mentoring affects mentoring processes and outcomes. Such studies could provide empirical evidence regarding the sensitive and responsive ways mentors could approach learning about someone’s life experience and traditions and start conversations about race, ethnicity, and socioeconomic status with a mentee.
FOOTNOTES
1We purposefully use the term “racially minoritized” to reflect the active role that systemic and societal racism has had and continues to have on individuals who are American Indian or Alaskan Native, Black or African American, Hawaiian or Native Pacific Islander, Hispanic and/or Latine, and North African or Middle Eastern.
2We recognize that sex and gender are distinct terms and that the language used to capture these distinctions continues to evolve. However, much of the prior research has conflated these terms. Given our interest in the ways that gender (i.e., an individual’s lived experience identifying as a woman, man, non-binary, etc.) influences mentoring relationship dynamics, we balance the language used by previous studies with our own purposeful language.
3The rescaled fit statistic values derived from equivalence testing for the structural model indicated for CFIT: “poor” ≤ 0.867, “mediocre” = 0.867 – 0.889, “fair” = 0.889 – 0.919, “close” = 0.919 – 0.970, and “excellent” are ≥ 0.970 and for RMSEAT: “poor” ≥ 0.124, “mediocre” = 0.099 – 0.124, “fair” = 0.060 – 0.099, “close” = 0.015 – 0.060, and “excellent” are ≤ 0.015.
4We elected to include individuals from North African or Middle Eastern racial backgrounds in our measure of racially minoritized individuals, recognizing their unique racialized experiences that have long been shaped by racism, discrimination, and microaggressions (Atkin et al., 2022).
ACKNOWLEDGMENTS
We thank the members of the Social Psychology of Research Experiences & Education lab and the Biology Education Research Group at the University of Georgia (UGA) for their feedback that improved our work. This work was supported by a National Science Foundation Graduate Research Fellowship awarded to T.T.T. under grant DGE 1842396 and the Georgia Athletic Association Professorship in Innovative Science Education awarded to E.L.D.. Any opinions, findings, conclusions, or recommendations expressed in this material are those of the authors and do not necessarily reflect the views of any of the funding organizations.