Assessing the Life Science Knowledge of Students and Teachers Represented by the K–8 National Science Standards
Abstract
We report on the development of an item test bank and associated instruments based on the National Research Council (NRC) K–8 life sciences content standards. Utilizing hundreds of studies in the science education research literature on student misconceptions, we constructed 476 unique multiple-choice items that measure the degree to which test takers hold either a misconception or an accepted scientific view. Tested nationally with 30,594 students, following their study of life science, and their 353 teachers, these items reveal a range of interesting results, particularly student difficulties in mastering the NRC standards. Teachers also answered test items and demonstrated a high level of subject matter knowledge reflecting the standards of the grade level at which they teach, but exhibiting few misconceptions of their own. In addition, teachers predicted the difficulty of each item for their students and which of the wrong answers would be the most popular. Teachers were found to generally overestimate their own students’ performance and to have a high level of awareness of the particular misconceptions that their students hold on the K–4 standards, but a low level of awareness of misconceptions related to the 5–8 standards.
INTRODUCTION
Growing up, staying healthy, keeping pets, and tending plants are but a few life sciences topics that children find compelling. Although most people may not associate life sciences with their daily routine, negotiating the many obstacles of a normal day draws on a basic understanding of and literacy in life sciences. Yet there is far more to life sciences than basic human need and understanding. Groundbreaking discoveries that make life possible, in some cases, or make life more comfortable, in others, can provide motivation for learning fundamental life sciences principles. The future of healthcare, stewardship of flora and fauna, and the use of knowledge to create new organisms will lie in the hands of future voters who are now studying the fundamentals of the life sciences.
One national effort to characterize the knowledge required for a scientifically literate citizenry provides well-vetted listings of key fundamental concepts, the National Science Education Standards (NSES; National Research Council [NRC], 1996). This document forms the basis of curriculum and evaluation frameworks developed by all 50 U.S. states. The national standards include a substantial body of life sciences concepts at the primary and middle school levels, grouped as the K–4 and 5–8 grade bands in the NRC standards.
While the NRC standards are specific concerning the particular science content knowledge required for life sciences literacy, the NRC did not develop assessments that could measure this knowledge. It was left to each of the U.S. states to incorporate science tests into its assessment system based on state standards. Opinions vary as to the quality of these tests and whether they truly assess students’ conceptual understanding or simply measure factual knowledge (Ferrara and Duncan, 2011). This paper reports on the development and validation of a unique bank of test items designed to assess the conceptual understanding of each of the life sciences concepts incorporated into the K–8 NRC standards. We seek to understand the extent to which both students and their teachers have mastered these concepts and to offer a method of measuring gains in life sciences content knowledge at the pre–high school level.
Accomplishment of this task first required the creation of a set of items that, as a whole, represent the entire body of the K–8 national standards relating to life sciences content. These items could then be compiled into tests for administration to a nationally representative sample of classrooms in which both teachers and students could be included. Comparisons could then be made between the degrees of mastery of different standards in different grade bands.
The assessment of science content knowledge is a complex issue, and educators vary in their opinions about the best way, out of many assessment options, to measure student understanding. Tools such as portfolios (Slater, 1997), clinical interviews (Duckworth, 1987), authentic assessment (Kamen, 1998), and concept mapping (Novak, 1998) have grown out of the research on cognitive models of learning, all of them attempting to characterize the path of an individual's conceptual change (Mintzes et al., 2005). Yet, due to the manner in which they are formulated, these more qualitative methods are often much more expensive and cumbersome to administer and score than more traditional, standardized, multiple-choice assessments.1 Over the past 25 yr, a different type of assessment instrument has been developed based on qualitative cognitive research, yet still quantitative in format, the distractor-driven multiple-choice (DDMC) test (Sadler, 1998; Briggs et al., 2006; de la Torre, 2009). Research into the nature and potency of these DDMC tests has shown great effectiveness in assessing the conceptual understanding of students (Halloun and Hestenes, 1985; Hufnagel, 2002).
BACKGROUND
All 50 states have implemented science standards (or frameworks) based on the NRC's NSES (NRC, 1996) (and the related American Association for the Advancement of Science Benchmarks [AAAS, 2001]). They are currently awaiting the Next Generation Science Standards (NGSS) (Achieve, 2013) based on the new NRC Framework for K–12 Science Education (NRC, 2012), and most states plan to incorporate relevant changes into their state science assessments. States (and groups of states) generally “outsource” the development of tests to for-profit companies that rarely incorporate any recent advances in test design (Chingos, 2012). Yet over the past 25 yr, science education researchers have refined a type of assessment instrument that takes full advantage of the research literature on how people learn scientific concepts, the DDMC tests (Sadler, 1998; Pellegrino et al., 2001; Herrmann-Abell and DeBoer, 2011). Although such tests can appear to be similar to conventional multiple-choice tests, they differ in several important ways that make them much more useful for diagnostic purposes to help in planning instruction for students or professional development of teachers and for assessing progress toward conceptual understanding.
Cognitive research on children's alternative ideas is generally accepted to have begun with Piaget's traditional structured clinical interview (Piaget and Inhelder, 1967), which demonstrated that children construct their own understandings based on their interactions with the world (Turkle, 2008). Their ideas can be quite different from those held by adults (Driver and Easley, 1978).2Prather (1985) identified a need for reliable diagnostic tests that could identify and classify students’ conceptions and would be of great utility for educators. Initially, open-ended, written tests were developed as a way to gather data from a greater number of subjects than is feasible through interviews. The first major success in incorporating student misconceptions into a multiple-choice format was the Force Concept Inventory in physics (Halloun and Hestenes, 1985). Such DDMC tests force a choice between a single correct answer and one or more misconceptions identified by researchers (Freyberg and Osborne, 1985).3 Such test items can only be constructed if the misconceptions that students hold have been previously identified, a definite shortcoming (Finley, 1986). However, well-crafted DDMC tests accurately and quickly ascertain the conceptual understandings (or misunderstandings) of students, as well as of teachers (Gilbert, 1977; Halloun and Hestenes, 1985).
Recent research has explored how DDMC tests behave psychometrically.4 Well-constructed tests help science teachers gain a window into students’ thinking. In addition, DDMC tests have the capability of identifying examples of student misconceptions, as well as determining how common they are within a population. Misconceptions, while they can change through instruction, appear to be quite stable in populations over long periods of time and appear to be similar across different cultures.5
To those unaware that certain misconceptions are irresistibly attractive to learners, we offer an example (Figure 1). DDMC item answer choices look quite conventional, but the statistical performance of these items is very different than conventional items, because a particular wrong answer is chosen by most of those students who incorrectly answer the item. Such a popular wrong answer, or distractor, is rarely found in a standardized test item. Psychometricians generally reject items with certain statistical profiles, particularly those for which moderately scoring students prefer a particular wrong answer with greater frequency than their lower-performing classmates (Sadler, 1998). Including popular misconceptions makes DDMC test items more difficult, and test developers advise against the use of distractors, which tend to trap even very knowledgeable students (Nunnally, 1964).
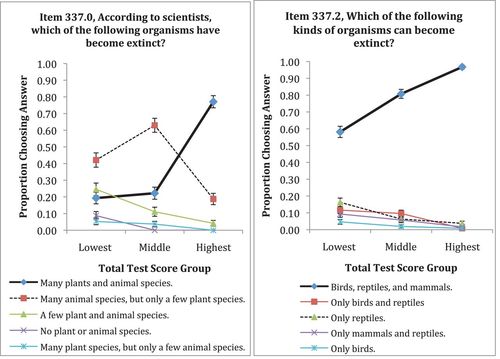
Figure 1. Similar Items from the MOSART Life Science Middle School Pilot Test. The proportion of students choosing each answer is shown for the lowest, middle, and highest third of students by total test score. Students can perform at artificially high levels on multiple-choice items if popular misconceptions are not included as choices. The correct choice is listed first for both items. The wrong answers are in descending order of popularity. Item 337.0 shows a strong distractor (n = 132), while item 337.2 does not (n = 218). Error bars: ± 1 SE.
Our belief is that this performance pattern is the point of a good test item, that is, it distinguishes between a student's preconceived ideas and those accepted by scientists. If the most prominent misconceptions are not included as distractors in test items, students may choose the correct answer via a process of elimination from a sea of weak distractors. Such items do little to inform teachers of their students’ initial ideas when given as part of a pretest prior to instruction. More importantly, these items do not adequately measure the degree to which students have fully accepted the scientific concept when given on a posttest, because students are not influenced by their misconceptions. When not confronted with an item offering a choice that clearly reflects their own thinking about a concept, students will often simply pick an answer that is similar to what a teacher said in class, for example, by recalling key words or vocabulary. Thus, if popular misconceptions are not included as answer choices in test items, educators can easily be misled into believing that students have mastered a particular concept, because students have chosen the correct answer. Such answer selections do not reflect the transformation that is the hallmark of conceptual change. In our opinion, multiple-choice items that do not employ distractors based on known misconceptions are of questionable utility for measuring conceptual understanding.
Because the misconceptions that students hold conflict with scientific conceptions, many researchers recommend that teachers be aware of their students’ ideas (Ausubel et al., 1978; Novick and Nussbaum, 1978; Nussbaum and Novick, 1982; Langford, 1989; Carlsen, 1999; Loughran et al., 2006; Sadler et al., 2013). Knowledge of student misconceptions is included as a particular component of Shulman's (1986) construct of pedagogical content knowledge (PCK) as “the conceptions and preconceptions that students of different ages and backgrounds bring with them to the learning of those most frequently taught topics and lessons. If those preconceptions are misconceptions, which they so often are, teachers need knowledge of the strategies most likely to be fruitful in reorganizing the understanding of learners, because those learners are unlikely to appear before them as blank slates” (pp. 9–10). Such a view recognizes that learning science is as much about unlearning old ideas as it is about learning new ones.
Duckworth (1987) advocates that teachers develop clinical interviewing skills to tease out student ideas individually or in small groups. Such action is recommended to help clarify teachers’ own ideas and lead to an appreciation of “children's science” (Osborne et al., 1983). Our team has attempted to develop this recommended facility in several professional development institutes with a view toward increasing teachers’ awareness of student ideas. Yet our experience is that most teachers experience great difficulty developing expertise in this procedure.6 Many teachers have requested that the results of interviews carried out systematically by researchers be aggregated by curricular topic so they can be referenced easily prior to instruction. Following Treagust's (1986) recommendation of the development and administration in classrooms of multiple-choice tests that reveal student conceptions, this paper helps to characterize the prevalence and popularity of particular misconceptions quantitatively, so teachers can structure lessons to deal with particular conceptual difficulties in K–8 life sciences. It should be noted that our study focuses on developing assessment instruments that can effectively measure the presence of misconceptions. The study did not examine how a teacher should address any particular misconception, or whether all misconceptions should be treated equally by teachers.
Teachers’ subject matter knowledge (SMK) is defined as having completed the course work and possessing a general conceptual understanding of a subject area (Shulman, 1986). SMK includes knowing how to organize scientifically accurate information to make it coherent to novice learners. Schwab (1978) calls this process substantive and syntactic structuring, and insists that simply knowing what is true scientifically is insufficient for effective teaching. In this study, we explore how teachers perform on the same tests that are given to their students. The testing of both students and their teachers similarly has only been found a few times in the research literature, and only in the field of precollege mathematics (Harbison and Hanushek, 1992; Mullens et al., 1996). Analysis of results at the content standard and the item level identifies any weakness in teacher knowledge, as well as any misconceptions that might be present.
METHODS
Much work has been done on the development of assessment instruments for college-level life sciences. Other than the Biology Concept Inventory (Klymkowsky et al., 2003; Garvin-Doxas et al., 2007; Garvin-Doxas and Klymkowsky, 2008), most of these concept inventories are directed to a specific field in life sciences rather than to a general assessment. The Conceptual Inventory of Natural Selection (Anderson et al., 2002; Anderson, 2003), the Genetics Concept Assessment (Smith et al., 2008), the Introductory Molecular and Cell Biology Assessment (Shi et al., 2010), animal development (Knight and Wood, 2005), and Host-Pathogen Interactions (Marbach-Ad et al., 2009, 2010) are some of the well-researched and topic-specific assessments currently available for the college level. The Project 2061 Science Assessment Project focused on a small number of key ideas at the middle school level and developed a large number of items that include common misconceptions as distractors (DeBoer et al., 2008).7
Our item inventory differs from these efforts in that it deals with all relevant concepts at the K–4 and 5–8 grade levels as defined by the NRC content standards. Our instruments were developed to serve several purposes:
To establish the levels of student understanding and the prevalence of particular misconceptions, both prior to and after instruction in relevant science courses.
To measure conceptual change (using pre test/posttest administration) in precollege students as a result of instruction.
To gauge teacher mastery of the concepts they teach.
To measure conceptual change in teachers as a result of gaining experience over time or as the result of professional development activities.
To examine teachers’ understanding of students’ conceptions (by predicting item difficulty and common incorrect responses made by students).
Building on the foundation of our prior work in developing assessments in physical science, earth science, and astronomy (Sadler et al., 2009), we embarked on item development for the grades K–8 life sciences content standards. We knew that to produce a Life Science Concept Inventory (LSCI) of tests for two grade bands (K–4 and 5–8), with each final test form containing 25–35 items, a test-item bank many times that size first had to be created. Only through extensive field testing could the psychometric properties (primarily difficulty and discrimination) of each item be established. Items that performed well and exhibited a range of difficulty could then be combined and tested together in order to comprehensively measure the broad range of concepts at each grade level.
Our development team followed a detailed process that involved seven key steps to build our test item bank (see Figure 2).
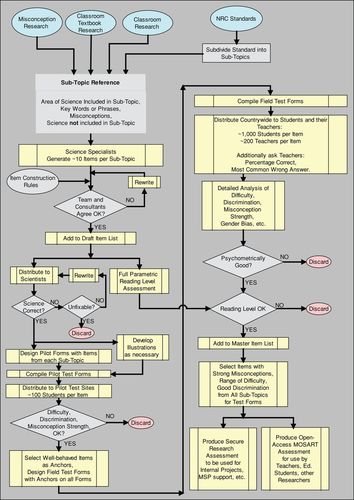
Figure 2. Flowchart of the inventory development process. A decade of item and instrument development has resulted in a complex but efficient process.
Review and Cataloging of Relevant Misconception Literature by NRC Standard
The research literature on student misconceptions is considerable. A team member searched each of several online databases (e.g., ERIC; Google Scholar; Duit, 2009) prior to a planned team meeting. Collections of learners’ and teachers’ misconceptions proved quite fruitful as we considered each NRC standard in turn (Driver et al., 1994). Both published and unpublished findings (e.g., dissertations) were reviewed. Often, the studies we found contained questions or probes used to reveal student ideas. These constructs could be used in drafting potential test items. It is important to consider that such studies are often qualitative in nature or utilize small, nonrepresentative samples of students. By drawing from the wealth of research literature, we identified a very large set of possible misconceptions that may be present in the student population, which helped us to ascertain whether such conceptions are common in a representative U.S. sample.
Standards Interpretation and Draft Item Construction
In the NSES, each major content standard contains a bulleted list of concise statements that rely on associated explanatory and illustrative materials and a general understanding of the scientific field. The goal for our team was the production of multiple-choice items that addressed the scientific concept(s) embedded in each of these concise statements, which we refer to in this article as subtopics,8 in such a manner that each item was:
scientifically accurate in both its stem and correct choice;
framed in a context used in U.S. schools to provide a familiar reference for students (e.g., it did not use an outlandish scenario);
grade-band-appropriate in the probing of a concept (e.g., it did not invoke concepts or terminology used in higher grade bands);
written at the appropriate grade-band reading level, so the item did not perform as a reading ability probe;
linked to a misconception found in the research literature, including at least one distractor that was directly based on a misconception; and
worded in such a way that any known misconception-related words were not used in an item stem if such use could bias the test taker toward the misconception-based distractor (misconception-related language was confined to the item's options).
Because the ultimate goal of our items was the construction of multiple-choice tests to be administered to students and teachers across a wide range of settings, our interpretation of the subtopics did not focus on any one curriculum or any particular set of experiments or pedagogical methods. Therefore, the process we used to interpret the standards differs somewhat from interpretation processes used for multiple goals, such as the one used by Krajcik et al., (2008). Considerable effort was expended to unpack each standard into the relevant subtopic and to generate a common understanding among the development team of the intended science content that it contained. Examples from the teaching experience of the group members, examination of popular texts and curricula, and released items from state tests helped form a consensus of the appropriate level and meaning of the NRC standard under discussion. We did not exclude any standard as too difficult for the intended grade band, but focused on how students in this age group might demonstrate mastery of a particular concept by recognizing a scientific statement from a set of nonscientific choices or by making an accurate prediction of an experimental outcome rather than one that might be more attractive to those holding a particular misconception. It should be noted that we developed the items only to probe for content knowledge and the presence of misconceptions, and all development work occurred prior to the publication of the NGSS, so our items do not intertwine content and practice, a core emphasis of the new standards.
One result of our broad focus was that we did not directly involve classroom teachers in the interpretation of the standards or in the review of the initial draft items. However, we did obtain information about the appropriateness of certain item features from teachers both before item development and during our pilot and field-testing steps. We recruited teachers nationwide to administer our pilot and field tests, a process that began before item development. As teachers were contacted, a team member asked (by email or phone) about the life sciences content taught by the teacher, the curriculum and other learning materials used by the teacher, and the teacher's general views concerning the NRC standards as compared with the teacher's own course content. In addition, we examined textbooks and other materials referenced by the teachers to gain insight into the various issues mentioned in the preceding list that kept us focused on our primary goal. During the pilot and field-testing steps, teachers were instructed to mark “0%” on items related to concepts they did not teach, providing an additional check on matching our items to what is most commonly taught in U.S. schools. Finally, the teacher version of our tests provided space for writing open-response comments about our items, and from these comments we obtained additional feedback on the NRC standards and our items; nearly all teachers at both the K–4 and 5–8 levels considered the entire content of our pilot and field tests to be fair, matching well to the content they taught. Because we recruited teachers to create a nationwide sample of classrooms that matched as closely as possible the actual demographics of U.S. schools, we felt that the total information received from the recruited teachers reasonably described life sciences content in U.S. schools as a whole.
The members of the six-person development team had a wide range of relevant expertise. Of the six team members, two were university biologists and one a psychometrician; three had been precollege biology/life sciences teachers; two conduct research on children's learning; two have extensive experience with K–12 science curriculum development; one is actively involved with in-service programs for life sciences teachers; one had managed all of our prior assessment development projects; and one has experience as a technical writer of science education materials.
The team met weekly for 2 h, with two consecutive meetings used to develop items for one subtopic. In the first of a pair of meetings, the relevant literature was presented, as well as other information needed to keep us focused on our goal. Out of this meeting came a joint understanding of the meaning of the subtopic and a summary of its component concepts and issues, which was compiled and disseminated by the project manager to all team members.9 The following week, the team met again, with the team's three content experts having written preliminary items (20–40 total items on average per subtopic) that incorporated misconceptions into the distractors. The entire team discussed each item to determine whether it: fit the subtopic; contained a plausible distractor drawn from the research literature; had a reasonable context and readability; and contained a scientifically correct answer. As a result of this discussion, many of these preliminary items were revised or combined, while others were discarded. In all, this process generally halved the number of items to 10–15. The project manager compiled clean copies of all improved items as initial draft items. These draft items were then sent to the team member responsible for coordinating the review efforts of external scientists (only this team member knew the identity of the external reviewers in terms of comments received about the items so that the reviewers could feel free to express their thoughts openly). The process continued in this manner, with some meetings eventually set aside to discuss comments returned by the scientists; these discussions would generate additional iterations of item review and editing. The detailed unpacking and item review process is shown in Figure 3.
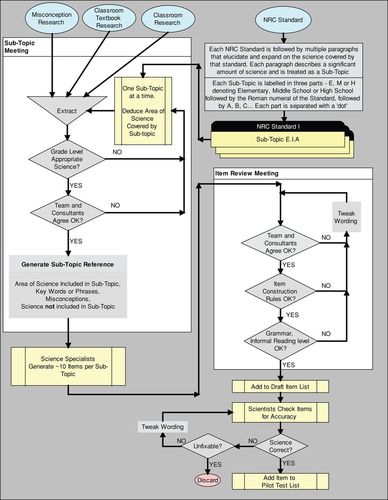
Figure 3. Flowchart of subtopic “unpacking” and item review.
Expert Review and Validation
The project recruited biologists nationwide from universities, national laboratories, and industry with an eye to ensuring the accuracy, clarity, and relevance of test items. Items from particular subtopics were sent to those considered experts in the related fields. For example, items concerning disease were examined by a high-ranking field scientist at the Centers for Disease Control; items concerning genetics were reviewed by a department head of cytogenetics at an internationally recognized teaching hospital; items concerning plants were sent to a field botanist from the Smithsonian Institution heading up a research team in the Amazon rain forest; items involving reproduction were vetted by a department head of obstetrics and gynecology at a major teaching hospital; and items involving climate change were sent to a group of climatologists who were members of an international team conducting research on atmospheric gases, among others. Several of our reviewers, who were also instrumental in developing the national standards and benchmarks, reviewed every item in both the K–4 and 5–8 inventories for science content and appropriateness relative to student learning and comprehension.
The vetting process involved sending to these science content experts the wording of the relevant standard along with a set of draft items. The scientists selected what they thought to be the correct answer and often suggested changes in wording. Some even rewrote entire items. The development team was surprised by objections to inclusion of some distractors drawn from findings in the research literature, receiving comments such as “surely no one thinks this,” demonstrating clearly that one can be a subject matter expert, but still be quite unaware of the misconceptions that people hold. Scientists’ comments were then discussed at a later team meeting and the relevant items were modified, with careful attention paid to keeping the reading level at the appropriate grade-band level. These revised items were sent out anew until all reviewing scientists agreed on the correct answer and were satisfied with the format. A scientific illustrator then produced accompanying drawings for items that required them. The items were then analyzed by a reading-level expert to make sure that none was at a level above that of the intended student grade band. In all, the K–4 standards work generated 213 different items and the 5–8 standards effort produced 484 items.
Pilot Testing
Once items for all standards had proceeded through the first three steps, pilot tests (10 forms for grades K–4, 20 for grades 5–8) were constructed such that each contained a subset of items with the purpose of getting a basic idea of the characteristics for each item. Three measurements were used to describe an item: difficulty (i.e., fraction of students answering the question correctly), discrimination (an item's correlation with the overall score on the pilot test), and misconception strength (i.e., of the students choosing the wrong answer, the fraction choosing the same incorrect option). Of these measures, the most important for the project at this stage was item discrimination because we wished to identify anchor, or core, items that could be included on all later field-test forms for each grade band. Anchor items allow for comparison of the overall performance levels of students on all test forms and can be used to standardize student performance if there is significant variation among the classrooms taking the tests. These pilot tests were constructed using items selected from across the relevant grade band to represent each standard. Each K–4 pilot test contained 23 content items, while the 5–8 tests had 26 items each; based on our earlier work, these were judged to be of appropriate length to permit completion by students within an average science class period.10 Classrooms were recruited from around the country to secure teachers (K–4: 35; 5–8: 40) and their students (K–4: 2136; 5–8: 3296), drawing on a large cadre of teachers who volunteered for this project in response to a nationwide mailing.
The K–4 pilot tests were administered to students beginning grade 5 (these fifth graders served as proxies for K–4 students11). Students in seventh- and eighth-grade classrooms completing a life sciences course were utilized for the 5–8 pilot tests. Forms were mailed and administered by the teachers, and the returned answer sheets were logged and scanned. On average, 265 (SD = 128) students answered each K–4 pilot test item. The average number of students answering each item for the 5–8 pilot tests was 189 (SD = 113). Item difficulty ranged from 0.02 to 1.00 for the K–4 items and 0.16 to 0.99 for the 5–8 items. However, these parameters do not take into account the overall knowledge level of the students, which it was only possible to establish later in the field tests that included the anchor items taken by all students within a grade band. Discrimination should be far more stable across ability and thus could be calculated using the pilot test data; it ranged from −0.06 to 0.60 for the K–4 items and −0.42 to 0.84 for the 5–8 items. Misconception strength was high (i.e., ≥ 0.50) for 52% of the K–4 items and 38% of the 5–8 items, indicating that we had been effective in identifying many distractors that were highly attractive to our target students.
Six items from the 10 K–4 pilot tests were selected for use as anchor items on all K–4 field-test forms. The six-anchor item set was selected primarily on the basis of three criteria in that they: 1) represented different standards, 2) had greater discrimination than other items related to the standard, and 3) included a distractor that was very popular, as evidence of a misconception. The anchors varied in initial difficulty and had high discrimination. In addition to the six items that appeared on all field-test forms, four additional items were chosen to appear on half of the field-test forms to represent the remaining standards. In much the same way, six anchor items were selected from the 5–8 pilot tests for the relevant field tests. In addition, two more items representing other standards were included on half of the field-test forms and two other items were included on the other half. As an example, one of the items chosen as an anchor for the middle school field test was item 337.0 (see Figure 1). With a difficulty of 0.37, it is relatively hard, but it shows a moderate discrimination of 0.37 and a misconception strength of 0.59. In comparison, item 337.2 is relatively easy, with a difficulty of 0.53 and a misconception strength of 0.33 indicating no strong misconception.
Field Testing
Teachers were recruited from a national mailing sent to elementary and middle school teachers. Each field-test instrument was measured for reliability (i.e., internal consistency) and validity (e.g., expert assessment of an item's scientific accuracy, its match to the NRC standards, and alignment with other test instruments).
The K–4 tests were given to fifth-grade students during the Fall of 2009, as near to the end of their K–4 experience as was feasible. Therefore, it can be assumed that the results measure student understanding after 5 years of study of the K–4 concepts. Teachers were also requested to select the correct answer for each test item as a way to determine their level of understanding of the concepts underlying the NRC standards. In addition, teachers were asked to predict the percentage of the students in their class who would answer each test item correctly; this was an attempt to measure the familiarity of teachers with their own students’ knowledge levels (Lightman and Sadler, 1993). A summary of the 15 field tests appears in Table 1. Student means ranged from 55 to 72% correct. Teacher means ranged from 89 to 97% correct. KR20, a measure of internal consistency (i.e., a measure of how well correct answers on individual items correlate with the total test score), was reasonable for student tests. Measures of internal consistency reflect the degree of variance within a test form. Therefore, tests that yield high means and small SDs do not yield as high on reliability as do tests in which there is more variability.
Students | Teachers | ||||||||||
---|---|---|---|---|---|---|---|---|---|---|---|
Test | Items | n | Mean | SD | KR20 | Anchor | ∆ | n | Mean | SD | Core |
101 | 23 | 349 | 0.69 | 0.17 | 0.74 | 0.70 | 0.01 | 7 | 0.91 | 0.16 | 0.80 |
102 | 22 | 292 | 0.55 | 0.17 | 0.72 | 0.61 | −0.08 | 4 | 0.95 | 0.09 | 0.95 |
103 | 22 | 165 | 0.69 | 0.15 | 0.66 | 0.74 | 0.04 | 1 | 0.95 | nc | 0.95 |
104 | 24 | 218 | 0.63 | 0.14 | 0.61 | 0.71 | 0.02 | 4 | 0.95 | 0.02 | 0.95 |
105 | 21 | 263 | 0.69 | 0.17 | 0.71 | 0.67 | −0.02 | 5 | 0.94 | 0.04 | 0.94 |
106 | 22 | 368 | 0.63 | 0.18 | 0.75 | 0.66 | −0.03 | 6 | 0.97 | 0.02 | 0.97 |
107 | 22 | 462 | 0.63 | 0.15 | 0.66 | 0.68 | −0.01 | 6 | 0.89 | 0.06 | 0.89 |
108 | 20 | 233 | 0.65 | 0.17 | 0.68 | 0.70 | 0.01 | 5 | 0.95 | 0.06 | 0.95 |
109 | 22 | 162 | 0.60 | 0.13 | 0.59 | 0.70 | 0.01 | 2 | 0.89 | 0.03 | 0.89 |
110 | 20 | 327 | 0.65 | 0.17 | 0.69 | 0.73 | 0.04 | 5 | 0.96 | 0.02 | 0.80 |
111 | 20 | 207 | 0.67 | 0.16 | 0.69 | 0.71 | 0.02 | 4 | 0.95 | 0.04 | 0.95 |
112 | 19 | 224 | 0.62 | 0.17 | 0.69 | 0.66 | −0.03 | 3 | 0.96 | 0.06 | 0.96 |
113 | 21 | 272 | 0.70 | 0.15 | 0.69 | 0.72 | 0.03 | 5 | 0.95 | 0.06 | 0.95 |
114 | 20 | 304 | 0.62 | 0.20 | 0.77 | 0.66 | −0.03 | 7 | 0.93 | 0.06 | 0.93 |
115 | 23 | 379 | 0.72 | 0.14 | 0.70 | 0.73 | 0.04 | 9 | 0.97 | 0.04 | 0.97 |
Core | 6 | 4225 | 0.65 | 0.17 | 0.41 | 0.69 | 0.00 | 73 | 0.94 | 0.07 | 0.97 |
Students in grades 7–8 were tested in April–June of academic year 2009–2010, as near to the end of their life sciences course as was possible. Therefore, it can be assumed that the results measured student understanding after nearly two semesters of study of the relevant grade-band concepts. The middle school life sciences teachers completed their own versions of the test forms in the same manner as the fifth grade teachers. A summary of the 16 field tests appears in Table 2. Student means ranged from 50 to 73% correct. Teacher means ranged from 89 to 98% correct. KR20 was reasonable for the student tests.
Students | Teachers | ||||||||||
---|---|---|---|---|---|---|---|---|---|---|---|
Test | Items | n | Mean | SD | KR20 | Anchor | ∆ | n | Mean | SD | Core |
101 | 24 | 885 | 0.60 | 0.18 | 0.76 | 0.63 | 0.03 | 15 | 0.90 | 0.08 | 0.95 |
102 | 25 | 1416 | 0.62 | 0.22 | 0.86 | 0.58 | −0.02 | 12 | 0.95 | 0.04 | 0.98 |
103 | 25 | 905 | 0.60 | 0.22 | 0.85 | 0.62 | 0.01 | 16 | 0.94 | 0.08 | 0.96 |
104 | 25 | 1178 | 0.61 | 0.20 | 0.82 | 0.60 | 0.00 | 12 | 0.94 | 0.04 | 0.99 |
105 | 26 | 656 | 0.50 | 0.19 | 0.79 | 0.60 | 0.00 | 6 | 0.91 | 0.11 | 0.98 |
106 | 23 | 1222 | 0.68 | 0.22 | 0.85 | 0.58 | −0.02 | 8 | 0.92 | 0.08 | 0.89 |
107 | 25 | 1470 | 0.61 | 0.19 | 0.81 | 0.61 | 0.00 | 12 | 0.91 | 0.07 | 0.93 |
108 | 26 | 2174 | 0.62 | 0.20 | 0.83 | 0.58 | −0.02 | 13 | 0.96 | 0.06 | 0.93 |
109 | 26 | 1233 | 0.60 | 0.18 | 0.79 | 0.64 | 0.04 | 14 | 0.95 | 0.04 | 0.97 |
110 | 25 | 1473 | 0.59 | 0.19 | 0.81 | 0.57 | −0.03 | 14 | 0.93 | 0.07 | 0.97 |
111 | 23 | 1632 | 0.64 | 0.22 | 0.84 | 0.58 | −0.02 | 13 | 0.98 | 0.02 | 0.98 |
112 | 24 | 1150 | 0.68 | 0.20 | 0.83 | 0.63 | 0.02 | 12 | 0.95 | 0.05 | 0.99 |
113 | 25 | 1622 | 0.61 | 0.18 | 0.79 | 0.64 | 0.04 | 15 | 0.94 | 0.04 | 0.97 |
114 | 25 | 1544 | 0.64 | 0.20 | 0.82 | 0.59 | −0.01 | 24 | 0.94 | 0.06 | 0.95 |
115 | 25 | 1277 | 0.58 | 0.19 | 0.81 | 0.61 | 0.01 | 8 | 0.89 | 0.07 | 0.95 |
116 | 23 | 1100 | 0.73 | 0.21 | 0.85 | 0.60 | 0.00 | 11 | 0.98 | 0.04 | 0.97 |
Core | 7 | 20,937 | 0.60 | 0.23 | 0.55 | 0.60 | 0.00 | 205 | 0.96 | 0.08 | 0.96 |
Reliability of the Assessment
Reliability is the property of consistency in test scores. Generally, reliability includes internal consistency, stability (or test–retest), alternate forms, and interrater reliability. Internal consistency refers to the degree of consistency among items on one test form given once. The field-test forms show evidence of internal consistency. The field-test forms are moderately reliable, with Cronbach's alpha ranging from 0.45 to 0.85. We also compared the performance of the anchor items across all forms of the field test and found no significant differences among field-test forms. Finally, for the teachers participating in the professional development institutes, we collected pre–post data from 484 teachers and found the correlation of stability to be 0.65. On the basis of these statistics, we conclude that the assessment produced moderately reliable results.
We adhere to the theoretical definition of validity proposed by Messick (1989, 1995), that is, validity is the overall evaluative degree to which empirical evidence supports the adequacy of interpretations and actions/scores or other modes of assessment. Validity is not a property of a test, but rather of the scores and the interpretations of those scores. Specifically, Messick unified the various types of validity into one quality he termed “construct validity” and the sources of evidence that underlie the six aspects of validity that he defined. These aspects include: 1) content, 2) substantive, 3) structural, 4) generalizability, 5) external, and 6) consequential.
Evidence supporting the content aspect of construct validity includes the development of the items using the domain definition specified in the NRC standards. This practice permits the all-important content concepts in the domain and supports the representativeness of the instrument. The review of items by active research scientists provides additional evidence of the content aspect of validity. The readability analysis of items yields evidence of the items probing the specific domains of K–4 and 5–8 life sciences, rather than the construct-irrelevant characteristic of reading level. The reading-level characteristic was addressed through analyses of multiple readability criteria done by a recognized expert.
The evidence of the substantive aspect of validity is supported by the research into misconceptions and measurement theory. As discussed earlier, misconceptions were identified by empirical research studies, which were also examined methodologically. Methodological issues included the examination of the characteristics of the sample and the technical quality of data collection methods. Other substantive evidence employed modern psychometric approaches, using analytics such as item response theory (IRT). Specifically, the response options included likely misconceptions documented by research studies. In addition, we computed statistics to examine the relative appeal of various responses. We identify a strong misconception as a single distractor in an item that is chosen by 50% or more of test takers who answer the item incorrectly. Using a polytomous item response model, a subset of IRT, we derived substantive evidence that test takers who respond correctly on items are students who are on the high end of the performance continuum; test takers who respond with the less frequently chosen incorrect responses are on the low end of that continuum; and test takers who chose the strong misconception option are generally in the middle of the continuum (see Figure 4).

Figure 4. Nominal response model graph for a grade 5–8 item. The graph illustrates the response patterns for a typical item with one strong misconception, as well as three other distractors. The curve representing the correct response (B) shows a low probability of that option being chosen by test takers at the low end of overall test performance (negative theta) and a higher probability for test takers who overall were more knowledgeable (positive theta). The curve for the strong misconception (A) shows that its probability peaks for test takers with lower and middle knowledge levels (theta = −1 to 0) and decreases for test takers who are increasingly knowledgeable. The item used was: Present-day giraffes have long necks because: (A) they stretch them to reach the trees for food (33%). (B) their ancestors adapted to have long necks over time (47%). (C) giraffes with the longest necks are the strongest and most perfect (7%). (D) their neck length increases their body temperature (9%). (E) their neck length increases their speed (5%).
The generalizability aspect of validity examines the extent to which score properties and interpretations generalize across population groups, settings, and tasks. The evidence from our assessment offers the comparative analysis of the sample groups across a wide selection of students. The sample groups were selected based on properties such as U.S. states, type of community (urban, suburban, rural), and type of school (public, private, parochial). Student responses were remarkably similar on identical items.
We have little evidence of the two remaining aspects of Messick's construct validity. We did not collect data beyond the various forms of the field-test items. Because we did not share any specific item-level data with pilot and field-test teachers, we do not feel that the consequential aspect of validity is pertinent for participating students. For professional development, we did share information about the teachers’ responses, but the demographic data we collected remain confidential.
Messick's definition of construct validity emphasizes the inferences drawn from response data. A recent study by Cizek et al. (2008) stated that one of the criteria they used for the validity of published tests was adhering to unified validity. They also concluded that the majority of the reviewed tests cited four sources of validity evidence. Given the evidence presented here, our items meet these criteria.
Instrument Creation
The final result of the analyses for both the K–4 and 5–8 field tests was the creation of two sets of instruments (secure research and publicly released). Each secure final form includes items that represent the standards and, for the most part, items that range in difficulty for each standard. The items are well behaved psychometrically, with appropriate and positive discrimination. The forms for posting on our self-service (public) website were constructed from a second set of items selected to be as similar as possible to the items on the final secure versions. The items on the two test versions are parallel in content, difficulty, and discrimination insomuch as possible.
RESULTS
Item Characteristics and Student Performance
Characteristics of each item were calculated from large-scale validation test data in order to select the best combination of item quality and coverage of all standards for use on each final test instrument. As test items are most often described by two parameters, difficulty (fraction correct) and discrimination (correlation of individual item scores with subjects’ total test score), Figure 5 shows the distribution of these two parameters graphed by the two grade bands. When an item shows a positive and large discrimination, the students with the correct response, on average, scored higher on the total test score. A negative or zero-order discrimination means that more students with low total scores answered the item correctly than did their higher-scoring peers. Items with the highest discrimination are typically answered correctly by 50–80% of the students. Many difficult items suffer rather low discrimination, but because the items cover all the standards, low discrimination items can be interpreted as representing concepts that are particularly difficult for students to master. Easy items also have low discrimination. The reason for this pattern is purely a function of the math. When difficulty is 0.50, discrimination can be as high as 1.0, which means everyone who answered the item correctly scored above the total test score mean and everyone who answered the item incorrectly scored below that mean.
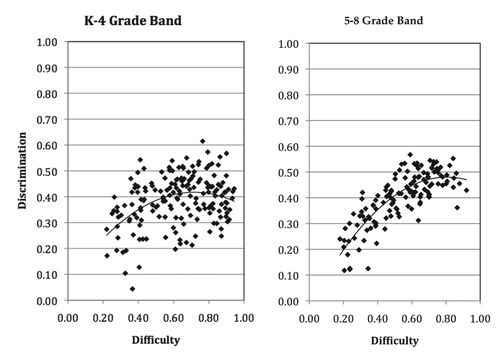
Figure 5. Item difficulty vs. discrimination for K–4 and 5–8 field-test items. Items range in difficulty and discrimination. Peak discrimination appears on relatively easy items, between 0.60 and 0.90 in difficulty.
Our team is particularly interested in a third statistic in addition to difficulty and discrimination: misconception strength. DDMC items are constructed to gauge the appeal of particular distractors representing misconceptions derived from the research literature. The popularity of these ideas—often investigated using only qualitative methods or small-scale studies—has only rarely been measured in large, nationally representative groups of students (Sadler, 1998). Through analysis of the allure of each item's distractors, it is possible to gauge their relative popularity. Figure 6 shows the proportion of students choosing the most popular wrong answer out of the total number of students choosing any wrong answer as a function of item difficulty.12 Of the 476 unique items in the K–8 LSCI, 283 have a single distractor (193 K–4 items and 90 5–8 items) that attracted more than half of the students who answered the item incorrectly.

Figure 6. Item difficulty vs. misconception strength for K–4 and 5–8 field-test items. The two grade-band item sets have nearly identical profiles, with a good spread of misconception strength, even among easy items. Half of all K–8 items have a misconception strength greater than 0.50.
Item Analysis and Integrating Teacher Knowledge
In addition to asking teachers to answer the same questions that we asked their students, we also asked them two additional queries per item. The first query asked the teachers to estimate the proportion of their students who would answer that item correctly. The second query asked the teachers to predict which incorrect option their students would choose most frequently. The responses to these queries were then compared with the actual most common incorrect response for each item.
Figure 7 shows the predictions of teachers compared with their students’ performances. Data points above the diagnonal dashed line indicate items for which students exceeded their teachers’ predicted performance. Data points below the diagonal line represent those items on which students performed less well than their teachers predicted. As can be seen from these two graphs, for the most part, teachers were overly optimistic in their predictions for items that were difficult (items above solid diagonal line). The reasons for this discrepancy may be related to the teachers’ own classroom assessments being less difficult than the most difficult Misconception Oriented Standards-based Assessment Resource for Teachers of Life Science (MOSART-LS) items. As discussed earlier, teacher-constructed test items may not include relevant and attractive distractors, which would result in a greater proportion of students choosing the correct answer.
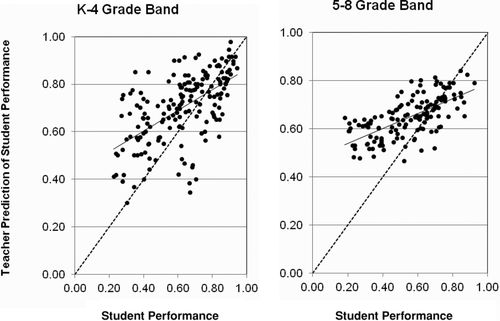
Figure 7. Teacher prediction of student performance vs. student performance by item for K–4 and 5–8 field tests. Each data point on each graph represents a single test item. The diagonal dashed line represents perfect agreement between teacher prediction of difficulty and actual student performance.
Many college-level educators and scientists worry that weaknesses exhibited by precollege students in science knowledge are the result of a lack of that knowledge by their science teachers. By comparing teacher performance with student performance on individual items, we can examine the relationship between teacher knowledge and student performance (after spending nearly a year in their teachers’ science classes in the case of the seventh- and eighth-grade students). Figure 8 plots teacher performance versus student performance by item. The cluster of items on the far right of each graph represents items for all teachers who answered the items correctly. For these items, the students of K–4 teachers ranged from 25% correct to 94% correct, averaging 64%. For 5–8 classrooms, students averaged 27% correct to 93% correct with an average of 60%. Hence, even when a teacher knows the answer to a particular item (indicating a certain measure of teacher knowledge), students do not attain mastery. Clearly, there are factors at work that impact student learning other than teacher knowledge, although the slope of the regression line shows that for items for which teachers perform poorly, students also do not perform as well.

Figure 8. Student performance vs. teacher performance on K–4 and 5–8 field tests by item. Each data point represents a single test item. A large fraction of items were answered correctly by all teachers. Best-fit line is plotted.
Relationship between Types of Teacher Knowledge
As can be seen from Figure 7, most teachers were able to select the correct response for the test items given to their students. Yet there were some items that were fairly difficult for the teachers. The relationship between that knowledge (SMK) and our particular measure of one aspect of PCK is more complex.
Figure 9 compares teacher SMK and PCK, with each data point representing an individual teacher. The clustering of data along the right-hand side of each graph is indicative of many teachers scoring 100% correct on the test form, with a large majority earning greater than 80% correct. Most teachers engaged in this study had high levels of SMK. The wide vertical spread of data shows that there is a wide range in ability of teachers to select the most common wrong answer chosen by students, our measure of PCK. K–4 teachers participating in this study demonstrated a greater familiarity with their own students’ ideas, identifying the most common wrong answer 74% of the time on items with strong misconceptions, versus only 45% for 5–8 teachers. Teacher SMK was far more comparable, with K–4 teachers having a mean of 92% and 5–8 teachers a mean of 94%.
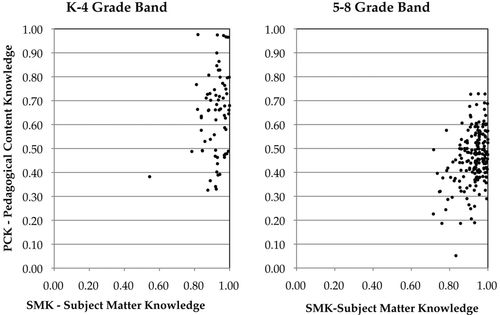
Figure 9. Teacher SMK vs. PCK of misconceptions. Data points represent individual teacher scores for items that have a strong misconception (i.e., misconception strength ≥ 0.50). SMK is measured as the fraction of items for which teachers selected the correct answer. PCK is the fraction of items for which teachers could identify the wrong answer most commonly chosen by their students.
K–4 Grade Band
Table 3 presents the results for student and teacher performance on the standards within the K–4 grade band. The number of items for each standard (last column) varies considerably, because they include only those items that passed the rigorous scrutiny and final approval of our expert reviewers. Many initial items were eliminated from use, because they were found to be unclear or dubiously accurate in their portrayal of the accepted scientific view, exhibited too high a reading level, or had structural problems. We also found that writing items for some standards was very difficult, yet easy for others, due to the science content of the standard, especially for some middle school standards. Teachers both selected what they thought to be the correct answer for each item on their students’ test and estimated the proportion of their own students who selected that answer. The mean scores, along with the SEs, are presented in Table 3 and Figure 10 for each of the standards examined.
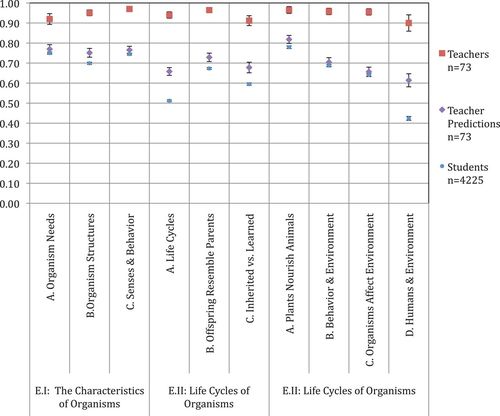
Figure 10. Student and teacher results for the K–4 grade band. Overall, students do not show mastery (at the 80% level) of any standard at their grade level. On average, teacher performance shows mastery, but with some gaps in knowledge. Teachers significantly overpredicted their students’ performance, as seen by most teacher predictions being greater than 2 SE above student performance. Error bars: ±1 SE.
Students | Teacher predictions | Teachers | ||||||
---|---|---|---|---|---|---|---|---|
Standard | Subtopic | Mean | SE | Mean | SE | Mean | SE | Number of items |
I. The Characteristics of Organisms | A. Organisms have basic needs. | 0.75 | 0.01 | 0.77 | 0.02 | 0.92 | 0.03 | 15 |
B. Plants and animals have different structures. | 0.70 | 0.01 | 0.75 | 0.02 | 0.95 | 0.01 | 22 | |
C. Behavior is influenced by cues. | 0.74 | 0.00 | 0.77 | 0.02 | 0.97 | 0.01 | 21 | |
II. Life Cycles of Organisms | A. Plants and animals have life cycles. | 0.51 | 0.00 | 0.66 | 0.02 | 0.94 | 0.02 | 26 |
B. Plants and animals resemble their parents. | 0.67 | 0.00 | 0.73 | 0.02 | 0.96 | 0.01 | 17 | |
C. Characteristics are inherited or learned. | 0.60 | 0.01 | 0.68 | 0.03 | 0.91 | 0.02 | 22 | |
III. Organisms and Their Environments | A. All animals depend on plants. | 0.78 | 0.01 | 0.82 | 0.02 | 0.96 | 0.02 | 9 |
B. Behavior is related to the environment. | 0.69 | 0.01 | 0.70 | 0.02 | 0.96 | 0.02 | 22 | |
C. All organisms cause environmental changes. | 0.64 | 0.01 | 0.66 | 0.02 | 0.96 | 0.02 | 19 | |
D. Humans need natural and artificial environments. | 0.42 | 0.01 | 0.61 | 0.03 | 0.90 | 0.04 | 5 |
Student mean score by standard fell into a range from 42% to 78% correct across the entire K–4 band. Students exhibited the greatest weaknesses in standards dealing with 1) humans and the environment and 2) life cycles. Teachers overpredicted their students’ performances on every standard, overestimating student mean scores by standard within a range from 0.01 to as high as 0.19. As a whole, teachers themselves did well on the test items across all K–4 standards.
The NRC standards at this level are concerned with the fundamental properties and needs of organisms, what they need to survive and to reproduce. On the whole, the standards focus on the plant and animal kingdoms, using as examples commonly known and physically large organisms, such as humans, bears, tigers, and trees. By understanding the most basic principles of how and why organisms survive, students begin to build a knowledge base on which future course work will be based. Human needs and behavior play a special role in life sciences at this level, because children can most easily relate to their own experiences and apply that understanding to how other organisms function.
5–8 Grade Band
Table 4 presents the results for student and teacher performance on the standards within the 5–8 grade band. The number of items for each standard (last column) varies considerably for the same reasons as for the K–4 item inventory. As in the K–4 item field testing, we had teachers both select what they thought to be the correct answer for each item on their students’ test and estimate the proportion of their own students who selected that answer. The mean scores along with the SEs are presented in Table 4 and Figure 11 for each of the standards examined.

Figure 11. Student and teacher results for the 5–8 grade band. Overall, students do not show mastery (at the 80% level) of any standard at their grade level. On average, teacher performance shows mastery, but with some gaps in knowledge. Teachers significantly overpredicted their students’ performance, as seen by most teacher predictions being greater than 2 SE above student performance. Error bars: ±1 SE.
Students | Teacher predictions | Teachers | ||||||
---|---|---|---|---|---|---|---|---|
Standard | Subtopic | Mean | SE | Mean | SE | Mean | SE | Number of items |
I. Structure and Function in Living Systems | A. Organisms have levels of organization. | 0.44 | 0.01 | 0.61 | 0.03 | 0.96 | 0.02 | 18 |
B. All organisms are composed of cells. | 0.49 | 0.01 | 0.66 | 0.03 | 0.93 | 0.03 | 21 | |
C. Cells perform many functions. | 0.67 | 0.00 | 0.67 | 0.02 | 0.97 | 0.01 | 25 | |
D. Specialized cells perform unique functions. | 0.51 | 0.00 | 0.62 | 0.02 | 0.92 | 0.02 | 25 | |
E. Humans have many different systems. | 0.53 | 0.01 | 0.63 | 0.02 | 0.85 | 0.04 | 18 | |
F. Disease is a breakdown in an organism. | 0.68 | 0.00 | 0.69 | 0.02 | 0.98 | 0.01 | 26 | |
II. Reproduction and Heredity | A. All organisms reproduce. | 0.54 | 0.01 | 0.69 | 0.03 | 0.95 | 0.02 | 26 |
B. Many species, including humans, reproduce sexually. | 0.73 | 0.00 | 0.74 | 0.01 | 0.98 | 0.01 | 26 | |
C. An organism passes instructions to a new generation. | 0.50 | 0.00 | 0.60 | 0.01 | 0.95 | 0.01 | 26 | |
D. Hereditary information is in genes. | 0.78 | 0.00 | 0.64 | 0.02 | 0.92 | 0.02 | 15 | |
E. Characteristics are inherited or come from environmental interactions. | 0.57 | 0.00 | 0.65 | 0.02 | 0.88 | 0.02 | 15 | |
III. Regulation and Behavior | A. Organisms must obtain and use resources to survive and reproduce. | 0.69 | 0.00 | 0.68 | 0.02 | 0.97 | 0.01 | 17 |
B. Homeostasis is key to organism survival. | 0.68 | 0.00 | 0.65 | 0.02 | 0.93 | 0.02 | 26 | |
C. Behavior is a response to a stimulus. | 0.66 | 0.00 | 0.67 | 0.02 | 0.97 | 0.01 | 17 | |
D. Behavior evolves through adaptation. | 0.44 | 0.00 | 0.59 | 0.02 | 0.91 | 0.02 | 22 | |
IV. Populations and Ecosystems | A. A population comprises all members of a species living together; populations plus environment form an ecosystem. | 0.54 | 0.00 | 0.63 | 0.01 | 0.92 | 0.01 | 27 |
B. Populations have functions in ecosystems. | 0.66 | 0.00 | 0.64 | 0.02 | 0.96 | 0.01 | 31 | |
C. Sunlight is the main energy source for ecosystems. | 0.49 | 0.00 | 0.65 | 0.02 | 0.90 | 0.02 | 25 | |
D. Populations are limited by various factors. | 0.78 | 0.00 | 0.69 | 0.01 | 0.96 | 0.01 | 20 | |
V. Diversity and Adaptations of Organisms | A. Millions of species are alive now and share a common ancestry. | 0.76 | 0.01 | 0.74 | 0.04 | 0.96 | 0.04 | 13 |
B. Species diversity arises from evolution. | 0.36 | 0.00 | 0.62 | 0.01 | 0.93 | 0.02 | 20 | |
C. Extinction has occurred and does occur. | 0.51 | 0.00 | 0.66 | 0.02 | 0.94 | 0.02 | 25 |
Student mean score by standard fell into a range from 36% to 78% correct across the entire 5–8 grade band. Teachers overpredicted their students’ performances for most standards, overestimating student mean scores by standard by as much as 0.26. But, interestingly, teachers underpredicted their students’ performances for six standards, one (“Heredity information is in the genes”) by 0.14. As a whole, teachers themselves did well on the test items across all of the 5–8 standards, with only a comparative weakness for the topic of human organ systems at 85% (but still above the proficiency level of 80%). Students appeared to be weakest in “Species diversity arises from evolution.”
The NRC standards at this level are concerned with building on the fundamental knowledge achieved in the K–4 grade band. More specificity is given on all topics, and in addition to observing large plants and animals, students are required to begin thinking about life in a more theoretical way, both micro- and macroscopically. The standards begin to probe the functions and structures of cells, as well as the “big picture” ideas of evolution and extinction, in order to prepare students for more in-depth studies in a variety of high school sciences, including ecology, environmental science, genetics, and biochemistry.
Comparison of the NRC Standards, Framework, and NGSS
We developed our items using as guidance the K–8 content standards in the NRC's NSES (NRC, 1996). Subsequently, the NRC published A Framework for K-12 Science Education (NRC, 2012), and, at the time of this writing, the NGSS13 were under final revision before formal release. To ensure that our assessments are relevant in light of these new guidelines, we examined the connections between the NRC standards and framework, and mapped each of the NRC standards (which are bulleted statements within the NSES content standards) to the relevant section of the NRC framework. We provide two concordances (Tables 5 and 6) to document the alignments that we found between the standards and framework. For both the K–4 and 5–8 content, we determined that all items we developed align with the life sciences content in the framework. As noted earlier, because our focus was on measuring conceptual understanding as guided by the NRC content standards, our items do not intertwine content and practice, a key emphasis of the NGSS.
Grades K–4 | Framework | Related NRC standards |
---|---|---|
LS1: From Molecules to Organisms: Structures and Processes | LS1.A: Structure and Function | E.I.A, E.I.B |
LS1.B: Growth and Development of Organisms | E.II.A | |
LS1.C: Organization for Matter and Energy Flow is Organisms | E.I.A, E.III.A | |
LS1.D: Information Processing | E.I.C | |
LS2: Ecosystems: Interactions, Energy, and Dynamics | LS2.A: Interdependent Relationships in Ecosystems | E.I.A, E.III.A, E.III.C |
LS2.B: Cycles of Matter and Energy Transfer in Ecosystems | E.III.A, E.III.B | |
LS2.C: Ecosystems Dynamics, Functioning, and Resilience | E.III.B, E.III.C | |
LS2.D: Social Interactions and Group Behavior | E.III.B | |
LS3: Heredity: Inheritance and Variation of Traits | LS3.A: Inheritance of Traits | E.II.B, E.II.C |
LS3.B: Variation of Traits | E.II.C | |
LS4: Biological Evolution: Unity and Diversity | LS4.A: Evidence of Common Ancestry and Diversity | E.III.B |
LS4.B: Natural Selection | E.II.C, E.III.B | |
LS4.C: Adaptation | E.I.A, E.III.C | |
LS4.D: Biodiversity and Humans | E.III.D |
Grades 5–8 | Framework | Related NRC standards |
---|---|---|
LS1: From Molecules to Organisms: Structures and Processes | LS1.A: Structure and Function | M.I.A, M.I.B, M.I.D, M.I.E, M.III.A |
LS1.B: Growth and Development of Organisms | M.II.A, M.II.E, M.III.C | |
LS1.C: Organization for Matter and Energy Flow is Organisms | M.I.C, M.IV.C | |
LS1.D: Information Processing | M.III.C | |
LS2: Ecosystems: Interactions, Energy, and Dynamics | LS2.A: Interdependent Relationships in Ecosystems | M.I.F, M.III.B, M.IV.A, M.IV.D |
LS2.B: Cycles of Matter and Energy Transfer in Ecosystems | M.IV.B | |
LS2.C: Ecosystems Dynamics, Functioning, and Resilience | M.IV.D | |
LS2.D: Social Interactions and Group Behavior | M.III.D | |
LS3: Heredity: Inheritance and Variation of Traits | LS3.A: Inheritance of Traits | M.II.C, M.II.D |
LS3.B: Variation of Traits | M.II.B | |
LS4: Biological Evolution: Unity and Diversity | LS4.A: Evidence of Common Ancestry and Diversity | M.III.B, M.V.A, M.V.C |
LS4.B: Natural Selection | M.V.B | |
LS4.C: Adaptation | M.V.A, M.V.B | |
LS4.D: Biodiversity and Humans | M.V.A |
DISCUSSION
The standards developed by the NRC (1996) represent the foundation for standards developed by each state. Several mentions are made in the NRC standards that students have particular misconceptions (e.g., “The student might have misconceptions about the role of sperm and eggs and about the sexual reproduction of flowering plants” [p.156]). However, no attention is paid to the degree of prevalence of such student ideas or how they can persist. Instead, the NRC standards tend to minimize the ease of changing such ideas, e.g., “Many misconceptions about the process of natural selection can be changed through instruction” (p. 184). There are no specific recommendations about how such instruction should be carried out to change student ideas or how to assess whether such ideas have, in fact, changed.
Our results, summarized in Figures 10 and 11, show the degree of mastery that students exhibit at the end of their exposure to K–4 life sciences instruction and at the end of a middle school life sciences course in grades 7 or 8. The results are not encouraging. Students do not reach levels of performance that educators might consider mastery. The teachers in this study estimated that their own students would perform at substantially higher levels than were actually measured. This leads us to believe that teachers either covered these concepts or assumed that students had already mastered the concepts.
Our development of test items that incorporate findings from the literature on student misconceptions produces a picture of student learning that departs from the classic “blank slate” version of learning science. As shown in Figure 1, using classical item analysis, and in Figure 4, using IRT, when a popular misconception is included as one of several distractors in a multiple-choice item, it can be even more appealing to midlevel students. Inadequate coverage of a particular concept may actually move students to find a misconception more palatable. If teachers use tests that do not specifically engage students’ prior ideas, students have a much better chance of answering an item correctly. However, this assessment approach will only give a false sense of security that students hold the scientific conception. Figure 1, in particular, shows that all students perform quite well on an item without a misconception distractor, but quite poorly on an item with such a distractor.
Teachers in our study did not exhibit much facility for predicting the difficulty of test items, that is, they could not predict with any accuracy how their own students would perform in answering multiple-choice questions that included misconceptions as distractors. Figure 7 shows that primary and middle school teachers overestimated student performance on difficult questions. However, teachers did quite well in answering the questions themselves. A high level of teacher knowledge (i.e., items for which teachers scores are 100%), as shown in Figure 8, did not guarantee high levels of student performance. Although teacher SMK is undoubtedly important, it does not appear to be the sole arbiter of student mastery. Instead, a teacher's ability to identify the wrong answer that is most attractive to their students, which we term PCK-M, may be more relevant. Based on our analyses, fifth-grade teachers appear to be more aware of their students’ misconceptions than seventh- and eighth-grade life sciences teachers.
These assessments can also be used as a pretest to plan professional development offerings to address gaps in teacher knowledge, because an emphasis on content knowledge is common in professional development efforts. Unlike studies that rely on teachers to subjectively report on the degree of increase in their own content knowledge to evaluate professional development programs (Garet et al., 2001), a pretest paired with a posttest (administered some time after the conclusion of the professional development program) can objectively gauge the efficacy of teacher institutes and workshops. One particularly useful application of these tests is for teachers to administer them to their students after instruction, but prior to the teachers engaging in professional development. In workshops that we have led, we have witnessed the tremendous impact on teachers when they learn that their own students still maintain certain misconceptions even after enthusiastic and engaging instruction. Providing such an experience to teachers can open spirited discussions of methodologies and motivate the study of more cognitively appropriate pedagogies and activities.
The authors have provided direct or indirect assessment support to 13 NSF Math and Science Partnership (MSP) programs and 40 U.S. Department of Education–funded MSP projects in the areas of physical, earth and space, and life sciences. In addition, we have carried out pre- and posttesting of 65 summer professional development institutes for middle school life sciences teachers under the Assessment of Life Science Intermediate School Educators (ALSISE) project supported by the National Institutes of Health (NIH). Work is underway to characterize the growth in teacher SMK and PCK in these professional development institutes.14
Teachers can use these tests to determine the strengths and weaknesses of their students at the start of a term. Examining the pretest performance of students can aid teachers in deciding on the appropriate activities to be used in their courses if they wish to positively impact conceptual understanding. An understanding of student misconceptions can also aid in determining which areas of students’ conceptual foundations require strengthening, particularly if more conceptually sophisticated content will be covered in a course.
We have endeavored to develop a test bank that has items for each of the national standards for both grade bands for K–8 life sciences. Each item was developed following an extensive review of the relevant research literature pertaining to misconceptions relating to the particular concept. Only a fraction of items were used to create assessment instruments. The potential to create new assessments that closely match the NGSS is a goal of the authors.
While it may be tempting for science teachers to think of misconceptions as something to “eradicate,” a more nuanced view should prevail. Teachers “should discuss and treat alternative conceptions not as errors, but as stepping stones to scientific understanding” (Sadler, 1998, p. 290). Having a misconception is evidence that a student is partway to an understanding a concept, in that he or she already has a way of thinking about some scientific issue. Driver and Easley (1978, p. 62) point out that misconceptions arise from the “alternative frameworks” that students generate by trying to explain events in the physical world. As such, although a misconception is produced by a student's model of the natural world, it is not the model itself. Like the tip of a buried ore deposit, there remains a lot below the surface that will be exposed only by exploration. As educators, we should seek to engage students in testing their ideas; misconceptions will only change when the underlying model changes due to a need for a more productive way of thinking about the world (Strike and Posner, 1992).
CONCLUSIONS
Our research has affirmed that many of the life sciences misconceptions discussed in the research literature are prevalent even after exposure to life sciences instruction in grades K–4 and 5–8. Our data gathering has the benefit of characterizing the relative popularity of misconceptions that teachers may encounter in the different grade bands, data that are only rarely found in the research literature. The frequency and strength of misconceptions revealed in our research, along with citations of relevant research literature for each, is included in the Supplemental Material accompanying this article. Such information is valuable, because teachers in our study generally overestimated the knowledge levels of their students, which may be due, at least in part, to an unfamiliarity with student misconceptions. Some teachers may possess misconceptions themselves about science content, although most teachers in our study scored well, providing evidence that they understand the science content they teach. We think that this overestimation of student performance reflects the fact that teachers often write their own tests and quizzes, which generally do not engage students’ misconceptions. Without the explicit inclusion of misconceptions—the personal ideas that students construct to explain how the world works—on teacher-constructed tests, students may often select the scientifically correct answer from the available options in a multiple-choice item. Open-ended items are little better. While students may seemingly be free to respond by drawing on their misconceptions, they rarely do so unless prompted to deal with common misconceptions. One telling example is a simplified food web item, an open-ended question asking for an explanation of how energy is transferred within a community. Students will often draw a food chain, one organism connected to another, knowing that the teacher will reward a basic energy transfer diagram. Instead, asking in what form energy is transferred, where it originates in a community, or how it circulates within the community (at a molecular level) will reveal whether or not students truly understand the nature of energy and the importance of homeostasis in a community (Brumby, 1982).
Teachers also have strengths and weaknesses, individually and as a group. When teachers have misconceptions, they are often the same ones that students hold. While these instances are somewhat rare, one should not assume that, as teachers learn concepts during their formal education, they will remember the difficulties they encountered or the ideas they previously held. Teachers are generally not very knowledgeable about the misconceptions of their students. It may be that teachers who do know their students’ misconceptions can construct learning activities that are far more effective than those teachers who assume that their students are simply blank slates ready to absorb a particularly cogent elucidation of a scientific conception.
Developed by a team of educators and scientists, each item in the K–8 LSCI was validated by several content experts for clarity and accuracy. Item reading levels are appropriate to each grade band. A selection of items were initially pilot-tested to select anchor items that appeared on all forms created for field testing. During field testing, a minimum of 500 students was used to collect data for each item tested. So that item parameters could be calculated, the middle school tests were administered in April–June of the appropriate courses, after most of the relevant course content had been covered, while the K–4 field tests were given at the beginning of the academic year for fifth graders to reflect learning through grade 4. At all levels, teachers performed relatively well, showing only a few consistent gaps in SMK at the primary and middle school levels. However, strong teacher performance on items did not go hand-in-hand with student mastery. Teachers typically overestimated their own students’ performances, especially on items that were more difficult than average.
By selecting items from all of the relevant NRC content standards and with a range of difficulty, final versions of the LSCI were created and validated at the appropriate grade levels. The development and validation of short-form assessments covering this broad range of standards makes these instruments useful in the evaluation of life sciences curricula and teaching practices by testing students, extending the possibilities beyond studies that examine learning a single concept.
Publicly available printable versions of these instruments are on our self-service assessment website, following the completion of a short tutorial on their use.15 Secure versions are available from the lead author for use in program evaluation. We also offer secure, online administration of these tests to teachers in professional development programs.16 A total of 30 NSF MSP programs—15 targeted, 11 comprehensive, and four institute—offer professional development in the life sciences. Our team has provided direct or indirect assessment support to 13 of these NSF MSPs. Nationally, MOSART tests have been used in the evaluation of 40 U.S. Department of Education–funded MSP projects.
Generating the LSCI required considerable effort on the part of staff and advisors, as well as the involvement of thousands of students and their teachers. Along the way, its creators learned much about test development. No doubt, our own understanding of SMK and PCK has been strengthened. Our hope is that the assessment tools created through this rigorous process will be of use to other educators, who will not have to face the daunting task of creating such instruments. Instead, other researchers and educators can avail themselves of the opportunity to use these tools to improve their own teaching, to measure the effectiveness of different teaching methods and materials, and to evaluate the efficacy of professional development activities for those who teach life sciences.
FOOTNOTES
1 For example, Vermont's statewide adoption of portfolio assessment was plagued by low interrater reliability (Koretz et al., 1994; Harlen, 2005), as well as political controversy (Mathews, 2004). Development of scoring rubrics and increased teacher training were augmented by use of outside authority to standardize teacher assessments and allow a fair comparison of scores. Even so, Vermont added a standardized test to increase reliability and lower the costs of assessing students (Vaishnav, 2000). As of the date of this article, Vermont was using only standardized tests for school accountability.
2 Contemporary attempts at identifying subjects’ alternative theories or scientific misconceptions in life sciences were first carried out in the domains of cosmography (Nussbaum and Novak, 1976), light (Guesne, 1978), and gravity (Gunstone and White, 1981).
3 Early efforts at developing multiple-choice tests around student misconceptions in astronomy included phases of the moon (Dai and Capie, 1990), cosmology (Lightman and Miller, 1989), cosmography (Nussbaum, 1979), gravity (Ogar, 1986), and compendia of concepts (Sadler, 1987; Schoon, 1988).
4 Gorin (2006) proposed that diagnostic tests based on cognitive models would be very helpful to teachers in identifying the reasoning that students use. Morris et al. (2006) evaluated the psychometric properties of DDMC items, and Ascalon et al. (2007) found that DDMC items are more difficult than open-ended items. Finally, Briggs et al. (2006) developed items for which each answer choice is linked to a developmental level of student understanding, facilitating the diagnostic interpretation of student item responses. DDMC items seek to provide greater diagnostic utility than typical multiple-choice items, while retaining their efficiency advantages.
5 An example in astronomy concerns notions of the shape of the earth. Nussbaum and Novak (1976) found that among second graders there is a popular notion that the earth is ball-shaped and we live inside on a flat area with air held inside by a hemispherical shell. Repeated studies of American children have found that this misconception has remained prevalent over the 25 yr since it was first discovered (Sneider and Pulos, 1983; Sneider and Ohadi, 1998). Similar views were later found across nations and cultures, including Nepal (Mali and Howe, 1979); Israel (Nussbaum, 1979); Greece (Vosniadou and Brewer, 1987); among Han and New Zealand European and Maori children (Bryce and Blown, 2006); and among Mexican-American children (Klein, 1982).
6 Most teachers find it difficult to not betray their own judgment of the correctness of a student's thinking in an interview situation either explicitly or through verbal or visual cues. When students think they are being judged, rather than having their ideas sincerely explored, they often withdraw into silence or respond with “I don't know.” Teachers love to teach, and students with “wrong” ideas often trigger a teaching response of wanting to correct a student's mistaken thinking.
8 For example, in K–4 NRC Standard I: The Characteristics of Organisms, there are three concise statements setting out the key concepts encompassed by the standard. Each of these concise statements was labeled by us for convenient team reference as a subsidiary standard or “substandard,” which we term a subtopic in this article. Because the NRC standards do not use any detailed labeling scheme, we created an alphanumeric subtopic reference system wherein, e.g., E.I.A refers to K–4 (Elementary) Standard I's first (A) concise statement. Such labeling allowed us to track items and sets of items throughout the entire development process.
9 Example of results of a subtopic discussion: 5–8 Life Science Standard IV: Populations and Ecosystems; Substandard C: “For ecosystems, the major source of energy is sunlight. Energy entering ecosystems as sunlight is transferred by producers into chemical energy through photosynthesis. That energy then passes from organism to organism in food webs.” See notes 1 and 2. Component concept 1: Sunlight is the major source of energy for an ecosystem. See notes 3 and 5–9. Component concept 2: Plants transfer solar energy into chemical energy via photosynthesis. See notes 10–12 Component concept 3: Chemical energy is dispersed throughout an ecosystem from organism to organism in food webs. See notes 4 and 13–15.
Notes
This subtopic expands on the concepts of different populations (e.g., producers and consumers) in ecosystems outlined in M.IV.B, q.v.
Ecosystems are not perceived by students as integrated entities, but rather as separate organisms within the ecosystem “doing their own thing.” Therefore, any reference to energy input to an ecosystem as a whole is not likely to be grasped by students.
Students (and many teachers) at the middle school level possess weak knowledge concerning the nature of energy and sunlight. The middle school physical science standard Transfer of Energy had the lowest student and teacher item performance of all grade 5–8 physical science standards.
Remember that atoms, molecules and chemical bonds are not referenced in the NRC middle school standards due to the wide range of misconceptions held by students. We did not use these terms in test items.
Misconception: Energy is a physical entity, possessing mass and weight.
Misconception: Energy is related to physical activity, especially motion. Motionless objects (such as plants) do not possess energy (or need it).
Misconception: Energy can be created and can vanish without a trace. There is no concept of conservation of energy.
Misconception: Light and heat are the same thing.
Misconception: Sunlight is an agent or reactant (a higher-level issue).
Misconception: Sunlight is a physical substance eaten by plants. Only the leaves of plants consume sunlight, as they have tiny mouths (the stomata).
Misconception: Chlorophyll flows through plants, carrying energy.
Misconception: Photosynthesis exists only so plants can make food for organisms that eat them; plants themselves obtain no benefit from photosynthesis—plant growth is related to plants eating soil or water or sunlight, not to photosynthesis.
Misconception: Food webs are only about eating, not energy transfer.
Misconception: Producers do not exist in deserts, in water, or in the polar regions.
Final point: The term “food” is often interpreted by students as anything that goes into the mouth of an organism. Therefore, use of “food” can create some confusion.
10 Although we first compiled a DDMC test for the evaluation of Project STAR, our work developing DDMC items and tests based on the national standards began in 2001 under National Science Foundation (NSF) grant REC-0087779, the Physical Science Assessment Project (PSAP). We chose to focus on the grade 5–8 standards, because we had recently completed work developing a middle school physical science supplementary curriculum. The result of this work is the 110-item Middle School Physical Science Inventory. The work described in this article was the fourth item-development project we undertook after the PSAP, and thus was heavily influenced by our past experience.
11 We used fifth graders as K–4 proxies, because reading ability is more disparate among younger children, making reliable administration of written tests difficult. We are currently investigating alternative assessment methods for large-scale studies of younger children, such as through use of pictures or video with narration.
12 Misconception strength equals .
13 The NRC framework, which is based on the standards and topics established in the National Science Education Standards (NSES), restructures the NSES content standards such that there are “cross-cutting concepts” across grade bands. Each grade band expands on the knowledge established in the prior band, while maintaining identical categories. The NGSS will be built directly upon the NRC framework and reorganize the cross-cutting concepts into more comprehensive manuals for both understanding and teaching. The “genealogy” of the NGSS thus lies on a clear line from the NSES through the NRC framework.
14 Teachers and MSPs can access free copies of our tests at www.cfa.harvard.edu/smgphp/mosart. This site was developed and is maintained with funds from the NSF.
15 www.cfa.harvard.edu/smgphp/mosart. This site was developed and is maintained with funds from the NSF for Projects MOSART and MOSART II.
16 Availability of the online tests is posted on the home page for Project MOSART II (NSF-0926272) on the NSF’s MSP website at www.mspnet.org.
ACKNOWLEDGMENTS
This work was carried out with support from the NSF's grant for MOSART-LS (NSF EHR-0830922) and from the NIH's grant for ALSISE (NIH 1RC1HD63686-01). We thank those scientists who reviewed and commented on the items in the development process. Annette Trenga handled data input and tracking of test forms. We appreciate the advice and support of Charles Alcock of the Harvard Smithsonian Center for Astrophysics. We greatly appreciate the involvement of teachers and their students in this project, without whom this research would have been impossible. This project was conducted with the approval of Harvard University's Committee on the Use of Human Subjects (protocol #F15916-101).