PORTAAL: A Classroom Observation Tool Assessing Evidence-Based Teaching Practices for Active Learning in Large Science, Technology, Engineering, and Mathematics Classes
Abstract
There is extensive evidence that active learning works better than a completely passive lecture. Despite this evidence, adoption of these evidence-based teaching practices remains low. In this paper, we offer one tool to help faculty members implement active learning. This tool identifies 21 readily implemented elements that have been shown to increase student outcomes related to achievement, logic development, or other relevant learning goals with college-age students. Thus, this tool both clarifies the research-supported elements of best practices for instructor implementation of active learning in the classroom setting and measures instructors’ alignment with these practices. We describe how we reviewed the discipline-based education research literature to identify best practices in active learning for adult learners in the classroom and used these results to develop an observation tool (Practical Observation Rubric To Assess Active Learning, or PORTAAL) that documents the extent to which instructors incorporate these practices into their classrooms. We then use PORTAAL to explore the classroom practices of 25 introductory biology instructors who employ some form of active learning. Overall, PORTAAL documents how well aligned classrooms are with research-supported best practices for active learning and provides specific feedback and guidance to instructors to allow them to identify what they do well and what could be improved.
Compared with traditional “passive” lecture, active-learning methods on average improve student achievement in college science, technology, engineering, and mathematics (STEM) courses (Freeman et al., 2014). Unfortunately, the quantity and quality of evidence supporting active-learning methods has not increased faculty and instructor adoption rates (Fraser et al., 2014). Thus, although the development of new and optimized classroom interventions continues to be important, many national agencies concerned with undergraduate education have broadened their efforts to include a call for the development of strategies that encourage the broader adoption of these research-based teaching methods at the college level (President’s Council of Advisors on Science and Technology, 2012; National Science Foundation [NSF], 2013).
Strategies developed to encourage faculty adoption of active-learning practices need to acknowledge the realities of faculty and instructor life and the many potential barriers to adoption identified in the literature. At the institutional level, these barriers include a reward system that can lead faculty members to devote less time and effort to teaching (Lee, 2000) and limited institutional effort to train graduate students or faculty members on teaching methods (Cole, 1982; Weimer, 1990). At the individual level, faculty members may not identify as teachers and therefore fail to put the same effort into their teaching as they do their research, may not recognize that the teaching strategies they use are not as effective as other strategies, or may not recognize that comfort and familiarity often dictate their choices of teaching method (Cole, 1982; Bouwma-Gearhart, 2012; Brownell and Tanner, 2012).
Even when faculty members are interested in learning new teaching practices, there remain multiple challenges to the effective implementation of those practices. First, there is a lack of clarity as to what active learning is, and this lack of clarity can lead to lack of fidelity of implementation of the teaching technique (O’Donnell, 2008; Turpen and Finkelstein, 2009; Borrego et al., 2013). For example, when asked to define active learning, faculty members in one study offered a range of answers, including simply “using clickers” or “group work” to “emphasizing higher-order skills” (Freeman et al., 2014). Many of these responses seem to conflate the tools used (clickers) to facilitate active learning with the actual methodology of active learning. In truth, both active-learning teaching methods and student learning are complex processes that do not have a single agreed-upon definition and can involve many components both inside and outside the classroom (Figure 1). This complexity, in and of itself, is a second barrier: changes in teaching practice can feel overwhelming because of the number of aspects that must be considered, and this can lead to paralysis and inaction (Kreber and Cranton, 2000). Finally, faculty members and instructors may not be familiar with the findings from the education research literature. With so many conflicting demands on their time, few faculty members have the time to immerse themselves in the education research literature. Furthermore, the education research literature frequently does not provide sufficient detail for the proper implementation of the educational innovations presented (Borrego et al., 2013).
Figure 1. PORTAAL captures one aspect of active learning: how the instructor structures the in-class experience. Active learning is a multifaceted practice that involves inputs from the instructor and students as well as events in and outside class. All these inputs influence the ultimate outcome of student learning.
We propose that the education research community interested in faculty change could better influence adoption of research-based best practices if we developed tools to efficiently communicate education research to instructors. Whatever these tools may be, they should include a clear description of the critical components of each teaching intervention such that a novice could implement them. Such an effort would provide faculty members with a clear set of instructions that would help them more readily implement teaching innovations with a higher degree of fidelity and, thus, possibly encourage the broader adoption of these research-based best practices for active learning. This paper is one attempt to create a tool that both clarifies the research-supported elements of best practices for instructor implementation of active learning in the classroom setting and helps instructors measure their alignment with these practices. This tool, Practical Observation Rubric To Assess Active Learning (PORTAAL), cannot address all the components of the complex learning environment (Figure 1) but can provide an accessible and informative entry point for implementing active learning in the classroom.
INTRODUCING PORTAAL: A PRACTICAL OBSERVATION RUBRIC TO ASSESS ACTIVE LEARNING
PORTAAL is intended to provide easy-to-implement, research-supported recommendations to STEM instructors trying to move from instructor-centered to more active learning–based instruction. For this paper, we operationally define active learning as any time students are actively working on problems or questions in class. We realize this is not an all-encompassing definition, but it is appropriate for the one area we are focusing on: behaviors in the classroom. Three goals guided the development of the tool:
Goal 1. PORTAAL is supported by literature: We identified dimensions of best practices from the education research literature for effective implementation of active learning. These practices are independent of particular active-learning methods (POGIL, case studies, etc.). | |||||
Goal 2. PORTAAL is easy to learn: We translated dimensions of best practices into elements that are observable and quantifiable in an active-learning classroom, making the tool quick and relatively easy to learn. | |||||
Goal 3. PORTAAL is validated and has high interrater reliability: The tool focuses on elements that do not require deep pedagogical or content expertise in a particular field to assess, making it possible for raters with a wide range of backgrounds to reliably use the tool. |
Although there are many classroom observation tools available (American Association for the Advancement of Science, 2013), none of these tools was explicitly designed to capture evidence of the implementation of research-supported critical elements for active learning in the classroom. Thus, in this paper, we will present our classroom observation tool, PORTAAL, and preliminary data demonstrating its effectiveness. Part 1 demonstrates how we used the three goals described above to develop our observation tool that is evidence-based, easy to use, and reliable. In Part 2, we demonstrate how we used PORTAAL to explore how active learning is being implemented in the classroom by documenting the teaching practices observed in a range of introductory biology classrooms at one university. Although PORTAAL was tested with biology courses, this instrument should work in any large STEM classroom, as the best practices are independent of content. Part 3 offers recommendations for how PORTAAL could be used by instructors, departments, and researchers to better align classroom teaching practices with research-based best practices for active learning.
PART 1: PORTAAL DEVELOPMENT AND TESTING
Goals 1 and 2: Identifying Research-Based Best Practices for Large Classrooms (Literature Review)
The validity of PORTAAL is based in the research literature. Each of the 21 elements is supported by at least one published peer-reviewed article that demonstrates its impact on a relevant student outcome.
Elements for inclusion in PORTAAL were gathered from articles and reviews published from 2008 to 2013 focused on classroom implementations or other relevant research on adult learning (i.e., lab studies) that documented changes in one of four types of outcomes: 1) improvement in student achievement on either formative (e.g., clicker questions, practice exams) or summative (e.g., exams) assessments, 2) improvement in student in-class conversations in terms of scientific argumentation and participation, 3) improvement in student self-reported learning when the survey used has been shown to predict student achievement, and 4) improvement of other measures related to student performance or logic development. Relevant other measures could include an increase in students’ self-reported belief that logic is important for learning biology or an increase in the number of students who participate in activities. These articles came from discipline-based education research (DBER) and cognitive, educational, and social psychology fields. Thus, the methods used to measure student outcomes vary widely. Even within a single outcome, the way the outcome was measured varied from study to study. For example, student achievement was measured in a range of ways: some studies measured student clicker question responses in a classroom, others looked at exam scores, and still others took measurements in psychology laboratory settings. The differences in how learning was measured could influence the magnitude of the results observed; however, for this tool, we accepted the authors’ assertions at face value that these were relevant proxies for learning and achievement. We do not claim that this literature represents all the published research-based best practices for implementing active learning in the classroom. Instead, these are the baseline recommendations, and, as more studies are published we will continue to modify PORTAAL to reflect the latest evidence-based teaching practices for active learning.
The majority of the articles reporting on active learning in the DBER literature involve whole-class transformations in which multiple features differ between the control and experimental classes. Although important, these studies were not useful for identifying specific features correlated with increases in student outcomes, because no one feature was tested in isolation. For example, an intervention by Freeman et al. (2011) increased the active learning in class by 1) adding volunteer and cold-call discussions, 2) providing reading quizzes before class, and 3) providing weekly practice exams. With all these changes, it was impossible to determine exactly which component led to the observed increase in academic achievement. Thus, articles of this type were not used in the development of PORTAAL. Instead, we focused on articles like the one by Smith et al. (2011) that explicitly test one element of the implementation of active learning (in this case, the role of peer discussions) to determine the impact of that single element on student outcomes.
Our survey of the research literature found that best practices for implementing active learning clustered along four dimensions: 1) practice, 2) logic development, 3) accountability, and 4) apprehension reduction. The first two dimensions deal with creating opportunities for practice and the skills this practice reinforces. The second pair addresses how to encourage all students to participate in that practice.
Dimension 1: Practice.
The first dimension in the PORTAAL rubric is a measure of the amount and quality of practice during class (Table 1). There are many articles validating the importance of opportunities to practice: student learning is positively correlated with the number of in-class clicker questions asked (Preszler et al., 2007); students who created their own explanations performed better on related exam questions than students who read expert explanations (Wood et al., 1994; Willoughby et al., 2000); and repeated practice testing is correlated with both increased learning (Dunlosky et al., 2013) and student metacognition (Thomas and McDaniel, 2007). Thus, best practice is to provide opportunities in class for students to practice (PORTAAL element practice 1, P1).
How element is observed in the classroom | Increases achievement | Improves conversations | Improves other measures | Citations | ||
---|---|---|---|---|---|---|
Dimension 1: Practice | ||||||
Elements | P1. Frequent practice | Minutes any student has the possibility of talking through content in class | ✓ | Wood et al., 1994; Willoughby et al., 2000; Preszler et al., 2007; Thomas and McDaniel, 2007; Dunlosky et al., 2013 (review) | ||
P2. Alignment of practice and assessment | In-class practice questions at same cognitive skills level as course assessments (requires access to exams) | ✓ | McDaniel et al., 1978; Morris et al., 1977; Thomas and McDaniel, 2007; Ericsson et al., 1993; Jensen et al., 2014; Wormald et al., 2009; Morgan et al., 2007 | |||
P3. Distributed practice | Percent of activities in which instructor reminds students to use prior knowledge | ✓ | deWinstanley et al., 2002; Dunlosky et al., 2013 | |||
P4. Immediate feedback | Percent of activities in which instructor hears student logic and has an opportunity to respond | ✓ | Renkl, 2002; Epstein et al., 2002; Ericsson et al., 1993; Trowbridge and Carson, 1932 |
In addition to the amount of practice, the quality and distribution of the practice is also important (Ericsson et al., 1993). For practice to increase achievement, the practice must be similar to the tasks students are expected to perform (transfer-appropriate principle; Morris et al., 1977; Ericsson et al., 1993; Thomas and McDaniel, 2007; Jensen et al., 2014). One method of measuring this alignment of practice and assessment is to determine how similar exam questions are to in-class questions. Jensen et al. (2014) provide a dramatic demonstration of the importance of this alignment: when students engage in higher-order skills in class but exams only test low-level skills, students fail to acquire higher-level skills. These results reinforce the concept that the test greatly influences what the students study. Additional studies have supported this finding that students learn what they are tested on (Morgan et al., 2007; Wormald et al., 2009). In PORTAAL, we use the cognitive domain of Bloom’s taxonomy to determine the alignment between in-class practice and exams (P2; cf. Crowe et al., 2008).
Finally, the temporal spacing of practice is important. Cognitive psychologists have shown that practice that is spaced out is more effective than massed practice on a topic (distributed practice; deWinstanley and Bjork, 2002; Dunlosky et al., 2013). These findings imply instructors should consider designing activities that revisit a past topic or ask students to relate the current topic to a past topic. Classroom observers could detect this distributed practice when instructors explicitly cue students to use their prior knowledge (P3).
Immediate feedback and correction also improves student performance (Trowbridge and Carson, 1932; Ericsson et al., 1993; Epstein et al., 2002; Renkl, 2002). In large lectures, this is primarily accomplished when students provide explanations for their answers to the instructor in front of the class as a whole (P4).
Dimension 2: Logic Development.
The second dimension in the PORTAAL rubric is a measure of the development of higher-order thinking skills (Table 2). Few articles validate the effect of this dimension on changes in achievement, because most exams test low-level questions (Momsen et al., 2010). However, there is extensive evidence documenting how this dimension increases the quality of student conversations or other relevant measurements, such as changes in goal orientation (i.e., students focusing more on understanding the material than on getting the correct answer).
How element is observed in classroom | Increases achievement | Improves conversations | Improves other measures | Citations | ||
---|---|---|---|---|---|---|
Dimension 2: Logic Development | ||||||
Elements | L1. Opportunities to practice higher-order skills in class | Percent of activities that require students to use higher-order cognitive skills | ✓ | Jensen et al., 2014; Ericsson et al., 1993; Morris et al., 1977 | ||
L2. Prompt student to explain/defend their answers | Percent of activities in which students are reminded to use logic | ✓1–3 | ✓4–6 | ✓ 6 | 1Willoughby et al., 2000; 2Schworm and Renkl, 2006; 3Wood et al., 1994; 4Knight et al., 2013; 5Scherr and Hammer, 2009; 6Turpen and Finkelstein, 2010 | |
L3. Allow students time to think before they discuss answers | Percent of activities in which students are explicitly given time to think alone before having to talk in groups or in front of class | ✓1 | ✓2 | 1Nielsen et al., 2012; 2Nicol and Boyle, 2003 | ||
L4. Students explain their answers to their peers | Percent of activities in which students work in small groups during student engagement | ✓1–6 | ✓7–11 | 1Smith et al., 2011; 2Sampson and Clark, 2009; 3Len, 2006–2007; 4Gadgil and Nokes-Malach, 2012; 5Wood et al., 1994; 6Wood et al., 1999; 7Menekse et al., 2013; 8Schworm and Renkl, 2006; 9Len, 2006–2007; 10Hoekstra and Mollborn, 2011; 11Okada and Simon, 1997 | ||
L5. Students solve problems without hints | Percent of activities in which answer is not hinted at between iterations of student engagement. | ✓1–4 | ✓3 | 1Perez et al., 2010; 2Brooks and Koresky, 2011; 3Nielsen et al., 2012; 4Kulhavy, 1977 | ||
L6. Students hear students describing their logic | Percent of activities in which students share their logic in front of the whole class | ✓ | Webb et al., 2008 (*), 2009 (*); Turpen and Finkelstein, 2010 (S) | |||
L7. Logic behind correct answer explained | Percent of activities in which correct answer is explained | ✓1– 3 | ✓2, 3 | 1Smith et al., 2011; 2Butler et al., 2013; 3Nicol and Boyle, 2003 (S); 3Nielsen et al., 2012 (S) | ||
L8. Logic behind why incorrect or partially incorrect answers are explained | Percent of activities in which alternative answers are discussed during debrief | ✓ | Nielsen et al., 2012 (S); Turpen and Finkelstein, 2010 (S) |
To provide students with opportunities to practice their logic development, it is necessary for instructors to formulate questions that require a higher level of thinking (PORTAAL element logic development 1, L1; Morris et al., 1977; Ericsson et al.,1993; Jensen et al., 2014). One method for writing questions that require logic and critical thinking is to specifically write questions at higher Bloom levels (cf. Crowe et al., 2008).
One of the simplest ways to improve student conversations and increase student focus on sense-making and logic when introducing activities is for instructors to remind students to provide the rationale for their answers (L2; Turpen and Finkelstein, 2010; Knight et al., 2013). Additional evidence for the importance of encouraging students to explain their logic comes from Willoughby et al. (2000) and Wood et al. (1994), who demonstrated that students who generate explanations perform better on exams than those who did not.
When students begin to work on an instructor-posed question (such as a clicker question), the inclusion of several elements can increase student outcomes related to logic development. First, providing an explicitly delineated time at the beginning of the discussion period when students have an opportunity to think through their answers on their own (L3) increases the likelihood that a student will choose to join in a subsequent small- or large-group discussion and thus improve the discussion (Nielsen et al., 2012). Students also self-report that having time to think independently before discussions allows time to think through the question and come up with their own ideas (Nicol and Boyle, 2003). This individual time can be set up as a short minute-writing exercise or as a clicker question answered individually.
Second, a deeper understanding of the material is often gained when students share and explain their answers to other students (i.e., work in small groups; L4; Wood et al., 1995, 1999; Renkl, 2002; Sampson and Clark, 2009; Menekse et al., 2013). This group work increases performance on isomorphic clicker questions and posttests compared with performance of students who only listened to an instructor explanation (Schworm and Renkl, 2006; Smith et al., 2011). Group work is particularly important for tasks that require students to transfer knowledge from one context to another (Gadgil and Nokes-Malach, 2012). Small-group work also increased the frequency of students spontaneously providing explanations for their answers (Okada and Simon, 1997). In addition, small-group discussions 1) improve student attitudes, particularly the attitudes of low-performing students, toward the discussion topic (Len, 2006–2007); 2) improve social cohesion and, through that, feelings of accountability (Hoekstra and Mollborn, 2011); and 3) develop students’ argumentation skills (Kuhn et al., 1997; Eichinger et al., 1991).
Another key element involves the instructor hinting at or revealing correct answers. Correct answers should not be hinted at between iterations of discussion (L5). For example, showing the histogram of clicker responses can cause more students to subsequently choose the most common answer whether or not it is right (Perez et al., 2010; Brooks and Koresky, 2011; Nielsen et al., 2012). Seeing the most common answer also leads to a reduction in quality of peer discussions (Nielsen et al., 2012). In addition, making the correct answer easier to access (through hints or showing a histogram of student responses) reduces the effort students put into determining the correct answer and therefore reduces learning outcomes (Kulhavy, 1977).
It is also important that the whole class hear a fellow student offer an explanation for the answer selected (L6). This provides all students with immediate feedback on their logic from peers or the instructor, which helps them correct or develop their ideas (deWinstanley and Bjork, 2002) and sets the tone that the instructor values student logic and critical thinking, not just the right answer (Turpen and Finkelstein, 2010). Although not explicitly tested at the college level, an instructor asking students to provide their logic in whole-class discussions has shown the additional benefit of increasing the amount of explaining students do in small-group discussions in K–12 classes (Webb et al., 2008, 2009).
Finally, it is important for the instructor to explain why the correct answer is correct (L7; Smith et al., 2011; Nielsen et al., 2012). This provides a model for students of the type of logical response expected. Some students consider this explanation key to a successful class discussion (Nicol and Boyle, 2003). Equally important is modeling the logic behind why the wrong answers are wrong (L8; deWinstanley and Bjork, 2002; Turpen and Finkelstein, 2010).
Dimension 3: Accountability.
Motivating students to participate is another critical component for the success of any active-learning classroom. This motivation could come through the relevancy of the activity (Bybee et al., 2006), but this relevancy could be challenging for an observer to know, so it was excluded from our tool. Instead, we focused on teacher-provided incentives that create attention around the activity (Table 3; deWinstanley and Bjork, 2002). One such incentive is to make activities worth course points (PORTAAL element accountability 1, A1). Awarding course points has been shown to increase student participation (especially the participation of low-performing students; Perez et al., 2010) and increase overall class attendance and performance (Freeman et al., 2007). There are two primary strategies used by instructors for assigning course points for in-class activities: participation and correct answer. Having students earn points only for correct answers has been shown to increase student performances on these questions and in the course (Freeman et al., 2007), but it has also been shown to reduce the quality of the small-group discussions, as 1) these interactions were dominated by the student in the group with the greatest knowledge, 2) there were fewer exchanges between students, and 3) fewer students participated in the discussions overall (James, 2006; James and Willoughby, 2011). In an experiment explicitly designed to test how grading scheme impacts learning, Willoughby and Gustafson (2009) found that although students answered more clicker questions correctly when points were earned based on correctness, this grading scheme did not lead to an increase in achievement on a posttest relative to the participation-point condition. This result was supported by the Freeman et al. (2007) study as well. These results, paired with the other studies, demonstrate that both conditions lead to equal learning but that the participation-point condition leads to more complex student discussions. Instructors should thus choose the outcome most important to them and use the associated grading scheme.
How element is observed in the classroom | Increases achievement | Improves conversations | Improves other measures | Citations | ||
---|---|---|---|---|---|---|
Dimension 3: Accountability | ||||||
Elements | A1. Activities worth course points | Percent activities worth course points (may require a syllabus or other student data source) | ✓ correct answer1, 2 participation3 | ✓ participation4–6 | 1Freeman et al., 2007; 2Len, 2006–2007; 3Willoughby and Gustafson, 2009; 4Perez et al., 2010; 5James and Willoughby, 2011; 6James, 2006 | |
A2. Activities involve small-group work, so more students have opportunity to participate | Percent activities in which students work in small groups | ✓ | Hoekstra and Mollborn, 2011; Chidambaram and Tung, 2005; Aggarwal and O’Brien, 2008 | |||
A3. Avoid volunteer bias by using cold call or random call | Percent activities in which cold or random call used | ✓ | Dallimore et al., 2013; Eddy et al., 2014 |
A second way to motivate student participation is by creating situations wherein students will have to explain their responses either in small groups (A2) or to the whole class (A3). However, most instructors elicit responses from students by calling on volunteers in the classroom setting (Eddy et al., 2014). Volunteer responses are generally dominated by one or a few students, and the majority of the class know they will not be called on and thus do not need to prepare to answer (Fritschner, 2000). Volunteer-based discussions also demonstrate a gender bias in who participates, with males speaking significantly more than females (Eddy et al., 2014). For these reasons, the research literature recommends instructors find alternative methods for encouraging students to talk in class.
Alternatives to volunteer responses include: small-group work (A2) and random or cold-calling (A3). Although participating in small-group work may still seem voluntary, psychology studies in classrooms have demonstrated that the smaller the group a student is in (e.g., pairs vs. the entire class) the more likely he or she is to participate in group work and the higher the quality of individual answers (Chidambaram and Tung, 2005; Aggarwal and O’Brien, 2008). The idea behind this pattern is that there is a dilution of responsibility and reward in a large class (students are fairly anonymous and will not get personally called out for not participating, and, even if they do participate, the chance they will be rewarded by being called on is low), which decreases motivation to participate (Kidwell and Bennett, 1993). In small groups, the situation is different: student effort (or lack thereof) is noticed by group mates. In addition, social cohesion, attachment to group mates, is also more likely to form between members of small groups than between members of a large lecture class, and this cohesion increases a student’s sense of accountability (Hoekstra and Mollborn, 2011).
Cold-calling involves calling on students by name to answer a question. Random call is a modified version of cold-calling in which instructors use a randomized class list to call on students. Although these methods may seem intimidating and punitive to students, researchers have shown that cold-calling, when done frequently, actually increases student self-reported comfort speaking in front of the class and is correlated with students becoming more willing to volunteer in class (Dallimore et al., 2013). In addition, random call both eliminates gender bias in participation (Eddy et al., 2014) and guarantees all students have an equal chance of being called on.
Dimension 4: Reducing Student Apprehension.
The final dimension is also related to increasing student motivation to participate in class. Instead of raising the incentive to participate, instructors can frame their activities in ways that reduce a student’s fear of participation. This can be done in a number of ways, but many of these would be hard to reliably document, so we focus instead on three strategies that are explicit and observable and have shown positive changes correlated with student learning and/or participation (Table 4).
How element is observed in the classroom | Increases achievement | Improves conversations | Improves other measures | Citations | ||
---|---|---|---|---|---|---|
Dimension 4: Reducing apprehension | ||||||
Elements | R1. Give students practice participating by enforcing participation through cold/random call | Percent activities with random or cold-calling used during student engagement or debrief | ✓ | Ellis, 2004 (S); Dallimore et al., 2010 | ||
R2. Student confirmation: provide praise to whole class for their work | Percent debriefs and engagements in which class received explicit positive feedback and/or encouragement | ✓1–3 | ✓3, 4 | 1Ellis, 2004 (S); 2Ellis, 2000 (S); 3Goodboy and Myers, 2008 (S); 4Fritschner, 2000 | ||
R3. Student confirmation: provide praise/encouragement to individual students | Percent student responses with explicit positive feedback and/or encouragement | ✓1–3 | ✓3, 4 | 1Ellis, 2004 (S); 2Ellis, 2000 (S); 3Goodboy and Myers, 2008 (S); 4Fritschner, 2000 | ||
R4. Student confirmation: do not belittle/insult student responses | Percent student responses that do not receive negative feedback | ✓1 | ✓2 | 1Ellis, 2004 (S); 2Fritschner, 2000 | ||
R5. Error framing: emphasize errors natural/instructional | Percent activities in which instructor reminds students that errors are nothing to be afraid of during introduction or student engagement periods | ✓ | Bell and Kozlowski, 2008 | |||
R6. Emphasize hard work over ability | Percent activities in which instructor explicitly praises student effort or improvement | ✓ | Aronson et al., 2002; Good et al., 2012 |
One of the most common ways instructors motivate students to participate is through confirmation. Confirmation behaviors are those that communicate to students that they are valued and important (Ellis, 2000). These instructor behaviors have been correlated with both affective learning (how much students like a subject or domain) and the more typical cognitive learning (Ellis, 2000, 2004; Goodboy and Myers, 2008). Behaviors students generally interpret as affirming include but are not limited to: 1) the instructor not focusing on a small group of students and ignoring others, which random call accomplishes (PORTAAL element apprehension reduction, R1), or praising the efforts of the whole class rather than an individual student (R2); and 2) communicating support for students by indicating that student comments are appreciated (praising a student’s contribution, R3) and not belittling a student’s contribution (R4). These behaviors can also influence students’ willingness to participate in class (Fritschner, 2000; Goodboy and Myers, 2008).
In addition to using confirmation behaviors, instructors can increase participation by framing 1) student mistakes as natural and useful and/or 2) student performance as a product of their effort rather than their intelligence. The first type of framing, called error framing (R5), increases student performance by lowering anxiety about making mistakes (Bell and Kozlowski, 2008). The framing itself is simple. In the Bell and Kozlowski (2008) study, students in the treatment group were simply told: “errors are a positive part of the training process” and “you can learn from your mistakes and develop a better understanding of the [topic/activity].” Emphasizing errors as natural, useful, and not something to be afraid of can also encourage students to take risks and engage in classroom discussions.
The second type of framing, the growth mind-set, is intended to change a student’s course-related goals. There is considerable evidence in the K–12 literature that students who hold a performance goal (to get a high grade in the course) will sacrifice opportunities for learning if those opportunities threaten their ability to appear “smart” (Elliot and Dweck, 1988). This risk avoidance manifests as not participating in small-group work or not being willing to answer instructor questions if the questions are challenging. One way to influence student goals for the class is through praise based on effort rather than ability. Praise based on ability (“being smart”) encourages students to adopt a performance goal rather than a mastery goal (a desire to get better at the task) and to shy away from tasks on which they might not perform well. Praise that focuses on effort encourages a mastery goal and can increase student willingness to participate in challenging tasks (R6; Mueller and Dweck, 1998). At the college level, an intervention that had students reflect on a situation in their own life in which hard work helped them improve also increased their achievement in their classes (Aronson et al., 2002). Furthermore, for some college students, the perception that their instructor holds mastery goals for the class leads to improved performance relative to students who believe the instructor has a performance orientation (Good et al., 2012).
In summary, we identified 21 elements that capture four dimensions of best practices for active learning (practice: P1–4; logic development: L1–8; accountability: A1–3; and apprehension reduction: R1–6; Supplemental Table 1).
Goal 3: Validity, Reliability, and Ease of Use of PORTAAL
Content Validity.
The validity of PORTAAL is based in the research literature. Each of the 21 elements is supported by at least one published peer-reviewed article that demonstrates its impact on a relevant student outcome (see Goals 1 and 2: Identifying Research-Based Best Practices for Large Classrooms (Literature Review). As more research is done on the finer points of active-learning methods in the classroom, we will continue to modify PORTAAL to keep it up to date.
Face Validity.
After the elements were identified from the research literature, we discussed how the elements might be observed in the classroom. The observations we developed are not necessarily perfect measures but are nevertheless indicators for the potential presence of each element. Furthermore, the defined observations met our goal that observers without deep pedagogical content knowledge can use it readily and reliably.
Though there are 21 elements in PORTAAL, three of the elements could be consolidated into other elements for an online face-validity survey (see the Supplemental Material). We presented these 18 observations to seven BER researchers who have published in the field within the past 2 yr. We asked these BER researchers whether they agreed or disagreed that the stated observation could describe the presence of a particular element in the classroom. If the reviewer disagreed, we asked him or her to indicate why. There was 100% agreement for 11 of the 18 observations. Seven elements had 86% agreement, and one element had 71% agreement (see Validity (DBER researcher agreement) column in Supplemental Table 2: BER Review Comments for more details).
Reliability.
PORTAAL observations are completed on an observation log (see https://sites.google.com/site/uwbioedresgroup/research/portaal-resources) that asks observers to record the timing, frequency, and presence/absence of events as they occur in an activity. For example, observers record the start and end time of a small-group discussion, they count the number of students who talk during an activity debrief, and they record whether or not students are explicitly reminded to explain their answers to one another. An activity was operationally defined as any time students engage with a novel problem or question. An activity can be challenging to delineate if there are a series of questions in a row. We enforced the rule that if the next question is beyond the scope of the initial question, then it is a new activity. Ultimately, activity characteristics are pooled to create an overall description of the class session. Our aim with PORTAAL was that the recording of these discrete and observable elements would make this tool reliable and easy to learn. In this section, we test whether we accomplished this aim.
PORTAAL observations are done in pairs. Each observer independently watches a class session and records his or her observation on the log, and then observers come to consensus on what they observed. In our study, we used two individuals who had no prior teaching experience (a recent graduate from the undergraduate biology program and a master’s student in engineering) to observe 15 different class sessions. To determine interobserver reliability, we analyzed their original independent observations before they came to consensus. We used the intraclass correlation coefficient (ICC) to assess reliability data, as our data were interval data (percentages). We used a two-way agreement, single-measures ICC (McGraw and Wong 1996). The resulting ICCs were all > 0.8, and thus all were in the “excellent” range (Supplemental Table 2; Cicchetti 1994). This indicated a high degree of agreement across both our coders and supports the reliability of the PORTAAL measures.
Next, to determine how easy it was for different types of observers to learn PORTAAL, we trained and assessed the observations of four additional sets of observers. One pair of observers were current undergraduates in fields other than biology with no teaching experience (individuals without deep pedagogical content knowledge or disciplinary content knowledge). Another set was a graduate student in biology and a postdoc who had some teaching experience. The final two sets of observers were instructors with extensive teaching experience.
To train these four pairs of observers, we developed a training manual and a set of three short practice videos (available at https://sites.google.com/site/uwbioedresgroup/research/portaal-resources). Training involves a moderate time commitment that can occur over multiple days: 1 h to read the manual, 1 h to practice Blooming questions and come to consensus with a partner, and 2–3 h of practice scoring sample videos and reaching consensus.
We compared the scores of the four training teams with the scores of our experienced pair of observers for the first training video to get a sense of how quickly new observers could learn PORTAAL. Although statistics could not be run on such small sample sizes, we found that, on their first attempt to score a video, the four pairs of novice observers exactly matched the expert ratings ≥ 90% of the time for 19 of the 21 dimensions of PORTAAL. There were only two dimensions with less than an 85% match with the experts. These two dimensions were Bloom level of the question (matched 80% of the activities) and whether or not an instructor provided an explanation for the correct answer (70% of the activities). These data validate that the discrete and observable elements in PORTAAL make this tool reliable and easy to learn.
PART 2: ASSESSING CLASSROOM PRACTICES WITH PORTAAL
Is There Variation in the Use of Active-Learning Best Practices in Large Lectures?
The use of active learning in STEM disciplines usually increases student achievement (Freeman et al., 2014), but the extent of instructor implementation of those active-learning strategies in the classroom varies. In this section, we test whether PORTAAL can document this variation.
Our Sample
We used PORTAAL to document the teaching practices of 25 instructors teaching in the three-quarter introductory biology series at a large public R1 university. The first course in the series focuses on evolution and ecology; the second on molecular, cellular and developmental biology; and the third on plant and animal physiology. The majority (n = 21) of the instructors in this study only taught half of the 10-wk term, while four taught the whole term. Classes ranged in size from 159 to more than 900 students. The instructors in this sample all used clickers or other forms of student engagement.
One of the strengths of this study is its retrospective design. At this university, all courses are routinely recorded to allow students in the classes to review the class sessions. We used this archived footage, rather than live observations, to preclude instructors from changing their teaching methods. The archived footage also allows observers to pause the video while they record observations. We do not believe it will ever be practical to implement PORTAAL in real time, so recordings are critical for effective use of this tool. Our classroom recordings were done from the back of the room, so we had a view of the instructors as they moved around the front of the room, the screen on which they projected their PowerPoints, and a large section of the students in the class. The sound in our recordings came from the microphone the instructor used, so we generally could hear what the instructor could hear.
Kane and Cantrell (2013) found that two trained individuals observing the same 45-min session of a teacher’s class captured instructor teaching methods as well as having four independent observations of four different class sessions, indicating that fewer classes can be observed if two observers are used. Based on this finding, in our study, to be conservative and to increase the number of student–teacher interactions sampled, we decided to observe three randomly selected class sessions from across the term for each instructor. These 75 videos were first independently scored by two individuals (a recent biology graduate and an engineering master’s student) using the PORTAAL observation log; this was followed by the observers reaching consensus on all observations.
In addition to the 25 instructors, we identified two instructors whose implementations of active learning have been documented to increase student achievement on instructor-written classroom exams. Bloom levels of exam questions were assessed, and exams were determined to be equivalent, if not a little more challenging, in the reformed course across the terms included in these studies (for details, see Freeman et al., 2011; Eddy et al., 2014). These two instructors changed aspects both inside and outside their classrooms, and, at this time, we cannot fully resolve what proportion of the student learning gains are due to the instructor’s classroom practices. However, we were interested to see whether these instructors were more frequently employing PORTAAL elements in their classrooms. We will call these instructors our “reference” instructors for the remainder of this paper. The data from these instructors are included on Figures 1–5 as possible targets for instructors who desire to achieve gains in student achievement. The same methods as those described above were used to score three videos of each of these two reference instructors.
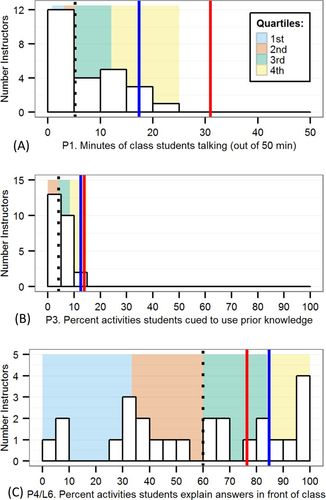
Figure 2. Dimension 1: Practice—variation in implementation of elements. Histograms demonstrating the variation in instructor classroom practice for each element of the dimension of practice. The black dotted line is the median for the 25 instructors; the red line is the practice of the instructor who reduced student failure rate by 65%; and the blue line is the instructor who reduced failure rate by 41%. Each quartile represents where the observations from 25% of the instructors fall. Quartiles can appear to be missing if they overlap with one another (e.g., if 50% of instructors have a score of 0 for a particular element, only the third and fourth quartiles will be visible on the graphs).

Figure 3. Dimension 2: Logic development—variation in implementation of elements. Histograms demonstrating the variation in instructor classroom practice for each element of the dimension of logic development. The black dotted line is the median for the 25 instructors; the red line is the practice of the instructor who reduced student failure rate by 65%; and the blue line is the instructor who reduced failure rate by 41%. Each quartile represents where the observations from 25% of the instructors fall. Quartiles can appear to be missing if they overlap with one another.

Figure 4. Dimension 3: Accountability—variation in implementation of elements. Histograms demonstrating the variation in instructor classroom practice for each element of the dimension of accountability. The black dotted line is the median for the 25 instructors; the red line is the practice of the instructor who reduced student failure rate by 65%; and the blue line is the instructor who reduced failure rate by 41%. Each quartile represents where the observations from 25% of the instructors fall. Quartiles can appear to be missing if they overlap with one another.
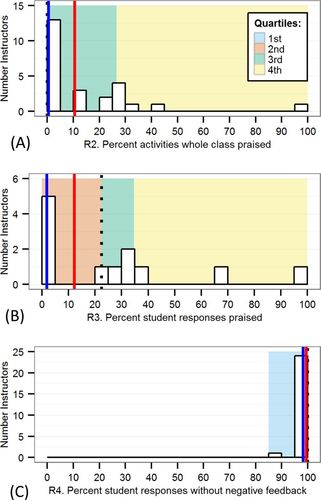
Figure 5. Dimension 4: Apprehension reduction—variation in implementation of elements. Histograms demonstrating the variation in instructor classroom practice for each element of the dimension of apprehension reduction. The black dotted line is the median for the 25 instructors; the red line is the practice of the instructor who reduced student failure rate by 65%; and the blue line is the instructor who reduced failure rate by 41%. Each quartile represents where the observations from 25% of the instructors fall. Quartiles can appear to be missing if they overlap with one another.
The blue reference instructor teaches the first course in an introductory biology sequence for mixed majors. The course covers general introductions to the nature of science, cell biology, genetics, evolution and ecology, and animal physiology, and averages more than 300 students a term. The blue instructor decreased failure rates (instances of a “C−” or lower) on exams by 41% (Eddy and Hogan, 2014) when the instructor changed from traditional lecturing to a more active-learning environment that incorporated greater use of group work in class and weekly reading quizzes.
The red reference instructor teaches the first course in an introductory biology sequence for biology majors. This course covers topics including evolution and ecology. The course ranges from 500 to 1000 students a term. This instructor has changed the classroom environment over time to incorporate more group work, more opportunities for in-class practice on higher-order problems, and less instructor explanation. Outside-of-class elements that changed included the addition of reading quizzes and practice exams. With these changes, the failure rate decreased dramatically (a 65% reduction; Freeman et al., 2011). Again, we cannot specifically identify the relative contribution of the change in classroom practices to the change in failure rate, but we can say it was one of three contributing elements.
Calculating PORTAAL Scores
The 21 elements in PORTAAL do not sum to a single score, as we do not know the relative importance of each element for student outcomes. Thus, instead of a score, instructors receive the average frequency or duration of each element across their three class sessions (the conversion chart can be found at https://sites.google.com/site/uwbioedresgroup/research/portaal-resources). We hypothesize that the more frequently instructors employ the elements documented in PORTAAL, the more students will learn. We test this hypothesis visually in a very preliminary way by looking at where the two reference instructors fall on each dimension. We do not expect these two instructors to practice all the elements, but we do predict on average that they will incorporate the elements more frequently.
To identify the variation in implementation of each element across the 25 instructors, we calculated and plotted the median and quartiles for each element. Thus, instructors are able to compare their PORTAAL element scores with this data set and select elements they wish to target for change.
Results
Overall, we found large variation in how instructors implement active learning in introductory biology classrooms. In addition, we see that both reference faculty members had values in the top (fourth) quartile for 52% of the PORTAAL elements, suggesting that more frequent use of PORTAAL elements increases student learning.
Dimension 1: Practice.
More than half the instructors allowed students <6 min per 50-min class session to engage in practice, whereas the two reference instructors allowed 17 and 31 min (P1; Figure 2A). Evidence for the use of distributed practice was low, with a median of 4.2% of activities explicitly cueing students to use prior knowledge, whereas the two reference instructors had ∼13% of activities focused on this (P3; Figure 2B). The element of immediacy of feedback on student ideas occurred much more frequently than any of the other dimensions of practice: the median number of activities in which instructors heard student explanations was 60%, and instructors in the third and fourth quartiles overlapped with the reference instructors (84.7% and 76.5%; P4; Figure 2C). We could not assess the alignment of practice (P2), as we did not have exams for all these instructors.
Dimension 2: Logic Development.
Logic development had the greatest variation of the four dimensions, with some elements performed routinely and others not at all. Instructors routinely followed best practices and did not show a clicker histogram of results or give students hints between iterations of student engagement (median 96.1% of activities; L5; Figure 3E), and instructors routinely explained why the correct answer was right during debriefs (71.8% of activities; L7; Figure 3F). Values for both these elements were similar in frequency to those of our reference instructors. Other elements of logic development were less evident: a median of 15% of activities involved higher-order cognitive skills (L1), whereas our reference instructors used higher-order questions in 34.7 and 64.2% of the activities (Figure 3A); 32.2% of activities involved students talking through their answers in small groups (L4), which was similar to the blue instructor but half that of the red instructor (Figure 3D); 8.3% of activities initiated student engagement with an initial think-alone period for students to formulate their answers (L3), which was less than either reference instructor (Figure 3C); and 10.3% of activities involved debriefs with explanations for why the wrong answers were wrong (L8), which was similar to the reference instructors (Figure 3G). Finally, in our sample, less than 25% of instructors explicitly reminded students to provide the logic for their answers when they introduced the activity (L2; Figure 3B). This was true for the reference instructors as well.
Dimension 3: Accountability.
On average, the median percent of activities with at least some form of accountability (points, random call, or small-group work) across our sample was high: 65.5%. Most of that accountability comes from assigning course points to engagement (22.7% of activities; A1; Figure 4A) and small-group work (A2; Figure 4D). Fewer than 25% of instructors use cold or random call in their courses (median 0% of activities; A3), including the blue instructor (Figure 4B). The red instructor uses random call during 56% of activities.
Dimension 4: Apprehension Reduction.
In general, instructors (including reference instructors) did not direct much effort toward reducing student’s apprehension around class participation in the observed videos: the median percent of student comments receiving positive feedback was 12.2% (R3; Figure 5B), and the median percent of activities in which positive feedback was directed to the class was only 7.5% (R2; Figure 5A). On the other hand, instructors were not creating intimidating environments with negative feedback (R4; Figure 5C). We only observed one instance of negative feedback across our entire sample. Finally, the version of PORTAAL we used to make these observations did not incorporate elements R5 (error framing) and R6 (a focus on hard work), so we cannot provide specific numbers for these elements. Anecdotally, neither observer believed they observed any explicit incidences of either.
Implications of PORTAAL Results
These PORTAAL results indicate there is considerable variation in instructor implementation of evidence-based best teaching practices in the classroom in introductory biology courses at a large R1 university. PORTAAL provides an indication of the amount of practice students undertake in class and identifies how well these opportunities for practice align with evidence-based best practices for active learning identified in the literature. By measuring elements that have been shown in the research literature to help students develop their logic, encourage the whole class to participate in the practice, and increase student comfort or engagement, PORTAAL provides more nuanced results that can be used by instructors to identify what they already do well and offer guidance as to specific research-supported actions they can take to more fully support student learning in their classrooms.
In our sample, we see that instructors, on average, frequently practice many of the PORTAAL elements: they do not regularly provide hints during the engagement part of the activity, do frequently explain why the right answer is correct, do make students accountable for participating, and do not discourage student participation with negative feedback. Our results also lead us to make specific recommendations for improvement: instructors in this sample could 1) increase opportunities for practice by incorporating more distributed practice and more higher-order problems in class; 2) improve the development of student logical-thinking skills by reminding students to explain their answers, providing students explicit opportunities to think before they talk, and using random call to spread participation across the class; 3) increase participation through accountability by using cold call or random call more frequently; and 4) reduce participation apprehension by reminding students that mistakes are part of learning and not something to be feared. Obviously, an instructor could not address all of these at once but could choose which to pursue based on his or her own interests and PORTAAL results.
In addition, PORTAAL can be used to identify how instructor teaching practices differ from one another. By comparing our two reference instructors with the 25 instructors and with one another, we see that the reference instructors employ more PORTAAL elements more frequently than the median of the instructors in our sample. In addition, the red instructor, who had the greatest decrease in failure rates, used several PORTAAL elements (including P1, L1, L3, and L4) more frequently than the blue instructor. This again suggests that more frequent use of PORTAAL elements increases student learning.
Limitations of PORTAAL
PORTAAL, like any tool, was designed with a particular purpose and scope: to reliably evaluate the alignment between instructor implementations of active learning and research-supported best practices in the classroom. Thus, the focus of the tool is on classroom practice, but the effectiveness of an active-learning classroom also depends on elements not captured by the tool, including characteristics of the students, characteristics of exams, course topics, and student activities outside class (Figure 1). For example, it is likely that the usefulness of what goes on in class is determined by how well students are prepared for class and how much practice they have outside of class. These out-of-class elements could in turn be influenced by student characteristics such as their prior academic preparation and their motivation (Figure 1). PORTAAL does not capture outside-class assignments or student-level characteristics. This is an important caveat, as most of the studies in this review were conducted at selective R1 universities that have a very specific population of students. In addition, we explicitly chose not to focus on how the instructor scaffolds course content. This is a critical aspect of instruction, but it is difficult to assess without deep pedagogical content knowledge. Finally, PORTAAL focuses on observable and explicit behaviors in the classroom. Some elements may not be perfectly captured by these types of measures. For example, teacher confirmation behaviors are only measured by explicit instances of praise, but there are many other ways that teachers can confirm students, such as body language or tone of voice. For a more nuanced picture of instructor confirmation, instructors could use the survey developed by Ellis (2000). In addition, our measure of distributed practice can only measure instances in which the instructor explicitly cues students to use prior knowledge, which will likely underestimate instances of distributed practice. These limitations are necessary for reliability in the instrument but may lead to underestimating the frequency of the element. The final limitation of PORTAAL is that it assumes the observers can record all the important interactions that go on in the classroom. This limits the classroom types this tool can effectively evaluate. PORTAAL is designed for large-enrollment courses in which it would be difficult for an instructor to interact individually with the majority of the students in a class period. PORTAAL would not work well in a small seminar-style discussion with frequent student-to-student, whole-class discussion or a lab course in which students are at different stages of a project. In addition, it is not feasible to use PORTAAL reliably in real time. We recommend videotaping the class with a focus on the instructor and a view of some of the students. Despite these limitations, we see this rubric as useful for the majority of STEM classes. Following the suggestions outlined in this tool does not guarantee greater student learning, but the tool is a solid, research-supported first step.
PART 3: CONCLUSION
How Can PORTAAL Increase Implementation of Evidence-Based Active-Learning Activities in the Classroom?
From our analysis of 25 instructors, it is evident there is extensive variation in implementing research-based best practices for active learning in the classroom. This result is not exclusive to biology, as it has also been seen in engineering (Borrego et al., 2013), physics (Henderson and Dancy, 2009), and extensively at the K–12 level (O’Donnell, 2008). One of the major causes of such variation may be the nebulous nature of active learning. One of the most popular definitions of active learning comes from Hake (1998): “those [practices] designed at least in part to promote conceptual understanding through interactive engagement of students in heads-on (always) and hands-on (usually) activities which yield immediate feedback through discussion with peers and/or instructors.” Although a good summary, this statement does not provide practical guidance on the proper implementation of active learning.
Unfortunately, the education literature does not always identify the key elements of teaching innovations or clarify how to implement them in the classroom (Fraser et al., 2014). The education research literature is written for education researchers and seldom provides sufficient detail or scaffolding for instructors who are not education researchers to read the methods and identify the critical elements for successful implementation (Borrego et al., 2013). PORTAAL is one effort to create a tool that translates the research-based best practices into explicit and approachable practices. It accomplishes the following:
PORTAAL identifies research-based best practices for implementing active learning in the classroom. PORTAAL distills the education literature into elements that have been shown to increase student outcomes in terms of learning, logic development, or measures correlated with both. Thus, the elements in this rubric could be considered “critical components” for the success of active learning, and fidelity to these elements should increase student outcomes as they did in the literature. In addition, the elements in PORTAAL go beyond simply identifying the amount or type of active learning occurring in the classroom and also guide instructors as to how active learning should be implemented in the classroom (with accountability, logic development, and apprehension reduction) for the greatest impact on student learning. Proper implementation of research-based best practices does not guarantee improvement in student learning, but it does increase the odds of success over less scientifically based approaches (Fraser et al., 2014). | |||||
PORTAAL deconstructs best practices into easily implemented elements. One of the reasons instructors may not attempt to change their classroom practices is because it can feel overwhelming, and no one can change their practice successfully in multiple dimensions at the same time (Kreber and Cranton, 2000). PORTAAL breaks classroom practices into four dimensions (practice, accountability, logic development, and apprehension reduction) and within these dimensions identifies discrete elements, each representing a concrete action an instructor can take to improve his or her implementation of active learning. Instructors could devote an entire year to working on one element or may decide to focus on an entire dimension. By organizing best practices into dimensions and elements, instructors now have a framework that makes the process of change more manageable. | |||||
PORTAAL provides reliable and unbiased feedback on classroom practices. Instructors traditionally get feedback on their classes through personal reflections on their own classrooms, comparing how their classes are run relative to their own experiences as a student, and student evaluations (Fraser et al., 2014). All of these methods are biased by their own interpretations or student expectations. PORTAAL aligns observable classroom practices to research-supported best practices in a reliable manner and can provide objective feedback to the instructor. |
Uses of PORTAAL
Higher education currently lacks a scientific approach to teaching evaluations (Fraser et al., 2014). PORTAAL offers one such scientific approach by aligning classroom practices to research-supported best practices. PORTAAL analysis of classrooms can be useful in multiple ways:
For an instructor: Individual instructors can score their current classrooms to identify what they do well and where they could improve. Once they have identified a dimension to improve, PORTAAL offers additional elements from that dimension to incorporate into their classes. Instructors could score their classrooms over time to determine whether they have effectively changed their teaching. Instructors could include these PORTAAL scores in their teaching portfolio to document the effort they put into teaching. In addition, instructors in learning communities could observe a colleague’s classroom and provide feedback based on PORTAAL observations. | |||||
For a department and college: A department could use PORTAAL to determine the level at which instructors in the department implement evidenced-based teaching practices in their classrooms and identify exemplary instructors and recognize them. They could then promote their department to incoming students as one in which instructors use best practices in STEM education. We could also imagine that an instructor’s longitudinal PORTAAL scores could also be one of many measures of teaching effectiveness for tenure and promotion decisions. Colleges could use PORTAAL to document changes associated with teaching initiatives as well as documentation of teaching effectiveness for accreditation. | |||||
For researchers: Many education studies compare treatments across instructors or across classrooms. Variation between instructors in how they teach their courses could erroneously lead to conclusions about the impact of the treatment. PORTAAL observations of each classroom in a study would allow researchers to objectively compare the similarity of classrooms within or across treatment groups in terms of elements that have been shown to influence student learning. If they did not see differences, then they could be more confident that differences in outcomes were due to the treatment and not differences in classroom implementation. PORTAAL could also be used by researchers interested in faculty change, as PORTAAL could help determine how close instructors are to research-based best practices before and after a faculty development program. |
In summary, active learning and evidence-based teaching practices will soon become the expected teaching method across college campuses. PORTAAL provides a suite of easy to implement and evidence-based elements that will ease instructors’ transitions to this more effective teaching method and assist instructors in objectively documenting their progress toward accomplishing this educational goal.
ACKNOWLEDGMENTS
Support for this study was provided by NSF TUES 1118890.We thank Carl Longton and Michael Mullen for help with classroom observations. We also appreciate feedback on previous drafts from Scott Freeman, Alison Crowe, Sara Brownell, Pam Pape-Lindstrom, Dan Grunspan, Hannah Jordt, Ben Wiggins, Julian Avila-Pacheco, and Anne Casper. This research was done under approved IRB 38945.