Group Random Call Can Positively Affect Student In-Class Clicker Discussions
Abstract
Understanding how instructional techniques and classroom norms influence in-class student interactions has the potential to positively impact student learning. Many previous studies have shown that students benefit from discussing their ideas with one another in class. In this study of introductory biology students, we explored how using an in-class accountability system might affect the nature of clicker-question discussions. Clicker-question discussions in which student groups were asked to report their ideas voluntarily (volunteer call) were compared with discussions in which student groups were randomly selected to report their ideas (random call). We hypothesized that the higher-accountability condition (random call) would impress upon students the importance of their discussions and thus positively influence how they interacted. Our results suggest that a higher proportion of discussions in the random call condition contained exchanges of reasoning, some forms of questioning, and both on- and off-topic comments compared with discussion in the volunteer call condition. Although group random call does not impact student performance on clicker questions, the positive impact of this instructional approach on exchanges of reasoning and other features suggests it may encourage some types of student interactions that support learning.
INTRODUCTION
Discussing clicker questions is known to be beneficial for student learning in class, both in terms of immediate impact on performance (e.g., Mazur, 1997; Smith et al., 2009) and for generating deeper understanding (Nicol and Boyle, 2003). For example, engineering students engaged in discussions of clicker questions report listening to one another’s arguments and making answer choices depending on the strength of another student’s justification, as well as gaining confidence in their own understanding from knowing that others are struggling with similar concepts. These students even report that discussions with others help to scaffold their knowledge (Nicol and Boyle, 2003). Students also prove to be sensitive to the cues they are given by their instructors and peer coaches before they initiate their discussions. They are significantly more likely to engage in productive discussions (such as using reasoning to explain their answers and questioning one another) when cued to use this approach (Knight et al., 2013, 2015). Thus, ample evidence supports the idea that discussing clicker questions is likely to benefit learning.
However, there is still discussion about how grading classroom participation may affect student participation in classroom activities. The concept of social interdependence (that interactions with other people are essential for human survival) may impact student behaviors in scenarios in which they are required to interact with one another to achieve a reward. Social interdependence in a learning environment typically manifests as students’ efforts to achieve and develop relationships with one another and show competence. Interdependence is achieved when people feel that the success of the individual is related to the success of the group (Johnson and Johnson, 2005). Along these lines, some grading incentives appear to have a positive effect on collaboration. In a study that rewarded group success, astronomy students who initially preferred to work independently willingly shifted to collaborating with other students in small groups when all students were given a “success bonus” of doubling their participation points if more than 80% of students correctly answered in-class questions (Len, 2006). Almost all students reported collaborating on these questions and achieved the required 80% correct, thus securing the promised bonus points as a class. When there was no grading bonus, students collaborated less and achieved a lower percent correct. In another study, students worked collaboratively in class but were tested individually. When they were told that one member of the group’s test would count as the grade for all group individuals, the average performance for the students was significantly higher than when each student’s grade depended only on his or her own performance (Sarfo and Elen, 2011). In a similar study, students in a group were allowed to interact and help one another in an entirely Web-based collaboration. If a randomly chosen student’s quiz grade counted as the entire group’s grade, students were more likely to collaborate and more likely to achieve higher grades (Jensen et al., 2002). In such examples, group members are highly motivated to make sure they can all perform at a high level, since their grades could be determined by someone else’s performance. On the other hand, when astronomy students were encouraged to discuss clicker questions with one another but were then graded individually on the correctness of their answers, students tended to be less collaborative, focusing on getting the correct answer from whoever seemed to know the answer rather than sharing ideas or reasoning in order to understand the concept (James, 2006). In this case, the individual grading scheme changed the nature of student collaboration in discussions.
There is an additional way to hold students accountable for their engagement in class without a grading incentive: “random call” of individuals or groups of students to report their ideas to the whole class. In this model, a task is demonstrated to be important with a reward of in-class validation, emphasizing the learning process and the value of sharing scientific ideas rather than correctness. In effect, the task (discussion) directs student attention to understanding through reasoning, providing a context for their learning (Tomanek and Montplaisir, 2004). When Dallimore and colleagues investigated individual random call, they showed that a greater number and variety of students volunteered to answer questions after random call became part of the classroom culture than when the only mechanism of calling on students was through asking them to volunteer. They also found that students in courses employing random call self-reported more comfort with in-class participation over time, while those in voluntary participation classes experienced no such change (Dallimore et al., 2012). The random call format, used previously with clicker questions by Freeman et al. (2011), drives engagement through potential accountability in front of peers and the instructor. It also emphasizes the importance of having a discussion of substance, since it enforces student sharing of ideas, even if those ideas are incorrect or not fully composed.
We investigated the impact of using a modified version of random call, in which groups, rather than individual students, were randomly called. The effects of group random call on student discussion, to our knowledge, have not yet been investigated. We specifically measured whether group random call influenced the characteristics of in-class student discussions of clicker questions by comparing student discussion in two sections of an introductory biology course. In one section, idea sharing following discussion was voluntary, and in the other section, sharing was enforced through randomly calling on groups to report their ideas to the rest of the class.
METHODS
Course Characteristics
We performed this study in a freshman-level introductory molecular and cell biology course that is required for students planning to major in this discipline and is also taken by students intending on other majors in the life sciences (e.g., integrative physiology and neuroscience). This course has a total enrollment of ∼450 students every Fall, taught in two unequally sized sections due to timing and room constraints. We studied the smaller section of this course in two consecutive Fall semesters that had total enrollments of 94 and 110 students, respectively. The same two instructors cotaught this course in both semesters using the same textbook, lecture materials, and clicker questions. The instructors spent class time (50 minutes, three times per week) engaged in lecture interspersed with three to five clicker questions per class period, using the iClicker response system, with time given for discussion and feedback on each question. The instructors followed a modification of the standard clicker-question cycle (Duncan, 2004) in both semesters: the instructor displayed the clicker question and either asked students to make an initial independent vote or asked them to think about the answer on their own; the instructor then cued students to enter into discussion in their small groups, reminding them to use reasons to back up their preferred answers. Students then revoted on their answers or voted for the first time depending on the initial instructions. Histograms of student votes were not shown until after the students gave reasons for their answers, which came after group discussion of their ideas. All students received equal participation credit for answering clicker questions.
In both study conditions, groups were formed in the first 2 weeks of the course. Students were asked to choose their own groups of three to four people and asked to sit and work with their groups for the whole semester. In the volunteer call condition, students were not asked to officially record the makeup of their groups, since groups were not called on by the instructor. In the random call condition, groups were given an index card with a number on the front and were asked to record their names on the back so that their group numbers and names could be called out by the instructor. No additional points were awarded to either volunteers or randomly called groups.
Variables
The condition varied in this study was the implementation of sharing after small-group clicker discussion was complete: student groups were either asked to volunteer an explanation (“volunteer call”) or were selected randomly (“random call”) to explain their groups’ discussions. Following the revote after discussion in the volunteer call condition, the instructor asked for volunteers to tell the rest of the class about the answers their groups chose and their reasons for choosing a particular answer. In the random call condition, the instructor randomly chose a numbered index card from a box and asked this group to tell the rest of the class about the answer their group chose and their reasons for choosing that answer.
Some of the discussions included in the volunteer call data set have been previously analyzed and reported on, including discussions involving nearby learning assistants (Knight et al., 2015). Only discussions without learning assistant involvement were included in the current analysis.
Study Participants
In the volunteer call condition, 23 students (six groups) out of 94 agreed to be recorded. In the random call condition, 24 students (six groups) out of 110 agreed to be recorded. Students consented to remain in their groups, sit in the same places in each class period, and have their discussions recorded for the time frame of the study. Multiple recordings of several groups of students, rather than single recordings of a larger number of groups of students, allowed us to control for clicker-question variation and group-specific discussion variation in our analyses. Analysis of demographic information provided by the registrar’s office established that students in the two conditions were not significantly different in terms of grade point average (GPA), gender distribution, and year in school (Table 1).
Section | Number of volunteers/total number of students | % Female | Class ranka (SD) | GPA (SD) |
---|---|---|---|---|
Volunteer call | 23/94 | 54 | 1.8 (0.9) | 2.9 (0.7) |
Random call | 24/110 | 61 | 1.8 (0.9) | 3.0 (0.8) |
Data Collection
Each of the volunteer students wore a wireless microphone (“Lavalier” style) during six class periods (approximately weeks 9 and 10 of a 15-week semester). We used a Nady receiver and a digital audio recorder (Zoom Corporation) to combine wirelessly transmitted audio from each volunteer group of students during their discussions of clicker questions. The audio recordings were transcribed into an Excel spreadsheet, paired with the clicker question the students had discussed, and given a unique transcript number. Each speaker was given a number within a transcript to facilitate tracking student interactions and to tally the number of speakers within each discussion. However, individual speakers could not be reliably identified across discussions, precluding us from identifying and following an individual’s specific contribution over time.
Only clicker questions that were identical in the two conditions were used, for a total of 13 questions. These questions were rated on the Bloom’s taxonomy scale (Anderson and Krathwohl, 2001; Crowe et al., 2008) by two experts not associated with the study (see also Knight et al., 2015). Seven were rated as higher order and six as lower order.
The complete data set for this study includes 110 discussions: 46 discussions by six groups of students in the volunteer call condition and 64 discussions by six groups of students in the random call condition. Owing to occasional problems with recording equipment, all question discussions were not captured for each group; on average, eight out of 13 possible discussions were captured per group in the volunteer call condition and 10 out of 13 possible per group in the random call condition.
Students were also surveyed online about their opinions regarding clicker use, group work, and classroom dynamics at the beginning and end of the semester, answering 21 questions on a Likert scale of strongly disagree (1) to strongly agree (5), seven of which were directly related to discussion of clicker questions.
Data Analysis
The time given by the instructor for each discussion and time spent in on-task discussion was noted for each recording, and percent productivity was calculated (discussion length/time given). The number of speakers was determined for each discussion by giving each speaker a number designation (e.g., 1, 2, 3). Each speaker’s utterance was recorded on a separate line so that the total number of utterances from all students participating could be calculated: this value is referred to as the “turns of speech” for each discussion. Transcripts were then coded for various characteristics that describe the discussion as a whole, such as aspects of reasoning, student–student questioning, statements made about the proposed answers, and other comments. The majority of these codes have been previously published (Knight et al., 2015) but are reproduced in Table 2 along with additional discussion characteristics developed for this data set with complete definitions and examples. These descriptors are referred to as “whole-discussion codes,” because they describe the overall characteristics of student interactions during a single clicker discussion. This also distinguishes them from the codes that describe the nature of each student utterance within a discussion (line-by-line codes), described further in the Results.
Whole-discussion code | Definition/characteristics | Examples |
---|---|---|
Reasoning | ||
Exchange of Quality Reasoning (0–3) | ||
0 No reason provided | “What did you vote?” “A.” | |
1 One person provides reason(s) | “I think it’s because of transcription being different.” “Yeah.” | |
2 Two or more people provide simple reason(s) | “I think it’s because transcription is different in eukaryotes and prokaryotes.” “Yeah, and because of the sigma factor …” | |
3 Two or more people provide reasons supported by evidence and a logical connection (warrants) | “I think it’s because … there’s no nucleus in bacteria, so that would be a difference between eukaryotes and bacteria.” “There’s no need to transport the transcript out of the cytoplasm since the enzyme for making the mRNA transcript is right there.” | |
Reasoning about Multiple Answers | ||
More than one answer is considered, using reasoning | “It doesn’t have anything to do with the membrane because.…”; “But I think the concentration does matter because…” | |
Student–student questioning | ||
Requesting Information | Asking for votes or basic information, like definitions | “What did you vote?” “What does that mean?” |
Requesting Reasoning | Asking to share an explanation | “Why did you say that?” “Why were you thinking that?” |
Requesting Feedback | Statement of reasoning, followed by asking for confirmation of own reasoning | “Because it takes energy to break bonds, right?” |
Statements | ||
Claim | A statement of preference for an answer | “It’s C.” “It can’t be A.” |
Background | Providing information, a definition, or clarification of the clicker question | “‘A’ bonds with ‘T.’” “A gene is a sequence of DNA.” |
Comments | ||
Acknowledgment | Yes or no response to another person’s statement | “Okay.” “No way.” |
Related comment | Directly pertains to the topic, but does not further the discussion | “That’s interesting.” “That’s tricky.” |
Unrelated comment | Joking, off-topic, not related | “I’m going to the football game on Saturday.” |
All discussion codes were developed using an iterative process described in detail by Knight et al. (2015). For whole-discussion codes, interrater reliability was established with 20% of the discussions, using two or three raters who achieved a Cronbach’s alpha greater than 0.75 for each code reported on. The remaining transcripts were divided up and coded by two raters. For line-by-line coding, two raters achieved an 84% agreement on 10% of the data set; the remaining transcripts were coded by a single rater.
SPSS, version 22, was used for all statistical analyses. To determine whether the characteristics of the discussions were different between the random call and volunteer call discussions, we used regression analysis for each outcome (code), with independent variables of random call section/volunteer call section, discussion length, clicker-question number, Bloom’s level of question, and number of speakers. Because the same sequence of clicker questions was used in both sections, we used the clicker question number as a covariate rather than study day (previously used in Knight et al., 2015) to account for any potential changes due to individual clicker questions. Linear regressions were conducted for all continuous outcomes (length of discussion, turns of speech, percent productivity, and performance). Ordinal logistic regressions were conducted for the scaled Exchange of Quality Reasoning code, and binary logistic regressions were conducted for the remaining codes, which were all scored as presence (1) or absence (0). For successful linear regression models, the assumptions of linearity, independence of errors, homoscedasticity, unusual points, and normality of residuals were met and produced significant overall models with p values < 0.01. Some linear regression models were not significant, suggesting there were no contributions of the covariates to the outcomes. For logistic regressions, there were no significant interactions between the continuous outcome variables, thus meeting the assumption of linearity for each analysis; R2 values were between 0.2 and 0.4 for each model. Multicollinearity, proportional odds, goodness of fit, and model fitting were all within acceptable parameters for ordinal regressions (Field, 2009). The result of each regression model shows the impact of each independent variable on the discussion code, described in terms of an odds ratio and p value. Odds ratios can be interpreted as follows: the value describes the likelihood of the outcome resulting as a consequence of the independent variable (e.g., the random call section predicts a 2.5-higher likelihood of an outcome, all else being equal).
Human Subjects Approval
This work was reviewed by the University of Colorado Institutional Review Board, and the use of human subjects was approved (expedited, protocol 11-0630).
RESULTS
Discussion Time
The average number of turns of speech in a discussion, length of time given for discussion, length of discussion, and percent productivity of discussion in each of the two conditions are shown in Table 3. The unit of analysis in these data is a single group discussion. Students in the random call section exchanged significantly more turns of speech per discussion on average than those in the volunteer call section. However, their discussions were not different in length. In fact, instructors gave students significantly less time on average to discuss each clicker question in the random call (77 ± 15 seconds) compared with the volunteer call section (91 ± 24 seconds). This was not purposeful on the part of instructors and was discovered only after all data had been collected. In 16% of the discussions from the random call section, students used more time than was given to them (i.e., kept discussing after the instructor had closed the discussion), while this never occurred in the volunteer call section. As a consequence, although the average time taken by student to engage in discussion was similar (55–62 seconds), the average percent productivity was significantly higher in the random call section. We therefore included discussion length as a covariate in regression analyses of the whole-discussion characteristics to control for its potential impact on other features of student discussion.
Time characteristics | Volunteer call (n = 46) | Random call (n = 64) |
---|---|---|
Turns of speech | 12 turns (6) | 16 turns (9)a |
Time given | 91 seconds (24) | 77 seconds (15)b |
Discussion length | 62 seconds (30) | 55 seconds (25) |
Percent productivity | 68 (27) | 72 (29)c |
Performance
We characterized performance on clicker questions in two ways: overall performance by the whole class and performance by each recorded group. Class performance averages on the 13 clicker questions used in this analysis were similar between the two sections: 71% (±18 SD) correct in the volunteer call condition and 68% (±18 SD) correct in the random call condition (p = 0.67, independent-samples t test). In the random call section, the instructors generally asked students to consider their answers to clicker questions individually but did not always ask for an individual vote, while in the volunteer call section, the instructors usually asked for an individual vote and a revote. Because of this difference, we cannot compare gains in clicker performance between the two sections. However, for reference, the average percent correct for the whole class in the volunteer section on the initial vote was 50% (±14 SD), similar to previously reported values for initial votes with introductory biology students (Knight et al., 2015).
For performance by recorded group, each discussion was scored for percent correct based on the number of voting members in the group. Thus, if two out of three group members voted correctly, the group percent correct was recorded as 66%. We used linear regression analysis to determine whether the volunteer or random call condition influenced the final percent correct for each group following their discussion, using clicker-question ID, Bloom’s level of question, number of speakers, and length of discussion as covariates. Despite normal distribution of the outcomes, the regression models were not significant, and R2 values were less than 0.05; thus, there is no significant difference in group performance between the volunteer and random call conditions.
Random Call Affects Overall (Whole-Discussion) Characteristics
The whole-discussion codes used in this analysis describe the general features of student clicker discussions with regard to their use of reasoning statements, different kinds of questioning, statements that further discussion, and other short comments (see Table 2 for a complete description of characteristics).
In the category of Reasoning, the Exchange of Quality Reasoning code is intended to capture both the use and exchange of reasoning statements. Thus, the different levels of this code start at 0, a discussion in which no reasoning is used by any students in the discussion, to 3, in which two or more students exchange reasoning statements that they have logically supported with evidence (often referred to as “warrants”; Toulmin, 1958). In the discussions explored here, students rarely reached level 3, although level 2 discussions (in which reasoning is exchanged but not necessarily at the level of a warrant) were much more common. Less than 20% of discussions involve no reasoning at all (Table 4).
Whole-discussion code | Volunteer call Percent of discussions (n = 46) | Random call Percent of discussions (n = 64) |
---|---|---|
Reasoning | ||
Exchange of Quality Reasoninga | ||
No reasoning (0) | 15 | 9 |
One person reasons (1) | 37 | 30 |
Two or more exchange reasons (2) | 39 | 50 |
Two or more exchange warrants (3) | 9 | 11 |
Reasoning about Multiple Answers | 48 | 41 |
Questioning | ||
Requesting Information | 74 | 88* |
Requesting Reasoning | 15 | 23 |
Requesting Feedback | 30 | 50* |
Statements | ||
Claim | 91 | 83 |
Background | 26 | 25 |
Comments | ||
Acknowledgment | 57 | 83* |
Related Comments | 74 | 94* |
Unrelated Comments | 13 | 63* |
Reasoning about Multiple Answers describes discussions in which the reason(s) for more than one answer choice are discussed. A single individual could describe his or her reasons for multiple answers, or multiple individuals could be discussing their reasons for different answers; thus, this code is distinct from the Exchange of Quality Reasoning. As shown in Table 4, Reasoning about Multiple Answers is seen in a little less than half of student discussions.
Students typically use three kinds of questions in their discussion. The most common question is to ask another student what he or she voted (Requesting Information). The next most common is Requesting Feedback, in which a student first describes his or her reason for an answer and then ends with a question, requesting others to corroborate the idea or to check whether the idea is correct. Least common is Requesting Reasoning, in which a student directly asks another student to explain his or her reason for an answer (Table 4).
We also followed the use of five additional characteristics grouped into two larger categories. The category of “Statements” includes Claims (e.g., “I voted C”) and Background (clarifying the clicker question). The category of “Comments” includes Acknowledgments (generally one-word agreements), Related Comments (comments such as “That’s interesting” or “I didn’t know that”), and Unrelated Comments (see Table 3 for detailed code descriptions). Most discussions used Claims, in which students explicitly state which answer they selected as they are discussing their ideas. Background statements were used equally in both conditions but were not as frequent as Claims (Table 4).
We explored whether any of these whole-discussion characteristics were significantly different between the random call and volunteer call conditions, taking into account the additional covariates of Bloom’s level of question, clicker question ID, number of speakers, and discussion length, all of which could affect the characteristics of student discussion (Table 5). In the categories of Reasoning and Questioning, three discussion features were used at a significantly higher level or frequency in the random call condition: Exchange of Quality Reasoning, Requesting Information, and Requesting Feedback. Discussions in the random call condition were 2.7 times more likely to use a higher level of Exchange of Quality Reasoning, 3.2 times more likely to use Requesting Information, and 2.8 times more likely to use Requesting Feedback than in the volunteer call condition, all else being equal (see odds ratios and p values in Table 5). In addition, the covariate of discussion length was an additional significant predictor for higher frequency or level of all characteristics except Requesting Information. The other covariates of Bloom’s level, clicker-question ID, and number of speakers were not significant predictors of most discussion characteristics. Within the Comments code (Acknowledgments, Related Comments, Unrelated Comments), all three discussion features were used at a significantly higher frequency in the random call than in the volunteer call condition, all else being equal (see odds ratios and p values in Table 5).
Exchange of Quality Reasoning | Reasoning about Multiple Answers | Requesting Information | Requesting Reasoning | Requesting Feedback | |
---|---|---|---|---|---|
Regression factors | Odds ratio (p value) | Odds ratio (p value) | Odds ratio (p value) | Odds ratio (p value) | Odds ratio (p value) |
Random call section | 2.66 (0.01)* | 1.08 (0.85) | 3.21 (0.04)* | 2.25 (0.14) | 2.84 (0.02)* |
Discussion length | 1.00 (0.00)* | 1.03 (0.00)* | 1.03 (0.21) | 1.03 (0.01)* | 1.01 (0.04)* |
Clicker question | 1.00 (0.92) | 0.97 (0.42) | 1.04 (0.40) | 0.98 (0.72) | 0.96 (0.35) |
Number of speakers | 0.91 (0.72) | 0.99 (0.96) | 1.66 (0.17) | 0.96 (0.91) | 1.48 (0.15) |
Bloom’s level (high) | 1.04 (0.91) | 0.61 (0.36) | 1.66 (0.36) | 1.15 (0.78) | 0.83 (0.66) |
Claims | Background | Acknowledgments | Related Comments | Unrelated Comments | |
Regression factors | Odds ratio (p value) | Odds ratio (p value) | Odds ratio (p value) | Odds ratio (p value) | Odds ratio (p value) |
Random call section | 0.40 (0.18) | 1.34 (0.56) | 4.49 (0.00)* | 6.31 (0.00)* | 13.1 (0.00)* |
Discussion length | 1.03 (0.06) | 1.03 (0.01)* | 1.02 (0.06) | 1.02 (0.17) | 1.01 (0.45) |
Clicker question | 1.05 (0.42) | 0.94 (0.19) | 0.91 (0.05)* | 0.87 (0.02)* | 0.93 (0.11) |
Number of speakers | 2.25 (0.09) | 1.97 (0.04)* | 1.09 (0.78) | 1.31 (0.46) | 1.04 (0.91) |
Bloom’s level (high) | 1.28 (0.70) | 0.56 (0.24) | 0.45 (0.10) | 1.06 (0.92) | 1.23 (0.64) |
Random Call Has Minimal Impact on the Frequency of Characteristics within Discussions
To determine whether the characteristics within discussions differed between the two conditions, we coded each turn of speech and then summarized the frequency of each code’s use within discussions by dividing the incidence of its use by the turns of speech in that discussion (Table 6). Because this line-by-line coding was for each turn of speech, codes such as Exchange of Reasoning and Reasoning about Multiple Answers could not be scored, as they require more than one turn of speech. Otherwise, each line-by-line code overlapped with a whole-discussion code (Table 2); for Reasoning, any statement that could be construed as reasoning was coded in this category, including Requesting Feedback questions, since these are statements of reasoning followed by a question. This prevented any double coding of student utterances.
Line-by-line code | Volunteer call Average % (SD) within discussion | Random call Average % (SD) within discussion |
---|---|---|
Reasoning (including feedback questions) | 26 (13) | 19 (10) |
Requesting Information | 20 (13) | 17 (9) |
Requesting Reasoning | 13 (13) | 7 (4) |
Claim | 27a (17) | 16 (9) |
Background | 19 (11) | 12 (7) |
Acknowledgment | 15 (6) | 17 (10) |
Related comment | 24 (15) | 24 (12) |
Unrelated comment | 17 (10) | 13 (9) |
Most frequencies of individual codes within a discussion were similar between the two conditions. To determine whether the frequencies of each code were significantly different between the volunteer versus random call sections, we used a multiple linear regression model, with Bloom’s level of question, discussion length, and clicker-question number as additional covariates. Although all frequencies were normally distributed, only frequencies of Claims, Requesting Information, and Related Comments produced models with R2 values greater than 0.2, and met the conditions of homoscedasticity (which measures the homogeneity of the variance). Ultimately, only the Claims frequency was significantly impacted by the discussion conditions: Claims were used at a higher frequency in the volunteer call condition (β = 0.39; p < 0.001). Clicker-question ID also impacted the use of Claims (β = 0.27; p = 0.005).
The Pattern of Student Talk Is Variable among Groups and across Clicker Questions
In addition to measuring frequency of characteristics within discussions, line-by-line coding of each discussion also allowed us to search for possible reproducible patterns of student interactions. In general, we do not see evidence that student discussions routinely follow an exact pattern, even when the frequency of discussion characteristics used is similar. However, there are some sequences that are similar: for example, a discussion may begin with a series of claims followed by a reasoning statement, or a student question could be followed by background and then a reasoning statement. In Figure 1, we illustrate the sequence of exchanges for two different clicker questions. In Figure 1A, both the random and volunteer call sections had similar exchanges; in Figure 1B, the random call section discussions were similar to each other but different from those in the volunteer call condition. In Figure 1A, discussion had a similar sequence and frequency of interactions and similar total number of turns of speech and length of discussion. In Figure 1B, the random call group discussions used both more turns of speech and more time for their discussions than the volunteer call groups. The random call groups also used a high frequency of Comments, which, as described in Table 4, were more common on average across random call discussion. Despite these differences, the sequences of talk shown here do not appear to impact the performance of the students, as shown by the percent correct (by group) for each discussion.
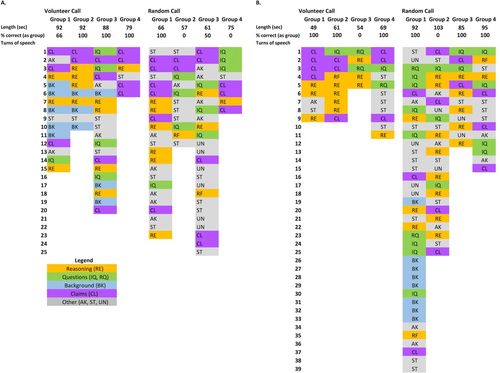
FIGURE 1. Order of student statements for discussions of the same clicker question by four different groups in each condition. Length of discussion and percent correct by group are shown for each discussion. Each color indicates a category of statements, with abbreviations for each code shown in each square. (A) Example in which discussions of a question were of similar length and turns of speech in both conditions. (B) Example in which discussions of a question were different: random call discussions took both more time and had more turns of speech than the volunteer call discussions. AK, Acknowledgments; ST, Related Comments; UN, Unrelated Comments.
Attitudes
Students were asked a series of 21 survey questions using a Likert scale of strongly disagree (1) to strongly agree (5) online at both the beginning and end of the semester. These questions were intended to capture opinions and feelings about such topics as willingness to work in groups, enjoyment of group work, willingness to ask teaching assistants for help, perceived value of clicker questions, and perceived value of group discussions. Table 7 shows the average student responses to the seven survey questions that were primarily about the value and format of group discussion. Students in each section agreed overall with the utility of providing reasons to one another during clicker discussions and with the value of group work. In addition, students in the random call section were asked about whether they took questions more seriously or learned more when they knew they might be called upon to explain their answer. Students on average were positive in their responses to these questions, both initially and at the end of the semester. There was no change from the beginning to the end of the semester in student attitudes in either section and no difference between attitudes in the volunteer and random call sections (Mann-Whitney U-test, p > 0.05).
Volunteer call (n = 40 students) | Random call (n = 90 students) | |
---|---|---|
Survey questions | Average rating (SD) | Average rating (SD) |
I usually learned more from a small-group discussion when I asked people to explain their thinking. | 4.2 (0.9) | 4.1 (0.8) |
I usually learned more from a small-group discussion when I explained my own thinking to the group. | 3.8 (1.1) | 3.9 (0.9) |
I probably got more questions right as a result of discussing them with a small group. | 4.2 (1.0) | 4.0 (0.8) |
In general, knowing how to work collaboratively is rewarded in the professional workplace. | 4.2 (0.9) | 4.3 (0.6) |
In general, students who work collaboratively in class are rewarded with higher grades. | 3.5 (1.0) | 3.4 (0.9) |
I take discussion time more seriously when my group has to be ready to contribute ideas to the whole class. | Not asked | 3.8 (0.8) |
I learn more from discussion if my group has to be ready to contribute ideas to the whole class | Not asked | 3.7 (0.8) |
DISCUSSION
Overall Conclusions
In this study, we characterized the elements of clicker-question discussions to determine whether the instructional practice of randomly calling on a group of students to share their ideas after discussion would result in different discussion characteristics than those elicited when volunteers were selected to share their ideas. We found that discussions in the random call condition were more likely to achieve a higher level of Exchange of Quality Reasoning, and were more likely to use both Feedback and Information types of questioning. In addition, discussions in the random call condition were more likely to use “fillers” such as one-word acknowledgments and short on- and off-topic comments. Closer examination of the frequency of discussion elements within a discussion indicated that individual discussions did not vary significantly except in their use of Claims, which was significantly less frequent in the random call compared with the volunteer call condition.
Discussion Time
Despite an on-average shorter time given by the instructor for discussions, students in the random call section still spent close to the same amount of time in discussion as did students in the volunteer call section. Discussion length was also a predictor of higher use of several characteristics generally associated with a valuable discussion (such as questioning and reasoning; Table 5), and longer discussions appear likely to be more productive overall. Because students in the random call section took more than the time allotted to them in 16% of the discussions, it seems possible that students in this section might have spent more time in discussion if they had been given more time, while in the volunteer call condition, students infrequently used the time allotted and never used more. It is possible that the lower-stakes nature of the volunteer call section demotivated discussion—that is, students were less likely to volunteer to share their answers and therefore did not require the full time given for discussions.
Performance
Performance on clicker questions does not appear to be affected by the two different classroom conditions. Given that there is no grade risk associated with incorrect answers in either condition, this is not surprising. Even if students have reasoned through different ideas and have a better understanding of the concepts they discussed, they may still fail to vote for the correct answer, as has been shown in previous studies (Knight et al., 2013, 2015). If credit for clicker-question responses depends on correctness, this higher level of accountability might affect clicker performance overall, although not necessarily differently in the two conditions of random and volunteer calling. In other studies in which group interdependence was emphasized, and in which students’ incorrect answers within a group penalized the group as a whole, students were more willing to collaborate with others and achieved an overall higher performance (Len, 2006; Sarfo and Elen, 2011). Grading incentives, in addition to affecting clicker performance measures, could also force a higher interdependence among group mates, potentially affecting their discussion characteristics.
There is evidence that collaboration on a task can impact student learning, particularly the challenging skill of developing an argument. For example, Sampson and Clark (2008) described several studies in which students who worked collaboratively to generate an argument were subsequently able to perform better individually on such tasks than those who did not work collaboratively. Osborne (2010) and Asterhan and Schwarz (2009) provide similar evidence that students are better able to engage in the process of communicating and exploring scientific arguments when they have practiced such skills collaboratively as opposed to individually. However, students do not naturally employ the process of logical reasoning and argumentation (Asterhan and Schwarz, 2007), and true conceptual change in student thinking may take considerably more time than typically given for in-class peer interaction (Asterhan and Schwarz, 2009). Although other studies have shown short-term positive effects of peer discussion (Smith et al., 2009) and the combined short-term positive effect of peer discussion and instructor explanation (Smith et al., 2011), demonstrating that classroom learning has a direct impact on longer-term measures of student learning is challenged by the large number of variables (amount of time spent studying, strategies used for studying, number of practice problems solved, level of motivation and engagement) that may impact performance. Each of these variables will need to be explored before we can understand how in-class discussions support long-term student performance and learning. Ultimately, to realistically measure the impact of student discussions on learning, one would likely need to measure reasoning and argumentation skills rather than mere performance on clicker questions.
Whole-Discussion Characteristics
Small differences in instructional practices have previously been shown to generate recognizably different classroom norms that can impact student impressions of instructor goals and student in-class behavior (Turpen and Finkelstein, 2009, 2010). The instructors of both courses described in our study used a “dialogic” approach in introducing clicker questions: students were asked to think about and make sense of concepts during learning through the instructor cue to discuss reasons for their clicker answers. Though there was a focus on reasoning with these cues, students in the volunteer condition were less likely to engage in as high a level of reasoning as students in the random call condition. Thus, even though the random call condition in our study is a much lower-stakes version of the accountability promoted by the social interdependence model (Johnson and Johnson, 2005), it appears to have triggered a higher likelihood of cooperation among students in a group than the volunteer call condition. Students who have a strong connection with their group mates may be more fully invested in understanding their peers’ answers; they may be more motivated to exchange ideas with the ultimate goal of being able to adequately report on their discussion in front of the rest of the class. It is also possible that the more frequent presence of filler comments in the discussions in the random call condition is related to students establishing a higher level of group identity and collaboration within their group. This could have led students to be more comfortable with one another, resulting in a higher likelihood of chatty behavior. Therefore, we suggest that the culture established by the practice of randomly calling on students to explain their ideas affects their likelihood of engaging in a discussion in which they fully explore the reasoning behind their ideas.
Within-Discussion Characteristics (Line-by-Line Coding)
In further exploring the characteristics of discussions by coding each student turn of speech, we found that discussions in the random call condition used significantly fewer claims but did not otherwise differ. A higher use of claims in a discussion often occurs when all students state their answer choices and then do little else to try to understand one another’s answers (see also Figure 1B, volunteer call discussions). It is possible that the volunteer call condition may have shifted the students into an “answer-making” mode, in which the focus was more on stating claims to ascertain the most commonly chosen answer rather than supporting any given answer with reasoning. This could occur if students felt there was no penalty to not engaging in productive discussion.
Our method of calculating code use based on the fraction of turns of speech may not accurately represent the actual time students spent in each type of interaction. For example, in the random call section, many student utterances were one-word comments, which would result in an overestimation of the fraction of the discussion spent on such comments. This could also explain why there is a somewhat higher (nonsignificant) frequency of reasoning statements in the volunteer call section than in the random call section, while, paradoxically, the Exchange of Quality Reasoning was significantly higher in the random call section. Together, these results suggest that the students in the volunteer call condition were frequently using short or incomplete reasoning statements, likely engaging more in behavior in which only one student offers reasoning, while those in the random call section were more likely exchanging fewer but longer, more complete statements of reasoning.
Finally, the line-by-line coding also showed that the sequence and frequency of exchanges during discussions may stem more from differences in clicker questions than differences in classroom norms. Clicker-question number was correlated with the frequency of claims used within a particular discussion (Table 5), suggesting that clicker questions vary in their ability to stimulate discussion, as also see in Figure 1 (compare discussions in A and B). In addition, as reported previously (Knight et al., 2015), the cognitive (Bloom’s) level of a clicker question is not correlated with any characteristics of student discussion, even though educators would expect that a more cognitively demanding clicker question would demand more or higher-level reasoning from students. In practice, the content of a question may be more inherently interesting or more challenging to a group of students regardless of what an instructor intended, or a group may just be in a mood that stimulates discussion. Many other variables not measured in this study, and perhaps not transparent to faculty or even students, could contribute to student discussion variation. What makes a question stimulating for students certainly bears further study.
Attitudes
Student responses to questions regarding their views on clickers and group discussions suggest that random call does not negatively impact student attitudes. Students who responded to the survey in both sections agreed that explaining their answers and asking for explanations from group mates would positively impact their learning. Students in the random call section also generally agreed that they were likely to be more serious and learn more under random call conditions compared with a scenario in which they might be asked to volunteer an answer, although they did not strongly agree with these statements. Although not all students responded to the attitude survey, those who did appear to view discussions with their neighbors as a practice that can have a positive impact on their learning, even when they may be put on the spot to explain themselves in front of the class. However, since we did not explicitly ask students if the random call made them uncomfortable, it is possible that they may not like the practice, even though they see its value. Chou and Lin (2015) showed that computer science students revealed such mixed feelings about collaboration. In their study, a system was used to assign students to discussion partners based on where they were sitting in class and used an accountability scoring mechanism that awarded students points based on the proportion of students within the group who answered the question correctly. Students were assigned to random seats each week, ensuring that their groups were regularly made up of different collaborators, and reported after each question whether or not they had collaborated. When students were prompted to form groups and collaborate with neighbors, they reported doing so 82% of the time; when not prompted, they reported collaborating only 60% of the time. Most students reported that the scoring system and the discussion prompting stimulated discussion; 79% liked the discussion prompting, but only 41% liked the collaborative scoring system. Thus, although the students did not mind discussing their ideas with others, they were not as positive about their grades reflecting their ability to come to consensus with other students. Thus, it is possible that implementing a grade-based accountability in addition to random call could have further impacted student attitudes, either positively or negatively.
Caveats
The discussions reported here are highly similar in their characteristics to previously characterized discussions of both introductory-level and advanced students (Knight et al., 2013, 2015). These similarities suggest that the experimental approach and the coding schemes developed can reliably capture student interactions during clicker discussions in biology courses. On the other hand, these discussions represent the interactions of a relatively small number of students who volunteered to be recorded in two sections of an introductory biology course at one university. Other groups of students who did not wish to be recorded may have exhibited different characteristics. Additionally, these characteristics could be unique to students in a large biology course or unique to this specific course. Other researchers are encouraged to use and adapt these coding schemes in other disciplines and/or with a wider range of students under varying conditions to determine whether these discussion characteristics are universal and whether group random call has a similar positive effect on student interactions.
Implications for Instruction
Creating a classroom norm for discussion accountability through random call, without introducing a grading incentive, can increase valued features of student discussions. Random call by group is likely less intimidating to students than calling on individual students and still generates student engagement. Group random call is also relatively easy to implement, even in a large classroom: instructors can use a variety of methods to call on groups, including die rolling, random number generators, the index card system described here, or a computerized version of the index card system. Giving students enough time to discuss clicker questions (at least 90 seconds) is also likely to result in a higher frequency of reasoning and questioning, as discussion length predicted an increased level of these features in this study.
There are additional benefits to using random call in the classroom: hearing from many different students, rather than just those who tend to volunteer, encourages all students to have a voice and respects the diversity of student thinking (Eddy and Hogan, 2014). Individual student random call also reduces gender gaps in student participation, since the participation is not voluntary—the random nature prevents one gender from being overrepresented (Eddy et al., 2014). Anecdotally, instructors also report that these conditions promote higher student attention and engagement in discussions, which has the potential to make a large classroom feel more equitable, intimate, and focused on learning. Creating additional positive interdependence among students by having a grade-related consequence for the efficacy of group work may further increase the use of these features. In group random call, there is the potential that a certain student within a group may be more likely to consistently be the reporter, thus potentially preventing each student from having a voice in the classroom. However, group random call without grade incentive also has the potential to allow students to benefit from group work without the fear of public speaking. For instructors who wish to pursue the route of additional grade accountability, several publications have suggested ways to reward interdependence while also giving students a chance to evaluate one another’s contributions (e.g., Kao, 2013; Jamal et al., 2014). Instructors can make personal decisions about how to balance grade accountability with the desire to promote a culture in which it is acceptable to be wrong and explore one’s ideas without fear of a negative effect on grades.
ACKNOWLEDGMENTS
This work was carried out with support from the National Science Foundation (DUE 1140789). We are grateful for excellent suggestions and assistance during this project from collaborator Erin Furtak; the members of our grant advisory board, Derek Briggs, Melissa Dancy, Noah Finkelstein, Laurel Hartley, and Valerie Otero; and our external evaluator, Sam McKagan. We also appreciate ongoing feedback from the discipline-based education research group of the University of Colorado. For help with data collection and transcription, thanks to then undergraduate assistants Erika Lai, Francis Li, Amedee Martella, Alex Meritt, Nick Myers, and Sarah Zimmermann. This work could not have been completed without the gracious support of instructors Nancy Guild and Jennifer Martin and the willingness of University of Colorado students to participate in this research.