Āwhina Revolution: A Bayesian Analysis of Undergraduate and Postgraduate Completion Rates from a Program for Māori and Pacific Success in STEM Disciplines
Abstract
Māori and Pacific students generally do not attain the same levels of tertiary success as New Zealanders of European descent, particularly in science, technology, engineering, and mathematics (STEM) subjects. Te Rōpū Āwhina (Āwhina), an equity initiative at Victoria University of Wellington in New Zealand between 1999 and 2015, aimed to produce Māori and Pacific professionals in STEM disciplines who contribute to Māori and Pacific community development and leadership. A hierarchical Bayesian approach was used to estimate posterior standardized completion rates for 3-year undergraduate and 2-year postgraduate degrees undertaken by non–Māori-Pacific and Māori-Pacific students. Results were consistent with an Āwhina effect, that is, Āwhina’s positive influence on (combined) Māori and Pacific success.
INTRODUCTION
New Zealand has experienced persistent ethnic disparities in tertiary1 academic success (Hunt et al., 2001; Coxon et al., 2002; Nikora et al., 2002; Levy and Williams, 2003; Scott, 2003; Benseman et al., 2006; Juhong and Maloney, 2006; Gorinski and Abernethy, 2007; Shulruf et al., 2008; Wilson et al., 2011; Theodore et al., 2016). The disparities are greater in science, technology, engineering, and mathematics (STEM) subjects, especially at the postgraduate level (New Zealand Government Ministry of Education, 2016a). In contrast the success of Māori (the indigenous people of New Zealand) and Pacific (New Zealanders descended from the indigenous peoples of Pacific Nations)2 STEM undergraduate and postgraduate students substantially improved under an initiative at Victoria University of Wellington (VUW).3 Te Rōpū Āwhina4 (Āwhina), active in the VUW STEM faculties between 1999 and 2015, was built around the Māori concept of whānau (literal meaning “extended family”). Whānau values of high expectations, achievement, aspirations, reciprocity, and collective success underpinned the Āwhina kaupapa (goal) of producing STEM professionals who would become leaders in Māori, Pacific, and other minority communities. Āwhina embodied many ideas suggested for reducing tertiary ethnic inequalities (Leggon and Pearson, 2009), with perhaps the closest U.S. example being the Meyerhoff Scholars Program (discussed in more detail later).
Using Āwhina survey data and VUW individual-level administrative student record data to investigate the impact of Āwhina whānau over its first 6 years, Wilson et al. (2011) found indications of an Āwhina “effect,” that is, the presence of Te Rōpū Āwhina was a positive influence on Māori and Pacific STEM success. This study was extended by Richardson et al. (2014), who found measurable improvements in STEM combined Māori-Pacific completions during the 1999–2010 period, an increase in the number of STEM Māori-Pacific completions relative to completions for all other STEM students (notably at the postgraduate level), and significant growth in STEM Māori-Pacific PhD completions. While similar dynamics were observed between 1999 and 2010 in the non-STEM faculties for Māori-Pacific completions, STEM Māori-Pacific numbers increased faster than all other STEM students as a whole, whereas non-STEM Māori-Pacific numbers increased at about the same rate as other non-STEM students.
This study generalizes earlier work by 1) measuring disparities using completion rates, a more appropriate measure of student performance than completions, because it includes the effect of study time for all students that attempt a particular qualification (thus, completion rates distinguish students who complete a qualification with different study times and add information from those who do not complete a qualification); 2) including analysis of completion rates at the postgraduate level, an area in which relative improvements in Māori-Pacific STEM success have proved elusive; and 3) using a hierarchical Bayesian (HB) regression approach to estimate ethnic-specific completion rates. Given the small number of completions and limited study time in some strata, for example, for students of Māori or Pacific ethnicity in STEM disciplines, HB methods provide significant advantages. This approach allows pooling of information across strata, and therefore some smoothing of posterior completion rates, by so-called “shrinkage” toward a prior covariate structure (Christiansen and Morris, 1997; Richardson et al., 2013). The shrinkage property was particularly useful in the present study, in which the median-year effect was of interest but its form uncertain, and where alternative forms (e.g., linear vs. spline) were harder to assess because of the computational effort required. Because studies of minorities often focus on small subsets of individuals, the HB method may be of general interest for such work. Other advantages of HB models are described in the Supplemental Material.
Several authors of this paper were long-standing members of Te Rōpū Āwhina whānau, and developing a robust evidence base to demonstrate effectiveness was a key element of Āwhina’s kaupapa. Thus, we hypothesized an Āwhina effect. To address this hypothesis, we used 1) Māori-Pacific completion rates in the STEM faculties as a proxy for the influence of Āwhina on Māori-Pacific success and 2) STEM non–Māori-Pacific and non-STEM Māori-Pacific completion rates as reference groups. Our specific objective was to evaluate whether completion rate dynamics were consistent with an Āwhina effect at the undergraduate and postgraduate levels.
Indigenous and Minority Disparities in Tertiary STEM Education
Evidence of an Āwhina effect would have particular significance for New Zealand, where equity in educational outcomes is weak, the level of income inequality is well above the Organisation for Economic Co-operation and Development (OECD) average, and the jobless poverty rate is high (OECD, 2015). Although the proportion of people with bachelor’s degrees or higher is increasing for all ethnic groups, the proportion for Māori (10%) and Pacific (9%) is lower than for non-Māori and non-Pacific New Zealanders (24%; New Zealand Government Ministry of Education, 2017). Similarly, tertiary participation rates for all ethnic groups are increasing (Marriott and Sim, 2015), but Māori and Pacific participation remains highest for nondegree qualifications (New Zealand Government Ministry of Education, 2016b). Moreover, relative to all students, greater proportions of Māori and Pacific students enrolled in bachelor’s degrees do not complete them (Meehan et al., 2017; New Zealand Government Ministry of Education, 2018). These disparities are even greater in STEM subjects, especially at the postgraduate level (New Zealand Government Ministry of Education, 2016a). Exacerbating the problem, Māori and Pacific populations are expected to grow more rapidly than the European population, with projected increases of 2.8, 2.4, and −9.1%, respectively, between 2013 and 2038 (Statistics New Zealand, 2017). Greater relative increases at younger ages are expected for both Māori and Pacific groups. Currently, Māori make up about 14.9% and Pacific 7.4% of the New Zealand (NZ) population (Statistics New Zealand, 2014). If the nation is to develop a highly skilled workforce to support predicted employment increases in high knowledge areas, this situation must change (McKinley et al., 2014).
Similar STEM disparities and population trends can be found elsewhere (for U.S. examples, see National Science Foundation, 2015; National Science Foundation’s National Science Board, 2016), and there is international recognition of the need to diversify the STEM workforce, with diversity acknowledged as bringing new perspectives to STEM disciplines (Robinson and Dechant, 1997; Page, 2008; National Academy of Sciences, National Academy of Engineering, and Institute of Medicine, 2011; O’Brien et al., 2015).
The national quantitative literature relevant to disparities in tertiary participation, retention, and success has typically used cross-sectional data sets aggregated across institutions: limited availability of good-quality individual-level data sets has also hampered research in this area (Koenig, 2009). However, some recent cohort studies based on NZ tertiary administrative student data have been published. Jia and Maloney (2014) used predictive risk models to determine factors that increase the risk of adverse student outcomes (noncompletion and nonretention) early in their studies at a large NZ university. A range of factors were found to influence outcomes, including part-time status, gender, and ethnicity. For example, Pacific students were most at risk of course noncompletion, and Māori students most at risk of nonretention. Scott (2005) used matched enrollment/completion data between 1998 and 2002 from publicly funded NZ tertiary institutions to estimate qualification completion rates (proportions) by demographic and other strata. Gender, ethnicity, and age were found to be statistically significant determinants, with Pacific students having the lowest completion rates across all qualifications. Similarly, Māori completion rates were low at the degree level or above. Juhong and Maloney (2006) reported that, for a cohort of students enrolled in arts and science degrees at an urban NZ university in 2000, Māori-Pacific students were significantly more likely to be enrolled in an arts degree (80% Māori; 74% Pacific) than Pākehā (New Zealanders of European descent) students (61%). Furthermore, during the period of the study, 57% of Māori and 62% of Pacific students dropped out compared with 40% of Pākehā. Poorer-performing students were more likely to drop out; and the lower their grade point average, the earlier this happened. Māori-Pacific students were more likely to drop out at any particular stage compared with Pākehā students and had mean grades 19 to 39% lower than Pākehā students after controlling for background.
There is also an extensive international literature that attests to historical inequalities in indigenous and minority student success in higher education and articulates reasons for those inequalities (Mullen, 2010; Stulberg and Weinberg, 2011; Wilson et al., 2011; Duncan and Murnane, 2014; Feagin and Feagin, 2014; Richardson et al., 2014; Slaughter et al., 2015). However, much of the national and international literature on tertiary participation, retention, and success focuses on student (rather than institutional) deficits (Brayboy and Castagno, 2008; Bang and Medin, 2010). In reality the educational experiences of indigenous and minority people are impacted by their social and cultural disconnection with tertiary institutions, and there is a growing literature directed at understanding the reasons for this disconnection and how tertiary institutions must change to reflect a diverse and rapidly changing student community (Hrabowski and Pearson, 1993; National Science and Technology Council, 2000; Hunt et al., 2001; Levy and Williams, 2003; Barker, 2007; Guillory and Wolverton, 2008; Makuwira, 2008; Trenor et al., 2008; Walters and Simoni, 2009; Bang and Medin, 2010; Biles and Biles, 2010; van der Meer et al., 2010; Kugelmass and Ready, 2011; Metz et al., 2011; Nakhid, 2011; Iverson and Jaggers, 2015).
Unfortunately, only a limited number of publications have evaluated initiatives aimed at reducing tertiary inequalities (BEST, 2004; Summers and Hrabowski, 2006; Maton et al., 2007, 2011; Koenig, 2009; Leggon and Pearson, 2009; Stolle-McAllister et al., 2011; Wilson et al., 2011; Ching, 2012; Ramirez, 2012; Brooks et al., 2013; Schroeder and Lazzell, 2013; Hrabowski, 2014; Richardson et al., 2014; Bernstein et al., 2015; Toven-Lindsey et al., 2015). This article aims to contribute to that literature.
Te Rōpū Āwhina
Wilson et al. (2011) noted that Āwhina was an on- and off-campus whānau that created a culturally relevant space within which all STEM students could flourish. It sought to empower young Māori and Pacific students by showing how they could achieve “success in two worlds,” that is, in their own communities and in STEM knowledge areas upon which the future well-being of their communities and, ultimately, New Zealand depends. The kaupapa of Āwhina was to foster Māori-Pacific development and leadership: however, it is important to note that Āwhina whānau members could be of any ethnicity.
From their first year at VUW, all whānau members were expected to strive for high grades, complete their degrees within the allocated time, and aspire to postgraduate studies. Whānau members were encouraged and assisted to 1) develop leadership skills and 2) understand their role as culture changers within the university, workplace, and community. To help cope with the demands of tertiary study, many had 1) accepted the responsibility of mentoring other students on campus (in their own discipline) or students off campus at Āwhina cluster secondary schools (equivalent to U.S. grades 8–12); 2) been exposed early to research by undertaking staff-supervised research projects funded by Āwhina; or 3) participated in the monthly Āwhina Postgraduate Seminar Series, during which postgraduates presented their research to the whānau. Although Āwhina had a limited budget, modest financial support was available for scholarships and awards, with Āwhina staff providing support for scholarship and grant applications when possible. The kaupapa and whānau values were realized through the high caliber and commitment of Āwhina staff; strong support from Āwhina whānau; broad autonomy to decide and implement the kaupapa; close connections between Āwhina whānau and communities, family, friends, faculty, and staff; seniority and permanence of the Āwhina leadership role; stable resourcing; and a robust evidence base. More details of how Te Rōpū Āwhina worked can be found in the Supplemental Material.
Several key attributes of Āwhina are seen in other successful initiatives for minority STEM students. Perhaps the most similar U.S. example in terms of structure, process, and empirical evidence is the Meyerhoff Scholars Program (Maton et al., 2011; Stolle-McAllister et al., 2011) at the University of Maryland, Baltimore County, which works collectively to foster a family-like environment and peer support for underrepresented minority students in STEM disciplines. It promotes excellence at the undergraduate level and encourages aspirations to complete STEM PhDs. The Meyerhoff Scholars Program has won national acclaim, having been the recipient of presidential and other awards, and the model is being replicated at other institutions (Gewin, 2014). The original program was limited to Black males (Hrabowski and Pearson, 1993) but is now open to all students committed to diversity in STEM and has been extensively studied (see Leggon and Pearson, 2009). Other examples include the University of Miami’s Science Made Sensible program (Lelliott et al., 2012), in which teachers and graduate students work together to increase middle-school student interest in science and improve the ability of graduate students to communicate science using, for example, peer mentoring and hands-on research experiences.
METHODOLOGY
Data and Analysis
Anonymized information from the VUW student database was used to estimate durations of study for qualifications since 1991. The VUW student management system contains data before 1991, but only since 1991 have most data items been collected and stored in a consistent manner. The student management system is the official record of academic achievement, including completed degrees. The database contains personal and demographic data, degree program(s), course, grade, enrollment, entry (direct entry from school, or special admission for adult students) and completion status5 for all VUW students. Ethical approval for the use of student records was sought and received from an institutional ethics committee (RM019438).
For this study, ethnicity is defined for Māori-Pacific and non–Māori-Pacific groups using a “total count” definition. Thus, the Māori-Pacific group included: Pacific or Māori only; Pacific and NZ European; Pacific and Māori; Māori and NZ European. All ethnicities recorded for a given student were used to compute that student’s total ethnicity. For example, a student who completed a qualification in 3 years might have reported up to nine different ethnicities: all were used to define the total ethnicity variable, that is, if any of the nine ethnicities were Māori or Pacific (and regardless of any other ethnicities reported) then the student was classified as Māori-Pacific.6 Strictly speaking, Māori-Pacific is not an ethnicity but is referred to as such in the interest of brevity.
This work focused on those students who studied for postgraduate qualifications expected to take 2 years (i.e., with an equivalent full-time study [EFTS] rating of 2) in STEM (e.g., master of science) and non-STEM (e.g., master of arts) disciplines and on students who studied for EFTS 3 undergraduate qualifications (e.g., bachelor of science, bachelor of arts). Using qualification-level (annual) data sets, we estimated the total duration of study for each qualification and whether it was completed or not. Gender and faculty group identifier (STEM or non-STEM) were also included in the study sample, because these factors may determine study success. Other variables such as entry qualification and decile7 of the last secondary school attended before tertiary study (a proxy for deprivation in adolescence) were excluded from the analysis data set. Such quantities lie on the causal pathway between ethnicity and successful completion of a degree, and the total effect (rather than direct or indirect effect) of ethnic group on tertiary completion rates was of primary interest.
For subsequent HB and empirical computation of postgraduate completion rates, durations of study (DoS) were derived from a longitudinal file containing an annual record of progress toward each qualification attempted by a student. To do this, we defined 5-year blocks starting in 1996 and finishing in 2014 (i.e., 1996–2000, 1997–2001, …, 2010–2014), henceforth identified by median years (1998, 1999, …, 2012). Then the DoS within each 5-year block for each qualification attempted was computed (in years) from the annual record, together with an indicator of whether the qualification was completed in that year block. In any given year block, students may have accumulated prior study time toward that qualification; and the more prior study time accumulated, the greater the likelihood of completing that qualification. Thus, prior duration of study (prior DoS or pDoS: 0, 1, 2, … years) for each year block was also calculated from the annual record of progress for each student enrolled in a qualification and classified into three groups (0, 1, and 2+years). Finally, completions and DoS for each qualification attempted were aggregated (over individual student records) by strata of ethnic group, gender, faculty group (of each qualification attempted), year block, and pDoS. The final aggregated data set used for HB postgraduate analyses consisted of total completions and DoS for EFTS 2 postgraduate degrees in strata of ethnic group (two levels), gender (two levels), faculty group (two levels), pDoS (three levels), and year block (15 levels, identified by median years: 1998, …, 2012).
The corresponding analysis for undergraduate completion rates used completions and DoS aggregated into 5-year blocks starting in 1995 and finishing in 2014. Year blocks were 1995–1999, 1996–2000, …, 2010–2014, identified by median years (1997, 1998, …, 2012). Prior duration of study was estimated for each student enrolled in a qualification for each year block and classified into five groups (0, 1, 2, 3, and 4+ years). The final aggregated data set for HB analyses of undergraduate completion rates consisted of total completions and DoS for EFTS 3 undergraduate degrees in strata of ethnic group (two levels), gender (two levels), faculty group (two levels), pDoS (five levels), and year block (16 levels, identified by median years: 1997, …, 2012).
To constrain lengthy computational times for HB models, we computed posterior completion rates from separate STEM and non-STEM data sets.
Prior Model Splines
Bayesian models with linear spline (Greenland, 1995) median-year terms in the prior model are reported here and, for brevity, are referred to as “spline models.” Preliminary regression and HB analyses suggested a linear spline median-year term in the prior model for postgraduate completion rates, with a knot around 2005 (STEM) or 2001 (non-STEM). For undergraduate completion rates, results are based on linear spline models with a knot around 2005 for STEM data, and linear models for non-STEM data. The prior model also included terms for gender, pDoS, ethnic group (all categorical), an interaction between ethnic group and (median) year, and autoregressive AR(1) errors. Apart from median-year effects in the prior model, the same final models were used for both STEM and non-STEM data sets.
All data were fitted in the R statistical environment (R Core Team, 2015) using jags (Plummer, 2003, 2014) and R2jags (Su and Yajima, 2012).
Hierarchical Bayesian Poisson Regression Model
Assuming postgraduate degree completion is a Poisson process such that, for time t(=1,...,15) and stratum j(=1,...,12) with completion ctj, completion rate λtj, and study time Stj, and using the notation x ~ D[a, b] to represent a random variable x distributed as D with mean a and variance b, a three-level Poisson model was defined by




Equation 1 links qualification completions (ctj) and completion times (Stj) in stratum tj of the analysis data set with the model-derived quantity λtj. Specifically, ctj is sampled from a Poisson distribution of mean and variance Stj ∙ λtj, which defines λtj as a completion rate. An empirical completion rate for the same stratum can be computed as ctj/Stj. The completion rate λtj had a gamma distribution with mean µtj and variance µtj2/ς , and the prior structural mean µtj depended on covariate vector xtj, parameter vector β, and autoregressive error term e through a log-link function (Eqs. 3 and 4). Second-level parameters, β (the regression “hyper-parameters”), ρ (the autoregressive parameter), ε (the autoregressive white noise process), and ζ (the completion rate variance or “shape” hyperparameter), were assigned independent prior distributions (“hyperpriors”) at the third level of the hierarchy (Eq. 5). Proper but noninformative prior distributions were used for each component of β, a common approach in Bayesian models (e.g., Gelman et al., 2004).
Given Eqs. 1 and 2, the observed completions ctj, conditional on hyperparameters β, ρ, ε, and ζ, have a marginal negative binomial distribution. However, the HB model goes further by allowing the prior covariate structure to influence the mean of the posterior rate, but with a degree of influence that depends on the overall support for the prior covariate structure in the data and on how much local information is available. Given the structure of the model defined by Eqs. 1 and 2, and conjugacy of the Poisson and Gamma distributions, the conditional posterior distribution for the completion rate is also gamma with mean

Thus, from Eq. 6, the conditional posterior mean for λtj is a weighted average of the prior structural mean µtj and the observed completion rate (ytj). The Btj (Eq. 7), which lie between zero and one, are known as shrinkage parameters, because larger values “shrink” the conditional posterior mean completion rates toward the prior structural mean. The gamma shape parameter ζ provides a measure of the influence of the prior structural mean. A relatively uninformative uniform shrinkage prior was adopted for ζ (Christiansen and Morris, 1997). The undergraduate completion rate HB model was identical, except for a different number of strata t (16) and j (20). A representation of the non-STEM undergraduate model in the jags language is provided in the Supplemental Material (see Supplemental Figure S2).
Completion Rates and Rate Ratios
To allow comparison across ethnic and faculty groups, we estimated stratum-specific posterior completion rates. Comparison of ethnic- and faculty-specific completion rates (reported below in the Results and Discussion sections as completions per year) was done by directly standardizing stratum-specific posterior rates to VUW DoS distributions in the 2012 year block, equivalent to using a (DoS) weighted average across strata that were not the focus of this analysis (gender and pDoS). This avoids bias from ethnic, faculty, and temporal differences in DoS distributions and follows the approach of Richardson et al. (2013). Standardized rate ratios were then computed from standardized rates and used to summarize differences in ethnic- and faculty-specific posterior completion rate distributions. For robust summarizations of posterior rates and rate ratio distributions, sample medians were used as the main estimate of centrality. The width of posterior distributions was quantified by 95% credible intervals (CIs).
RESULTS
Undergraduate Completions and Study Time
Figure 1 shows trends in total STEM (Figure 1, a and c) and non-STEM (Figure 1, b and d) undergraduate completions and study time. For Māori-Pacific students in the STEM faculties (Figure 1a), completions and study time increased steadily until about median year 2009, with more rapid increases thereafter. Similar overall trends were observed for non–Māori-Pacific students (Figure 1c). For the non-STEM faculties (Figure 1b), completions and study time increased steadily for Māori-Pacific students until median year 2010, followed by a decline in both measures. As for the STEM faculties, broadly similar trends were seen for non–Māori-Pacific students (Figure 1d). Not surprisingly, study time (roughly proportional to the total number of Māori-Pacific students) and completions were considerably smaller in the STEM faculties.

FIGURE 1. (a) Māori-Pacific (MP) STEM, (b) Māori-Pacific (MP) non-STEM, (c) non–Māori-Pacific (nMP) STEM, and (d) non–Māori-Pacific (nMP) non-STEM study time (years) and completion of qualifications (quals) for undergraduate EFTS 3 degrees in 5-year blocks having median years 1997–2012. Data points were computed by aggregating across gender and prior duration of study. Smooth trends were estimated using a generalized additive model. A crude estimate of the number of students per 5-year block can be obtained by dividing study time by 3, though there will be some double counting from students taking multiple degrees.
Undergraduate Completion Rates and Rate Ratios
Posterior and empirical standardized undergraduate completion rates and rate ratios for the STEM faculties from linear spline HB models are shown in Figure 2a together with standardized empirical rates. For the Māori-Pacific group, posterior median completion rates increased from 0.19/year (95% CI 0.17–0.20) in median year 1999 to about 0.24/year (95% CI 0.22–0.26) in median year 2005, while remaining roughly constant thereafter (at about 0.25/year). Māori-Pacific to non–Māori-Pacific median rate ratios (Figure 2b) increased from about 0.65 (95% CI 0.59–0.72) in median year 1999 to about 0.82 (95% CI 0.74–0.90) in median year 2005, and declined slightly thereafter reaching 0.78 in median year 2012 (95% CI 0.71–0.85).
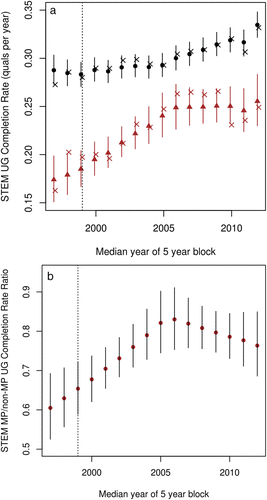
FIGURE 2. (a) Standardized empirical (crosses) and posterior completion rates for Māori-Pacific (triangles) and non–Māori-Pacific (circles) students taking STEM undergraduate (UG) EFTS 3 degrees. Posterior completion rates were estimated using an HB linear spline model. (b) Standardized posterior Māori-Pacific/non–Māori-Pacific (MP/non-MP) rate ratios for students taking STEM undergraduate (UG) EFTS 3 degrees. (a, b) Vertical lines on posterior HB completion rates are 95% CIs. The dashed vertical line indicates the start of Āwhina.
In the non-STEM faculties (Figure 3a), standardized posterior completion rates from the linear HB model were significantly lower for the Māori-Pacific group. However, trends were similar for both ethnic groups, with an increase in posterior completion rates until median year 2007, followed by a decrease. Completion rates for the non–Māori-Pacific group were about 0.28/year (95% CI 0.27–0.29) in median year 1999, 0.33/year (95% CI 0.32-0.33) in median year 2007, and about 0.28/year (95% CI 0.27–0.29) in median year 2012. The corresponding figures for the Māori-Pacific group were 0.18/year (95% CI 0.17–0.19), 0.22/year (95% CI 0.22–0.23), and 0.20/year (95% CI 0.19–0.21), respectively. There is little difference between empirical and posterior rates, and considerable nonlinearity in overall trend, suggesting less dominance by (i.e., shrinkage toward) the prior model than was the case for the STEM faculties. Given the larger total study times and completions in the non-STEM faculties, this is not surprising (see also Supplemental Figure S1, a and b, and associated discussion in the Supplemental Material). Māori-Pacific to non–Māori-Pacific rate ratios (Figure 3b) increased slightly between median years 1999 and 2012, from about 0.65 (95% CI 0.63–0.68) to about 0.71 (95% CI 0.69–0.74). Interestingly, improvement in the rate ratio toward the end of the time series arose because completion rates for the Māori-Pacific group declined more slowly than for the non–Māori-Pacific group.
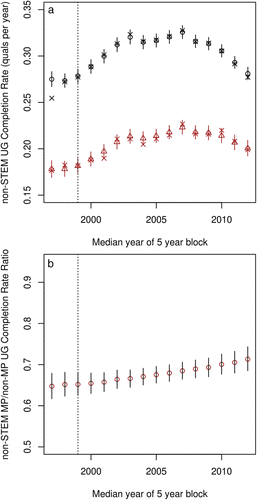
FIGURE 3. (a) Standardized empirical (crosses) and posterior completion rates for Māori-Pacific (triangles) and non–Māori-Pacific students (circles) taking non-STEM undergraduate (UG) EFTS 3 degrees. Posterior completion rates were estimated using an HB linear model. (b) Standardized posterior Māori-Pacific/non–Māori-Pacific (MP/non-MP) rate rate ratios for students taking non-STEM undergraduate (UG) EFTS 3 degrees. (a, b) Vertical lines on posterior HB completion rates are 95% CIs. The dashed vertical line indicates the start of Āwhina.
Posterior rate ratios suggest a steady increase in STEM Māori-Pacific completion rates relative to the non-STEM faculty group (see Figure 4) between median years 1999 (1.02, 95% CI 0.91–1.14) and 2012 (1.27, 95% CI 1.14–1.43).

FIGURE 4. Standardized posterior STEM Māori-Pacific/non-STEM Māori-Pacific (MP) rate ratios for students taking undergraduate (UG) EFTS 3 degrees. STEM posterior completion rates were estimated using an HB linear spline model; non-STEM posterior completion rates were estimated using an HB linear model. Vertical lines on posterior HB completion rates are 95% CIs. The dashed vertical line indicates the start of Āwhina.
Postgraduate Completion Rates and Rate Ratios
Posterior and empirical standardized completion rates and rate ratios for the STEM faculties from HB models are shown in Figure 5a. For the Māori-Pacific group, posterior median completion rates increased from 0.20/year (95% CI 0.12–0.33) in median year 1999 to about 0.35/year (95% CI 0.27–0.45) in median year 2005, while remaining roughly constant thereafter (at about 0.35–0.37/year). Māori-Pacific to non–Māori-Pacific median rate ratios (Figure 5b) increased from about 0.63 (95% CI 0.37–1.07) in median year 1999 to about 0.92 (95% CI 0.70–1.19) in median year 2005, and declined slightly thereafter to 0.85 in median year 2012 (95% CI 0.66–1.09).
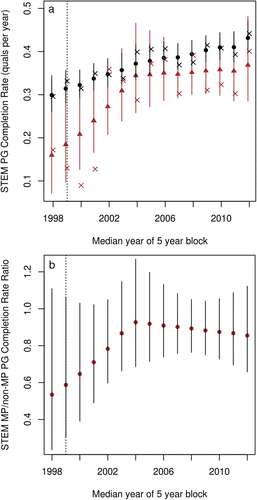
FIGURE 5. (a) Standardized empirical (crosses) and posterior completion rates for Māori-Pacific (triangles) and non–Māori-Pacific students (circles) taking STEM postgraduate (PG) EFTS 2 qualifications. Posterior completion rates were estimated using an HB linear spline model. (b) Standardized posterior Māori-Pacific/non–Māori-Pacific (MP/non-MP) rate ratios for students taking STEM postgraduate EFTS 2 degrees. (a, b) Vertical lines on posterior HB completion rates are 95% CIs. The dashed vertical line indicates the start of Āwhina.
In the non-STEM faculties (Figure 6a), standardized posterior completion rates were similar in magnitude for both ethnic groups in median year 1998 but declined between 1998 and 2002, with Māori-Pacific rates declining faster. Thereafter, posterior completion rates increased, but more slowly for the Māori-Pacific group. Completion rates for the non–Māori-Pacific group were about 0.30/year (95% CI 0.28–0.31) in median year 1999, 0.28/year (95% CI 0.26–0.30) in median year 2002, and about 0.42/year (95% CI 0.39–0.44) in median year 2012. The corresponding figures for the Māori-Pacific group were 0.29/year (95% CI 0.26–0.32), 0.22/year (95% CI 0.20–0.25), and 0.29/year (95% CI 0.26–0.33), respectively. Māori-Pacific to non–Māori-Pacific rate ratios (Figure 6b) declined steadily over the time series, from about 0.98 (95% CI 0.88–1.09) in median year 1999 to about 0.70 (95% CI 0.62–0.80) in median year 2012.
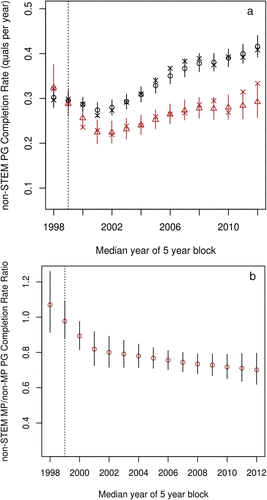
FIGURE 6. (a) Standardized empirical (crosses) and posterior completion rates for Māori-Pacific (triangles) and non–Māori-Pacific students (circles) taking non-STEM postgraduate (PG) EFTS 2 degrees. Posterior completion rates were estimated using an HB linear spline model. (b) Standardized posterior Māori-Pacific/non–Māori-Pacific (MP/non-MP) rate rate ratios for students taking non-STEM postgraduate EFTS 2 degrees. (a, b) Vertical lines on posterior HB completion rates are 95% CIs. The dashed vertical line indicates the start of Āwhina.
Posterior rate ratios suggest STEM Māori-Pacific completion rates relative to the non-STEM faculty group (see Figure 7) increased between median years 1999 (0.64, 95% CI 0.33–1.11) and 2004 (1.43, 95% CI 1.06–1.63), fell slightly until median year 2007 (1.27, 95% CI 1.05–1.53), and then remained roughly constant (1.26, 95% CI 0.95–1.66 in median year 2012).

FIGURE 7. Standardized posterior Māori-Pacific (MP) STEM/non-STEM completion rate ratios for students taking postgraduate (PG) EFTS 2 degrees. Posterior completion rates were estimated using HB linear spline models. Vertical lines on posterior HB completion rates are 95% CIs. The dashed vertical line indicates the start of Āwhina.
DISCUSSION
Undergraduate
In the non-STEM faculties, the temporal trend in Māori-Pacific undergraduate completion rates was similar to that of non–Māori-Pacific students, namely, an increase until median year 2007 followed by a decrease, but magnitudes were smaller for Māori-Pacific students. According to rate ratio estimates, non-STEM disparities reduced slowly. However, in the STEM faculties, Māori-Pacific completion rates increased faster than non–Māori-Pacific completion rates until about median year 2005, after which they plateaued at approximately 0.25/year, while non–Māori-Pacific rates continued to increase. Māori-Pacific to non–Māori-Pacific rate ratios reflected these different trends.
Completion rate dynamics appear to fall into two periods bounded by median years 2005 and 2007. Before median year 2005, posterior completion rates increased for both faculty and ethnic groups, but Māori-Pacific posterior completion rates increased faster than for the non–Māori-Pacific group, particularly in the STEM faculties. After median year 2007, posterior completion rates in the non-STEM faculties began to fall for both ethnic groups, though a little more slowly for the Māori-Pacific group. In the STEM faculties, non–Māori-Pacific completion rates continued to increase, and while Māori-Pacific completion rates remained relatively constant, they did not fall as in the non-STEM faculties.
The dynamics of undergraduate completion rates revealed in this analysis were presumably responsive to factors internal and external to the university. Before median year 2005, such factors combined to raise completion rates in all faculty and ethnic groups. After 2007, this was not the case. Considering external factors first, non-STEM completion rates may have fallen as a consequence of societal and institutional responses to the 2008 financial crisis. For example, pressure on household income/wealth or concerns about future income/wealth may have forced students to pursue paid income while studying, thereby decreasing completions and/or increasing study time. Similarly, the adoption of more stringent minimum grade rules for continuation of study (or more strict enforcement of existing rules) could have had the same effect. However, no decline in completion rates was seen for non–Māori-Pacific or Māori-Pacific students in the STEM faculties, so responses to the financial crisis cannot be the whole story.
There have also been repeated signals to tertiary institutions by the current NZ government (elected in 2008) to become more responsive to the needs of employers and the economy, and addressing shortages in science-related disciplines would fall within this mandate (New Zealand Government, 2014, 2015). This and the consequent media coverage might have encouraged students to enroll in STEM rather than non-STEM degrees, perhaps contributing to the observed enrollment patterns in STEM faculties after 2009. However, enrollment is only part of the completion rate story. Increasing STEM completion rates requires degree completions to outstrip study time, and the reverse is true with respect to decreasing completion rates: not just more students but different types of students (e.g., better prepared) are needed. Thus, if the proportion of better prepared students choosing to take STEM rather than non-STEM courses changed over time, STEM and non-STEM completion rate trends might change in the direction observed. Nevertheless, reconciling such an effect with observed trends would require careful “tuning,” including ethnic-specific differences. In the absence of further evidence, the hypothesis of government influence on student enrollment can also only be part of the post 2005–2007 completion rate story.
Turning to internal factors, an Āwhina effect provides a relatively simple explanation for why Māori-Pacific completion rates in STEM faculties overtook those in more traditional areas of study (non-STEM) and why there was a significant reduction in disparities within the STEM faculties, particularly before 2005. However, while an Āwhina effect provides a plausible explanation for the dynamics of completion rates in the STEM faculties, the possibility of a national relative improvement in STEM Māori-Pacific undergraduate completion rates cannot be ruled out a priori. Further research is required to determine whether the hypothesis (of an Āwhina effect) is consistent with analyses that use a different reference group, such as EFTS 3 STEM degrees at other NZ tertiary institutions.
The observed slow increase in non-STEM Māori-Pacific completion rates relative to the non-Ma¯ori-Pacific group is probably not attributable to inadequate levels of resourcing aimed at improving Māori-Pacific tertiary success. Since 2000, all NZ Universities received government grants to improve outcomes for Māori and Pacific students. At Āwhina’s host university, these grants were allocated to initiatives in non-STEM faculties and central services. In contrast, Āwhina was excluded from this funding as a pre-existing program and was wholly supported by the STEM faculties at a lower level of funding. Since 2011, some non-STEM equity initiatives have also received substantial funding from levies paid by all full-time students for services not covered by university tuition fees.
Postgraduate
In the non-STEM faculties, postgraduate completion rates for Māori-Pacific and non–Māori-Pacific students decreased from 1998 until 2002 and then increased, but Māori-Pacific rates increasingly fell behind those of their non–Māori-Pacific counterparts, that is, ethnic disparities increased. In contrast, there was a general trend of increasing completions rates in the STEM faculties and decreasing ethnic disparities.
As in the undergraduate EFTS 3 case, an Āwhina effect provides a relatively straightforward explanation for the reduction in postgraduate disparities within the STEM faculties, but further research is required to confirm its existence. In the postgraduate case, there was little evidence that the 2008 recession and consequent changes in institutional settings had much impact on completion rates in either faculty group. Perhaps postgraduate students are more resilient to such factors, but this hypothesis will require further investigation, which may prove challenging. For example, to increase study time and completions within strata of ethnicity, this analysis combined Māori and Pacific students into one group, obscuring subgroup heterogeneity effects that are probably important. The heterogeneity problem may be worse for the Pacific group, because it is an amalgam of distinct Pacific ethnicities (e.g., Samoan, Cook Island Māori, Tongan, Niuean, and Fijian) whose educational status and history is likely to vary, particularly because some were born overseas and some will be overseas students. In the postgraduate sample used here, Pacific students were underrepresented, suggesting that ethnic heterogeneity may be less of a methodological challenge than at the undergraduate level: Pacific students constitute about 0.7% of the total STEM student population who started their postgraduate degrees in 2010, whereas Māori represent 8.1% of the same group. Unfortunately, low Pacific student numbers in the sample also mean that exploration of Pacific-specific postgraduate completion rate dynamics will be more difficult.
Conclusions and Future Prospects
The results presented here are consistent with a significant Āwhina effect at both undergraduate and postgraduate level. The Āwhina kaupapa, dedication of whānau members, and modest additional income from external contracts and sponsorships may have enabled Āwhina to meet the needs of increasing Māori-Pacific whānau numbers despite limited resources. In the authors’ view, national and international evidence is by now sufficiently strong to justify extending the program to other faculties and other NZ tertiary institutions in a systematic and strategic manner. Doing so in accord with the kaupapa and whānau values of Āwhina, while resourcing for success and rigorously evaluating progress, would provide useful additional tests for an Āwhina effect.8 In contrast, the ad hoc introduction of inadequately evaluated equity initiatives are unlikely to be useful: international evidence, including from Āwhina itself, suggests that promising initiatives are hard to build and sustain but easy to dismantle (National Academy of Sciences, National Academy of Engineering, and Institute of Medicine, 2011; Allen-Ramdial and Campbell, 2014).
What, then, is the way forward for university equity programs? Confirmation of an Āwhina effect would have important lessons about the culture of tertiary institutions, their cultural and social disconnections with indigenous and minority students, and their social obligations and responsiveness. For example, researchers have previously noted that high-level institutional support, and the caliber, commitment, continuity, longevity, and seniority of equity program leaders are critical for the long-term sustainability of initiatives like Āwhina (National Academy of Sciences, National Academy of Engineering, and Institute of Medicine, 2011; Maton et al., 2012). It would also reflect and reinforce empirical evidence for other factors documented by research to be important in reducing inequalities in tertiary (STEM) success, such as mentoring (Maton et al., 2011) and reciprocity (Smith et al., 2014). Leggon and Gaines (2017) summarized lessons learned from a number of promising STEM equity programs (including Āwhina). These include commitment to social justice of all relevant stakeholders; clear communication of the purposes, goals, and objectives of equity programs to all stakeholders; the development of partnerships between program staff and others both inside and outside the host institution; linkages between and among institutions aimed at diversifying the STEM workforce; a transformative pedagogy that shows learners that science is relevant to their lives and changes perceptions of who does science; developing and nurturing supportive communities of scholars and families (e.g., via mentoring); robust evaluation to provide strong evidence for program effectiveness; and continued research on effective or promising policies, programs, practices, and strategies that seek to diversify and enhance the STEM workforce. Richardson et al. (2017) argued that, given that promising initiatives and methods for their evaluation already exist, national policy responses might also be required. These include significant financial penalties for institutions that fail to reduce disparities; provision of long-term external funding for equity initiatives having robust evidence of success; a requirement that institutions evaluate the success of equity initiatives using best practices methods and peer review by independent external experts; dedicated funding for research aimed at improving equity initiatives and methods of evaluation; and public funding for international conferences tasked with sharing experiences and lessons from promising programs, methods of evaluation, and training of future leaders.
Limitations
Completion rates were based on annual summaries of individual-level student data that might be improved by using course-level data but are not expected to introduce significant bias into aggregated estimates of completion rates. The non-STEM faculties have been used as a reference group against which to assess the performance of Māori-Pacific tertiary students over time, and in particular to test the hypothesis of an Āwhina effect. Such a reference group has advantages, such as being within the same institution, but also disadvantages, such as different degrees, with students potentially having different characteristics that might influence outcomes. Future research should use a national tertiary student record data set to check the impact of different reference groups. As noted earlier, this work has not probed the impact of ethnic heterogeneity, and gender/discipline effects are also likely to be important within the Māori-Pacific group. Furthermore, the analysis is not causal in the sense of Pearl (2009) or Robins and Hernán (2009), and research using such methods is needed. For example, causal paths through socioeconomic status might be expected to contribute significantly to the overall effect of ethnicity on postgraduate success, so estimating the relative importance of direct and indirect pathways between ethnicity and tertiary postgraduate success, perhaps using the counterfactual approach discussed by VanderWeele (2015), would be of considerable interest. However, dedicated (and expensive) large-scale longitudinal surveys coincident with promising programs will likely be required to estimate causal effects, and defining membership of initiatives like Āwhina will be methodologically challenging. Of course, more complex causal models have their own limitations, particularly for sparse data sets, and there is still a place for the simpler noncausal approaches based on administrative data sets used here.
Despite these limitations, this research contributes to the limited but growing literature on inequities in STEM disciplines (Leggon and Gaines, 2017). Even though millions of dollars have been spent on U.S. STEM intervention programs, few have been rigorously evaluated (see BEST, 2004; Leggon and Pearson, 2009; U.S. Government Accounting Office, 2012), though private foundations and federal agencies have begun requiring such programs to be evaluated, especially by a third party.
FOOTNOTES
1Tertiary institutions in New Zealand include universities, polytechnics, technical institutes, and wānanga (Māori tertiary institutions). Postgraduate degrees are mainly offered by universities, which also offer academic (as opposed to vocational) undergraduate and postgraduate degrees.
2The six largest Pacific ethnic groups living in New Zealand are Samoan, Cook Islands, Tongan, Niuean, Fijian, and Tokelauan.
3Victoria University of Wellington, one of eight universities in New Zealand, is located in New Zealand’s capital city of Wellington. The Wellington region has a population of around 470,000 of which 8% are Pacific and 13% Māori (Statistics New Zealand, 2013). Approximately 19,700 students attend VUW; 79% identify as European, 11% Māori, 6% Pacific, 13% Asian, and 5% as “other” (Tertiary Education Commission, 2017). Note that these ethnic categories are not exclusive. The university has 10 faculties, including science, engineering, architecture, and design, referred to hereafter as the SEAD faculties, which cover STEM and STEM-related disciplines. Non-SEAD faculties include law, humanities and social sciences, education, and business. Each faculty contains at least one discipline-specific school. In SEAD, the schools (with their respective faculty given in brackets) are 1) architecture; design (faculty of architecture and design); 2) engineering and computer science (faculty of engineering); 3) biological science; chemical and physical sciences; geography, environment, and earth sciences; mathematics and statistics; psychology (faculty of science).
4Translated as Te Rōpū (the group) Āwhina (support) whānau (family)—in other words, Te Rōpū Āwhina in the STEM faculties is the STEM-supportive family.
5A completed degree is one for which academic requirements have been met and that has been audited by an authorized VUW staff member.
6For most official reporting purposes, students are counted as Māori in a particular year if any of their recorded ethnicities are Māori, and as Pacific if any of their recorded ethnicities are Pacific ethnicities; i.e., Māori and Pacific are not treated as distinct groups. The collection of ethnicity data in this manner is a government reporting requirement, and the NZ Ministry of Education and the NZ Tertiary Education Commission use this method of counting and reporting on Māori and Pacific students.
7The decile rating indicates the extent to which a school draws its students from low socioeconomic communities: low decile schools have higher proportions of students from low socioeconomic communities.
8After the retirement of the Deputy Dean (Equity) in December 2015, Āwhina was replaced by an institutional “support” program based on those in the non-STEM faculties. In effect, Āwhina was replaced by a program having no evidence of success. When sufficient post-Āwhina data have accumulated, an analysis of STEM/non-STEM completion rates would strengthen evidence for an Āwhina effect but will require easier access to data from individual universities.
ACKNOWLEDGMENTS
We acknowledge past and present staff in the Āwhina faculties, the late Sir Paul Callaghan, and the MacDiarmid Institute for Advanced Materials and Nanotechnology. This work would not have been possible without the strong commitment of all Āwhina whānau members. We acknowledge and thank them for their significant contributions to Āwhina. We also thank Cheryl Leggon, Hazel Phillips, and Andrew Tarr for their help with earlier drafts of this paper.