Identifying Troublesome Jargon in Biology: Discrepancies between Student Performance and Perceived Understanding
Abstract
The excessive “jargon” load in biology may be a hurdle for developing conceptual understanding as well as achieving core competencies such as scientific literacy and communication. Little work has been done to characterize student understanding of biology-specific jargon. To address this issue, we aimed to determine the types of biology jargon terms that students struggle with most, the alignment between students’ perceived understanding and performance defining the terms, and common errors in student-provided definitions. Students in two biology classes were asked to report their understanding of, and provide definitions for, course-specific vocabulary terms: 1276 student responses to 72 terms were analyzed. Generally, students showed an overestimation of their own understanding. The least accurate self-assessment occurred for terms to which students had substantial prior exposure and terms with discordant meanings in biology versus everyday language. Students were more accurate when assessing their understanding of terms describing abstract molecular structures, and these were often perceived as more difficult than other types of terms. This research provides insights about which types of technical vocabulary may create a barrier to developing deeper conceptual understanding, and highlights a need to consider student understanding of different types of jargon in supporting learning and scientific literacy.
INTRODUCTION
Scientific communication is a core competency of biological literacy (American Association for the Advancement of Science, 2011; National Research Council, 2012), underscoring the importance of effective teaching of the language of biology. To achieve scientific literacy and successfully communicate about concepts in biology, one must master (understand and effectively use) the discipline-specific vocabulary (National Academies of Sciences, Engineering, and Medicine, 2016). Hence, learning in science requires not only developing an understanding of the concepts and mastery of skills, but it is also about learning the language of the discipline.
Despite this widespread agreement about the importance of learning discipline-specific language, the importance of language in learning science is often overlooked (Wellington and Osborne, 2001). The role of language may be framed within Vygotsky’s seminal claims: that thoughts require language and language requires thought (1986). Given that thought is required to learn, it naturally follows that language is absolutely required for learning. In this way, learning both conceptual understanding and language are intertwined (Wellington and Osborne, 2001). Early in learning, novices may use the language that experts use, but without the same depth of meaning or understanding behind the words. Increased experience with concepts leads to a deepening of one’s understanding of the concept, and as such, the depth and breadth of the meaning of language associated with the concept also grows (Howe, 1996). If, however, conceptual understanding does not grow, language comprehension will also stagnate. Because of the tight connection between learning and language, conceptual understanding may accordingly suffer when language learning is not supported. We therefore aim to explore this commonly acknowledged but understudied relationship, seeking to understand how technical language learning can impact student conceptual learning. This study investigates types of technical vocabulary that may be a barrier to student understanding, based on either the students’ perceived lack of understanding of the terms or their poor performance at defining these terms.
Correctly using such technical, discipline-specific vocabulary may be a particularly prominent hurdle in undergraduate biology. These courses are notorious for the vast quantity of terms introduced: often as many or more than in a high school language course (Wandersee, 1988; Groves, 1995). Much of this vocabulary is technical, unintuitive, ambiguous, or abstract, and so it may be considered “jargon,” especially by a novice in the field. It is important to emphasize here that jargon is a necessary part of science discourse in postsecondary education. However, if much of the discipline-specific technical vocabulary we are using is found to be unintuitive, ambiguous, or generally difficult to understand (jargon), then this jargon may, at times, be a barrier to the development of sound conceptual understanding and the development of strong scientific literacy skills (Snow, 2010).
The types of technical vocabulary presented and used in typical biology classes include terms that are so specialized they would not be seen outside the field (such as epitope), as well as terms that are used (differently) in everyday language (such as model). The complexity of learning concepts and the language needed to communicate about those concepts must not be overlooked if we are to improve students’ scientific literacy skills. Previous studies suggest that understanding the meaning of words is associated with developing a deep vocabulary knowledge (Schmitt, 2008), which will facilitate one’s ability to use the words effectively and appropriately in communication. In an effort to focus classroom time on concepts and higher-order thinking and problem solving (such as analysis, application of knowledge, evaluation of ideas), learning and mastering vocabulary may have become a lower priority in many undergraduate biology classrooms, potentially leading to deficits in understanding and negative impacts on scientific literacy. Research on second-language learners, as well as elementary school students’ learning of science vocabulary, has revealed interesting insights into how people learn the language of science. For example, developing an understanding of words and terms may be enhanced by forming connections between words and concepts. Studying second-language learners, Gu and Johnson (1996) found that, when students took a “meaning-oriented” approach to learning new words and employed metacognitive strategies, they had significantly better language-learning outcomes compared with students who relied heavily on memorizing terms. Rosen et al. (2012) found that presenting abstract words in conjunction with an image and the word used in a context sentence resulted in better transfer of students’ understanding of the word on a posttest compared with when students learned the word without the image and context sentence. These studies highlight some of the challenges associated with learning discipline-specific terms.
Others have shown that there is a decrease in conceptual learning and performance when students are introduced to or are interpreting jargon-heavy material (Cassels and Johnstone 1983, 1984; Brown and Ryoo, 2008; McDonnell et al., 2016). This may relate to cognitive load theory, relating to the capacity of working memory (Sweller, 1988): trying to simultaneously learn new vocabulary and new concepts may overwhelm the working memory and thus reduce learning in both areas. A similar effect has been seen in other studies in which students were ineffective at learning problem-solving skills and concepts in aggregate (Navon and Gropher, 1979; Sweller, 1988).
Despite the potential negative impacts of a heavy “jargon load” in biology, little work has investigated student learning of biology-specific vocabulary, such as identifying terms with which students struggle most. Our previous work showed that delaying the introduction of jargon until after the first exposure to a new topic can result in improved undergraduate biology student learning of concepts (McDonnell et al., 2016; also investigated by Brown and Ryoo [2008] in elementary school students). However, a broader picture of the general categories of jargon in biology (e.g., processes, molecular terms, terms with dual meanings) and what challenges these categories pose to students is unclear. Many jargon terms are simply unintuitive, essentially a completely different language for students to learn. Other vocabulary may rely on the transfer of knowledge from other disciplines, further complicating the learning and use of some terms. For example, several terms in cell and molecular biology presume a familiarity with chemistry (e.g., “hydrophobic” and “pyrimidine”), which can be a barrier to student learning; even if chemistry is a prerequisite course, we may not be able to assume students have mastered that vocabulary. Aside from this unintuitive vocabulary, students may also misunderstand terms that have context-dependent meanings. For example, terms that have meanings in everyday (non–biology specific) language that differ from their use in science, such as “adapt,” “fitness,” and “significant,” are often described as incompatible ambiguity terms (Ryan, 1985; Marshall et al., 1991; Rector et al., 2013). Select terms also have slightly different meanings among science, technology, engineering, and mathematics (STEM) disciplines, adding another layer of confusion about the true meaning and use of these terms (Kouba, 1989). These confusions may impede a student’s ability to learn biology-specific concepts that rely on those terms (Rector et al., 2013) and may possibly lead to the formation of misconceptions about these concepts. There may also be a tendency for students to erroneously assume they have already developed an understanding of various terms, particularly those that are familiar from previous courses. Such assumptions could result in an overestimation of understanding (Rozenblit and Keil, 2002). If students have not previously developed a clear understanding of the “familiar” jargon terms, they may lack the metacognitive abilities to recognize their misunderstandings and address such deficiencies (Kruger and Dunning, 1999). These familiarity problems may add to the possible barriers for developing a proper understanding of the terms and the concepts associated with them. Therefore, to support learning of biology-specific vocabulary and concepts, it is necessary to pay attention to student understanding of different types of vocabulary to facilitate student learning of the concepts and language of biology.
The goal of this study was to improve our understanding of the types of technical vocabulary (jargon) that may be most troublesome for undergraduate biology learners. To do so, we aimed to answer three questions by surveying students in two large biology courses: 1) What types of technical vocabulary terms do students struggle with most (e.g., familiar, abstract, multiple-meaning terms)? 2) What is the degree of alignment between students’ perceived understanding and performance at defining the terms? 3) Are there common errors in student-provided definitions of various biology technical vocabulary terms? To approach these questions, we assessed students’ perceived understanding and the correctness of student-provided definitions of biology-specific terms presented in second-year (sophomore) undergraduate biology courses using a voluntary online survey. We hypothesized that students would overestimate their understanding of terms that were familiar or that had meanings in both biology and everyday language. We also hypothesized that the most common errors would be inaccurate definitions of the terms. To investigate our questions, we first quantified students’ perceived understanding of 72 different biology-specific terms that belonged to multiple categories we defined in consultation with the course instructors (e.g., Molecular terms and Incompatible Ambiguity terms). We then investigated relationships between perceived understanding and correctness of student-provided definitions. The findings of this research provide insights to inform teaching of course material that involves these jargon terms and categories of terms and pose further research questions related to students’ learning of discipline-specific vocabulary.
METHODS
Course Context, Vocabulary, Survey Design, and Participants
Participants in this study were enrolled in one of two sophomore undergraduate biology courses (Cell Biology and Genetics) at a large research university in the Pacific Northwest in Fall 2014. The broad goals of these two courses were to relate structure to function for the major cell systems (both courses); to illustrate how cellular systems interact in complex processes (Cell Biology); and to examine fundamental genetic principles such as mutation, phenotype, segregation, linkage, complementation, and gene interaction (Genetics). There were no vocabulary-specific study strategies or assessments provided in these courses to improve scientific language development. Students were not provided with vocabulary lists in these courses; they would have been introduced to relevant terms through preclass reading assignments and/or and in class. The terms were further used by the instructor in examples and answers to problems, as well as in homework problems. The exams in these courses required students to apply their knowledge to solve problems and articulate their understanding of concepts through their problem solutions. Students were required to analyze data, and exams were mostly constructed-response questions. Questions were rarely at the recall level (e.g., students were not asked to define terms on exams). Rather, students were expected to know the meaning of the various terms used in the course so they could recognize them when used in questions and to use terms appropriately in their written response answers to homework and exam questions. If students used question-relevant technical vocabulary incorrectly in written responses to homework and exam questions, they lost points for using technical vocabulary incorrectly.
To investigate types of discipline-specific vocabulary in these two courses, we compiled vocabulary terms used in the courses in consultation with the course instructors. All terms had been introduced and used in the courses surveyed, and students were expected to be familiar with, know the meaning of, and use these terms appropriately by the end of the course (examples in Table 1, full list in Supplemental Table S1). In total, 76 terms were compiled.
Category | Number of terms | Definition | Example terms |
---|---|---|---|
Incompatible Ambiguity | 20 | The term has a use in everyday English vernacular as well as in biology. | Adaptation, template, model |
Information | 19 | The term relates to descriptions and transfer of information. | Allele, diploid, locus |
Molecular | 23 | The term relates to molecular or macromolecular structures. | Tubulin, epitope, ligand |
Organelle | 11 | The term is the name of an organelle or part of an organelle. | Vacuole, vesicle |
Practice | 7 | The term relates to the practice of science itself. | Assay, experimental control, model |
Process | 7 | The term is a cellular or biological process. | Mutation, secretion, gene regulation |
In this study, we assessed two domains: 1) students’ perceived understanding of the technical vocabulary terms (henceforth referred to as “perceived understanding”), and 2) students’ ability to provide correct and complete definitions of the various technical vocabulary terms (henceforth referred to as “performance”). To assess perceived understanding of and performance surrounding the terms, we used an online vocabulary survey. Students were given the option to voluntarily complete the survey at the end of the semester, before the final exam. When students took the survey, they were presented with five randomly selected terms relevant to their course. For each of the five terms, students were sequentially asked 1) whether they recognized the term (yes or no), 2) whether they understood the term (yes or no), and 3) to define the term in their own words to the best of their ability. Responses to questions 1 and 2 were used to assess perceived understanding, and responses to question 3 were used to assess performance. Students accessed the survey through their course-specific website and could take the survey as many times as they wanted, with a subset of five vocabulary terms randomly chosen each time. Instructors promoted the survey as a good opportunity for students to test themselves on key technical vocabulary terms before the final exam and to participate in the research study, but there were no additional incentives awarded for participation or correctness. There were no additional instructions provided to students (e.g., they were not provided with a review list of terms to study). Of the 1637 registered students in these two courses, 263 (16%) took the survey at least once. Before doing any analysis, we assigned a unique label to each student to obscure his or her identity. Student responses were not coupled to their course performance, and no additional demographic questions were asked (such as language status). Survey responses were approved for use in this research study under the institutional research policy of the University of British Columbia’s research ethics board.
Data Used in Analysis
Although 76 terms were included in the surveys, after terms with fewer than 10 responses were removed, responses to 72 terms to analyze remained. Individual responses that appeared to be copied from the textbook or online sources were also removed (e.g., if the response was identical to those in the course textbook or an online source). All responses for students with at least one case of suspected plagiarism were removed from the study. In addition, because students could take the survey multiple times and the same term could randomly appear multiple times in a student’s multiple attempts, we only included the first response to a given term from an individual; in this way, we removed pseudo-replicates. There were two instances when a student left the question “Do you understand this term?” blank, and in these two cases, we assumed that the students did indeed understand, given their complete and accurate definitions provided in response to the third question. In the final analysis, we used 1276 responses from 263 students.
Analysis: Students’ Perceived Understanding of Technical Vocabulary Terms
To assess students’ perception of their understanding of each term, we focused on their answers to the survey question “Do you understand this term?” In particular, we compared terms based on the percentage of responses that indicated participants understood the term (% Thought Understood). This percentage was calculated by dividing the sum of those who did understand the term by the sum of all responses for that term, excluding responses in which students did not answer whether they recognized or understood the term.
The 72 terms were also sorted into non–mutually exclusive categories. To do this, we identified common characteristics among groups of terms and labeled the categories that we felt best described the terms. The categories were based on the meanings of the terms and the terms’ context within the courses. We consulted with instructors for the two courses, who either confirmed or altered our preliminary categorizations. As a result, we categorized 20 of the 72 terms as Incompatible Ambiguity, 23 as Molecular, seven as Practice, 11 as Organelle, seven as Process, and 19 as Information (Table 1).
Each category was treated as a two-level factor, assigned a “1” if the term fit in the category or a “0” if it did not. To see whether there was a difference in % Thought Understood between terms in a single category versus all other terms, we ran Kruskal-Wallis rank-sum tests in R (v. 3.2.4) for each category. These tests were used because the data were not normally distributed and the sample sizes of each level were not equal; the assumptions required for reliable analysis of variance tests were therefore not met. A Bonferroni correction was applied to the significance level for each test (∝ = 0.05) to account for the number of tests performed: the adjusted significance level (∝′) for each test was 0.008 (0.05 divided by six tests performed).
Analysis: Correctness of Student Definitions
The remainder of the analysis focused on the Information, Incompatible Ambiguity, and Molecular categories, as these categories showed the highest and lowest student perceived understanding. We coded the correctness of and identified common errors in student-provided definitions for 23 Molecular terms, 19 Information terms, and 12 additional Incompatible Ambiguity terms that were neither Molecular nor Information. Coding was performed regardless of reported understanding. To code for correctness, we compared student-provided definitions with those agreed upon by the course instructors, which often were the definitions found in course textbooks (Alberts et al., 2010; Griffiths et al., 2012). Examples of some definitions can be found later in this article in Table 5. Blank responses, definitions for which students wrote, “I don’t know,” or a random string of letters were coded “0.” Definitions that were incorrect were designated “1,” while incomplete or partially correct responses were designated “1.5,” and completely correct responses were designated “2.” All coding (completed by J.Z.) was double-checked by another researcher (M.B. or L.M.) to reach a consensus and reduce bias. In total, 999 student responses were coded for correctness: 349 Molecular responses, 418 Information responses, and 232 additional Incompatible Ambiguity responses (166 of the 398 Incompatible Ambiguity responses we coded in total were for terms also classified as either Molecular or Information).
We calculated the percentage of students who provided a correct definition (% Correct), an incorrect definition (% Incorrect), or a partially correct definition (% Partially Correct) or who did not provide a definition (% Unanswered) for the coded terms. We also quantified “matches” and “mismatches” in perception versus correctness (Figure 1). Matches include the percentage of students reporting they understood the term who also wrote the correct definition, as well as students claiming to not understand the term who wrote an incorrect definition or no definition. Mismatches include the percentage of students claiming to understand the term (% Thought Understood) who wrote an incorrect definition or no definition and that of students claiming to not understand the definition (% Not Understood) who provided a correct definition.
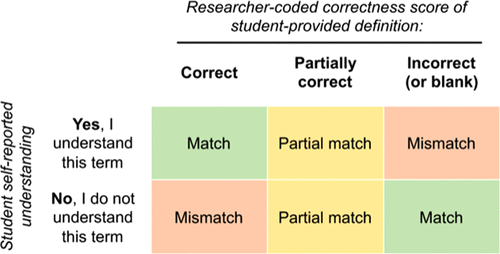
FIGURE 1. Scoring matches by assessing alignment of definitions for correctness with perceived understanding. Each student survey response was given a score for correctness, and a match code (Match, Mismatch, Partial Match). Correctness was determined by comparing student definitions with the definition for the term deemed acceptable based on the biological meaning and use of the term in the course.
We analyzed the data to see whether differences existed in % Thought Understood, % Correct, % Incorrect, % Match, % Mismatch, and % Partially Correct between Molecular and Information terms. As before, Kruskal-Wallis tests were used. We only performed Kruskal-Wallis tests comparing Information and Molecular terms, because these terms were mutually exclusive: no Information terms were also Molecular terms. In contrast, several Information and Molecular terms were also categorized as Incompatible Ambiguity and therefore could not be compared against Incompatible Ambiguity terms. Following these tests, we calculated correlations between % Correct and % Thought Understood to see whether the terms that are more commonly understood are also more often correct, and vice versa.
To determine whether students held common misunderstandings of the terms, we scored the types of errors in the incorrect definitions for Information, Molecular, and Incompatible Ambiguity terms. Four types of errors were scored (Table 2).
Type of error | Explanation and example |
---|---|
Omission | A definition that is incomplete because a major component required for the definition has been omitted (e.g., definitions of kinase that do not mention that it is a protein or enzyme) |
Defined something else | A definition of a term other than the term presented, either a term with a related meaning (e.g., intron defined instead of exon) or similar spelling (e.g., paternal defined instead of parental) |
Inaccuracy | A definition containing incorrect information, such as definitions of clathrin that claim it is a type of vesicle, rather than a protein |
Everyday language meaning (nonbiological meaning) | A term that has been defined in its everyday language, nonbiological context, such as defining “adaptation” as “changing to adjust to a new environment” (scored only for terms that fell into the Incompatible Ambiguity terms) |
RESULTS
Students’ Perceived Understanding of Technical Vocabulary Terms
The percentage of students who thought that they understood the given term ranged from 0% (1 term: “epitope”) to 100% (18 different terms), with a study-wide mean of 81.3% (± 2.46% SE) of % Thought Understood. The fact that no students felt they understood the term “epitope” stood out; however, it was confirmed with instructors that this term was used multiple times in the course (we elaborate on this in the Discussion). The majority of the 10 terms with the lowest % Thought Understood values were Molecular terms, with one scientific Practice term (“assay”) included as well (Table 3 and Supplemental Table S1). Of the 72 terms surveyed, there were 18 terms that 100% of the respondents reported they understood the term; of these, three were Molecular terms, eight were Information terms, and seven were Incompatible Ambiguity terms (Table 3 and Supplemental Table S1).
Jargon term | Number of responses | Category | % Thought Understood |
---|---|---|---|
Lowest % Thought Understood: | |||
Epitope | 13 | Molecular | 0.00 |
Assay | 19 | Practice | 26.3 |
Allosteric | 15 | Molecular, Process | 26.7 |
Cyclin | 13 | Molecular | 38.5 |
Protease | 13 | Molecular | 38.5 |
Macromolecular complex | 20 | Molecular | 40.0 |
Ligand | 17 | Molecular | 41.2 |
Activator | 17 | Molecular, Incompatible Ambiguity | 58.8 |
Myosin | 13 | Molecular | 61.5 |
SNARE | 11 | Molecular | 63.6 |
Highest % Thought Understood: | |||
Actin | 17 | Molecular | 100 |
Allele | 24 | Information | 100 |
Centromere | 17 | Molecular | 100 |
Centrosome | 14 | Organelle | 100 |
Chromosome | 10 | Organelle | 100 |
Complementation | 19 | Incompatible Ambiguity | 100 |
Diploid | 34 | Information | 100 |
Dominant | 28 | Information, Incompatible Ambiguity | 100 |
Experimental control | 14 | Practice | 100 |
Gene | 14 | Information | 100 |
Hydrophobic interaction | 12 | Molecular | 100 |
Mutation | 12 | Process | 100 |
Necessary | 23 | Incompatible Ambiguity, Practice | 100 |
Recombinant | 21 | Information | 100 |
Sufficient | 11 | Incompatible Ambiguity | 100 |
Template | 12 | Incompatible Ambiguity, Information | 100 |
Transcription | 18 | Incompatible Ambiguity, Information | 100 |
Translation | 13 | Incompatible Ambiguity, Information | 100 |
We then examined the mean % Thought Understood by category, which ranged from 68 to 91% (Figure 2). Of the six jargon categories, mean % Thought Understood was lower for Molecular terms than all other terms (n of Molecular terms = 23, n of other terms = 49, Kruskal-Wallis test χ2 = 10.9, p < 0.008) and higher, though marginally statistically significant, for Information terms than for all other terms (n of Information terms = 19, n other terms = 53, Kruskal-Wallis test χ2 = 6.47, p = 0.01; Figure 2 and Supplemental Table S2). Mean % Thought Understood was also higher for Incompatible Ambiguity terms than all other terms (n of Incompatible Ambiguity terms = 20, n of other terms = 52, Kruskal-Wallis test χ2 = 4.89, p = 0.03; Figure 2 and Supplemental Table S2).
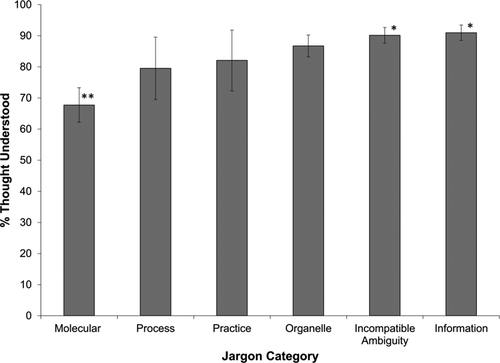
FIGURE 2. Mean of students’ perceived understanding (% Thought Understood) for terms in each of the six non–mutually exclusive jargon categories. Students perceived themselves as understanding Information terms better than all other terms and understanding Molecular terms less well than all other terms. N (number of terms in a category) = 23 Molecular terms, seven Process, seven Practice, 11 Organelle, 20 Incompatible Ambiguity, and 19 Information. All terms can be found in Supplemental Table S1. Kruskal-Wallis test of terms in tested category against all other terms: **, p < 0.008; *, p < 0.05. Error bars are standard error of the mean.
The remainder of the results will focus on the Information, Incompatible Ambiguity, and Molecular categories, as these categories showed the highest and lowest student perceived understanding.
Comparing Correctness of Student-Provided Definitions with Perceived Understanding
One goal of this study was to determine the relationship between perceived understanding of the terms versus demonstrated performance. Performance was determined by scoring the student-provided definitions for correctness. Most student-provided definitions were incorrect or partially correct, with ∼40% of all definitions scored as completely correct. This is low, considering the survey was deployed to these students in the sophomore-level Cell Biology and Genetics courses at the end of the term. When we considered correct and partially correct answers together, students were least correct when defining Molecular terms and performed best on Information terms (Figure 3). This difference is the result of significantly more of the definitions for Information terms being partially correct compared with those provided for Molecular terms (Kruskal-Wallis test χ2 = 8.09, p < 0.005; Table 4).
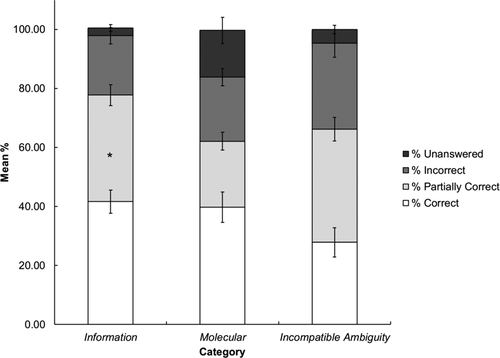
FIGURE 3. Distribution of correctness for student-provided definitions of terms in the Information, Molecular, and Incompatible Ambiguity categories. Error bars are standard error of the mean. *Kruskal-Wallis test χ2 = 8.09, p < 0.008 comparing % Partially Correct between Molecular and Information categories. N (number of terms in a category) = 19 Information terms, 23 Molecular terms, and 20 Incompatible Ambiguity terms.
Kruskal-Wallis between Molecular and Information | |||||
---|---|---|---|---|---|
Type of match (mean) | Incompatible Ambiguity (20 terms) | Molecular (23 terms) | Information (20 terms) | χ2 | p |
% Match | 35.4 ± 4.09 | 60.1 ± 4.56 | 44.6 ± 4.18 | 5.53 | 0.02 |
% Mismatch | 27.2 ± 4.05 | 18.5 ± 2.95 | 19.9 ± 3.22 | 0.08 | 0.77 |
% Partial Match | 38.3 ± 4.04 | 22.4 ± 3.06 | 36.1 ± 3.58 | 8.09 | 0.005 |
Comparing students’ perceived understanding (% Thought Understood) with their performance (correctness scores) reveals a widespread overestimation in understanding of the terms in these three categories (Figure 2 vs. Figure 3). For example, although the mean % Thought Understood was 25% higher for Information terms compared with Molecular terms, there was no significant difference in the mean correctness or incorrectness of the definitions provided (Figure 3, “% Correct” Kruskal-Wallis test χ2 = 0.02, p = 0.90; “% Incorrect” Kruskal-Wallis test χ2 = 0.37, p = 0.54). To further quantify the degree of overestimation of understanding, we also scored each student’s definition as a match or mismatch by comparing the perceived understanding for a given term (yes or no) with the correctness score of the definition the student provided (scoring detailed in Figure 1). The % Match was higher for Molecular terms than for Information terms (Kruskal-Wallis test χ2 = 5.53, p = 0.02; Figure 4 and Table 4), confirming that students were better able to assess their ability to correctly define terms in the Molecular category. The majority of mismatches were the result of overestimation (students indicated that they understood, but provided an incorrect definition), although there were instances of understanding being underestimated (students reported the term was not understood, but provided a correct definition). The % Partial Match values indicate that students generally had some correct understanding of the terms.
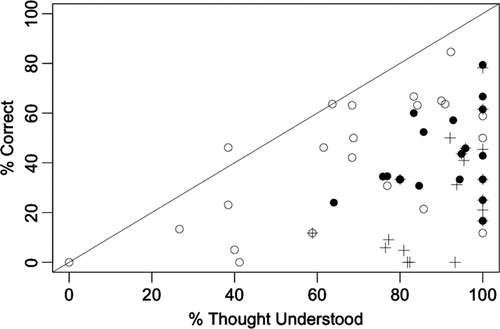
FIGURE 4. Comparison of perceived understanding (% Thought Understood) with correctness (% Correct) for 23 Molecular terms (white circles), 19 Information terms (black circles), and 20 Incompatible Ambiguity terms (plus signs). Molecular and Information terms were mutually exclusive, but Incompatible Ambiguity terms were not: some Incompatible Ambiguity terms (represented by a plus sign on top of a circle) were categorized as both Incompatible Ambiguity and either Molecular or Information. Each data point represents a unique term. The black line bisecting the plot represents a 1:1 ratio; points that lie on this line have equal % Correct and % Thought Understood values, while those below and to the right of the line have greater % Thought Understood than % Correct values.
The general tendency to overestimate was not the result of extreme overestimating of a few terms in a given category, but was universal for almost all terms, particularly for Information and Incompatible Ambiguity terms (Figure 4).
Common Errors in Understanding of Biology-Specific Terms
Most of the errors in student-provided definitions for any given term were void of specific commonalities, with the exception of providing definitions for highly similar words (“transcription” and “translation,” “centrosome” and “centromere”). Although we initially set out to score incorrect definitions for four types of errors (Table 2), omission errors were the most consistently identified type of error in student-provided definitions for multiple terms. Most correct definitions contained two components: an explanation of structure (e.g., what it is) and an explanation of function (e.g., what it does or what it can be used for). For example, the term “gene” can be defined as a DNA sequence (structure) that codes for an RNA product (function); and the term “model” can be defined as a representation of a system (structure) that we can test (function). The most frequent error in incorrect or partially incorrect student-provided definitions was an omission of one part of a definition: either the structure or function component (Table 5). There was no relationship between type of term and whether structure or function was the most common omission, as there were a nearly equal proportion of structure omissions and function omissions in definitions for any given term.
Jargon category | Term | Standard definition used in course | Common omission type: example of omission |
---|---|---|---|
Incompatible Ambiguity | Checkpoint | Mechanism by which the cell cycle control system can regulate progression through the cycle, ensuring that conditions are favorable and each process has been completed before proceeding to the next stage | Structure: that it is a mechanism; that it is regulatory |
Conformation | Precise, three-dimensional shape of a protein or other macromolecules, based on the spatial location of its atoms in relation to one another; or, the folded, three-dimensional structure of a polypeptide chain | Structure: involves 3D shape, involves atoms | |
Model | A representation of a system (based on data) that we can test |
| |
Sufficient | The minimum component(s) that are enough to allow for a function or process to occur | Function: missing functionality, minimum requirement (only a few for each of these) | |
Theory | A tested and evidence-supported reasoning for a phenomenon of nature | Function: explains a phenomenon of nature | |
Information | Allele | A sequence variation of a particular locus or gene | Structure: variation in sequence |
Exon | A coding DNA sequence that is transcribed (and sometimes translated) | Function: that it is translated (coding sequence) | |
Oncogene | A gain-of-function allele/mutant of a proto-oncogene (gene that codes for a product that is involved in cell cycle control) | Structure: mutant, gain-of-function, allele of proto-oncogene | |
Promoter | A DNA sequence that is recognized/bound by transcription factors or RNA polymerase |
| |
Transcription | Process that makes an RNA copy of a DNA sequence | Function: no mention of RNA | |
Molecular | Activator | A protein that recognizes/binds to a sequence of DNA (enhancer) to promote transcription | Structure: it is a protein |
CDK | An enzyme (kinase) that can phosphorylate substrates, involved in regulating variety of processes (such as the cell cycle) | Function: phosphorylation involved | |
Centromere | A region on the chromosome where microtubules attach (at the kinetochore/during cell division) | Function: where spindle fibers bind/kinetochore | |
Kinase | An enzyme that phosphorylates another molecule (with use of ATP) |
|
Surprisingly, very few of the definitions provided by students for the Incompatible Ambiguity terms included the everyday language definition, with the exception of “adaptation,” for which nearly all students provided an everyday language definition rather than the evolutionary biology definition of the term. This may be the result of the use of the term in the course, which will be discussed in more detail in the Discussion.
DISCUSSION
In this work, we present a systematic analysis of student perceived understanding of and demonstrated performance at accurately defining various discipline-specific vocabulary terms in biology. The differences between perception of (self-reporting) and performance surrounding different types of vocabulary sheds light on the complexity of learning the language of biology (“jargon problem”) in two different undergraduate classes and suggests a need for further study and more nuanced teaching approaches that support the development of a strong understanding of discipline-specific language. Here, we discuss the major themes that emerged from our work, the limitations of this study, and the implications for teaching.
Student Perception of Understanding Different Types of Terms: False Familiarity?
Students’ perception of understanding was not equal for different types of biology-specific vocabulary. As a category, terms related to abstract phenomena (Molecular terms) were generally, and accurately, perceived to be the most poorly understood, whereas all other terms had consistently high levels of perceived understanding, with Information terms having the highest. However, students were less accurate at assessing their understanding of Information and Incompatible Ambiguity terms, and the most commonly incorrect and misunderstood terms were found in the Incompatible Ambiguity category. Students may have a tendency to overestimate their explanatory abilities (Rozenblit and Keil, 2002), which could lead to the overestimation of understanding of much of this jargon and a reduced ability to correctly self-assess. Notably, the Information and Incompatible Ambiguity categories both include terms to which students likely have had significant prior exposure.
Familiarity can impact students’ ability to recognize how much they know and possibly lead to incorrect assumptions about how well they know the material (Reder and Ritter, 1992; Willingham, 2003). This can pose a problem for developing a deep understanding, because familiarity can short-circuit metacognitive processes that are important in effective learning (Kruger and Dunning, 1999; Ambrose et al., 2010; Tanner, 2012). Many of the terms in the Information and Incompatible Ambiguity categories would have been encountered in prerequisite college courses, and even in contexts dating back to high school or earlier, including “gene,” “transcription,” “theory,” and “sufficient” (British Columbia Ministry of Education, 2006). Students reported greater understanding of these terms compared with others, such as those in the Molecular category, which could be a reflection of their increased familiarity with said terms. Marshall et al. (1991) found that students struggled with terms frequently used in science, even if those terms were not representing complex concepts; these authors called such terms “non-technical” terms (e.g., “negative,” “accumulate,” and “consistent”). This could be attributed to a combination of the fact that misunderstandings of these terms may go unrecognized by students because of the familiarity of the words from use in both everyday discourse and science communication and the fact that instructors may rarely teach or reinforce the meaning of these common, nontechnical terms. The potential negative effects of familiarity on learning and teaching or correcting misunderstandings may partially explain why students tended to overestimate understanding, particularly for Information and Incompatible Ambiguity terms, as seen by the high number of mismatches in actual understanding versus perceived understanding.
The application of existing pedagogical interventions may be used to help make students aware of their misunderstandings or prevent the pitfalls of familiarity. Testing on presumed familiar terms early in the course can inform the instructor of student misunderstandings and, with appropriate feedback, can inform and motivate students to correct misunderstandings (Tanner, 2012; Maxwell et al., 2015). Early testing and immediate feedback can serve to remove assumptions about prior knowledge and trigger metacognitive awareness of inappropriate or missing prior knowledge or trigger appropriate prior knowledge (Ambrose et al., 2010). As we found, students may have partial understanding and require specific feedback to identify what part of their understanding is missing. Applying the concepts of retrieval practice (Roediger and Butler, 2011; Pan et al., 2015) by embedding frequent opportunities for students to demonstrate understanding of vocabulary may also aid in retention of correct understanding. Instructors may want to consider using a tool similar to the one used in this study as a precourse survey to assess student familiarity and understanding of terms commonly used in the course.
It should also be noted that familiarity with a term may impede the instructor’s ability to recognize when certain terms are truly new to students. Take, for example, the term “epitope” used in this study. Instructors in the course felt this was a term that students should know, one that was used in the course in more than one context, and yet none of the surveyed students felt they knew what the term meant. The instructors’ familiarity with the meaning of the term may have resulted in them neglecting to sufficiently instruct students on the meaning. Similar to the importance of reviewing how the learning objectives of a course are being assessed, we suggest that it is also useful to evaluate the technical vocabulary being used in a course, and how often the terms are explained or used in varied examples.
Students Perceive Molecular Terms to Be More Challenging
Students less frequently claimed understanding of Molecular terms compared with Information and Incompatible Ambiguity terms. Although some of the Molecular terms were likely new to students, there were many that students were expected to have encountered in previous, prerequisite courses. Thus, we do not believe that a lack of exposure is the only reason that students ranked Molecular terms as harder to understand and more accurately perceived their understanding of these terms. The variety of difficulties with learning molecular life sciences content, including the difficulty with jargon and visualizations of abstract phenomena, have been acknowledged by others (Tibell and Rundgren, 2010). We hypothesize that Molecular terms pose a particular challenge to students because of the microscopic, and therefore abstract, nature of the phenomena these terms describe. Challenges with learning abstract terms versus concrete terms have been shown in other contexts. For example, De Groot and Keijzer (2000) found that experienced foreign language learners attempting to learn new vocabulary were more likely to forget abstract words, and it took longer for the learners to recall abstract words than concrete words. Cassels and Johnstone (1984), reiterated by Johnstone (1991), proposed the lack of sensory examples (e.g., visual models) for much of the microscopic and biochemical phenomena in chemistry and science, represented by the technical vocabulary used to describe them, makes it particularly challenging to learn associated material. This may be reflected in our results. Compare, for example, students perceived understanding of Molecular versus Organelle terms. We predict that students likely had much more familiarity and developed visual models of the Organelles, making them seem easier to understand. In contrast, we predict that the opposite is true of Molecular terms such as “protease” and “allosteric”; students may lack a visual model, or sensory example, of these terms and thus find them harder to understand and learn. Terms describing microscopic and abstract phenomena were also found in other categories, such as the genetic terms “allele” and “gene.” However, we believe that the repeated exposure to these terms likely provided students with a richer connection to the terms, and thus greater sense of perceived understanding. In addition, terms such as “gene” and “allele” may have been introduced to students several times, using several different visual representations, which provides more opportunities for students to adopt their own visual models of these abstract terms. In comparison, we speculate that students likely do not have multiple, varied visual models of “epitope” to reflect upon, thus reducing their ability to understand and recall the meaning of the term.
As educators, we can strive to find more opportunities to help students build visual models of abstract phenomena and the vocabulary associated with them. Min et al. (2014) showed that a visual model added to a particular test problem improved student understanding of abstract concepts and their perceived understanding. Mayer (1989) also found that visual models added to learning material improved students’ conceptual recall. Cohen and Johnson (2011) found that learning of science terms was improved in elementary students when the learners were tasked with creating an image to illustrate the term, compared with when they were required to use the term in a sentence. This may point to the value in developing mental models of technical, and perhaps in particular, abstract terms.
Providing opportunities to tangibly connect abstract technical vocabulary to prior knowledge may also demystify some of these terms and strengthen student understanding, and thus learning, of the terms and the concepts they represent or to which they relate (Rosen et al., 2012). This could be accomplished by providing examples from multiple contexts, helping students develop their own mental models, and contrasting visual models to identify what works and does not work. Making connections between newly learned abstract phenomena to existing knowledge and visual models may also be aided by first describing concepts using everyday language, to which students may more easily relate. Previous work shows that, during the initial learning of a new concept, student understanding of concepts (McDonnell et al., 2016) or concepts and jargon (Brown and Ryoo, 2008) can be improved when excessive technical vocabulary is temporarily removed and replaced with more familiar everyday language explanations. Practice explaining understanding of concepts, with correct use of technical vocabulary, would also likely be beneficial at increasing students’ ability to fluently use discipline-specific language (Chi et al., 1994).
Omissions Suggest Superficial Understanding
The low correctness scores of student-provided definitions could often be attributed to an omission of either the structure or function component of the definition for the given term. Take, for example, the term “repressor,” which was defined as a molecule (or protein) that binds to a region of DNA and prevents expression (transcription). The molecule/protein is the structure, and the act of repressing expression is the function. Not all terms required these two components, but when they did, it was common for students to define one or the other, but not both, leading to a low % Correct, and moderate % Partially Correct scores on definitions. We postulate that this is a reflection of an overall superficial and poor understanding of the meaning of many of the terms. Students are not often provided with a structure–function breakdown of the meanings of various technical vocabulary terms, and thus they may develop a superficial or partial understanding of the term (they were not given such instruction in the courses surveyed). When introducing and reinforcing technical vocabulary, we propose that it may be important to have students recognize the critical components of a definition and to emphasize the importance of addressing the structure and function components when testing student understanding. One could also imagine using a structure–function framework or an expert-like mechanistic model (Trujillo et al., 2015, 2016) to develop and test understanding of technical vocabulary.
It should also be noted that, although many students may recognize a complete definition containing both the structure and function elements as more correct than a definition omitting one of these elements, it is likely far more challenging for students to articulate a full and correct definition in writing (as was done in our survey) than it is for them to recognize correctness or even use the term in the correct context. It may be worthwhile contrasting students’ ability to recognize correct definitions versus to produce correct definitions to further categorize student understanding of technical vocabulary terms.
Perhaps surprisingly, and in contrast to Rector et al. (2013), we did not see a high frequency of students providing the everyday definitions of the Incompatible Ambiguity terms, where we might have expected them to do so given the dual use of these terms in science and everyday language. It is likely that the context (an in-course survey about scientific vocabulary) was a signal to use the scientific definition. Additionally, it may be that the terms from this category were so often used in students’ science classes that they had developed a science-context understanding of the terms, albeit often incorrect or partially correct. The one exception to this was for the term “adaptation,” to which 100% of the students provided the everyday language meaning of this term compared with the evolutionary meaning. As Kouba (1989) found, mathematicians and scientists in their study often differed in the way they used the same terminology. A similar explanation may apply to our findings: in the course content of the biology classes surveyed, the word “adaptation” was used in the colloquial sense. Such differences in the way we use terminology among courses and STEM disciplines may impact student understanding in subsequent courses and thus deserve consideration.
Limitations and Follow-Up Questions
In this work, we assessed understanding of discipline-specific vocabulary from a relatively large population of students. Although we were limited to only two courses, it is worth noting that similar trends were observed in both of these different courses, taught by different instructors, suggesting that our results are likely relevant in other courses across biology programs. Follow-up work that investigates student usage of vocabulary in other biology subdisciplines, such as ecology, evolution, and physiology, would provide further insights on the types of troublesome jargon in these subdisciplines. We encourage additional work to determine which terms are problematic for students, and why, and subsequent efforts to incorporate additional factors in analysis that may provide refined understanding of the challenges with learning technical vocabulary. Such factors include assessing familiarity or exposure to terms, as this factor may inform reasons why students have potentially unexpected gaps between perceived understanding and actual understanding. Another factor that may influence student responses could be English language fluency. Many of the students in our population may not use English as their primary language of communication. Data on student language status and proficiency were not collected as part of this study, and so we were unable to account for these variables. Future work should include this factor to determine whether there is an additional relationship between language fluency and student perceived understanding of and performance using jargon terms.
The methods used here to assess student perceptions and understanding were useful, but have limitations. The question we used to measure students’ perceived understanding had only binary answers: “yes” or “no.” This may have forced some students who had intermediate confidence in their understanding to falsely choose an option. In future, it would be useful to provide students with a confidence scale that would provide a finer scale for determining whether students are accurate in assessing their understanding. Additionally, we assessed student performance in a context-independent manner, which may influence correctness. This style of vocabulary-recall testing has been used in other work (Kang, 1995), but it is not consistent with how students might normally use this vocabulary, such as within an essay, in an explanation to a problem they have solved, or in discussion.
We have also considered that student performance on the tested vocabulary may be lower than expected as a result of the course culture. As stated in the Methods, the courses surveyed did not include explicit assessment and direct feedback of students’ ability to define terms. The terms were introduced in readings and in class and were used as part of course discourse, and it was expected that students would learn and know the meaning of the terms in order to recognize and use them in their written responses to high-stakes test questions. A lack of high-stakes assessment and feedback on definitions of the majority of terms could result in an incomplete understanding. Future work could also focus on determining the impact of including technical vocabulary understanding on high-stakes assessments on student perceived and actual understanding of the terms. Furthermore, it would be useful to investigate the relationships between students’ ability to correctly define and use jargon on their overall course performance—which encompasses more than simple conceptual understanding. Cassels and Johnstone (1984) found that substituting certain jargon terms in chemistry multiple-choice questions resulted in significant increases in student performance, stating that jargon may make the meaning less accessible, which could negatively impact overall student performance. Given the importance of jargon in postsecondary STEM education, further investigation to understand the relationship between mastery of technical vocabulary and student performance across a variety of assessment types is warranted.
With such easy access to digital information, one might wonder whether it is important to know and understand technical vocabulary terms when definitions can always easily and quickly be looked up. Similarly, to emphasize concepts in our teaching, one could imagine minimizing or even omitting the technical language entirely. However, although easy access can be leveraged to fill in the gaps when encountering new technical vocabulary terms, the ability to use a dictionary (online or otherwise) is not equivalent to the mastery of the language—the ability to communicate fluently as part of scientific discourse. Indeed, one critical aspect of our role as educators is to support student development of skills for the discipline they are entering. For better or worse, technical vocabulary is a disciplinary norm in the sciences, and so learning and using the language of science is just as critical as learning the underpinning concepts. Relative emphasis on the approaches toward learning vocabulary may, of course, be context dependent. For example, in nonmajors’ and introductory courses, we may particularly aim to explicitly encourage students to not be intimidated by jargon, but rather to learn how to navigate and make sense of scientific writing that contains jargon in order to use available information to be informed citizens.
CONCLUSION
Learning and proper use of discipline-specific vocabulary is necessary to advance in science. A lack of vocabulary can impede literacy and learning (reviewed in Joshi, 2005). Students move in a progression of phases toward biological literacy; they begin as novices who may be able to identify terms and then provide definitions, ideally progressing toward fully understanding concepts, explaining ideas, and making connections between biology and other disciplines (Uno and Bybee, 1994). These higher-order literacy skills will be impeded if students do not master the language of science, in our case, of biology. To improve scientific literacy, it is important to identify discipline-specific vocabulary, or jargon, and provide specific pedagogical support to help students accurately assess their own understanding, develop a firm understanding of the meanings, and appropriately use discipline-specific vocabulary. To improve vocabulary learning, we must incorporate more opportunities for students to engage directly with the terms (Schmitt, 2008) and receive feedback on their performance. This will assist in improving students’ ability to achieve goals of science literacy and communication that are valued components of a biology education.
ACKNOWLEDGMENTS
Thank you very much to the course instructors for giving feedback about terminology to assess and for supporting us in sharing the survey with students: N. Abraham, L. Chen, J. Cooke, L. Kunst, M. Graves, P. Kalas, J. Klenz, and R. Young. Thank you to the students who completed the survey. For helpful feedback about this study and article, we thank Carl Wieman, Trish Schulte, Ella Tour, and Stanley Lo. This project was partly funded by a development grant from UBC Skylight (Science Centre for Learning and Teaching).