Assessing High Performers in the Life Sciences: Characteristics of Exams Used at the International Biology Olympiad (IBO) and Their Implications for Life Science Education
Abstract
For decades, studies have revealed students’ decreasing interest in science. Extracurricular learning opportunities—the Science Olympiads being a publicly well-known example—are an important means identified to tackle this challenge and help students further differentiate their interests. Better understanding the underlying constructs and characteristics of Science Olympiad exams can provide several implications not just for Science Olympiads, but also science education more broadly, for example, with regard to how the competitions’ international juries defines expectations for high performance in the life sciences. This study analyzes exams set by the International Biology Olympiad (IBO) as an example for a top-tier international competition in the life sciences. The findings extend previous works on test item characteristics toward student competitions and high-performer education. We conducted a systematic analysis of N = 703 closed-ended and laboratory test items from six IBO assessment years across the competition’s history. A categorical framework was developed to analyze items according to four areas: formal characteristics, content and practices, cognitive aspects, and the use of representations. Our findings highlight assessment characteristics used to challenge high-performing students. We derive implications for general life sciences education, as well as for further developing the assessments of Science Olympiads.
INTRODUCTION
Only a small group of students are high performers in science education (9% average in Programme for International Student Assessment [PISA], Organisation for Economic Cooperation and Development [OECD], 2009). Of the strongest science performers, about three-fourths value science education and have high science-related self-concepts and self-efficacy.1 They also get substantially more involved in extracurricular science learning. Yet only approximately half of the highest science performers are also interested in studying science or in working toward science-related careers (e.g., OECD, 2009; see also Prenzel et al., 2002; OECD, 2016). Research suggests extracurricular learning opportunities can help provide appropriate learning resources for high performers and support their interests in science (e.g., Campbell and Walberg, 2010; Subotnik et al., 2011; Dionne et al., 2012). Science competitions represent a major strand among these, with the Science Olympiads being a publicly well-known example.
The latter provide high performers with in-depth science learning at a level that schools may struggle to provide (Tuan et al., 2005; Dionne et al., 2012). From the perspective of differentiated education for high performers, these competitions offer performance-appropriate, competitive challenges for students with special interests that furthermore suit the character traits of high performers, for example, a preference for competitive situations (Udvari and Schneider, 2000). Ideally, the Science Olympiads help refine the participants’ science-related interests and motivate them to pursue careers in the field (Lind and Friege, 2004; Feldhusen, 2005; Subotnik et al., 2011).
From a broader science learning perspective, the Science Olympiads also have the function of engaging a wide spectrum of students of varying levels of ability with science at entry-level competition rounds, often over several months. These rounds are typically advertised in public and private schools nationwide and strongly supported by educational authorities, sometimes resulting in more than 100,000 participants per country. This leads to the secondary effect of multiple schools offering preparatory after-school clubs that provide deepened science-learning opportunities. In summary, the educational impact of the Science Olympiads on participants has been assumed to be substantial, reaching far beyond specialized education for the strongest performers. However, the empirical evidence of their educational effects still needs to be further studied (cf. Wu and Chen, 2001; Campbell and Walberg, 2010; Sahin, 2013; Schmidt and Kelter, 2017).
Better understanding of how the Science Olympiads contribute to students’ science learning can provide relevant implications not just for the competitions themselves. Learning from their educational characteristics and foci can also strengthen broader school science education (Subotnik et al., 2011): For example, understanding the characteristics of Science Olympiad assessments can provide insights into the elements perceived as relevant for science learning by international groups of experts. Comparing competition exams and their historical developments to the current foci of school science education standards can provide new perspectives on the validity of constructs used in these standards (e.g., Next Generation Science Standards Lead States [NGSS], 2013; OECD, 2017).
Accordingly, we conducted a study to provide insights into the characteristics of assessments for high performers, aiming to identify both implications for science competitions and general education in the life sciences. We employed the long-established International Biology Olympiad (IBO) as an example for an international, top-tier student competition in the life sciences and present an item analysis of both closed-ended assessments and laboratory practicals stemming from six assessment years across IBO’s 30-year history. A qualitative approach is used to develop a categorical framework that can describe IBO assessment characteristics in four areas: formal item features, underlying scientific content and practices, aspects of cognition, and the items’ use of representations.
Two assumptions underlie this study that cannot be explored empirically within the frame of this article: First, we assume that all analyzed competition test items operate at a very high level of item difficulty in relation to average high school exams. Reporting psychometric properties of these tests would, in our experience, only be meaningful in relation to the highly specific sample of the strongest students in the life sciences. Hence, this article focuses on the test items’ content and structural characteristics, as these aspects have implications for a wider audience in science education. As a second assumption, our study assigns importance to competition exams as a potential learning opportunity for the life sciences. However, the impact of the competition’s exams is only in part linked to the specific exam context, but also to the long preparation for them, as well as to other potential learning opportunities during the competition week.
THEORETICAL BACKGROUND
The theoretical and empirical underpinnings for our assessment analysis derive from three fields: First, we review challenges related to high-performer education that underlie student competitions. Second, we focus on student competitions, their goals and present the study subject, IBO. Finally, we review assessment item characteristics known to influence item difficulty and describe how these formed the basis of our item analysis framework.
High-Performing Science Students Require Challenging Learning Opportunities
In this section, we refer to the concept of “giftedness” to discuss the particular learning needs of high performers in the life sciences, as these influence how IBO exams are designed and carried out. The term “giftedness” is well supported by theoretical and empirical works that describe and operationalize, for example, why certain students perform as well as they do in a certain domain (e.g., Heller, 2005; Subotnik et al., 2011). Importantly, giftedness does not denote “elitist” education. In the context of the Science Olympiads, elitist education is strongly discouraged (e.g., by IBO’s competition rules) and has been critically analyzed by the research community (e.g., Subotnik et al., 2011; Steegh et al., 2019).
Some leading contemporary approaches define giftedness in terms of outstandingly high performance in a specific domain (Subotnik et al., 2011), thus clearly separating it from generic personal qualities. Giftedness is a multidimensional construct in which measurable performance is predicted by several personal (e.g., intellect, skills, self-concept) and environmental factors (e.g., family, mentors, learning opportunities). Giftedness is considered developmental, as it can be influenced by environmental conditions and training (Heller, 2005; Subotnik et al., 2011; Worrell et al., 2012).
This has implications for educational programs for gifted students. These programs are built on several assumptions, including that gifted students have the potential to further develop their performance and make significant and valuable contributions to society later in their careers (e.g., Campbell and Walberg, 2010). It does not suffice to be identified as “gifted” based on, for example, a high IQ alone, as this is no guarantee for a later outstanding contribution of that person in a domain (Subotnik et al., 2011). Research has identified multiple factors linked to the progression of gifted students toward domain-specific productivity, including environmental conditions (e.g., family climate or outstanding teachers; Olszewski-Kubilius, 2008; Robinson, 2008; Ellison and Swanson, 2016); noncognitive personal characteristics like interests, goals, coping abilities, competitiveness, commitment, or self-concepts (e.g., Tai et al., 2006; Makel et al., 2012; Blankenburg et al., 2015); and the effort and time invested (Worrell et al., 2012). In summary, gifted students do not necessarily reach outstanding performance in a domain on their own—they need appropriate support and learning opportunities.
Studies show that many high performers enjoy challenges in science (Udvari and Schneider, 2000; OECD, 2009). To engage students more in pursuing science careers (especially underrepresented groups; Steegh et al., 2019), in-school or extracurricular learning can especially influence students’ interests (e.g., Sadler et al., 2012). When schools experience difficulties in providing challenging learning opportunities for high performers, these can be provided through extracurricular learning opportunities.
Student Competitions Provide Appropriate Learning Opportunities for High Performers
The purpose of the Science Olympiads is to offer engaging, competitive learning opportunities for domain-specific content and practice for high-performing students and those with special interest in science. The Science Olympiads—particularly at the international level—also function to provide (intercultural) exchange between like-minded individuals (Robinson, 2008; Stang et al., 2014; Köhler, 2017). Competitions aim to motivate students with potential for future science careers and help them extend and differentiate their abilities in this domain. While only a few studies have thus far systematically researched the degree to which science competitions attain these goals, some findings point to the (long-term) effectiveness of the Science Olympiads (e.g., Campbell, 1996; Campbell and Walberg, 2010; Wai et al., 2010).
Science competitions often try to cater to the requirements and expectations of their participants. Researchers have shed further light on students’ motivational reasons for participating in competitions. The identified factors appear to be diverse and competition specific (e.g., project-focused science fairs vs. task-based Science Olympiads). Dionne et al. (2012) found that interest in/values assigned to science content, students’ self-efficacy in science, and students’ prospects of obtaining rewards for participation (material and social) were key factors predicting students’ willingness to participate in a national science fair. Abernathy and Vinyard (2001) investigated differences in students’ perceived rewards for participating in science fairs and Science Olympiads. While both groups valued the exchange with other students and appearing in public, science fair participants primarily valued, for example, winning prizes and preparing for their futures, while Olympiad participants were primarily motivated by, for example, a wish to learn about scientific processes/university life. Studies have also determined factors predicting students’ participation tendency and competition success and identified, for instance, boredom in science class, expectancy of success, or prior participation in the competition as key factors (Urhahne et al., 2012; Stang et al., 2014; Blankenburg et al., 2015).
Despite these insights on predictors for participation or competition success, the Science Olympiads determine “winners” not based on personal characteristics, but according to domain-specific performance that underlies the respective competition exams (cf. Subotnik et al., 2011). Better understanding of what these exams assess therefore has implications for both the design of science competitions, but also for broader science education. So far, research has provided very little insight into this issue (cf. Köhler, 2017). Implications of the Science Olympiads could be linked to necessary adaptations of competition curricula, a more efficient preparation of competitors, or a more effective support for underrepresented groups (Steegh et al., 2019). Studying educational arrangements of learning opportunities for high performers can help derive suitable strategies to also enhance broader general science learning (VanTassel-Baska et al., 2009). Science Olympiad assessments are typically developed by larger groups of international experts in their respective fields. A competition exam analysis hence also provides insights into abilities considered relevant for future science learning, as defined by an international expert group from a given field. Investigating changes over the history of competition exams can especially reveal educational foci set by the item authors, which also allows relevant comparisons with the foci set for school science education (e.g., NGSS, 2013; OECD, 2017).
IBO as a Task-Based Science Competition
This study provides insights to the IBO as an example for the life sciences. Similar Science Olympiads (e.g., International Chemistry, Physics, Mathematics, or Junior Science Olympiads) have specific assessment foci, but are otherwise organizationally similar to IBO. IBO is an annual, task-based competition in life sciences for secondary students. As of 2019, IBO encompassed 74 national biology competitions that send their four best students to IBO. National competitions are open to all public school students and typically comprise three to four successive competition rounds that become increasingly more challenging. Unlike most previous research on science competitions (e.g., Blankenburg et al., 2015), this article focuses on an international competition. The annual 1-week IBO event is hosted by alternating countries. The Methods section provides details on the nature and development of the theoretical2 (i.e., closed-ended assessment items) and practical (i.e., hands-on laboratory work) exam types used in the IBO. In addition to the academic parts of the competition week, the contestants participate in social events, an award ceremony, and a farewell ceremony, which contribute to a combined IBO experience.
Item Characteristics Reflect Assessment Goals and Predict Test Difficulty
We established earlier that gaining insight into the traits of student competition assessments can be useful with regard to strategies for both specialized high-performer and general education assessments. Our study builds on prior research that analyzed national and international science education assessments with regard to characteristic features of assessment items. Our study extends these findings into the field of student competitions, particularly for IBO as an example for the life sciences. The analyses presented in this article follow four main areas of item characteristics identified by previous works (e.g., Prenzel et al., 2002; Florian et al., 2014; Florian et al., 2015). Item characteristics for the four areas can reveal both IBO’s theoretical and practical assessments items and are hence reviewed in the following sections.
Formal Item Characteristics.
Item format (e.g., multiple choice, constructed response) has substantial implications for the psychometric properties of test items and the assessed construct (e.g., Prenzel et al., 2002). In a review of university assessment strategies, Lindner et al. (2015) argued for the diagnostic value of closed-ended formats on the basis of substantial correlations between these and open-ended items. The authors refer to limitations of closed-ended formats for extreme performance bands and the assessment of creative processes. Some other relevant formal characteristics of items shown to effect item difficulty are text and sentence length, the share of polysyllabic words, and the use of negatives (e.g., Freedle and Kostin, 1993).
Cognitive Aspects.
Multiple frameworks of varying scope have been developed to describe which cognitive processes underlie a certain goal, task, procedure, or test item. Items constructed according to these frameworks appear to have varying effects on item difficulty (e.g., Anderson and Krathwohl, 2001; Crowe et al., 2008; Kauertz et al., 2010). A well-known framework for cognition, the taxonomy of educational objectives (Bloom et al., 1956; Anderson and Krathwohl, 2001; Stern, 2017), distinguishes the dimensions “types of knowledge” (i.e., declarative, conceptual, procedural, metacognitive) and “cognitive processes” (i.e., remember, understand, apply, analyze, evaluate, create). In this regard, it has been shown that the number of facts and the number of links between these facts in an item increase item difficulty, thus supporting the assumption of a hierarchy among the lower levels of types of knowledge (Kauertz et al., 2010; Neumann et al., 2013; Florian et al., 2014). While lower-level cognitive processes appear hierarchical in nature, higher-order cognitive processes (apply and higher) can also appear independently of one another, thus suggesting some underlying hierarchy of these processes, but not in the sense of a clear cumulative hierarchy (Anderson and Krathwohl, 2001, p. 267; Crowe et al., 2008; Stanny, 2016). Closed-ended item formats can likely be used to assess all of Bloom’s cognitive processes, with the exception of the processes related to the creation of products (Crowe et al., 2008). Bloom’s cognitive processes have also been shown to overlap in their interpretation (Stanny, 2016) and are inherently connected to domain-specific practices and contexts (Florian et al., 2014).
Content and Practice Elements.
Contact and practice are often regarded as the major characteristics of test items in domain-specific assessments (Prenzel et al., 2002; Florian et al., 2014; Tricot and Sweller, 2014). At its most basic level, knowing certain facts is part of almost all test items and has hence been analyzed as a major predictor of item difficulty (Prenzel et al., 2002). The degree to which students answer items based on either prior knowledge or additionally provided information substantially effects test performance (Florian et al., 2014). These findings underline a relevant distinction: Being “good” in a science domain is not just about knowing facts (content aspects), but also about being able to apply this knowledge (performance aspect). Modern school science standards have adapted these two elements, with disciplinary core ideas (or crosscutting concepts) representing content ideas and scientific practices representing the performance elements (e.g., NGSS, 2013). With regard to the cognitive processes mentioned earlier and their limitations, it thus appears fruitful to extend a domain-general perspective through a more detailed, discipline-specific perspective—for example, scientific practices.
Use of Representations.
Representations in test items can both decrease or increase item difficulty and/or item processing time, depending on their function and features and the resulting cognitive load (e.g., Lindner et al., 2017). For example, the addition of unnecessary graphical elements increases processing time, but not item difficulty. In contrast, the requirement to analyze data from graphs requires an additional skill and can increase item difficulty (Prenzel et al., 2002; Mesic and Muratovic, 2011; Florian et al., 2014; Strobel et al., 2019). In school science education, redundancies are often used, and students are presented with the same information in a combination of depictive (e.g., graphs), descriptive (text), and symbolic (e.g., formulas) representations to decrease cognitive processing load (Ainsworth, 1999; Schnotz, 2005; Wernecke et al., 2016). As the effect of representations on students’ learning and item difficulties is so varied, categorization systems (Slough and McTigue, 2013) can illuminate the functions of depictive or symbolic representations (Schnotz, 2005) and their interactions with text.
Research Questions and Goals
Specialized learning opportunities are required for high performers and and students particularly interested in science. Science Olympiads, such as the IBO in the field of the life sciences, try to cater to these needs. Identifying characteristics of Science Olympiad exams can improve assessment quality and transparency of future Olympiads, but also has implications for broader life science education. We analyze IBO assessments as an example of a top-tier student competition in the life sciences, as there is as yet very little understanding about the characteristics of competition exams (cf. Köhler, 2017, pp. 31–33). Our work is guided by two research questions:
RQ 1: What are the characteristics of IBO practical and theoretical exam items with regard to their formal, content, practice, and cognitive characteristics, as well as their integration of representations?
RQ 2: How did IBO theoretical exam items develop over the course of IBO’s history with regard to these characteristics?
METHODS
Approach
We conducted an item analysis following principles of qualitative content analysis (Mayring, 2014), in which both theoretical and practical IBO assessments were categorized using a purpose-built analytical framework. The applied procedure is similar to that of previous item analyses (Florian et al., 2014, 2015; Köhler, 2017; Prenzel et al., 2002), albeit with a larger range of categories and an orientation toward science competitions and high-performer education programs. The analytical framework was developed based on theoretical and empirical backgrounds (deductive elements), as well as on the analyzed material itself (inductive elements). The latter elements refer to the definition of lower-level categories in the framework, as well as the category definitions. After developing this framework and using it to characterize IBO items, we analyzed the resulting categories quantitatively regarding differences in item characteristics between IBO years.
Exam Backgrounds and Item Sample
A typical IBO assessment consists of two elements: first, approximately 100 tasks in the form of “theory” exams (i.e., closed-ended assessment items), which students have to answer within six hours; and second, three to four “practical” exams (i.e., hands-on laboratory activities), for which students typically have another six to eight hours altogether. In recent years the practicals fell into approximately 20 separate passages of related content and procedures, which we considered as units of analysis (items) for our study.
The development of all IBO exams is guided by international item-authoring guidelines, but nonetheless varies between hosts. The development process is extensive: First, a group of specialists from different biological domains develops, reviews, and adapts an item pool over a period of usually two or more years. Practical exams are typically piloted with university students or IBO alumni to ensure clarity of instructions and feasibility of hands-on activities. Theoretical exams are less formally piloted than other large assessments (e.g., regarding psychometric properties) due to the difficulty of obtaining an appropriate piloting sample. The development of these tests’ suitability hence relies at least partially on expert opinion. After a host country finishes item development, an international panel of approximately 10–20 life science researchers and educators review, correct, and change all tests in the week before the IBO competition. In a last step, all theoretical and practical tests are thoroughly discussed and revised by the international IBO jury at the beginning of the IBO week (e.g., IBO 2019: ca. 271 scientists from 72 countries).
Figure 1 provides an example item from an IBO theory exam.
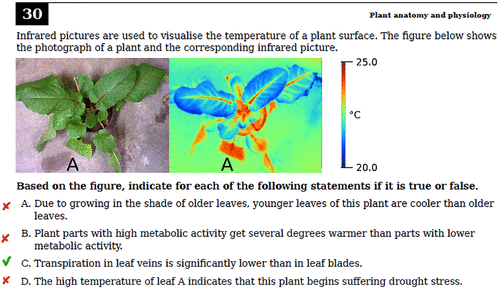
FIGURE 1. Example item from an IBO theoretical exam (IBO 2013/Switzerland, theory exam 1, item 30). The example was chosen for the sake of accessibility. The comparatively short item has a low level of technicality but is rather difficult. Similar to the majority of other (current) IBO items, students are required to use their factual and conceptual background knowledge to make sense of provided data/representations. Note: Many IBO theory and practical tests are freely accessible online and can be adapted for educational purposes: www.ibo-info.org/en/info/papers.html m = mean; SD = standard deviation; p = item difficulty expressed as the share of IBO participants solving all item answering options correctly (range 0–1).
Item characteristics | Sample item | All theory items, m (SD) |
---|---|---|
Average item readability score (US grade level) | 6.7 | 10.51 (3.28) |
Item length (words) | 115 | 113.26 (60.10) |
Item difficulty (p) | 0.12 | 0.47 (0.25) |
We chose six out of the 28 then available IBO assessment years (i.e., tests from the annual IBO competitions). Table 1 shows the details for the sample. The particular years were chosen to represent four periods in IBO’s assessment history (see Table 1). For the present period, we selected three IBOs to attain a larger item pool for this most recent phase. In total, N = 703 individual items with N = 980 answering options were analyzed.
IBO year (assessment cohort) and location | Period of IBO assessments and characteristics | N theory items | N practical items | N total items |
---|---|---|---|---|
IBO 1993 Utrecht, Netherlands | IBO’s founding years: Short items with heavy focus on reproduction | 140 | 4 | 144 |
IBO 1998 Kiel, Germany | First revision: Elaboration of an assessment strategy; evaluation through educational experts | 120 | 12 | 132 |
IBO 2009 Tsukuba, Japan | Second revision: Movement away from reproduction toward data analysis | 88 | 20 | 108 |
IBO 2013 Bern, Switzerland | Present: Computerized testing; focus on authentic contexts, scoring based on multiple true-false statements | 92 | 22 | 120 |
IBO 2014 Bali, Indonesia | 96 | 17 | 113 | |
IBO 2017 Coventry UK | 92 | Not available | 92 | |
Total | 628 | 75 | 703 |
In our sample, we observed approximately eight times as many items from theory than from practical exams. Furthermore, the number of items varied between assessment years, especially among the practicals. We analyzed data separately for theory and practical exams, and we chose to present findings as standardized scores (i.e., counts/100 items) to take this into account. Due to the small and varied number of practical items, we decided to report averages across all analyzed IBO practicals. We hence do not focus on trends across time for this exam type (see Research Question 2).
Baseline Categorical Framework and Test Coding
A concise baseline categorical framework was developed as a first step. The respective categories, subcategories, and traits for each category were based on prior studies or on educational frameworks (e.g., curricula). This initial categorical system was tested on items from one Olympiad assessment year (96 items). The test coding had the following purposes:
Critically check whether the selected categorical system covers the traits of IBO items well: Exclude categories that do not differentiate between items. Identify missing item traits that required additional categories of analysis.
Test available coding instructions from prior studies or reference frameworks that the selected categories stemmed from.
Gather experience rating the advanced IBO items.
The final categorical framework applied in this study was developed based on the experiences with this test coding.
Construction of the Final Item Analysis Framework
The selected categories for the final item analysis framework fall into the four areas of item characteristics presented in the Theoretical Background section: formal characteristics, scientific content and practices, cognitive aspects, and use of representations. Table 2 lists the respective categories of analysis and example traits, as well the source(s) each category is based on. Supplemental Material 1 provides a detailed version of Table 2, including all categories, traits, exemplary coding instructions, and example items.
Category | Example traits: “The item represents the aspect…” | References |
---|---|---|
Area 1: Formal item characteristics | ||
Response type | Complex multiple choice | Marso and Pigge (1991) |
Language: readability (Flesch-Kincaid, Gunning-Fog, and SMOG readability formulas) | U.S. grade level (e.g., 11.3) | Gunning (1969); McLaughlin (1969); Kincaid et al. (1975) |
Area 2: Content and practices | ||
Disciplinary core ideas | Structure and function Steering and regulation | KMK (2004); NGSS (2013) |
Scientific practices | Asking questions and defining problems Analyzing and using data | KMK (2004); NGSS (2013) |
Context authenticity:
| Yes/No | Adapted from Weiss and Müller (2015) |
Biological domain | Cell biology | IBO Operational Guidelines, information available at www.ibo-info.org/rules-guidelines.html |
Taxonomic order | Primates | Various |
Organizational level | Organism | Solomon et al. (2011) |
Area 3: Cognitive aspects | ||
Type of knowledge | Factual knowledge Procedural knowledge | Anderson and Krathwohl (2001); Bloom et al. (1956) |
Cognitive processes | Understand Analyze | |
Area 4: Use of representations | ||
Representation type | Depictive | Schnotz (2005) |
Representation functions | Systems reference (low to high) Use of captions (low to high) Semantic relationship: text and graphic (e.g., decorative, organizational) | Slough and McTigue (2013) |
In addition to the points raised in the Theoretical Background section, the following provides more specific reasons for the selection of categories in the four areas of analysis.
Formal Item Characteristics.
To approximate the difficulty of language used in the advanced IBO test items, computerized measures of readability were calculated for each IBO test item. Multiple such measures have been developed (Fry et al., 2003) and used widely in research (e.g., Yasseri et al., 2012; Wang et al., 2013). Based on measures of syntactic (e.g., length of sentences) and semantic difficulty (e.g., frequency of words with multiple syllables), these measures estimate a U.S.-equivalent school grade level for which the text would be most suitable. For our purposes, we calculated averages across three commonly used readability indices: Flesch-Kincaid (Kincaid et al., 1975), Gunning-Fog (Gunning, 1969), and simple measure of Gobbledygook (SMOG) (McLaughlin, 1969, see: https://en.wikipedia .org/wiki/SMOG). While these measures provide an objective means to compare texts from different sources (here, e.g., IBO years), they cannot provide finer-grained insights (e.g., regarding text coherence).
Content and Practices.
We oriented our analysis along high school biology/science standards to provide reference to IBO contestants’ high school background. However, as IBO’s participants stem from more than 70 countries, an “average” curricular reference point was not feasible. Therefore, we selected two life science/biology standards to serve as examples (United States: NGSS, 2013; Germany: Ständige Konferenz der Kultusminister der Länder in der Bundesrepublik Deutschland [KMK], 2004). These standards were chosen because they make use of discipline-specific “core ideas” and “scientific practices.” These two curricular elements appeared significant for three reasons:
Relevance for IBO: The two elements were identified in the test rating as major features in recent IBO items.
Relevance for research and assessment: Disciplinary core ideas and scientific practices are focus points of major contributions to disciplinary education research (e.g., Schwarz et al., 2009; Fortus et al., 2019) and large-scale assessments (e.g., Prenzel et al., 2002).
Curricular trends: Disciplinary core ideas and scientific practices are structural curricular features that can be found in similar form in several nations’ current biology education standards (e.g., Eleftheria et al., 2016).
In addition to the references to curricula regarding content and practices, we also analyzed the items with regard to a focus on the nature of science (NoS), as underlined in other educational frameworks (Harlen, 2010, 2015; OECD, 2017; cf. Lederman et al., 2001; Conley et al., 2004).
Cognitive Aspects.
In comparing different rating systems for cognitive complexity, the test rating showed that the revised taxonomy of educational objectives (Anderson and Krathwohl, 2001; Bloom et al., 1956; Stern, 2017) was both comprehensive enough to suit the wide range of IBO items but also specific enough to allow us to differentiate between items. In our analytical framework, we also considered whether IBO items cover typical elements of problem solving (e.g., OECD, 2014). However, as our analyses showed that almost none of the IBO tasks appeared as typical problem-solving tasks, our article does not focus on this aspect.
Use of Representations.
The test rating showed that we would (partially) score complex representations, often consisting of several subelements and incorporating different data sources, unlike most standard representation types (e.g., bar charts) found in schoolbooks (Wernecke et al., 2016). For the analyses, we combined Schnotz’s (2005) system of representation types as an umbrella measure with a more specific analysis using elements of Slough and McTigue’s (2013) Graphical Analysis Protocol that focuses on representation functions. Here, we selected “systematicity” due to the biological focus of our analysis; “semanticity” (i.e., connection between text and graphs), due to the parsimonious nature of IBO items; and an analysis of the level of captions used by IBO items. We did not include a detailed analysis of multiple external representations (MERs; e.g., Ainsworth, 1999), as we found only few examples in which the three functions of MER (being complementary, restrictive, and helping knowledge construction) were clearly realized.
Testing, Category Refinement, and Evaluation of the Categorical System
For each category, inclusion and exclusion criteria were further developed after the test coding and examples were added. The coding criteria were developed in an iterative process over multiple weeks by S.O. and a research assistant. We approached the rating largely from the position of a test taker by taking into account all item elements visible in a test situation, that is, stem, representations, and response options (foils). We equally considered correct and incorrect foils. We frequented supplementary online solution commentary published alongside the test items by IBO in those cases where the scope of an item (part) was unclear to us.
We conducted several cycles of coding, training, category refinement, and test interratings to ensure sufficient alignment between raters. Across all of these cycles, 19% of all analyzed items were interrated. The results from the final rating round are reported and include 11% of randomly selected items from all analyzed IBO items. The seemingly small share of items for the final rating has to be seen in regard to the large amount of material analyzed for this study (Syed and Nelson, 2015). Due to the rarity of some item traits, several categories of our system occurred only rarely among the interrated items. As a result, marginal cells in reliability computation of Cohen’s kappa caused nonsensical results (low kappa albeit high agreement). This effect has been reported frequently in the literature and is known as the “kappa paradox” (Cicchetti and Feinstein, 1990; Feinstein and Cicchetti, 1990). Robust alternatives to Cohen’s kappa (e.g., Guildford’s g; Xu and Lorber, 2014) were considered as alternatives but did not apply for our type of data set. As an alternative to the nonapplicable kappa statistic and following recommendation for such cases (Cicchetti and Feinstein, 1990; Feinstein and Cicchetti, 1990), Table 3 provides the percentage of mean agreement between the two raters. Here, overall agreement was high (average: 86%), indicating that different raters can apply our categorical system to arrive at similar results.
Category | Mean interrater agreement (%) |
---|---|
Disciplinary core ideas | 74 |
Scientific practices | 88 |
Context authenticity | 79 |
Knowledge types | 92 |
Cognitive processes | 85 |
Representations | 86 |
Average | 84 |
Statistical Analysis: Differences between IBO Years
In a preliminary analytical step, we determined whether the IBO years were a globally structuring feature of our data set by applying two-step cluster analysis in SPSS (Bayesian Information Criterion as a cluster criterion; preset 2–20 underlying clusters). The results suggested that neither the item years nor the assumed “eras” of IBO exams (see Table 2) are key defining features of IBO items, but rather that the items are structured by more specific item characteristics, for example, individual cognitive processes and scientific processes. Accordingly, we use IBO years only as a reference point to structure our findings, while we do not imply an overall significant difference in item characteristics between these years.
Based on these initial findings, we provide a more detailed analysis on differences between IBO years for individual item characteristics by conducting one-factorial analysis of variance (ANOVA) in SPSS, using robust Games-Howell post hoc tests. To improve readability of our findings, we highlight statistical differences in our results only for those categories of analysis where 1) trends across multiple years were discernible and 2) the ANOVA results suggested significant differences between multiple years.
RESULTS
We report our findings for both research questions in one section, as they are inherently linked. Like earlier sections, this section is structured by the four areas of item characteristics.
Formal Item Characteristics
The item type remained stable across analyzed IBO years with a strong focus on closed-ended formats (single choice, multiple choice, [multiple] true-false) in the theoretical exams (> 90% of items). Practicals used a mix of hands-on activities and asked students to summarize their findings in short open responses (ca. 20%; e.g., filling in blanks, labeling, entering measurements), multiple true-false statements (ca. 26%; e.g., considering possible conclusions from experiments), and open responses (ca. 54%; e.g., graphs or products from laboratory work).
IBO items were strikingly concise with few to no redundancies. However, across the analyzed IBOs, the number of words per item strongly varied for both theoretical (m = 113.26 ± 60.1 SD, range: 15–349) and practical tasks (m = 422.04 words/task ± 314.61 SD, range: 112–1667). The practical tasks consist of sets of procedurally related activities, including extended technical instructions and contextualization. We approximated the share of challenging words with reference to the applied readability formulas (e.g., McLaughlin, 1969) as words with three or more syllables. This measure appeared rather constant across IBO’s theory and practical exams (16–20%), thus suggesting a high technicality of IBO items. Related to this, automated scoring of text readability showed that reading load was high across all IBO years, but roughly corresponded with the contestants’ ages (e.g., U.S. grade equivalent for IBO 2017: 11.23). Nonetheless, many items exhibited such a large share of advanced technical terms that the respective reading level was significantly higher than a typically appropriate reading level for this age group.
Scientific Content and Practices
The share of items focusing on genetics and other molecular-level processes increased over the years (e.g., ca. 20% in earlier years, ca. 40% in 2017: F(5, 618) = 4.93, p < 0.001, η² = 0.04). The majority of items (57%) represented no particular species or species order, with only 11% of items targeting Primates as the most prevalently occurring order. Other re-occurring orders reflected typical model organisms that were covered by the items, for example, Diptera, Poales, Brassicales. Items from all IBOs stretched almost the full spectrum of organizational levels, with foci across all IBOs at the molecular (ca. 30%), organism (ca. 25%), and population levels (ca. 20%).
Disciplinary Core Ideas.
Figure 2 presents the share of disciplinary core ideas (KMK, 2004; NGSS, 2013) across the analyzed IBO years for the theoretical exams and, as an average value across years, for the practicals (see Methods). To improve accessibility, Figure 2 presents only the most relevant core ideas with regard to our findings; refer to Supplemental Material 2 for an extended figure with all core ideas.
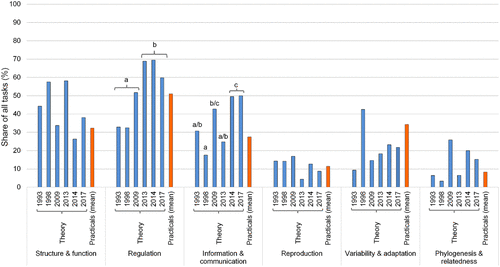
FIGURE 2. Shares (%) of items incorporating different core ideas (KMK, 2004; NGSS, 2013) across six IBO assessment cohorts. Different letters above the columns refer to statistically significant differences between IBO years. They are only displayed for categories were trends across multiple IBO years are discernable. Supplemental Material 2 provides an extended version of this figure with all analyzed core ideas.
The analysis indicates that all core ideas from the biology curriculum are clearly represented in IBO—this is the case for both theory exams and practicals. Less than 1% of the items did not fit any of the core ideas, many of which stemmed from older IBOs that related to short items with a focus on reproduction of highly specific knowledge. Furthermore, IBO items often involved several core ideas, with an increasing trend from 1.9/item (1993) to 2.5/item (2014, 2017).
Particularly the ideas Structure & Function (43% of all theory items), Regulation (53%), and Information and Communication (36%) appeared to receive stronger attention across IBOs, while items concerning Reproduction (12%) or Phylogenesis and Relatedness (13%) were less frequently found. Theoretical and practical exams appeared to have a similar focus in terms of core ideas.
Figure 2 also points at a comparatively large and unsystematic variance between individual IBOs (see, e.g., Structure & Function, Phylogenesis and Relatedness), thus veiling developments over IBO’s history. An increased emphasis in recent years on genetics or other molecular-level pathways can be seen reflected in the significantly higher emphasis on Regulation (F(5, 618) = 2.98, p < 0.001, η² = 0.10) and Information and Communication (F(5, 618) = 8.57, p < 0.001, η² = 0.07). Even though school education places emphasis on evolution as a central theme to guide biology education, the related core ideas (Reproduction, Variability and Adaptation, Phylogenesis and Relatedness) receive comparatively moderate attention in IBO items. Post hoc analysis revealed that the observable trend for Variability and Adaption (see Figure 2; F(5, 618) = 9.67, p < 0.001) was only significant for the 1998 outlier, but not across IBOs.
Besides the findings in Figure 2, our analysis of NoS-related items (e.g., Harlen, 2010, 2015) found only two items that partially referred to this topic (e.g., when touching upon ethical implications of selecting medical tests). However, neither of them provided a clear example of deeper involvement of NoS.
Scientific Practices.
The distribution of scientific practices in IBO items (Figure 3) was less equally distributed than the core ideas (Figure 2), showed clearer differences between theory and practical exams, and had more pronounced trends across IBO years.
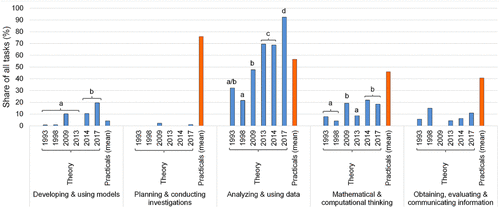
FIGURE 3. Shares (%) of items incorporating different scientific practices (NGSS, 2013) across six IBO assessment cohorts. Different letters above the columns refer to statistically significant differences between IBO years. They are only displayed for categories were trends across multiple IBO years are discernable. Supplemental Material 2 provides an extended version of this figure with all analyzed scientific practices.
The practices Asking Questions and Defining Problems, Constructing Explanations, and Engaging in Argumentation were practically absent (<5% average across IBOs) from both theoretical and practical exams and did not show any significant changes across time (compare extended version of Figure 3 in Supplemental Material 2).
The practice Developing and Using Models appeared rarely, but with increasing frequency (theory exams 1990s: <1%; IBO 2017: ca. 20%, F(5, 618) = 11.3, p < 0.001, η² = 0.08). However, this practice was largely connected to theoretical exams and, more importantly, to students’ application of provided models. Other elements of this practice—for example, model development, critique, or revision—were not addressed. In contrast, Planning and Conducting Investigations was largely limited to practicals (63% average across IBOs), while being almost completely absent from theoretical exams across all IBOs. Similar to the modeling practice, many of the tasks rated for Planning and Conducting Investigations asked students to carry out procedures or draw conclusions from their findings. Few items asked students to take a more active role in the scientific process, for example, by designing or critiquing research plans. Analyzing and Using Data was the practice most widely spread among IBO theoretical and practical items (average: 55%; respectively 57% of items across IBOs), and it was also the one with the most pronounced change over time. Among theoretical items, this practice become approximately three times more prevalent from the 1990s to 2017 (93%, F(5, 618) = 37.84, p < 0.001, η² = 0.23). Mathematical and Computational Thinking was used to varying amounts across IBOs, showing only a rough trend of increasing application (F(5, 618) = 5.19, p < 0.001, η² = 0.04). This practice was incorporated in both theoretical (average: 32% of items) and practical (50%) exams, while calculations were largely limited to the latter exam type.
Context Authenticity.
The trend of increasing numbers of theoretical items involving data analysis (see Figure 3) coincided with steadily more items that addressed authentic pieces of research (1993: 6%; 2017: 48%; F(5, 618) = 29.12, p < 0.001, η² = 0.19). In the practicals, this share of items was high across all IBOs (average: 79%). In contrast, items with relevance to students’ real-world experiences remained relatively low across assessment years (mean theory/practical: 10%/16%). Items coded under this category covered phenomena that students could encounter in nonacademic settings (e.g., media, museums) and focused, e.g., on a specific biological phenomenon associated with the IBO destination or on issues related to the student’s body. While several tasks in more recent IBOs asked students to analyze data from phenomena linked to socioscientific issues (SSIs; e.g., climate change, deforestation, genetic engineering), students were typically not asked to analyze or discuss these items in a typically manner, that is, with complex, ill-structured problems or active argumentation and decision making by the learner (e.g., Klosterman and Sadler, 2010).
Cognitive Aspects
In the application of Bloom’s revised framework (Bloom et al., 1956; Anderson and Krathwohl, 2001), we focus on the items’ cognitive processes (Figure 4) and refer to the respective types of knowledge for additional insights (Figure 5).
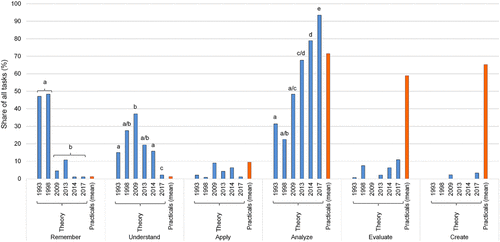
FIGURE 4. Shares (%) of items incorporating different cognitive processes (Anderson and Krathwohl, 2001; Bloom et al., 1956) underlying IBO items for six IBO assessment cohorts. The lower three cognitive processes (remember–understand–apply) are hierarchical in nature. Hence, an item appears only under, e.g., remember if none of the higher-order processes apply for the item. Vice versa, at item coded for understand will almost certainly also include elements of remembering. Different letters above the columns refer to statistically significant differences between IBO years. They are only displayed for categories were trends across multiple IBO years are discernable.
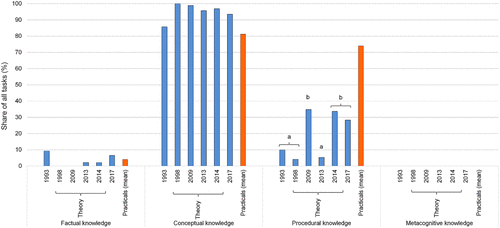
FIGURE 5. Shares (%) of items incorporating different types of knowledge (Anderson and Krathwohl, 2001; Bloom et al., 1956) across six IBO assessment cohorts. The lower two levels (factual- and conceptual knowledge) are hierarchical in nature. Hence, an item for factual knowledge only appears in the graph if conceptual knowledge does not apply. Vice versa, conceptual knowledge almost certainly includes factual knowledge. Different letters above the columns refer to statistically significant differences between IBO years. They are only displayed for categories were trends across multiple IBO years are discernable.
Within the six cognitive processes, the three lower levels (remember, understand, apply) were reported to be hierarchical in nature (Anderson and Krathwohl, 2001; Crowe et al., 2008; cf. Stanny, 2016): Our results are in line with this assumption and support the hierarchical relation of the three lower cognitive processes. Accordingly, Figure 4 records the three lower processes only if none of the higher processes co-occurred in the same item. In contrast, the cognitive processes analyze, evaluate, and create appear independently and in different combinations with each other.
Most notably, the results highlight clear differences in item design between the early IBO years and more recent IBOs: Among the theoretical exams in the 1990s, almovst half of all tasks were purely concerned with reproduction (remembering), while the share of such tasks (ca. 1%) is negligible in most recent IBOs (F(5, 618) = 47.57, p < 0.001, η² = 0.28). However, a look at the underlying types of knowledge (Figure 5) revealed that, across all analyzed IBOs, the required reproduction did not primarily encompass factual (mean across all IBOs: 3%), but rather conceptual knowledge; that is, interrelationships between several facts (mean: 95%).
After the first IBOs with focus on reproduction (1993, 1998), items with understanding (e.g., summarizing, interpreting, explaining) as the main cognitive process appear to peak with the second wave of revisions in IBO exams (2009; Figure 4; cf. compare Table 1; F(5, 618) = 8.96, p < 0.001, η² = 0.07). The subsequent decrease in this category is likely a result of a strong increase in items that included analyzing as the main cognitive process (1990s: ca. 30%; 2017: 93%; F(5, 618) = 43.36, p < 0.001, η² = 0.26), which is also visible in the analysis of scientific processes (Figure 3). As noted earlier, this increase can be linked to the strengthened trend requiring IBO participants to use their prior knowledge when analyzing authentic pieces of biological research.
The application of given procedures (e.g., applying a given formula; Figure 4) as a stand-alone cognitive process in an item appeared rarely across IBO’s theory and practical tasks and did not show a major change across the exam years (mean all items: 4%). According to the hierarchy principle (discussed earlier), application was instead most often part of higher-order cognitive processes. This observation is also visible in the increasing share of items (average across IBOs: 20%) that included procedural knowledge (Figure 5; e.g., knowledge of skills and techniques a; F(5, 618) = 15.45, p < 0.001, η² = 0.11), for which students were not just asked to apply their procedural knowledge, but also to use it as part of, for example, analyzing data.
Items coded under the cognitive process evaluation (i.e., checking or critiquing) required students, for example, to judge the appropriateness of procedures, or the internal/external coherence of data/information. Items including evaluation were rare across IBOs (1–11%; cf. Figure 3). Almost none of the analyzed theoretical items (average: < 1%) required students to create something when designing their own solutions, experiments, or models.
The practical exams were generally characterized by a stronger emphasis on higher-order cognitive processes than the theoretical exams (Figure 4). Here, two-thirds of all items required students to analyze, evaluate, or create, while tasks with relatively simple “cookbook-like” instructions (category apply) were found in less than 10% of tasks. The large share of practicals in the create category (i.e., reorganization of elements into a coherent new unit) has limitations, though: The respective tasks typically asked students to prepare graphs, calculations, or other representations as summaries of their earlier analyses or students had to create certain laboratory outputs. However, few items required students to create products, research plans, or models. A look at the types of knowledge (Figure 5) reveals the emphasis on hands-on activities during practicals, where three-fourths of practical items encompass elements of procedural knowledge.
Finally, neither in the practicals nor in the theoretical items did we observe items that explicitly assessed metacognitive knowledge (e.g., strategic thinking, self-knowledge).
Use of Representations 3
While practical exams used a relatively constant number of representations per item over the years (average: 1.12), the increased emphasis of IBO theoretical items on students’ work with data (see Figures 3 and 4) is reflected in a steady increase in the average number of depictive representations per item: 0.24 (1993) to 2.00 (2017). If multiple representations were used in one item, these were often connected with one another to form a complex unit.
Across both exam types the share of types of representations (Schnotz, 2005) was distributed as approximately 50% depictive logical (e.g., graphs, tables) and approximately 40% depictive realistic (e.g., drawings), with the remainder being symbolic (e.g., formulas) representations. A more detailed look at the types of representations revealed an emphasis on stylized drawings, tables, and graphs, while the remaining range of representations was wide in recent IBOs, including flow diagrams, maps, or outputs of particular laboratory techniques (e.g., gel electrophoresis bands).
On average, IBO’s items appeared to have several unique tendencies when compared with biology schoolbooks’ use of representations (e.g., Wernecke et al., 2016): While the items’ use of representations showed some notable differences between host countries (i.e., IBO years), they consistently made only sparse use of captions across all IBO years: Only approximately 6%/18% (theory/practical) of representations had a formal, detailed heading; 63%/61% were introduced briefly in a short title or in the item stem; while the remaining items had neither captions nor introductions in the item stem (Slough and McTigue, 2013). As systems are considered a disciplinary core idea in biology (e.g., KMK, 2004; NGSS, 2013), we also analyzed the extent of systems references in representations. Across IBO years, the largest share of both theoretical and practical items used either low-level (theory: 36%; practicals: 43%) or intermediate (53%/36%) perspectives on systems, while only a small share of items (11%/21%) provided a high-level systems perspective, including multiple components and a change of the system over time.
Finally, we analyzed semanticity in IBO items, that is, the connection of information provided by an item’s text and representations. Figure 6 shows a clear tendency for both theoretical and practical items across (most) IBOs:4 As the vast majority of representations add new information (connection) to the items’ text, the relationships between text and depictive representations in IBO items causes very few redundancies. The results underline a general feature of IBO items: The representations (especially in recent IBOs) are typically the central meaning–carrying element of the item, especially in theoretical tasks. In more recent IBOs, representations were added to provide more context for the item (e.g., by providing a picture of the researcher behind provided data). This is manifested in the sharp increase of decorative elements at IBO 2017 (F(5, 618) = 87.27, p < 0.001, η² = 0.41).
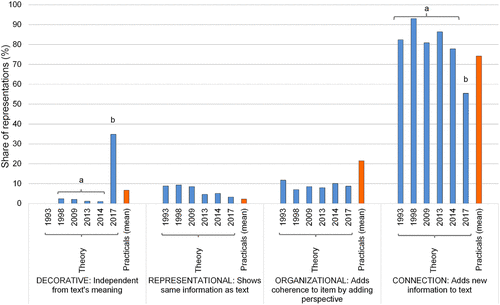
FIGURE 6. Shares (%) of items incorporating different levels of semanticity between text and graphical representations (Slough and McTigue, 2013) across six IBO assessment cohorts. As data are displayed as shares of 100 representations, this figure does not portrait the increasing number of items over IBO’s history. Different letters above the columns refer to statistically significant differences between IBO years. They are only displayed for categories were trends across multiple IBO years are discernable.
DISCUSSION
Conclusion for RQ 1: What Are Characteristics of IBO Exam Items?
Our findings provide insight into the characteristics of assessment items from IBO as an example for a task-based student competition in the life sciences. The findings extend previous insights from general education assessments (e.g., Florian et al., 2014, 2015). Our findings can be used to derive characteristics for a prototypical (recent) IBO test item. Such an item would:
almost certainly be highly concise in language with few to no redundancies;
likely use age-appropriate language, albeit with multiple technical terms;
have a context set out along an authentic piece of biological research that is unlikely based on students’ lives;
almost certainly require test takers to analyze biological phenomena that address multiple disciplinary core ideas;
almost certainly require the analysis of data, provided in oftentimes nonstandard, complex representations that use low-level captions and low/intermediate levels of systems references;
likely include higher-order cognitive processes (theory: analyze; practicals: analyze, evaluate, and create) that incorporate lower-level cognitive processes (predominantly remember and understand) and different types of knowledge (predominantly conceptual knowledge);
unlikely ask the test taker to reflect on her/his domain-specific strategies or actions (meta-cognitive knowledge) or NoS or to evaluate scientific ideas in the frame of SSIs; and
unlikely require (a deep use of) the scientific practices of Asking Questions and Defining Problems, Developing and Using Models, Planning and Conducting Investigations (exception: practicals), Constructing Explanations, or Engaging in Argumentation.
Conclusion for RQ 2: How Did Item Characteristics Change over Time?
Our analysis documents two major changes in assessment strategy over the history of IBO: 1) a process away from reproduction toward analyzing and using data and 2) a process toward stronger contextualization and authenticity of the analyzed phenomena (see, e.g., cognitive processes in Figure 4). By extension, the name “theory” for exams using closed-ended test items appears rather unsuitable: While several items do require students to apply theories (e.g., theory of natural selection) to make sense of observations or data, this is not a systematic feature across IBO theory items; neither do they markedly ask students about specific theories. In contrast, approaches have been presented that systematically require students to structure their explanations of phenomena by building on and evaluating theoretical underpinnings (e.g., Reiser et al., 2001; Sandoval and Reiser, 2004). Unlike the IBO theory exam items, these approaches, for example, include a strong emphasis on students’ epistemological understanding and their ability to construct arguments that draw and weigh explicit connections between claims, underlying theories, and different pieces of evidence.
Despite the observed developments in IBO exams, our findings suggest a relatively large variance in assessment foci between changing host countries (e.g., among disciplinary core ideas). While we did discover several significant and clear trends for specific item characteristics across IBO years, the variance between individual IBOs for other categories (e.g., core ideas; see Figure 2) hid long-term assessment developments. Varying foci by IBO host countries are to be expected and may be explained, for example, by foci in national educational norms or expertise areas of item developers. However, the variance between individual years suggests that the transparency about the content and structures underlying IBO exams is not yet ideal. As a result, it is hard to align the preparations (i.e., individual studying, lower-level national competition rounds) with the content and procedural skills that will be tested at the international competition. To help in this point, host countries announce topics beforehand and sometimes include additional information on the laboratory skills required for the practicals. Nonetheless, the country coordinators who coach teams through the students’ long preparatory phase must rely, at least to some degree, on educated guesses and years of experience at IBO.
Our findings suggest that the observed variance between IBO years is more tied to biological content and practices (e.g., type and number of core ideas, type of scientific practices as well as the corresponding cognitive processes) and less to general constructs (e.g., item length, difficulty of reading load). This is likely because the latter are more bound to conventions in the IBO community. Our observation also points at a structural difference between IBO assessments versus standardized school assessments (e.g., OECD, 2017): While IBO assessments appear to prominently vary items along content dimensions, general education assessments often create variation in item pools by systematically crossing content ideas, procedural elements, and aspects of complexity or contexts (e.g., Kauertz et al., 2010; NGSS, 2013; OECD, 2017).
Learning Opportunities for High Performers: Perspectives from IBO
Via their exams, IBO seeks to challenge gifted students’ abilities in the life sciences. In comparison to educational frameworks for science learning (e.g., KMK, 2004; NGSS, 2013; OECD, 2017), IBO’s approach appears to differ in several aspects: These frameworks appear to employ, for example, a deeper perspective on systems, a broader range of scientific practices (e.g., development of models or research plans, argumentation), more focus on the NoS, socioscientific issues, and the application of metacognitive strategies. Science competitions have been reported to benefit students by deepening the exposition to inquiry learning (Tuan et al., 2005; Dionne et al., 2012). Hence, the low focus on related scientific practices (e.g., ask questions or to develop research designs) in IBO exams is surprising. Similarly, low shares of inquiry-focused items have been reported for the International Junior Science Olympiad (Köhler, 2017, p. 51).
However, this surprising absence of contemporary elements of science education in competition exams should not be interpreted in the sense that these elements are not deemed relevant by the IBO community: IBO’s theoretical exams are particularly strongly guided by regulations regarding the assessment format (closed-ended, conciseness of text). Hence, the low regard for the mentioned elements is likely related to the fact that these are much harder to assess via closed-ended formats than other elements (e.g., data analysis). This has also been shown in previous studies, which found that closed-format items have limitations for the assessment of higher-order cognitive processes, particularly regarding the creation of artifacts or designs, for example (Crowe et al., 2008; Lindner et al., 2015).
It should also be noted that the mentioned minor or missing elements in IBO exams could nonetheless play a role regarding student participation in IBO, as they may obtain higher significance in national biology competitions that students have to qualify in before entering the international level.
Implications and Perspectives
IBO.
Our findings are relevant for IBO’s assessment strategies. The current focus on closed-ended item formats seems a reflection of the narrow time schedule of IBO competitions. This likely introduces a limit to what is being tested. A downside of using closed-ended items at IBO is the difficulty related to their piloting, as comparable (international) samples are difficult to access and approximations have to be done by working with university students or IBO alumni. This means it is difficult to identify nonnormative student conceptions that can be used to set up incorrect item foils, as is usually done in closed-ended assessment item design. Extending answer formats to complex closed-ended items such as mappings, filling in, or extending representations, as well as to multitier items or short closed-ended formats might be a way to better get students to apply their knowledge and become more engaged in different scientific practices. Some of these formats have been tested in recent IBO exams. Thus, opening up IBO’s assessment strategy could better include important elements identified by science standards and international assessments (KMK, 2004; Klosterman and Sadler, 2010; NGSS, 2013; Lindner et al., 2015; OECD, 2017).
Several “practical skills” have been defined in IBO’s Operational Guidelines.5 These encompass both domain-general skills (e.g., hypothesis formulation) and domain-specific biological skills (e.g., staining of slides, gel electrophoresis). Most of the elements we found to be absent or underrepresented in IBO items do not appear in the competition rules (e.g., Constructing Explanations, NoS, SSIs), while others do appear in the regulations, but are apparently rarely realized in the developed tasks (e.g., Defining Hypotheses, Experimental Designs and Variable Control).
These findings, as well as the observed variance between IBOs suggest that IBO’s guidelines could profit from a revision to include a more detailed framework for item development, as is realized in large-scale assessments (e.g., OECD, 2017). Inspirations for the revision of IBO’s assessment framework can first of all be seen in international science education and assessments standards like the PISA framework or OECD’s Future of Education and Skills: Education 2030 (e.g., OECD, 2017, 2018). These, as well as national science standards (e.g., NGSS, 2013), address the absent elements described earlier. Such a step will improve the degree to which IBO assessments target and deepen future IBO competitors’ school learning opportunities. In addition, researchers have presented more specific frameworks for constructing assessments for particular fields of life science education. For example, approaches to constructing assessment for developing hypotheses and designing investigations (e.g., Brownell et al., 2013; Deane et al., 2014), using/developing models (e.g., Schwarz et al., 2009), constructing argumentation (e.g., Osborne et al., 2016), or for the NoS (e.g., Lederman et al., 2001) have been proposed and could guide the development of future IBO assessment design standards.
The extension of IBO assessment criteria for these fields would increase transparency for students and tutors regarding underlying assessment criteria. An increased transparency about exam characteristics could especially help less experienced IBO members in preparing their students for the exams.
General Education and Assessment.
Our findings highlight relevant abilities that a large, international community of life science education experts (i.e., the IBO jury) regard as relevant for future life science skills. The following points summarize possible implications for general education with regard to identified characteristics of IBO exams.6
Performance on Hands-On Activities.
Throughout IBO history, the competition has put strong emphasis on students’ performance in hands-on laboratory work (practical exams). Some aspects of procedural knowledge can also be tested in paper-and-pencil exams (e.g., sketching a research procedure), but carrying it out and overcoming connected obstacles requires different skill sets. This becomes apparent in our study in the different emphasis of theory versus practical exams (see Figures 4 and 5). In many countries, school exams still appear largely based on written exams. Recent science education/assessment frameworks (e.g., NGSS, 2013; OECD, 2017) have underlined the relevance of knowledge-in-use (Harris et al., 2015), stressing that content knowledge has to be applied using scientific practices (and, e.g., aspects of epistemic knowledge or interdisciplinary ideas) to explain phenomena. By extension, IBO’s focus on hands-on activities should be a reminder to general education to more widely include practical skills in science classrooms and assessments (Schwichow et al., 2016).
Insight to Validity: Disciplinary Core Ideas in IBO.
The full range of disciplinary core ideas for biology (KMK, 2004; NGSS, 2013) clearly surfaced throughout the analyzed IBO assessment items as a major structuring element of recent science curricula. Furthermore, our analysis did not uncover additional core ideas from IBO exams other than those from the science standards. Almost all of the analyzed items matched at least one, often several, of the core ideas. We find these results noteworthy with regard to two observations: 1) Many of the analyzed IBO test items were written before the educational frameworks and core ideas were developed. 2) IBO assessments are authored each year by changing groups of life science experts typically less familiar with the mentioned educational standards and core ideas. The item developers use life science phenomena addressed by current research as a basis to design test items. As these authentic life science phenomena appeared to cover the curricular core ideas well, we conclude that our findings strengthen the claim for content validity of the employed system of core ideas (KMK, 2004; NGSS, 2013).
Analyzing and Using Data Is a Key Scientific Practice for the Life Sciences.
Among the multiple scientific practices and skills defined in the science standards (e.g., KMK, 2004; NGSS, 2013), Analyzing and Using Data stood out in IBO exams as the most frequently used practice. As described, this focus might partially be an artifact of the item formats employed. However, a central position of this practice in the life sciences seems plausible: First, analyzing functions as an umbrella-like cognitive process by including lower-order cognitive processes (e.g., Crowe et al., 2008). Second, the analysis of representations revealed that IBO expects high performers in the life sciences to possess a strong literacy in reading representations: IBO participants have to analyze multiple types of standard and complex data representations without much help from captions and without being able to rely on additional information in text form (Figure 6). Prior studies reported that curricular foci that proved effective for high-performing students can also provide useful learning opportunities for students of average performance (VanTassel-Baska et al., 2009; Subotnik et al., 2011; Neubrand et al., 2016). By extension, students’ abilities to quickly access information from different types of representations is not just relevant for high performers. Practicing this in school biology more intensely would likely contribute to students’ ability to understand life science phenomena (OECD, 2017).
Value of Using Closed-Ended Item Formats.
Earlier, we argued for the value of extending IBO’s assessment format repertoire with regard to incorporating, for example, a wider range of scientific processes. Nonetheless, IBO’s 30-year experience with exams and our analyses lead us to conclude that closed-ended formats do allow the assessment of a wide range of abilities at a sophisticated level. Other studies have drawn similar conclusions (Crowe et al., 2008; Lindner et al., 2015). The ease of application of closed-ended items enables school science education to use these formats more widely and combine them with open-ended and hands-on assessments for those aspects that are hard to test via closed-ended formats (e.g., argumentation, designing and testing inquiry activities).
IBO Exams Are a Teaching and Assessment Resource.
The published IBO test items represent an impressive knowledge stock, as they encompass hundreds of authentic biological contexts, many of which cover recent findings in the life sciences. While the test items are most likely too difficult for the wide performance spectrum of secondary students, the items’ contexts can provide educators with ideas for designing assessments or teaching materials. Additionally, all general education assessments also need to develop difficult items. IBO items can provide a stock for these developments, and our findings can provide suggestions for possible item features suitable for such tasks.
Future Research.
Linking to previous studies (e.g., Prenzel et al., 2002; Florian et al., 2014), the derived item features can be used in regression models or cluster analyses to determine, for example, relevant traits that predict item difficulty. Additional studies could extend our research by comparing assessment strategies and organizational frames of national competitions from different educational backgrounds, thus determining how they are linked to team success at the international level. Future analyses of Science Olympiad exams could more specifically define the standards by which highest performance is defined in this domain. As upper anchors in performance expectations, these findings can provide a valuable perspective for the development of national science standards and assessments (Subotnik et al., 2011; Alonzo and Gotwals, 2012).
Limitations
The ability to identify trends over time in IBO’s assessment strategy is limited by the large volume of items per IBO and the resultant small number of analyzed assessment years. While our selection of these years was criteria based, the chosen years are not fully representative. While some parts of the observed variance between IBO years is likely related to individual foci set by the hosting countries, other parts can be related to more long-term changes in educational strategies set by the IBO community. Innovations in the assessment strategies of IBO exams have been introduced by individual host countries and then taken over in later years if they proved successful (e.g., computer-based assessments in IBO 2013). However, to observe these steps empirically, a much larger sample of IBO years would be required.
We used IBO as an example for task-based competitions in the life sciences. The comparability between this and other science competitions has limitations, for example, with regard to different item formats. However, multiple similarities across comparable competitions can be found in their organization, the use of both theoretical and practical assessments, or restrictions in the length of items (e.g., Eisenkraft and Kotlicki, 2010).
In the light of prior research on competition participation/success factors (e.g., Dionne et al., 2012; Urhahne et al., 2012) and competition outcomes (e.g., Abernathy and Vineyard, 2001; Campbell and Walberg, 2010), our analysis of exam characteristics addresses only one of several factors making up the unique experience of a Science Olympiad. While we provided examples of how our findings can be put to use, future studies should also shed light on the effects of competitions on students’ domain-specific learning, the effect on their social preferences (e.g., openness, willingness for international collaboration), and their affective-motivational dispositions (e.g., Subotnik et al., 2011).
FOOTNOTES
1 That is perceptions of oneself with regard to a domain/perceptions of one’s abilities to accomplish in a domain (Huang, 2012).
2 The names for “theory” and “practical” exams refer to the names conventionally used in the IBO community. Even though we discuss the term “theory exam” critically later, we use both terms throughout the article to simplify orientation if readers access the exams on the competion’s website.
3 For the sake of readability, we use the term “representations” in this section, if not stated otherwise, as an umbrella term for “depictive representations” and symbolic “representations.”
4 Because the data in Figure 6 are reported as shares of all representations, the decrease in the connection category (F(5, 618) = 15.23, p < 0.001, η² = 0.11) is an effect caused by an increase in the number of items with decorative function.
5 These are IBO’s competition rules and regulations. Accessible at: www.ibo-info.org/rules-guidelines.html.
6 We do not draw implications based on item characteristics that were absent from IBO exams (e.g., NoS, metacognitive knowledge). The absence of these elements does not imply that the IBO community regards the elements as irrelevant for life science education.
ACKNOWLEDGMENTS
We thank Jasmin Colakoglu for conducting preparatory works for this study. The IBO Steering Committee and IBO community provided valuable feedback in developing our analysis plan. Two anonymous reviewers and the editor contributed substantially to the quality of this work - we are highly grateful for their expertise and guidance.