Probing Internal Assumptions of the Revised Bloom’s Taxonomy
Abstract
Bloom’s taxonomy is a classification of learning objectives originally developed for general educational purposes. The taxonomy was revised to expand beyond cognitive processes and to include knowledge types as an orthogonal dimension. As Bloom’s taxonomy is a tool widely used in biology education by researchers and instructors, it is important to examine the underlying assumptions embedded within how people may implicitly understand and use the taxonomy. In this paper, we empirically examine two major assumptions: the independence of the knowledge-type and cognitive-process dimensions and the use of action verbs as proxies for different cognitive processes. Contingency analysis on 940 assessment items revealed that the knowledge-type and cognitive-process dimensions are related and not independent. Subsequent correspondence analysis identified two principle axes in how the two dimensions are related, with three clusters of knowledge types and cognitive processes. Using the Shannon evenness index, we did not find a clear relationship between question prompt words (including action verbs) and cognitive processes in the assessment items. Based on these results, we suggest that both dimensions of the revised Bloom’s taxonomy should be used and that question prompt words or action verbs alone are not sufficient in classifying the embedded learning objectives within assessment items.
INTRODUCTION
In recent years, reform in undergraduate science education has called for the development of skills such as problem solving and critical thinking to assure the successful transition from education to employment (National Research Council [NRC], 2003, 2007, 2012; National Academies of Sciences, Engineering, and Medicine [NASEM], 2018). As educators, we should work to ensure that classroom assessments are aligned with our educational goals (Wiggins and McTighe, 2005; Astin and Antonio, 2012). Alignment is critical for our understanding of what educational interventions and instructional practices lead to the results we aim to achieve (Handelsman et al., 2007). However, assessments as an aspect of alignment have historically lagged behind other curricular innovations (Orpwood, 2001; Pellegrino, 2013), despite explicit guidance from policy documents.
Researchers have developed a number of classification systems for the purpose of improving curricular alignment. Biggs’s structure of the observed learning outcome (SOLO) classifies learning outcomes of student work in terms of complexity (Biggs and Collis, 1982). The Three-Dimensional Learning Assessment Protocol (3D-LAP), developed based on Next Generation Science Standards (NGSS; NRC, 2013), breaks down learning into three dimensions, including scientific and engineering principles, crosscutting concepts, and disciplinary core ideas (Laverty et al., 2016). This study focuses on another commonly used classification system: Bloom’s taxonomy (Bloom et al., 1956; Anderson et al., 2001).
The motivation behind the creation of the original Bloom’s taxonomy was to shed light on the behaviors that are important to student learning (Bloom et al., 1956). The taxonomy has been studied extensively and was ultimately revised in 2001 (Anderson et al., 2001). Bloom’s taxonomy has been adopted as a research and educational tool by many disciplines, from mathematics to music (Hanna, 2007; Starr et al., 2008; Halawi et al., 2009; Karaali, 2011; Coleman, 2013). In undergraduate biology education, Bloom’s taxonomy is widely used in assessing course alignment (Crowe et al., 2008; Jensen et al., 2014), identifying assessment objectives in introductory courses (Momsen et al., 2010, 2013) and mapping out strategies to write effective assessments (Bissell and Lemons, 2006; Lemons and Lemons, 2013; Arneson and Offerdahl, 2018).
Assumptions are embedded within any categorization system, and the meaning and validity of conclusions drawn from a system are situated in such assumptions. Articulating and testing embedded assumptions will help us better understand the tools we are using in research and instruction. For example, Bigg’s SOLO levels are assumed to correspond with Piaget’s levels of child development in that they both require mastery of earlier stages to master future stages of abstraction (Biggs and Collis, 1989). The 3D-LAP relies on the potential for an assessment item to elicit a particular type of learning in a student, which assumes the features described in their three dimensions are at least somewhat valuable proxies for student cognition (Laverty et al., 2016). For a system as widely used as Bloom’s taxonomy, it is important for researchers and instructors to contemplate the underlying assumptions embedded within the structure of the taxonomy, especially as the taxonomy is revised and used in new ways. Here, we articulate and examine key assumptions embedded within how people use Bloom’s taxonomy.
Evolution of Bloom’s Taxonomy
In the original taxonomy, learning was organized into six cognitive-process categories: knowledge, comprehension, application, analysis, synthesis, and evaluation. (In this paper, we will use italicized words when referring to Bloom’s taxonomy categories.) Each category had certain associated cognitive behaviors or action verbs, for example, recalling and recognizing for knowledge or defending and judging for evaluation (Bloom et al., 1956). However, the cognitive-process categories of the taxonomy were never presented or conceived of as equal subdivisions; rather, they were structured as levels of a cumulative hierarchy organized from simple to complex and from concrete to abstract. This structure assumes a linear progression of the six cognitive-process categories, with links between adjacent levels (e.g., from knowledge to comprehension) but not between nonadjacent levels (e.g., from knowledge to application; Stoker and Kropp, 1971). In other words, performance on knowledge questions should directly predict performance on comprehension questions but not performance on application questions or any other subsequent cognitive-process categories.
Because of the wide reception of the original Bloom’s taxonomy, studies examined the underlying assumptions of the cumulative hierarchy structure (Furst, 1981; Kreitzer and Madaus, 1994; Booker, 2007). Empirical evidence based on student performance data indicated that: 1) an underlying factor predicts performance on assessment items in all six cognitive-process categories (Madaus et al., 1973); 2) the knowledge category may be part of a different structure (Seddon 1978; Hill and McGaw, 1981); 3) direct connections exist between nonconsecutive categories, such as comprehension and analysis (Hill and McGaw, 1981); and 4) the synthesis and evaluation categories are swapped in terms of complexity (Kropp et al., 1966). Furthermore, researchers and instructors alike found it difficult to distinguish between the categories or found the distinctions not helpful (Colder, 1983). These challenges prompted a revision of the taxonomy (Table 1).
Problems | Revised features |
---|---|
Knowledge as an underlying factor distinct from cognitive processes | Separate knowledge into a dimension orthogonal to the cognitive processes |
Direct connections between nonconsecutive categories | Loosen interpretation of the cumulative hierarchical structure in practice |
Synthesis and evaluation categories swapped in terms of complexity | Change the order of create and evaluate categories in the cognitive processes |
Distinctions among the different categories unclear in practice | Clarify ambiguities by creating extensive subcategories for each category |
The revised taxonomy sought to clarify ambiguities among the different categories in each of the two dimensions by creating extensive subcategories. This expansion of subcategories was done in response to the difficulties of distinguishing the different categories, thus limiting the usability of the original taxonomy (Colder, 1983). For example, instead of simply having an application category in the original taxonomy, apply in the revised taxonomy is subdivided into executing routine procedures in a familiar task versus choosing and then implementing a procedure in an unfamiliar task (Anderson et al., 2001). Analogously, conceptual knowledge is subdivided into knowledge of classifications and categories, knowledge of principles and generalizations, and knowledge of theories, models, and structures (Anderson et al., 2001). The revised taxonomy suggests that, when categorizing a learning objective or assessment item, one should look to the subcategories for specificity and to help place the objective or assessment in a larger category (Krathwohl, 2002).
Even though the cumulative hierarchy model of the original taxonomy did not have a strong basis in evidence, the revised taxonomy holds steady in its theoretical interpretation that both the knowledge-type and cognitive-process dimensions are organized from simple to complex and from concrete to abstract, perhaps with some potential overlap at either end of each category (Krathwohl, 2002). This conceptualization was motivated by the desire to move from rote learning to meaningful learning, with the assumption that more complex knowledge types and cognitive processes are more meaningful (Anderson et al., 2001). However, the revised taxonomy acknowledges that the cumulative hierarchy is not strict and instead suggests a relaxed hierarchy (Anderson et al. 2001). In practice, mastery of the simple categories may not necessarily be required for mastery of more complex categories (Krathwohl, 2002; Agarwal, 2019). Researchers found empirical evidence for a relaxed hierarchy within the cognitive-process dimension, with overlap occurring among nonadjacent levels (Hill and McGaw, 1981, Agarwal, 2019). Because the assumption of the cumulative hierarchy has been extensively studied within the original and revised taxonomies, we will not be focusing on this assumption in our study. In the following sections, we outline two key features of the revised Bloom’s taxonomy and their underlying untested assumptions, which are embedded within how people use the taxonomy in research and practice.
Assumption 1: Independence of Dimensions
The revised taxonomy consists of two dimensions, acknowledging the empirical evidence that knowledge types make an orthogonal dimension to cognitive processes (Seddon 1978; Hill and McGaw, 1981). Any learning objective or assessment item requires some form of knowledge and a cognitive action to perform with that knowledge (Kreitzer and Madaus, 1994; Krathwohl, 2002). The revised taxonomy classifies knowledge into four types: factual, conceptual, procedural, and metacognitive (Anderson et al., 2001). Each assessment item should be classified by the intersection of a knowledge type and a cognitive process (Krathwohl, 2002).
In the revised taxonomy, the knowledge-type and cognitive-process dimensions are conceived as independent, with student learning happening at the intersection (Krathwohl, 2002). This structural element is evident in the presentation of every combination of knowledge type and cognitive process as possible. Researchers and instructors alike, especially in biology education, tend to only use the cognitive-process dimension (Allen and Tanner, 2002; Crowe et al., 2008; Momsen et al., 2010, 2013; Freeman et al., 2011; Jensen et al., 2014; Thompson and O’Loughlin, 2015; Semsar and Casagrand, 2017; Lalwani and Agrawal, 2018). The exclusion of the knowledge-type dimension may stem from two potential explanations: 1) Knowledge type does not matter to biology education researchers and instructors for the questions they are asking, and/or 2) people implicitly assume that the two dimensions are so closely associated that only one is needed when using Bloom’s taxonomy. Many policy documents involve both knowledge-type and cognitive-process dimensions, suggesting that the first explanation is unlikely to hold true (Table 2). Therefore, it is important to examine the independence of the two dimensions of Bloom’s taxonomy, as researchers and educators may implicitly believe that the dimensions are correlated enough that the cognitive-process dimension alone is sufficient for describing student learning.
Framework | Content | Action | Intersection |
---|---|---|---|
Revised Bloom’s taxonomy | Knowledge Type | Cognitive process | Learning objective |
V&C | Core concepts | Core competencies and disciplinary practices | Biological literacy |
NGSS | Disciplinary core ideas, crosscutting concepts | Science and engineering practices | Standards |
AP Biology | Big ideas, enduring understanding | Science practices | Performance expectations |
MCAT | Foundational concepts, content categories | Scientific inquiry and reasoning skills | Problem solving |
Assumption 2: Verbs as Proxies for Cognitive Processes
The revised cognitive-process dimension shifts the categories into their verb forms to emphasize the action focus (Krathwohl, 2002). This dimension includes: remember, understand, apply, analyze, evaluate, and create. To account for some of the psychometric inconsistencies identified in previous studies (Kropp et al., 1966; Seddon 1978; Hill and McGaw, 1981), the new remember category takes the place of the original knowledge category, and synthesis becomes create and switches with evaluate in terms of complexity.
In the original taxonomy, each category had certain associated cognitive behaviors or action verbs, for example, recalling and recognizing for knowledge or defending and judging for evaluation (Bloom et al., 1956). In the revision, the cognitive-process categories and subcategories were codified as verbs themselves (Krathwohl, 2002). Perhaps this change reinforces the assumption that verbs could be proxies for their associated cognitive actions. In other words, someone looking to write assessment items in a certain category could simply include the verbs of that category. In this assumption, using the verb “judge” will automatically require students to engage in the cognitive process of evaluate. Such associations have continued as a common practice (Stanny, 2016), including attempts to automate classification of assessment items by Bloom’s taxonomy using verbs alone (Omar et al., 2012).
Research Questions
The two assumptions, that is, independence of dimensions and verbs as proxies, are both evident in how researchers and instructors use Bloom’s taxonomy today and have not been empirically examined. Therefore, our research questions are as follows:
Are the knowledge-type and cognitive-process dimensions independent?
Can the verbs embedded within assessment items be used as proxies for cognitive processes in Bloom’s taxonomy?
METHODS
Data Source
Our data set consisted of a total of 940 assessment items. Of these, 834 were from 12 lower- and upper-division courses across eight biology subdisciplines taught by 16 different instructors in the years 2011–2015 (Figure 1). There courses were offered at a private, not-for-profit, large, primarily residential, doctoral university in the midwestern United States, described by the Carnegie Classification of Institutions of Higher Education (McCormick & Zhao, 2005) in the category of highest research activity and with a 4-year, full-time, more selective, and lower transfer-in undergraduate profile. In our preliminary data analysis, we discovered that the assessment items skewed with an overrepresentation of remember and understand questions. Therefore, another 106 items were added for a total of 940 items. The additional items were included from published sample questions from the Advanced Placement (AP) Biology exam (n = 51) and the biological and biochemical sciences section of the Medical College Admission Test (MCAT) exam (n = 55). These standardized exams are created through a committee process and had been recently redesigned with the intention to include more assessment items with higher-order cognitive processes (Wood, 2009; Schwartzstein et al., 2013).

FIGURE 1. Features of our data set. We analyzed assessment items from a variety of biology courses (A); lower- and upper-division courses (B); and assessment sources such as exam, quiz, review, and homework problems (C). The data set also included 51 AP Biology and 55 MCAT questions, which accounted for about 11% of the total sample (n = 940). The AP Biology and MCAT questions were combined as one category and included in the pie charts for completeness of the data set.
Development of the Coding Scheme
The revised Bloom’s taxonomy intentionally uses language that is generalizable across contexts and encourages more detailed expansion within individual disciplines (Bloom et al., 1956; Anderson et al., 2001). Therefore, a coding scheme more specific to undergraduate biology assessment items is needed. Our articulation of the taxonomy was a culmination of discussions throughout an iterative coding process, identifying ambiguities to offer an elaboration of the revised taxonomy in the context of biology. To familiarize ourselves with the revised taxonomy, researchers independently generated their own assessment items for each of the six cognitive processes in the revised Bloom’s taxonomy and discussed how they would categorize one another’s questions. Subsequently, the team categorized the generated items according to the knowledge dimension. All discussions focused on the specific features of these items and their variations that would determine the appropriate categorizations and designations.
After this initial training, the data set was divided in subsets of about 50 assessment items. For each subset, the researchers analyzed the items independently, identifying a knowledge-type category and a cognitive-process category, along with the corresponding subcategories, for each item. The research team then discussed each item and arrived at consensus for the main categories. Subcategories were not subject to consensus but were used as explanations and points for discussion. In each round of the consensus process, we had extensive discussions on each item to minimize ambiguity in the coding scheme and further delineate features of the various knowledge-type and cognitive-process categories, as well as their subcategories. Our final coding scheme is described in the Coding Scheme section.
Context-dependent information can change the way an assessment item is coded. For example, if an instructor passed out a study guide that listed various accepted analyses for a graph, the cognitive process needed to answer a question about that graph would become remember instead of analyze. In our coding process, only the information given within each assessment item was considered, as we did not have insights into what was discussed in the context of individual courses. The same assumption was made in other studies examining biology assessment items using Bloom’s taxonomy (Crowe et al., 2008; Momsen et al., 2013). Groups of related assessment items, such as associated specific diagrams, figures, or models, were kept together for contextual information. These items were coded individually, as each of them may still use different knowledge types and cognitive processes.
Researchers
Four researchers engaged in the iterative coding process. Two were undergraduates (T.M.L. and A.T.Y.) who completed at least half of the introductory biology course sequences for their respective majors at the beginning of the project. We reasoned that undergraduates are especially suited for this type of project, because they are proximal in expertise to students who would encounter these problems on exams or standardized exams. The third researcher (B.H.E.) was a graduate student in biology education research with an undergraduate degree in biological sciences. These three researchers were directly involved in coding assessment items and data analysis. The fourth researcher (S.M.L.) was a biology faculty member with discipline-based education research expertise who engaged in all research discussions.
Reliability
Out of the 940 assessment items in the data set, 17% (n = 159) were coded by all three primary researchers, 47% (n = 442) by two researchers, and 36% (n = 339) by a single researcher. Fleiss’ kappa, a generalized form of Cohen’s kappa beyond two raters, was used to measure interrater reliability in the subset coded by all three researchers (Fleiss, 1971). Initial interrater reliability for the knowledge-type and cognitive-process categories were κ = 0.68 and κ = 0.70, respectively, both falling within the range of substantial agreement (Landis and Koch, 1977). Disagreements within the items with more than one coder were resolved through discussions, and the final consensus was recorded for subsequent data analysis.
Independence of Dimensions
We performed statistical analyses to test whether the knowledge-type and cognitive-process dimensions of the revised Bloom’s taxonomy are independent of each other. All statistical analyses were performed in JMP Pro (v. 11.0 to v. 16.0). We used contingency analysis to tabulate the two-dimensional categorical data (i.e., the knowledge-type and cognitive-process categories) from observed frequencies in our data set of biology assessment items and the Fisher’s exact test of independence, which is not affected by small numbers, to assess the independence of the two dimensions (Agresti, 1992), as some intersections of knowledge types and cognitive processes have small numbers (Supplemental Table S1). In the Fisher’s exact test of independence, the null hypothesis states that the proportions or distributions of one dimension (i.e., cognitive-process categories) are the same across the different values of the other dimension (i.e., knowledge-type categories; McDonald, 2009). When the null hypothesis is rejected, it means that the knowledge-type and cognitive-process categories are related and not independent.
Subsequently, we used correspondence analysis to determine the relationship among the different categories, because our data set contains categorical data. Correspondence analysis is a multivariate method that decomposes the contingency table statistics into orthogonal factors and displays the set of categorical data in a descriptive graphical form, analogous to principal component analysis for continuous data (Jolliffe and Ringrose, 2006). Multidimensional principle axes are calculated to capture as much variation in the data as possible; ultimately, the correspondence analysis results in Cartesian coordinates that denote relationships among categories, where closer categories are considered more related to one another (Greenacre, 2010). Points on the two major dimensions identified in correspondence analyses were put into groups by cluster analysis with hierarchical clustering (Johnson, 1967).
Verbs as Proxies
We expanded our testing of the assumption that verbs within assessment item prompts are being used as proxies for cognitive processes to include question words in addition action verbs. We use “prompt words” as the inclusive term for both. We tabulated specific words used in assessment item prompts as an additional dimension of data to compare with the cognitive-process and knowledge-type categories coded for each assessment item. Some assessment items did not have discrete prompts, consisting of words such as “be,” “to,” or “as” to infer the question being asked. Other assessment items used formatting to imply the questions that students were expected to answer, such as fill in the blanks. For these reasons, 169 assessment items (18% of n = 940) were excluded from the prompt word data, resulting in a final sample of 771 items for this analysis. Prompt words were independently recorded by two researchers (B.H.E. and T.D.) who reached consensus for all assessment items.
Shannon evenness index (J′) was used to test the hypothesis that prompt words or action verbs can be used as a proxy for cognitive processes. Commonly used to examine biodiversity, J′ measures how evenly different species are distributed within an ecosystem by considering the number of species present and the frequency of individuals within each species (Pielou, 1966). Here, we adapt J′ to examine the distribution of prompt words in relation to cognitive processes in assessment items. Incidentally, Shannon indices were originally developed to determine the entropy or uncertainty of words in a string of text (Shannon, 1949), suggesting that it is reasonable to use J′ to measure the evenness of the distribution of prompt words.
J′ ranges between 0 and 1, with higher values signifying a more even distribution of the use of a specific prompt word across all six cognitive processes, and lower values signifying a stronger association of a prompt word to a given cognitive process. J′ is calculated with the following formulas (Pielou, 1966):
where S is the number of categories or cognitive processes observed, pi the proportion of a specific cognitive process used out of the total frequency of a given prompt word, and i the index for the different cognitive processes.
Coding Scheme
In this section, we describe the details of our final coding scheme. Our coding scheme is not meant to be definitive or universal, as course contexts and prior experiences can affect how instructors and students interpret specific learning objectives. Rather, the following description and explanation reflect our interpretation of the revised Bloom’s taxonomy. When appropriate, we also clarify distinctions between different categories in the knowledge-type and cognitive-process dimensions with additional frameworks or examples from the existing literature.
Types of Knowledge
Factual and conceptual knowledge can be distinguished based on the context of the question (Anderson et al., 2001). We found in our process of delineating these distinctions within our data set that facts consist of a discrete set of details, elements, or specific terminology, whereas concepts draw upon relationships among different facts. Our decision was further informed by the literature on expert versus novice knowledge. Whereas novices tend to see information as isolated facts, experts notice meaningful patterns that connect information (NRC, 2000). The assessment item in Figure 2A asks students about the definition of the different levels of protein structures. To answer the assessment item in Figure 2B, students need to have knowledge not only about the characteristics of kcat/KM but also how it can be used and why it is significant. The multiple-choice option of kcat/KM “reflect[ing] the property of the enzyme when substrate concentration is at saturation” suggests that students need to know what happens to enzymes when they are saturated with substrates and how this phenomenon relates to the definition of kcat/KM.
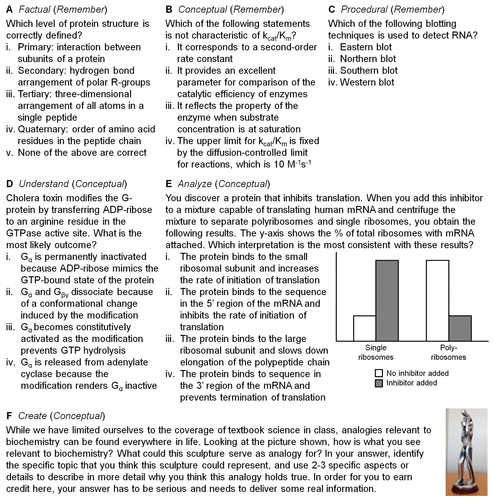
FIGURE 2. Example assessment items. We selected examples to highlight differences among knowledge types: factual (A), conceptual (B), and procedural (C); and cognitive processes: understand (D), analyze (E), and create (F). The dimension not highlighted is also included in parentheses for reference.
Procedural knowledge consists of information on how and when to use specific skills, algorithms, techniques, or methods; this type of knowledge can be drawn both in theory or practice and can be divided into three subcategories (Anderson et al., 2001). In biology, knowledge of skills and algorithms can include knowing how to read a graph or calculate results using equations. Knowledge of techniques and methods can range from proper pipetting techniques to the scientific method. In additional, procedural knowledge includes criteria for determining when to use appropriate procedures, for example, knowing when a Western blot is a more appropriate technique than a Northern blot to test a hypothesis. The assessment item in Figure 2C asks students to know which blotting technique is used to detect RNA.
Metacognitive knowledge refers to awareness about oneself and one’s cognition in general, which include strategic knowledge, conditional knowledge, and self-knowledge (Anderson et al., 2001). Students draw upon metacognitive knowledge when thinking about effective test-taking strategies, being aware of theoretical assumptions and experimental limitations, or knowing one’s strengths and weaknesses. Recent studies have placed an emphasis in the role of metacognition in learning and teaching (Bransford et al., 1999; Dauer et al., 2013; NASEM, 2018). However, we did not identify any assessment items in our data set using metacognitive knowledge. In a study on model-based reasoning, students were first tasked to create a diagram and write a paragraph to explain a phenomenon; subsequently, students were also asked whether they created the diagram or wrote the paragraph first and why (Dauer et al., 2013). The latter prompt represents a question on metacognitive knowledge.
Cognitive Processes
Remember occurs when relevant information is presented with little to no abstraction and is retrieved from memory consistent with how it was originally presented; two subcategories include: recognize and recall (Anderson et al., 2001). To recognize is to identify previously seen information. Recalling involves remembering information with no options to select from. While both subcategories require retrieving information from memory, recalling may be more cognitively demanding (Anderson et al., 2001).
Understand and analyze have many similarities (Bloom et al., 1956; Anderson et al., 2001). Even the expanded subcategories in the revised Bloom’s taxonomy are seemingly synonymous between the two cognitive processes. For example, classifying, comparing, and explaining as subcategories of understand parallel organizing, differentiating, and attributing as subcategories of analyze. Such close equivalences remained a constant point of ambiguity and dispute (Elmas et al., 2020). To articulate a clear distinction between the two cognitive processes, we draw inspirations from Biggs’s SOLO. Like the original Bloom’s taxonomy, Biggs’s SOLO is a hierarchical framework: prestructural, unistructural, multistructural, rational, and extended abstract (Figure 3), and these different levels can be applied to classify students’ outcomes based on the complexity of their work (Biggs and Collis, 1982). In Biggs’s SOLO, students demonstrate the unistructural learning outcome when they are able make a single generalization between two ideas (Biggs and Collis, 1982). This best corresponded to our articulation of understand, a cognitive process that emphasizes the construction of a single relationship. When an assessment item asks students not only to draw multiple connections (multistructure) but also to piece together these relationships in the context of a larger whole (relational), students are asked to analyze. The analyze cognitive process, therefore, requires the construction of a more complex mental structure, as well as connections on how its constituent parts function together (Dewey, 1933; Zagzebski, 2001). We acknowledge that Biggs’s SOLO is intended for students’ demonstrated work, whereas Bloom’s taxonomy is designed to characterize learning objectives. We do not claim that these two taxonomies are used to measure the same constructs; rather, we borrow from the conceptual organization in Biggs’s SOLO as a framework to inform our thinking of how to distinguish the different cognitive processes in the revised Bloom’s taxonomy.

FIGURE 3. Biggs’s SOLO as a framework for cognitive processes. Biggs’s SOLO describes five levels of complexity in terms of work completed by students. In the prestructural stage, student work tends to be so incomplete that they miss the purpose of the question. There is no Bloom’s taxonomy equivalent, as learning objectives should not call for students to miss the point of the question. Unistructural work draws a single connection between two ideas, which parallels the understand cognitive process, such as drawing a conclusion based on a direct cause-and-effect connection. Multistructural work demonstrates multiple unistructural connections but does not articulate the relationship among these connections. In our data set, we did not see a Bloom’s taxonomy equivalent, as assessment items calling for multistructural outcomes were likely coded as separate understand questions. The relational stage, which parallels the analyze cognitive process, not only demonstrates multiple connections but also how these connections are related to one another as part of a larger whole. The extended-abstract stage, which parallels the create cognitive process, goes beyond the relational stage by connecting the coherent structure to relevant outside information, often bringing in different perspectives and generating novel insights. Figure adapted from Biggs and Collis (1982).
The assessment item in Figure 2D prompts students to explain how the structure of a G-protein affects its function. Students use the understand cognitive process to determine a single cause-and-effect relationship: Once the active-site residue is modified, the protein function is altered. In contrast, the assessment item in Figure 2E asks students to analyze by piecing together multiple connections and the relationships among these connections. To determine the effect of the inhibitor on translation requires answering and connecting the following questions. How are single and polyribosomes related to the process of translation? What do they signify in the experimental results? What are the important differences observed when the inhibitor is present or absent? What roles do the small and large subunits, as well as the mRNA, play in the initiation and/or elongation steps of translation? Although both understand and analyze require drawing relationships, understand only requires the establishment of a single relationship, and analyze emphasizes how multiple connections work in concert to serve an overall purpose or structure. We note that the presence of experimental data in Figure 2E does not automatically make it an analyze question. It is conceivable to rewrite the assessment item in Figure 2D to include experimental data while still asking students to understand by determining a single cause-and-effect relationship.
Apply involves using methods or patterns in a given situation, both in theory and in practice, and there are two subcategories: execute and implement (Anderson et al., 2001). In biology, established methods or patterns may include setting up a polymerase chain reaction, using an equation such as Hardy-Weinberg equilibrium, or reading a graph. Executing involves carrying out previously established methods or patterns when encountering a familiar and routine task such as an exercise (Anderson et al., 2001). Implementing entails students working within an existing framework to select a method to solve an unfamiliar problem, and the method is typically more generalized and may have branchpoints of decisions embedded within or multiple position outcomes (Anderson et al., 2001). In principle, the framework in question can be based on any type of knowledge in the revised Bloom’s taxonomy (Anderson et al., 2001).
The revised Bloom’s taxonomy pointed out that the apply cognitive process is likely associated with procedural knowledge, as this type of knowledge encompasses methods and patterns (Anderson et al., 2001). Both knowledge and cognitive process are used in unison to achieve a specific learning objective. Accessing the knowledge of how or when to do something (i.e., procedural knowledge) is different from carrying out a task that uses these established methods or patterns (i.e., apply). It is conceivable to have an assessment item that asks students to remember specific procedural knowledge, for example, to recall different types of microscopy procedures.
Evaluate involves making judgments based on information or evidence, such as the efficiency of a given laboratory method or how consistent a hypothesis is with the supporting evidence. The criteria to determine the certainty or uncertainty of the judgments can be based on a set of metrics specified in the question, in the subcategory of critiquing. Alternatively, the subcategory of checking involves judgments on the internal consistency of the information or evidence, without external metrics being provided. In developing such judgments in the evaluate cognitive process, students are asked to make an argument: There is no definitive right or wrong answer, only more or less supported arguments (Anderson et al., 2001).
Create involves organizing multiple components to form a novel, coherent, and functional whole (Anderson et al., 2001). While this echoes some of the ideas in analyze, such as taking different elements of a structure into consideration, what sets create apart is the requirement that something novel is generated. For example, the assessment item in Figure 2F asks students to generate analogies between biochemistry concepts and features in a sculpture. This example falls under the extended abstract category of Biggs’s SOLO (Figure 3), where students are expected to connect multiple relational structures across different frameworks or domains of knowledge. Other forms of create use cognitive processes that are typically aligned with activities within scientific investigations. The subcategory of generating involves prompting multiple propositions to explain a given phenomenon, thereby creating new possibilities of knowledge. The subcategories of planning and producing often go hand in hand, such as designing a series of adaptive experiments to investigate an unknown phenomenon or to arrive at a solution. Like evaluate, the create cognitive process requires the communication of novel ideas.
RESULTS
The Two Dimensions Are Related and Not Independent
Regarding the knowledge-type dimension, a majority of the assessment items called for factual or conceptual knowledge (38% and 49%, respectively), with the remaining assessment items calling for procedural knowledge (13%; Figure 4A and Table 3). With respect to the cognitive-process dimension, remember and understand (44% and 37%, respectively) were much more common than other four cognitive processes (Figure 4A and Table 3). Combining both dimensions, factual knowledge showed the least variation when associated to the cognitive processes; nearly three-quarters of these assessment items asked students to remember (Figure 4A and Table 3). Conceptual knowledge was used in combination with the most varied distribution of cognitive processes, accounting for the majority of assessment items calling for the analyze, evaluate, and create cognitive processes, whereas procedural knowledge accounted for the majority of assessment items calling for the apply cognitive processes (Figure 4A and Table 3).

FIGURE 4. Correlation between the knowledge-type and cognitive-process dimensions. (A) Stacked bar graph shows the distribution of assessment items in various combinations of knowledge and cognitive processes. Fisher’s exact test reveals that the two dimensions are statistically related (p < 0.0001). (B) Correspondence analysis (scatter plot) and hierarchical clustering (dashed ovals) of data reveal three predominant combinations of knowledge types and cognitive processes. The principle axes c1 and c2 accounted for 61.5% and 38.5% of variance in the data, respectively.
A | Remember | Understand | Apply | Analyze | Evaluate | Create | n | J′ |
---|---|---|---|---|---|---|---|---|
Which | 85 | 91 | 2 | 8 | 11 | 0 | 197 | 0.66 |
What | 55 | 62 | 25 | 14 | 7 | 0 | 163 | 0.85 |
Describe | 30 | 20 | 1 | 4 | 1 | 3 | 59 | 0.66 |
How | 9 | 30 | 5 | 2 | 1 | 0 | 47 | 0.66 |
Explain | 6 | 27 | 0 | 1 | 3 | 1 | 38 | 0.58 |
B | Remember | Understand | Apply | Analyze | Evaluate | Create | n | J′ |
Describe | 30 | 20 | 1 | 4 | 1 | 3 | 59 | 0.66 |
Explain | 6 | 27 | 0 | 1 | 3 | 1 | 38 | 0.58 |
Name | 13 | 14 | 0 | 0 | 0 | 0 | 27 | 1.00 |
Draw | 0 | 14 | 2 | 0 | 0 | 2 | 18 | 0.62 |
Identify | 3 | 5 | 0 | 0 | 0 | 1 | 9 | 0.58 |
Fisher’s exact test between the knowledge types and cognitive processes revealed a significant relationship between the two dimensions (p < 0.0001). When the dimensions are considered together, the combination of factual knowledge and remember is the most common (28%), followed by the combination of conceptual knowledge and understand (23%; Figure 4A and Table 3). These observed combinations are more easily visualized through a graphical representation from correspondence analysis followed by hierarchical clustering. The combination of factual knowledge and remember has the strongest association, signified by the near-direct overlap of both points in the correspondence analysis (Figure 4B). Following hierarchical clustering, our results revealed reveal three predominant combinations of knowledge and cognitive processes: factual knowledge with remember; conceptual knowledge with understand, analyze, evaluate, and create; and procedural knowledge with apply (Figure 4B).
Verbs Are Poor Proxies for Cognitive Processes
For each assessment item, prompt words were recorded to investigate whether such words were associated with specific cognitive processes (Table 3). First, we found that more than half of the assessment items in our data did not use an action verb; instead, 57% of the items used question words such as “which” or “what.” Of the five most frequently used prompt words, three were question words (“which,” “what,” and “how”), and only two were action verbs (“describe” and “explain”). Each of these five prompt words spans at least five out of the six cognitive-process categories and have J′ values ranging from 0.58 to 0.85 in our data set (Table 3A). We further examined the five most frequently used action verbs (“describe,” “explain,” “name,” “draw,” and “identify”), which have J′ values ranging from 0.58 to 1.00 in our data set (Table 3B). These high J′ values indicate a spread of these prompt words being used across different categories and the lack of association between a given prompt word and a corresponding cognitive process.
DISCUSSION
The findings indicate that, at least in the data set of biology assessment items in our study, the two dimensions of the revised Bloom’s taxonomy are related and not independent. We also found that assessment items in biology tend to coincide in certain combinations of knowledge types and cognitive processes. We reason that there may be two potential explanations for these observed combinations. The combinations could be: 1) the result of underlying features of biology as a discipline or 2) the result of the behavioral habits of people, such as instructors, who create assessment items. We futher explore these two possibilities in the following paragraphs.
The nature of certain knowledge types and the nature of certain cognitive processes may necessitate that some combinations are statistically more likely to occur in assessment items in biology, similar to how one might expect more apply questions in a traditional mathematics course focused on using formulas for calculations. Other combinations, while possible in theory, may not carry practical relevance for the discipline. For example, it may be irrelevant to ever consider making an assessment item that involves creating factual knowledge in biology. Also, while statistically unlikely, some combinations such as conceptual knowledge and apply were still observed in our data set. Assessment items in these low-frequency combinations may be of particular interest for further examination (Supplemental Figure S1).
It could be that questions involving certain knowledge types and certain cognitive processes are easier or more desirable for instructors to write, making them more likely to occur in assessments. For instance, assessment items that ask students to recall or understand a concept may be easier to write than something that would require a student to create something or evaluate it. Another factor that we were not able to consider in our data was how the format of the question could affect what combinations of knowledge type and cognitive process are more likely. Both the original and revised Bloom’s taxonomy imply that at least cognitive processes may be limited by question format (e.g., multiple choice, short answer, drawing); however, no study has empirically examined this assumption directly (Anderson et al., 2001; Crowe et al., 2008).
Finally, our data demonstrate that prompt words within assessment items are generally not predictive of cognitive processes. For example, while prompt words such as “describe” and “identify” are often considered in relation to the remember cognitive process, they can also be associated with the create cognitive process in an appropriate context (Figure 2F). In this case, students are asked to identify concepts that they have learned in the course and describe how physical features of the given sculpture are analogies for the course concepts. We conclude that prompt words do not reliably predict cognitive processes; thus, instructors creating assessment items and researchers categorizing them should not simply connect the prompt words in an assessment item with associated verbs and cognitive processes of the revised Bloom’s taxonomy. As such, rubrics for researchers and instructors on how to use the revised Bloom’s taxonomy should not include a column for associated verbs, despite the form that the cognitive processes of the revised taxonomy takes. Instead, the whole context of the assessment item should be considered.
Limitations
As with earlier studies using Bloom’s taxonomy (Crowe et al., 2008; Momsen et al., 2013), we made a similar assumption not to consider course contexts when we coded the assessment items. This means that certain items, if, for instance, they were mentioned word for word in class, may be asking students to use a different set of knowledge types and cognitive processes depending on what was previously presented to students in the course.
Due to the low frequency of certain combinations of knowledge types and cognitive processes in our data set, it is possible that the particular clustering we identified may not be fully generalizable. As such, we do not attempt to make universal claims that are true of all assessment items in biology. However, it is worthwhile to note that across our many instructors and courses, certain combinations remained rare for potential reasons described earlier in the Discussion.
While conducting our study, we also revealed another explanation for the dependence of the two dimensions of the taxonomy that has to do with the procedure of coding. In our methods, we followed convention and coded each assessment item with one knowledge type and one cognitive process corresponding to the presumed learning objective embedded in the assessment item (Bloom et al., 1956; Anderson et al., 2001; Bissell and Lemons, 2006; Lemons and Lemons, 2013; Hanna, 2007; Crowe et al., 2008; Starr et al., 2008; Halawi et al., 2009; Momsen et al., 2010, 2013; Karaali, 2011; Coleman, 2013; Jensen et al., 2014; Arneson and Offerdahl, 2018). However, an assessment item could potentially have multiple embedded or implicit learning objectives. For example, when asking students to analyze experimental data, we may assume that students are already familiar with the technical vocabulary in the assessment item without explicitly labeling such factual knowledge as part of the learning objective. Therefore, assigning a single knowledge type and a single cognitive process may not fully capture an instructor’s or student’s thought process when encountering an assessment item. Instead, we propose that future studies could consider an expanded coding process wherein each assessment item is analyzed for all the embedded types of knowledge and cognitive processes.
Implications
While our data do not suggest that certain combinations of knowledge types and cognitive processes are more or less desirable as learning objectives in undergraduate biology education, we recommend that researchers and instructors who use the revised Bloom’s taxonomy should do the following: First, given that there were exceptions to the clustering of certain knowledge types and cognitive processes in the form of rare combinations (Supplemental Figure S1), coding both dimensions would ensure an item is not misclassified. Furthermore, we suggest that instructors who create assessment items should articulate both dimensions for students, as they are both engaging with a type of content (knowledge type) and performing an action (cognitive process). This suggestion is aligned with policy documents (Table 2). Existing Bloom’s taxonomy tools in biology (Crowe et al., 2008; Arneson and Offerdahl, 2018) and the 3D-LAP (Laverty et al., 2016) have made important contributions to helping instructors be intentional about the composition of their assessments. Whereas the 3D-LAP aligns well with the NGSS with two dimensions dealing with content and actions, we believe that Bloom’s taxonomy tools in biology could be more aligned to policy documents with the inclusion of a content-focused knowledge-type dimension.
Our findings are best understood in light of studies that have shown how the intended and enacted object of learning, as well as what students learn, can be misaligned (Bussey et al., 2013; Dietiker et al., 2018). In this case, learning objectives (intended object of learning) may be misaligned with assessment items (enacted object of learning). Additionally, if an instructor were to assume that a particular verb would indicate a particular cognitive process when it in fact does not, that could result in further misalignment of the intended and enacted objects of learning with what students learn. Prompt words in general should not be used to assume information about the embedded learning objective within an assessment item. Instead, the context of the question and how researchers, instructors, and students approach assessment items should be considered.
Ultimately, a more transparent scaffoldiing system is needed to support instructors in articulating what they are asking for as students think about how to approach a problem. An expanded coding process like the one we describe may provide such a scaffold. To code all the embedded knowledge types and cognitive processes within an assessment item, both instructors and students would need to articulate the various steps they take within a problem. Then, instructors and students could compare their steps to reveal any potential misalignments.
ACKNOWLEDGMENTS
We are grateful to the faculty participants who contributed assessment items for this analysis. We thank L. Smith and E. Tour for feedback on earlier versions of the article. T.M.L. and A.T.Y. were supported in part by the Undergraduate Research Assistant Program at the Office of Undergraduate Research, Northwestern University. S.M.L. was supported in part by the Faculty Career Development Program at University of California San Diego. This project was initiated with support by an institutional award from the Howard Hughes Medical Institute for undergraduate biology education under award no. 52006934 at Northwestern University. This material is based upon work supported by the National Science Foundation under grant no. DUE-1821724.