Relationships between Prediction Accuracy, Metacognitive Reflection, and Performance in Introductory Genetics Students
Abstract
Cognitive scientists have previously shown that students’ perceptions of their learning and performance on assessments often do not match reality. This process of self-assessing performance is a component of metacognition, which also includes the practice of thinking about one’s knowledge and identifying and implementing strategies to improve understanding. We used a mixed-methods approach to investigate the relationship between students’ perceptions of their performance through grade predictions, their metacognitive reflections after receiving their grades, and their actual performance during a semester-long introductory genetics course. We found that, as a group, students do not display better predictive accuracy nor more metacognitive reflections over the semester. However, those who shift from overpredicting to matching or underpredicting also show improved performance. Higher performers are overall more likely to answer reflection questions than lower-performing peers. Although high-performing students are usually more metacognitive in their reflections, an increase in a student’s frequency of metacognitive responses over time does not necessarily predict a grade increase. We illustrate several example trends in student reflections and suggest possible next steps for helping students implement better metacognitive regulation.
INTRODUCTION
The Construct of Metacognition
Since Flavell (1979) originally proposed the concept of metacognition, many researchers have contributed to elaborating its components. Kitchener (1983) included metacognition as one of the three progressive levels of cognition: cognition (understanding), metacognition (knowledge of and reflection on cognition), and epistemic cognition (the nature of how we know). Nelson and Narens (1990) focused on metacognitive reflection, outlining two components: monitoring (assessing learning and performance) and control (regulating cognitive activity by planning and carrying out changes). Schraw and colleagues (Schraw and Dennison, 1994; Schraw and Moshman, 1995) further defined reflection as metacognitive regulation, which they described as planning which strategies to use, monitoring progress toward achieving a goal, and evaluating success at achieving that goal. They also framed the component of metacognitive knowledge, defining the three subcomponents as declarative (knowledge task demands and learning strategies), procedural (how to use learning strategies), and conditional (when and why to use specific strategies). In this paper, we use the Schraw and Moshman (1995) framework, as it best characterizes the two components of potential metacognitive practices among students as they struggle to align their perception of their knowledge with their actual knowledge. This framework has also frequently been used by other biology education researchers, allowing for comparisons among different research studies (reviewed in Stanton et al., 2021).
Although students infrequently engage naturally in metacognitive practices during their learning process (e.g., Stanton et al., 2015), some situations stimulate activation of metacognition. One such situation is engaging in solving a complex problem, because generating a solution requires reflection on prior knowledge and integrating that knowledge into a new problem space. When students are aware that they do not understand a concept, they are exhibiting metacognitive knowledge, which helps them prepare to learn a new skill (Schraw and Nietfeld, 1998; Ifenthaler, 2012). Subsequently engaging students in different metacognitive regulatory practices can also lead them to choose better learning strategies and can improve performance (Veenman et al., 2006; Mevarech and Amrany, 2008; Pintrich, 2010; Bjork et al., 2013; Panadero, 2017). Some of these practices include post-exam analysis exercises (Williams et al., 2011; Mynlieff et al., 2014; Stanton et al., 2015), cues to check their answers in multiple circumstances (on practice problems, homework, and exams; McDonnell and Mullally, 2016), and using enhanced answer keys and reflections (Sabel et al., 2017). Similarly, using the steps of planning an approach and then checking to see whether the approach worked is also correlated with problem-solving success and improved performance (Kalyuga, 2010; Avena et al., 2021). Often, metacognitive awareness (when students realize that they are uncertain of their knowledge) can stimulate metacognitive regulation, which can manifest when students perform poorly on an exam and/or when they are studying for an exam (e.g., Dye and Stanton, 2017).
Grade Prediction as a Form of Metacognitive Monitoring
In general, humans are not adept at employing best strategies for learning. Students often fail to employ effective methods such as spacing out their studying rather than cramming (Cepeda et al., 2006) and self-testing rather than restudying (Roediger and Karpicke, 2006; Karpicke and Roediger, 2008). When people perceive a practice as effortful, they predict they will not learn well and report they will be unlikely to use the practice again (Kirk-Johnson et al., 2019). Perception of effort also leads people to avoid beneficial strategies, even when they have identified such strategies as better, largely because they are not comfortable with them and perceive them as more difficult to execute (Dye and Stanton, 2017). In addition, students often make errors in judgment both about their success (i.e., they overpredict their grades on exams) and their knowledge (they think they know more than they do: Kruger and Dunning, 1999; Kornell and Bjork, 2009). Recent studies in psychology and biology have shown that most students overpredict their exam scores, particularly on the first exam. Overpredictors, who typically have lower exam scores, can shift over time to improve their prediction accuracy (Dang et al., 2018; Osterhage et al., 2019), but ultimately, these shifts are limited and do not always extend to higher performers (Hacker et al., 2008; Miller and Geraci, 2011). These and other studies suggest that, although student perceptions are often mismatched with reality, helping students to become better aware of the limits of their knowledge may be a critical step toward improving student learning. Theoretically, if students have more metacognitive awareness, this may lead to more targeted and successful studying, because they may be able to recognize when they do not fully understand a concept. This, in turn, could lead to better performance.
Both defining and measuring metacognition is challenging, given that it is a latent construct (Veenman et al., 2006). As different levels of cognition are overlapping, it can be difficult to differentiate between a person’s cognition (knowledge) and metacognition (knowledge of knowledge). In addition, a person may have an internal metacognitive narrative that helps regulate learning, but not be able to describe that narrative to others (Azevedo, 2020). Although prior researchers have generated survey instruments to capture students’ baseline metacognitive awareness and/or regulation, including the Metacognitive Awareness Inventory (MAI; Schraw and Dennison, 1994) and the Motivated Strategies for Learning Questionnaire (Pintrich et al., 1993), such instruments do not always correlate well with students’ in-line use of metacognition and self-regulation or with small changes in students’ habits during a course (Veenman, 2011; Tock and Moxley, 2017; Harrison and Vallin, 2018). In this study, we aimed to connect students’ awareness of their knowledge with subsequent regulation; thus, we chose a measure of metacognitive knowledge (accuracy of quiz grade prediction) and a measure of metacognitive regulation (reflections after each quiz), along with quiz performance. Our specific research questions were:
How do grade predictions relate to performance and subsequent changes in prediction and performance?
What themes arise in students’ reflections about their performance?
Do metacognitive reflection scores correlate with performance or improvement in performance across a semester?
METHODS
This study used a mixed-methods approach, combining both qualitative analysis and quantitative research methods to understand a phenomenon more deeply (Johnson et al., 2007). Our goal was to make student metacognitive regulation practices visible by capturing the quantitative measure of post-quiz grade prediction accuracy along with student reflections after seeing the results of their quiz performance. The student written reflections serve as a rich and detailed data set that can be interpreted using the qualitative process of assigning themes or codes to student writing (Hammer and Berland, 2014). We share the qualitative results along with quantitative numerical representations to demonstrate patterns and trends captured in the data. This allows for robust comparisons as well as statistical analyses.
Data Collection
Participants were enrolled in an introductory-level Principles of Genetics course at the University of Colorado Boulder in two consecutive spring semesters (2020 and 2021). This course is the second in a two-course introductory series, with the first being Introduction to Cell and Molecular Biology. One of the authors (J.K.K.) taught this course along with another faculty member in both semesters, using identical materials and the same active approach, which included daily clicker questions and group work. The two iterations used only slight variations on assessment questions. The most notable difference between the courses was that the first half of the course was in-person and the second half was remote in 2020, while the entire course was remote in 2021. Course enrollment was 347 students in 2020 and 340 in 2021, with 234 and 262 students, respectively, who completed the course and consented to participate in the research. Demographics were self-reported during the first week in the class survey (which also included the Genetics Concept Assessment [GCA]). Demographics across the 2 years were nearly identical, as shown in Table 1.
Year | First- and second-year students | Female | Latinx | White:Asian:Black:multi-ethnic:no response |
---|---|---|---|---|
2020 | 79 | 71 | 13 | 75:15:2:5:3 |
2021 | 68 | 62 | 12 | 74:13:1:6:6 |
Students were invited to complete the multiple-choice GCA (Smith et al., 2008) for extra credit during the first week of class to assess baseline conceptual knowledge; this incoming score also helped determine whether students from different sections or years could be pooled together for analysis. The same GCA questions were also on the final exam, comprising 40% of the exam points. Students took a quiz every 2 weeks, for a total of six quizzes. Quizzes consisted of 10–12 multiple-choice questions and three to four short answer questions; nearly all questions involved application or analysis, such as interpreting scenarios, figures, or graphs and making predictions or drawing conclusions. The lowest quiz grade was automatically dropped, and the total quiz score comprised 50% of the course points. The final course grade included daily preclass assignments, weekly problem sets, in-class individual and group participation, and the final exam. In 2020, the first three quizzes were in person, while the last three were online. In 2021, all six quizzes were administered online. Some quizzes in each semester had a second, group component, but all data collected and reported on here involve only individual performance. Due to a technical error, no data were collected for quiz 2 in 2021, and thus only data from five of the six quizzes are reported for both semesters.
The last question of each quiz asked students to predict their individual performance by selecting a letter grade of “A”–“F” (Table 2). Grade prediction accuracy was calculated as follows: each grade prediction (“F” to “A”) was converted into a whole number from 0 (“F”) to 4 (“A”). Actual quiz scores were also converted to the same numerical rank, and the accuracy of each prediction was calculated by subtracting the predicted quiz score from the actual quiz score. The numerical difference between the two represents the magnitude of inaccuracy, while the sign indicates overprediction (–), underprediction (+), or matching (0).
Prediction question | Reflection questions | |
---|---|---|
At end of each quiz | What grade do you think you got on this quiz?(A, B, C, D, F) | |
Upon receiving graded quiz | How did you perform on this quiz compared with your prediction? (better, same, worse)Why do you think you performed as you did?Explain why you are/are not satisfied with your performance.How do you know the strategies you are using are working well for your learning? |
After receiving their graded quiz, students were invited to access a Qualtrics survey to complete a short post-quiz reflection containing three questions asking students to evaluate their performance and strategies for a total of 1 extra-credit point. Students were first asked to choose whether they performed better, the same, or worse than their initial prediction, and were then asked to respond to a set of open-ended questions (Table 2). There were no other explicitly metacognitive exercises in the course aside from these optional reflections.
Qualitative Analysis
We initially used open coding and thematic analysis (Saldana, 2016) to explore the data set of student reflections. From student answers, authors J.K.K. and M.E.P. and a series of undergraduate assistants identified that students had many types of strategies, many ways in which they intended to exert control over their performance, and many ways of exploring their knowledge. They also varied in the depths of their explanations (e.g., some students responded in general terms, others with specific ideas). After many rounds of trial and error, we again considered our ultimate goal: to determine whether or not students responded metacognitively to the prompts rather than characterizing the many different approaches they described. Thus, we returned to the Schraw and Moshman (1995) framework to determine whether the main categories of knowledge and regulation could capture the nature of the student responses. Authors J.K.K. and M.E.P., along with two undergraduate assistants read and iteratively coded ∼200 answers together to decide on the five themes shown in Table 3, which include the metacognitive categories of Knowledge and Regulation, along with three additional non-metacognitive categories of Generic, Performance, and Blame. The categories are not mutually exclusive: A student answer was often characterized by multiple codes. Two authors (J.K.K. and M.E.P.) subsequently coded a total of 150 reflections together, adjudicating differences to finalize the coding scheme. To measure interrater agreement, J.K.K., M.E.P. and a graduate assistant coded an additional set of 86 answers. In this set of codes, there were 129 instances in which at least one rater identified a code in a reflection. For each of these instances, we identified the number of raters who agreed. We then calculated a final interrater agreement of 75% by dividing the total number of times all raters agreed by 129. The remaining answers (∼500) were divided up and coded individually by each of the three raters, with 10% overlap to ensure continued accuracy.
Code | Description | Examples |
---|---|---|
Regulation | Expresses an internal measure of why or how a particular grade was achieved, including accepting responsibility and planning future possible strategies | “I feel I definitely could have studied more for this quiz. It’s my fault I got the F.”“[I was successful because] I took my time working through each problem.”“Next time, I will be sure to do the practice test and review the problem set questions.” |
Knowledge | Expresses an internal awareness of understanding, specific knowledge, or skill. | “If I can go through the material and know what I’m looking for and how to find it.”“I need to study more in-depth and focus on understanding and applying concepts.”“I feel like I know the information.” |
Blame | Describes an external factor as a reason for performance | “Some of the wording was confusing.”“The test questions are nothing like the practice questions.” |
Performance | Uses grade as a metric of success. | “A ’C’ is bad.”“Wasn’t an A.” |
Generic | Non-reflective response, stating a fact without an accompanying strategy or qualifier | “I studied.”“I didn’t study enough.” |
Statistical Analysis
After qualitatively coding all responses, we generated a quantitative metacognitive reflection score (MRS) by using the codes already ascribed to each answer. Each of the three answers per quiz was given a binary score of 0 or 1: If a student’s answer had been coded as containing any metacognitive practices, it was given a 1, otherwise, a 0 (see Results for details). Thus, for any quiz reflection, a student’s MRS could range from 0 to 3. This score was then used for further analyses.
We used linear regressions for continuous data and Spearman correlations for categorical data (e.g., prediction accuracy) to determine relationships between measures (prediction accuracies, grades, MRS). Sex, class standing (first and second year vs. other years), GCA pre score, and race/ethnicity did not contribute significantly to any regression models and thus are not reported on here. Where pairwise comparisons were more suitable, we used a one-way analysis of variance (ANOVA) with Tukey post hoc comparison, t test, or chi-square tests to draw conclusions about differences between groups and differences in distributions.
Human Subjects Approval
The use of human subjects was approved by the University of Colorado Boulder Institutional Review Board (protocol 17-0726).
RESULTS
Student Performance Is Similar across Years
Despite variation in format (in person and remote vs. remote only) across the two semesters of data collection, students performed similarly on assessments (Table 4). Consenting students’ performance was slightly but not significantly higher than overall student performance. Only a subset of the consenting students took the GCA pretest each year (175 in 2020 and 189 in 2021); all students took the GCA posttest as part of the final exam. Note that because the GCA pretest was taken as a survey for which student consent was required, we cannot calculate the GCA scores for non-consenters (quiz, exam, and course grades can be reported in aggregate without consent). Because of the overwhelming similarities in data from 2020 and 2021, we present most analyses without distinguishing between year, unless otherwise indicated.
Year | Number of students | Quiz % | Final exam % | Course % | GCA pre % | GCA post % |
---|---|---|---|---|---|---|
2020 | 239 | 78.4 (18) | 75.7 (14) | 85.8 (9) | 36.9 (14) | 81.8 (14) |
2021 | 262 | 79.0 (19) | 75.7 (15) | 86.1 (10) | 36.9 (16) | 80.3 (16) |
Both years | 688 (all enrolled students) | 78.8 (19) | 74.4 (16) | 84.0 (12) | N/A | N/A |
Direction and Magnitude of Prediction Inaccuracy Is Correlated with Performance
Our first research question was: How do grade predictions relate to performance and subsequent changes in prediction and performance? We first looked at the proportion of students who predicted accurately and inaccurately across quizzes and years. A student who achieves an “A” (4) cannot overpredict, while a student who achieves an “F” (0) but predicted an “A” overpredicts by 4 grade points. On each quiz, more students overpredicted their grades than matched or underpredicted: Over all quizzes and years, 40% of students overpredicted, while 25% underpredicted and 35% matched (Supplemental Figure 1). There were minor differences by individual quiz, related to average performance. Quiz 1 2020 and quiz 4 2021 each had the lowest average score (75%) for their years. For each of these quizzes, there were significantly more overpredictors than for the matched quiz from the other year (chi-square, quiz 1: χ2 = 34.3; quiz 4: χ2 = 49.4, both p < 0.001). All other quizzes within each year had nonsignificant differences in the proportion of overpredictors (chi-square, quiz 3: χ2 = 1.15; quiz 5: χ2 = 1.27; quiz 6: χ2 = 1.65, all p > 0.05). The mean quiz grade across all quizzes and years was 78.8 (±19 SD), while the mean prediction accuracy was -0.37 (an overprediction of a little more than one-third of a letter grade; ±1.25 SD).
When looking at individual quiz prediction and grade, we found that the magnitude and direction of prediction inaccuracy was strongly correlated with quiz grade. In other words, students who performed most poorly on a quiz generally overpredicted their scores on that quiz by the largest amount, while those who accurately predicted their quiz grades (matched) fell in the middle of the grade range, and those who underpredicted the most scored the highest (Figure 1). Student average quiz prediction inaccuracy was also significantly correlated (by linear regression, n = 497) with average quiz grade (F = 448.75, r2 = 0.48), final exam grade (F = 113.95, r2 = 0.21), and final course grade (F = 336.24, r2 = 0.40), all p < 0.001 (Supplemental Figure 2). Thus, overall, overpredictors performed most poorly, matchers performed in the middle, and underpredictors performed the best.

FIGURE 1. The magnitude and value (positive vs. negative) of prediction accuracy (x-axis) is correlated with quiz grade (y-axis). Each point represents a single prediction accuracy and quiz grade. Color indicates quiz number; n = 2432 from 497 students. Spearman’s rank correlation, r = 0.73, n = 2432, p < 0.001.
Overprediction Is Associated with Subsequent Improvement
To determine whether a mismatch in grade prediction and actual grade could influence the next quiz grade, we compared the prediction accuracy on a given quiz (quiz n) to the change in score between that quiz score and the student’s subsequent quiz score (quiz n + 1 score − quiz n score). In Figure 2, those who did not change their grades from one quiz to the next fall along the “0” line; those who decreased their quiz grades fall below the line, and those who increased their grades fall above the line. There is a significant, moderate correlation (Spearman’s rank correlation, r = −0.38, n = 2432, p < 0.001) between the magnitude of the predictive inaccuracy and the change in quiz score. In other words, those who overpredicted a quiz grade tended to show improvement on the next quiz, those who matched tended to show little change, and those who underpredicted tended to decrease their performance on the next quiz.

FIGURE 2. The accuracy of a quiz grade prediction (x-axis) is correlated with the change in quiz score between that quiz and the subsequent quiz (y-axis). Color indicates quiz number; a student may be represented up to four times on the graph (n = 497 students). Spearman’s rank correlation, r = −0.38, n = 2432, p < 0.001
Students Who Shift from Overpredicting to Matching or Underpredicting also Improve Their Quiz Scores
Students can vary widely in their prediction accuracy from quiz to quiz. Some students overpredict initially, get closer to a matching prediction on the next quiz, but then overpredict or underpredict on a later quiz. Others initially match and then under- or overpredict, then match again. Although a match between prediction and performance is ideal, we consider students who start off overpredicting and end up either matching or underpredicting to have improved their prediction accuracy. To answer the question of whether improvement in accuracy relates to improvement in quiz scores, we selected students who had provided grade predictions for four or more quizzes. We selected this cutoff because the accuracy prediction was a question on each quiz; students who missed more than three quizzes or failed to answer the accuracy prediction question on more than three quizzes were excluded. For both prediction accuracy and quiz grade, we plotted the scores over time and calculated the slope of each line as the representation of change over time (Figure 3A). A linear regression demonstrates that the change in prediction accuracy significantly and positively predicted the change in quiz grade (F = 545.3, r2 = 0.57, p < 0.001). In general, those who shift from overprediction to matching or underprediction over time also increase their quiz grades over time (Figure 3B).

FIGURE 3. The slope of change in quiz grade (left) and change in prediction accuracy (right) was calculated for each student who made four or more predictions (n = 406). (A) Illustration of the slopes for a single student (red dot in B). (B) Each student’s changes are represented as a single dot. The slope of the prediction accuracy predicts the slope of quiz grade (linear regression: F = 545.3, r2 = 0.57, r = 0.76, p < 0.001).
Post-Quiz Reflections Display a Range of Metacognitive Responses
To address research question 2 (What themes arise in students’ reflections about their performance?), we analyzed students’ written post-quiz reflections and established codes to describe their responses. The optional, extra-credit survey questions asked students to reflect on their performance, explain their satisfaction level, and evaluate the strategies they were using. Not all students participated in post-quiz reflections, and the number of participants varied for each quiz. As described in Methods, we arrived at five broad themes to characterize student answers: Metacognitive Knowledge, Metacognitive Regulation, Blame, Performance, and Generic (Table 3). A single answer was frequently coded into multiple themes. Answers in the Metacognitive Knowledge category (referred to as “Knowledge” henceforth) primarily focus on what students know about their thinking, learning, and the demands of the task, thus falling under the category of declarative metacognitive knowledge (Schraw and Dennison, 1994; Schraw and Moshman, 1995; Dye and Stanton, 2017; Stanton et al., 2021). Answers in the Metacognitive Regulation category (referred to as “Regulation” henceforth) primarily focus on how to control thinking for the purpose of learning (Schraw and Dennison, 1994; Schraw and Moshman, 1995; Dye and Stanton, 2017). Responses coded into the Generic category were those without a metacognitive component, such as “I studied” or “I don’t know.” Because these responses offer little information as to what students were thinking, we chose not to explore them further here.
In answering the first two survey questions, which asked students to reflect on their performance (“Why do you think you performed as you did?” and “Explain why you are/are not satisfied with your performance”), students’ responses frequently involved Regulation and/or Knowledge. Answers in the Regulation theme described taking responsibility for performance, describing how or why different study strategies were successful or unsuccessful, the amount of time or timing of studying, and other practices to help monitor progress. In general, these responses reflected an internal awareness of their learning and the capacity to regulate specific actions through personal commitment. Answers in the Knowledge theme generally mentioned depth of knowledge, such as conceptual understanding and/or application versus memorization. These responses showed an internal awareness specific to understanding their own knowledge or lack of knowledge. In the Performance theme, students focused explicitly on their grades or a change in grade on assessments (problem sets and quizzes) or on the disconnect between their expected and actual performance. These responses used an external measure (their grades) to determine satisfaction and knowledge. Finally, responses in the Blame theme placed responsibility, usually for poor performance, on an external factor, such as a situation in the student’s life, the instructor’s wording of a question, or lack of resources.
The third question (“How do you know the strategies you are using are working well for your learning?”) asked students to evaluate their learning strategies. Responses were still described by the same main themes, as students often mentioned depth of knowledge (e.g., “I can answer many different practice questions and explain to others”), ease of using specific strategies, and performance as a measure of learning. Responses to this question also included describing feelings of confidence and comfort, but also frustration, all of which were coded as Regulation, because they frequently were combined with a sense of purpose (e.g., “I need to do more practice questions so that I feel more confident”).
Across responses to the three questions for all quizzes, answers containing Regulation were the most common, followed by Performance, then Knowledge, and finally, Blame. Although there was a trend toward more use of Regulation on later quizzes, there were no significant differences in the distribution of themes across quizzes (Supplemental Figure 3); in other words, students do not shift the way they respond to these questions over time.
However, there were significant differences in the proportion of students who responded to the survey questions (and consented to be studied) based on quiz grade (Figure 4). More higher-performing students responded to the survey than those with lower performance: only 28% of students who achieved a “D” or “F” on a quiz participated in the post-quiz reflection, while 31% of those with “C” and “B” grades participated, and 38% of those with “A” grades participated, χ2 (3, n = 2611) = 144.1, p = 0.00. Lower-performing students were also significantly more likely to place blame on an external factor than higher-performing students, χ2 (3, n = 90) = 8.9, p = 0.03: those with “D”/“F” quiz grades blamed in 15% of reflections, while those with “C” grades blamed in 12%, “B” grades in 8%, and those with “A” grades in only 4% (Figure 4). There were no other significant differences in responses.

FIGURE 4. The distribution of types of responses from students earning different grades across all quizzes. The number of individuals responding in each grade bin is shown at the top of each column and differs significantly across bins. For those earning “D” and “F” grades, 33% responded, for “C” grades, 36%, for “B” grades, 33%, and for “A” grades, 40%, χ2 (3, n = 2611) = 144.1, p = 0.00. Those with “D”/“F” quiz grades were significantly more likely than higher performers to place blame on an external factor, χ2 (3, n = 90) = 8.9, p = 0.03.
Metacognitive Reflection Scores Have Limited Predictive Value for Performance
To address research question 3 (Do metacognitive reflection scores correlate with performance or improvement in performance across a semester?), we developed a simple numeric score to represent the metacognitive nature of student reflections, the MRS. For each question, per quiz, any reflection coded as Regulation and/or Knowledge was scored as a 1, while those coded only as Blame, Performance, and/or Generic were scored as a 0. Thus, a student’s MRS could range from 0 to 3, because there were three questions for each post-quiz reflection. We did not count each incidence of metacognition within a response, because we did not want to privilege answers from individuals who chose to write lengthier responses over those who wrote less but still had clearly identifiable examples of metacognition. Instead, we scored the presence/absence of metacognition in response to each survey question asked.
To visualize the relationship between MRS and performance, we looked at each individual’s MRS for a given quiz compared with that specific quiz score (Figure 5). Nonparticipants (consenting students who did not answer the reflection questions for a given quiz) had significantly lower quiz scores on average than each group of participants who did answer the reflection questions, followed by those with an MRS of 0 (not metacognitive), MRS score of 1, and MRS scores of 2 and 3 (not different from each other, but significantly higher than all other categories; one-way ANOVA; post hoc Tukey tests, F = 23.1, p < 0.001). In other words, students earning a higher score on a quiz gave more metacognitive responses in their post-quiz reflections for that quiz than did lower-performing students. Despite this, MRS values vary widely within individuals over time. Thus, when comparing average MRS scores by individual (n = 470) with performance measures, the relationships, although significant, are likely not meaningful. Linear regressions between average MRS and average quiz score (F = 26.1, r2 = 0.06), final exam grade (F = 20.2, r2 = 0.04), and course grade (F = 32.2, r2 = 0.04), are each significant (p < 0.001) but have very low r2 values. MRS values similarly fail to have a meaningful relationship with prediction accuracy for the same quiz, despite a significant p value (p < 0.001; Spearman’s regression: F = 11.69, Rank r2 = 0.01; unpublished data).
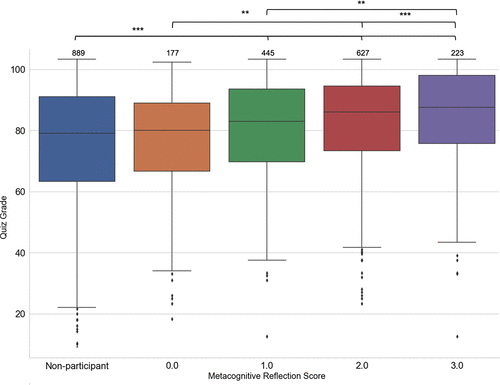
FIGURE 5. Post-quiz reflections are on average more metacognitive from students with higher quiz grades (one-way ANOVA, F = 23.1, p < 0.001). The number of individual reflections is shown in parentheses at the top of each MRS (0–3). Nonparticipants are consenting students who did not answer the reflection questions for a given quiz. The mean quiz grade for each category is indicated. Tukey post hoc comparisons showed that nonparticipants had significantly lower quiz grades than those with an MRS of 1, 2, or 3. Those with an MRS of 0 had significantly lower quiz grades than those with an MRS of 2 and 3, and those with an MRS of 1 had significantly lower quiz grades than those with an MRS of 3. **p < 0.01; ***p < 0.001.
Students also varied widely in whether their MRS changed over the semester. We hypothesized that if students became more metacognitive over the semester (indicated by increased MRS), they may also have improved their performance on quizzes over time. To determine whether an increase in MRS predicted an increase in performance, we visualized the relationship between change in MRS over time and change in quiz performance, using the same approach as used for prediction accuracy (Figure 3). Again, we selected only students who had provided post-quiz reflections for three or more quizzes and used only the data points for which students had both an MRS and a quiz score. We calculated the change in MRS by plotting the data points over time for each student and using the slope of the representative line as a measure of change. A linear regression demonstrated that there is no statistical relationship between change in MRS and change in quiz score (linear regression: F = 1.41, r2 = 0.01, n = 308, p = 0.24). However, we used the visualization of change in MRS and quiz score over time (Figure 6) to describe trends in the behaviors of students who fall into different quadrants, as described in the following sections.

FIGURE 6. Distribution of change over the semester in MRS compared with change in quiz scores. Each axis represents the average amount of change for quizzes (y-axis) and MRS (x-axis). Each point represents a single student who responded to at least three post-quiz reflections (n = 336 out of 501 consenting students). The number of students represented in each quadrant is as follows: increased quiz grade, decreased MRS (upper left): 82; increased quiz grade, increased MRS (upper right): 76; decreased quiz grade, decreased MRS (lower left): 91; decreased quiz grade, increased MRS (lower right): 58. There were 29 students who had either an MRS or quiz slope of 0. The relationship between MRS change and quiz score change is not significant (linear regression: F = 1.41, r2 = 0.01, p = 0.24). Each brightly colored dot represents a student whose responses were further evaluated: Responses were chosen to capture the broad range of trends observed, connecting the variability in performance and metacognition to student behavior.
Students’ Metacognitive Reflections Show Several Trends over Time
Decreasing MRS and Quiz Scores.
Students in the lower left quadrant of Figure 6 were those whose MRS and quiz scores both decreased over time. Some of these students started with metacognitive responses but shifted to describing performance, making generic comments about studying, or expressing burnout and frustration as the semester progressed. For example, one student’s reflection early on was “I go back to old questions to see if I understand them and the methods of understanding them,” while toward the end of the semester, the student stated, “I am not doing as well with online science classes,” and “I’m in a bit of a slump” (bright yellow dot, Figure 6).
Another student (pale yellow dot) started off ready to put in more effort after receiving a “B” on the first quiz: “I could have studied much more … I’m ready to work harder”; but later said: “I had a really busy week with other exams and essays,” and “I guess I studied the wrong material.” This student had a steady decrease in performance over time.
Increasing MRS and Quiz Scores.
On the opposite end are students who fall in the upper right quadrant, increasing both MRS and quiz scores. One student (light purple dot) reported studying by cramming last minute at the beginning of the semester and stated, “I would like to do a little more studying,” a typical Generic response. Toward the end of the semester, this student’s reflections focused on a deeper learning strategy: “I want to explain the information to someone else to make sure I understand.”
Another student (dark purple dot) initially focused on performance: “[I need to]… take better notes,” and “I judge my studying strategies based off of my performance on the quizzes.” Later, this student gave Knowledge responses such as “I have learned that I am a very visual learner, so diagrams to explain a scenario are very helpful for me to make strong learning connections to the content.”
No Change in MRS.
There are students along the y-axis (no change in MRS) who both increase and decrease their quiz scores. Those whose quiz scores increased dramatically (e.g., green dot, who went from an “F” on an early quiz to an “A” on the last) had highly metacognitive reflections across all their responses. Early in the semester, one student said: “I can answer the questions on the practice quiz and understand why the answer is correct”; in the middle: “I review using application problems or redoing the activities”; and at the end: “I learn better when I get to apply my learning like in the activities … I was unsure about a few questions but went with my gut and they were right.”
Little Change in Either MRS or Quiz Score.
Some students fluctuated only a small amount over time in terms of both MRS (within ±0.5 of no change) and quiz score (just above or below the x-axis). For example, one student (orange dot) performed well on all quizzes and had generally high MRS scores: “I … went back through the quiz to understand what I got wrong and why,” and “I feel prepared when I can take the practice quiz with little confusion and no notes.” At the end of the course, this student said: “I benefit [from] going back through and making notes on why an answer was the correct one and why others weren’t.”
A different student (dark blue dot) had consistently low MRS scores, with a slight increase toward the end of the course, and low quiz grades. This student focused almost solely on performance, blaming external factors, and/or making generic statements about the learning process at the beginning: “I studied but not intensely,” and “I’d like to do better but idk [I don’t know] how”; and later in the semester: “I haven’t been studying the way I usually do … it’s been very stressful lately and I haven’t had the time to really bear down and study."
Opposite Trends in MRS versus Quiz Scores.
Finally, there are students whose reflections seem out of line with their increasing or decreasing quiz scores: Students in the upper left quadrant are those who had decreasing MRS over time but increasing quiz scores, while students in the lower right quadrant had increasing MRS with decreasing quiz scores. For most of these students, their change in MRS is small, and upon scrutiny, may not be meaningful.
For example, in the category of grade increase with decreased MRS, a student (pink dot) started with an MRS of 1 and decreased to 0, expressing metacognitive knowledge early on: “The questions were [on a topic] I was least confident in … I felt I knew the material better.” Later, when performing better, this student stated: “Test scores” and “I am satisfied because I thought I was going to get a C.”
Another student (red dot) had higher MRS scores throughout, with a small drop at the end. This student initially made metacognitive knowledge statements, such as: “If I can understand the thought process and why you do certain things for certain questions, the material makes a lot more sense to me.” Later, the student made exclusively metacognitive regulation statements such as: “I have realized I learn when I go through the problems on my own and work them out.”
This student’s later statement suggests the student now has regulative capacity, an ideal shift that could yield more effective studying, and the higher grade obtained. The decreased MRS score, in this case, is not actually representative of a decrease in metacognition but merely a decrease in the number of times the student mentioned a metacognitive practice.
DISCUSSION
In this study, we confirmed prior findings that students tend to overpredict their grades (Kruger and Dunning, 1999) and that overpredictors generally perform worse than underpredictors (Dang et al., 2018; Osterhage et al., 2019). As shown in Figure 1, students with the largest overpredictions performed the most poorly and those with the largest underpredictions performed the best on individual quizzes. We also showed that students who consistently overpredicted their quiz grades tended to be low performers on other assessments: Students’ average grade predictions were strongly correlated with their summative grades (average quiz, final exam, and final course percent; Supplemental Figure 2). Given that the accuracy of grade predictions has been suggested as a readout of a student’s metacognitive monitoring capacity (Miller and Geraci, 2011), we suggest that, if students do not learn from an overprediction to appropriately adjust their strategies, they will likely continue to perform poorly. Possible reasons for lack of improvement could be not changing strategies at all or choosing a new strategy, but not using it appropriately, or not using it effectively. Students who consistently overpredict are likely unaware of how little they know, while students who develops such awareness may be able to predict their performance more accurately. In our study, students overall did not improve their prediction accuracy or performance over the course of the semester. This contrasts with the findings of Osterhage et al. (2019), who found improvements in both; however, given that higher grades are linked to higher prediction accuracy, this discrepancy is not surprising. In fact, in our study, students who made the most substantial positive grade changes from one quiz to the next were, on average, those who had initially made the largest overpredictions (Figure 2). Importantly, we found that individual students who trend from overpredicting toward matching or underpredicting also have the most improvement in their quiz scores (Figure 3). Underpredicting is not numerically more accurate than overpredicting, but shifting from over- to underpredicting does represent a positive change in student perception. Although underpredicting may be an expression of underconfidence (Hacker et al., 2008), at least students have become more aware of what they do not know or have not yet mastered.
What motivates a student to respond to the feedback of an overprediction? Because little is required of a student when selecting a predicted grade, it is possible that some students guess their grades without engaging in metacognitive awareness. Likely only students who are shocked by a lower grade than expected are motivated to understand their mistakes and expend more effort the next time (Dye and Stanton, 2017). Students who perform about the same or better than expected on a quiz may be overconfident, assuming they are more knowledgeable than they are. They may in turn overpredict their next score, because despite the fact that they put in less effort than necessary, their expectations remained the same. This can result in continuous overprediction and lack of improvement. Because students tend not to make as many changes past the halfway point of a course, being confronted with the need for change early is most beneficial (Sebesta and Speth, 2017; Osterhage et al., 2019). In Osterhage et al. (2019), students were exposed to the idea of miscalibration as a lack of awareness before their first exam. They were further encouraged to implement more retrieval practice to improve their knowledge. These students both performed better and had better calibration than a baseline group not exposed to either of these ideas. Thus, performing poorly and overpredicting early in the semester may work in a student’s favor, providing motivation to develop the necessary metacognitive regulation skills to succeed. Even though not all students will try to make an accurate prediction when asked, this simple instructional practice still has power in prompting students to reflect on their study strategies and learning.
The open-response questions we asked of students after they received their graded quizzes were also intended to stimulate students to engage in a metacognitive practice, using the knowledge of whether their predictions matched their actual performance. We hypothesized that students whose expectations had not been met (overpredictors) would take this opportunity to reflect on why their grades did not match their predictions. However, students who overpredicted did not subsequently provide more metacognitive responses than those who matched or underpredicted (unpublished data). This matches our other finding that low performers, who have by definition the most to gain, are actually less likely to make metacognitive reflections (Figure 4). In fact, many students chose not to reflect on their performance (and consent to this research): Although 73% of students consented to the research study and participated, only about 35% answered three or more sets of reflection questions. For each set of quiz reflections, a higher proportion of students with “A” grades responded than those in any other grade category (Figure 4). This sampling problem, although not extreme in our case, is a known issue: Higher-performing students tend to volunteer more for research studies than lower-performing students (Brooks et al., 2015), and minoritized students are also underrepresented (Theobald et al., 2020).
Although we do not know why lower performers responded less frequently in our course, possible reasons include that they did not want to think about their low performance, did not see value in the reflection exercise, or were disengaged from the course in general. Work on how students view failure suggests that mindset (fixed vs. growth), goal orientation (focus on mastery vs. performance), and fear of failure interact to influence how students ultimately cope with performance that does not match expectation (Henry et al., 2019). For example, students with a fixed (or stable) view of their ability may respond poorly to failure (give up), while those who believe they can improve are willing to take on effortful and/or new strategies. In fact, students in our data set who performed poorly and did respond to the reflection questions were significantly more likely than higher-performing students to attribute their poor grades to an external factor (our Blame code; Figure 4). This trend has been found by others as well (Hacker et al., 2008). Ultimately, low-performing students who attribute failure to external factors rather than to effort are unlikely to take advantage of the opportunity to learn from failure (Henry et al., 2019). These students are the ones who likely need the most help in learning how to use metacognitive strategies and are most likely to benefit from exactly these interventions.
Recent work from Sebesta and Speth (2017), in which students were surveyed regarding their approaches to studying, showed that students who improved their exam scores over the semester used five strategies more than those who decreased or maintained lower exam grades: self-evaluation, goal setting and planning, seeking information, reviewing notes, and reviewing exams. All of these strategies involve building metacognitive knowledge and metacognitive regulation (planning, monitoring, and evaluating). Students in our sample who had the highest use of such statements were also usually high performers (Figure 5). It is impossible to know whether students who performed well in Principles of Genetics from the outset were already aware of the benefits of metacognition (either consciously or unconsciously) or were better prepared than lower performers. It is, however, clear that not all high performers supply metacognitive responses. Some were metacognitive throughout the course, while others gave completely non-metacognitive responses, and many others bounced around in their responses. Because they are already successful, some high performers may feel they do not need consider how or why their strategies are working. Thus, the lack of correlation between change in MRS and change in performance over time (Figure 6) is not surprising. Some students became more metacognitive and improved their performance, while other became more metacognitive but continue to perform poorly. In reality, a single semester of experiences may just not be enough time for students to demonstrate growth in metacognition.
Why does a higher MRS (more metacognitive reflections) not drive quiz score improvement for all students? Because we combined metacognitive knowledge and regulation responses when generating the MRS score, and because we scored only presence or absence of metacognition, we may have missed subtle shifts from knowledge to regulation, as illustrated by one student (red dot, Figure 6), who demonstrated exactly this transition. Those who continued to achieve lower grades even though they expressed metacognitive ideas were likely at the “emerging” level of metacognition, meaning they could identify valuable practices but did not know how to implement them (Stanton et al., 2015). Awareness alone does not translate into improved performance: Only those who can actually regulate their behaviors and change their study strategies have this potential. Ultimately, fully understanding student changes in behavior will require detailed, fine-grained analyses, including exploring student motivation and self-efficacy and how other behavioral and environmental components affect self-regulation (Pintrich et al., 2000; Winne, 2018).
Putting It All Together
There is some evidence that, when students receive training and practice, they can improve their use of skills associated with metacognitive regulation (Perels et al., 2005; Rodriguez et al., 2018). For example, Rodriguez et al. (2018) found that training students to use self-testing resulted in an increased use of this strategy. Because self-testing is likely to reveal to students what they do and do not know, engaging in this practice may ultimately result in increased metacognitive regulation. However, making a lasting shift in student practices, enough to impact performance, has proven difficult. For example, one popular approach to stimulate metacognition is “exam wrappers,” in which students reflect on their performance by answering a series of reflection questions in which they identify errors and plan how they will prepare for the next exam (Lovett, 2013). Although students report value in such exercises, metacognitive scores and performance are not often directly affected. Soicher and Gurung (2016) compared students who had been prompted to do one of three exam reviews: simply review their exams; review and report which questions they answered incorrectly; or review and answer how they prepared, what kind of errors they made, and how they should prepare for the next exam. All groups of students made small improvements in their metacognitive awareness score (measured with the MAI; Schraw and Dennison, 1994) from the beginning to end of the course, but there were no differences in exam or course performance among the three groups. This is consistent with earlier findings that students need repeated exposure to metacognitive practices across multiple courses to improve their metacognitive awareness (Lovett, 2013). Another strategy has been to encourage the use of enhanced answer keys for exams, in which the instructor explains the rationale for each answer and provides a series of reflection questions to actively engage students in being metacognitive. These questions direct students to think about how they can increase their understanding and consider what to do differently in studying (Sabel et al., 2017). Interviewed students who used the keys demonstrated metacognitive awareness, reporting that the keys could be used as study tools and that the reflections could be incorporated into their studying. These students also performed better than those who did not use the keys. Ultimately, simply prompting students to be metacognitive is likely not a strong enough intervention to result in improved metacognitive awareness or performance (Stanton et al., 2015). Furthermore, because students who do not perform well are less likely to respond to metacognitive exercises, the challenge remains for instructors to create conditions in which all students access and use tools to improve metacognition and self-regulation (Tanner, 2012).
There is ample evidence that students benefit from self-testing and spacing rather than cramming (e.g., Roediger and Karpicke, 2006; Rodriguez et al., 2018), including using practice tests as a method to gauge preparedness. Osterhage (2021) showed that students who chose to take practice tests ultimately improved their performance over time compared with those who did not. However, the effects of practice tests on metacognitive awareness were different for overall lower-performing compared with higher-performing students. Lower-performing students continued to overpredict their performance despite engaging in practice testing (likely in part because their scores continued to be low), while higher-performing students moved toward underpredicting their performance. We also saw this trend (Figures 2 and 3) and interpret it as evidence that access to practice tests cannot benefit students if they do not connect their low performance to a need to study and prepare differently for tests. Students who are faced with evidence that they are not ready for a test through poor performance on a practice test still need to access additional practice questions tagged by content in order to truly learn the material. We think one way to provide this support to students is to design a system that delivers study options in a personalized way. One approach could be for students to mark questions as difficult or easy on a homework assignment, later be prompted with new questions on that content as they begin to prepare for an upcoming exam, and then be prompted to complete reflections on their learning at the same time. Combining metacognitive reflection and repeated testing in a setting the students are already using for their course work could make effective study strategies accessible to all students and allow them to positively regulate their learning.
Limitations
As has been true for all students and instructors since 2020, we faced disruption to our normal class context due to COVID-19. Half of Spring 2020 was in person, while the second half, including quizzes, was remote. For Spring 2021, the entire course and all quizzes were remote. It is possible that the online transition influenced student performance, motivation, and reflections. There is no obvious trend in the data to suggest this, aside from several comments from students that the online environment either worked very well or very poorly for them. In addition, we acknowledge the role of self-selection bias within our sample, as students who consistently respond to surveys and consent to have their data used are not a random sample, and some who respond without much detail may be thinking things they choose not to express. Finally, we acknowledge that neither prediction accuracy nor the MRS is a complete measure of student metacognition. Both surely result in a less-nuanced representation of complex student ideas than could be obtained through extensive interviews (Dye and Stanton, 2017). However, such scoring systems do allow for collection of student metacognition from many students rather than just those who can be interviewed.
ACKNOWLEDGMENTS
We thank Gretchen Wettstein for critical initial explorations of metacognition and self-regulated learning; Aidan Barker, Stefan Dalecki, and Grace Kennedy for coding assistance; and Josh Sun and Lucas Laughlin for preliminary statistical assistance. We also appreciate the valuable feedback from Lisa Corwin and Stephen Luckey on drafts of the article.