The Benefits of Participating in a Learning Assistant Program on the Metacognitive Awareness and Motivation of Learning Assistants
Abstract
Learning assistant (LA) programs train undergraduate students to foster peer discussion and facilitate active-learning activities in undergraduate science, technology, engineering, and mathematics (STEM) classes. Students who take courses that are supported by LAs demonstrate better conceptual understanding, lower failure rates, and higher satisfaction with the course. There is less work, however, on the impact that participating in LA programs has on the LAs themselves. The current study implements a pretest–posttest design to assess changes in LAs’ metacognition and motivation to succeed in STEM across their first and second quarters as an LA. Our findings suggest that participating in this program may help LAs become more reflective learners, as was demonstrated by an increase in their scores on the Metacognitive Awareness Inventory (MAI) after the first quarter. LAs also showed increases on the Intrinsic Motivation and Self-Efficacy subscales of the Science Motivation Questionnaire. Students who participated in the program for an additional quarter continued to show increases in their MAI scores and maintained the gains that were observed in their motivation. Taken together, this work suggests that, in addition to benefiting the learner, LA programs may have positive impacts on the LAs themselves.
There is no shortage of educational interventions aimed at increasing students’ success in science, technology, engineering, and mathematics (STEM). Some interventions target students’ understanding of specific course material or foundational concepts, whereas others aim to improve students’ approach to learning more broadly (e.g., by improving metacognition and study habits; Hoskins et al., 2017; van den Hurk et al., 2019; Brown-Kramer, 2021). Interventions that provide peer support or mentorship in the form of learning communities or peer learning assistants seem to be particularly beneficial (Groccia and Miller, 1996; Talbot et al., 2015; White et al., 2016). The Learning Assistant (LA) Program is one such form of peer instruction. Students who take courses that are supported by LAs demonstrate better conceptual understanding, lower failure rates, and higher satisfaction with the course (Otero et al., 2010; Talbot et al., 2015; White et al., 2016; Sellami et al., 2017). Although the benefits of providing LA support to students have been demonstrated across a variety of courses and programs (for a review, see Barrasso and Spilios, 2021), there is less work examining the impact on the undergraduate LAs themselves. Analysis of LAs’ written reflections suggest that LAs develop greater content understanding and stronger science identities through participating in the program (Close et al., 2016; Huvard et al., 2020). Students who have been LAs are also more likely to graduate compared to a matched sample of their peers (Otero, 2015), but the mechanisms behind some of these shifts have not yet been explored. Given the type of training and experiences that LAs have in supporting their peers, participating in a learning assistant program has the potential to make them better learners and change their attitudes about STEM, which may in turn contribute to some of these beneficial outcomes. In the current study, we examine changes in undergraduate students’ metacognition and STEM motivation before and after participating in the LA program.
The original model of the LA program was developed at the University of Colorado, Boulder in 2003 (Otero et al., 2010). This program was designed to incorporate more opportunities for active learning in large classes by recruiting undergraduate students and training them to support their peers’ learning (Otero et al., 2010). As such, the primary role of LAs was to facilitate learning during class time by fostering discussion and engaging with small groups of students. This model has since been adopted at more than 500 institutions (www.learningassistantalliance.org).
Undergraduate LA programs that are modeled after the University of Colorado program consist of three core components: 1) a pedagogy seminar that covers a variety of topics in learning and teaching, 2) an assistantship in a specific lab or lecture course, and 3) weekly meetings with the instructor and/or graduate teaching assistants (TAs) from that course. LA programs are distinct from other models of near-peer instruction and TA support in that LAs facilitate learning during class time (rather than in supplemental sessions) and do not grade or assess student work.
The LA program at University of California, Los Angeles (UCLA) began in 2016 with a team of 12 LAs in three courses. Since then, the program has grown to enroll approximately 500 LAs per quarter who support learning in more than 40 STEM courses (see https://ceils.ucla.edu/learning-communities-trainings/learning-assistant-program/). As in the model described earlier, all first-time LAs at UCLA take a seminar in pedagogy that includes instruction on how to scaffold student learning by asking open-ended questions and encouraging collaboration. LAs work with undergraduates in various instructional settings (e.g., in labs, office hours) as determined by the needs of their specific courses. The LAs also complete weekly reflections on their experiences and attend weekly course content meetings to prepare to support students.
In other peer instruction models in which students act as “teachers,” students report more positive attitudes toward the material they teach and better understanding of the content (Cohen et al., 1982; Amaral and Vala, 2009; Chrispeels et al., 2014). Indeed, participating in the LA program has been shown to strengthen LAs’ content knowledge of the specific courses that they support (Otero et al., 2010). This increase could be attributed, at least in part, to the known benefits of preparing to teach (Fiorella and Mayer, 2013) and having greater exposure to the course materials. In the current study, rather than assessing LAs’ understanding of discipline-specific topics, we are interested in how students’ experiences in this program might shift their approaches to learning more broadly.
Assessing Improvements in Metacognitive Awareness
If we think that participating in the LA program will help LAs become better learners, there are a variety of ways that we can assess this improvement. One measure of a “good learner” is metacognitive awareness. Metacognition, broadly, is the ability to think about one’s own thoughts (e.g., Flavell, 1979; Veenman and Spaans, 2005, Veenman et al., 2006). Metacognition can include a variety of cognitive processes, such as being able to select an appropriate problem-solving strategy and being able to monitor progress toward one’s own learning goals. Students who exhibit greater metacognitive awareness (i.e., who are better able to reflect on their own thinking and performance) tend to perform better academically (Nietfeld et al., 2005; Kelemen et al., 2007; Young and Fry, 2008; Ohtani and Hisasaka, 2018). They also tend to implement more effective learning strategies such as elaboration, organization, and critical thinking (Schraw and Moshman, 1995; Sperling et al., 2004). Given that LAs are trained to think carefully and reflectively about student learning, participating in the LA program could make LAs more sophisticated learners by improving their metacognitive awareness.
There is some evidence to suggest that interventions that teach metacognitive strategies can be effective at improving both metacognitive awareness (Saenz et al., 2019) and, by extension, content understanding (Mynlieff et al., 2014; Hensley et al., 2021). We know that student metacognition can be modified by a variety of different experiences, such as receiving appropriate feedback or failing to retrieve an item from memory (Miller and Geraci, 2014; Molin et al., 2020). In one comprehensive study, Saenz et al. (2019) systematically compared the efficacy of five different interventions aimed at improving metacognition on a logical reasoning task. The interventions took place between two successive administrations of the reasoning task. Participants either reviewed test questions, received salient feedback about their performance and metacognitive accuracy, were shown a warning lecture about how students are often overconfident, were told that they could earn money if they were well calibrated, reflected on their knowledge, or completed a maze activity (control). In this task, the most successful intervention involved making feedback salient to the participants.
Metacognitive growth has also been assessed in a variety of classroom settings (Kramarski and Mevarech, 1997; Miller and Geraci, 2011; Nietfeld et al., 2006; Callender et al., 2016; Sabel et al., 2017; Molin et al., 2020). In one study, students in two sections of an introductory biology class completed the Metacognitive Awareness in Reading Strategies Inventory (MARSI) scale at the beginning and end of the course (Hill et al., 2014). One section of the class was given two 50-minute study skills lectures that included the Survey-Question-Read-Recite-Review method and an in-person discussion about metacognition; the other section watched the lesson online. Students in both sections were asked to apply the strategies they learned on homework assignments that followed the lessons. At posttest, students in both sections scored higher on the MARSI and measures of reading comprehension. Although students’ ability to read and reflect on academic materials improved, it is unclear whether similar gains would be seen on broader measures of metacognition (that assess how students approach and solve problems in general rather than specifically examining changes in reading strategies).
When metacognitive interventions are aimed at changing students’ thinking about a specific task (i.e., such as being able to reflect on the strategies needed to complete a specific task/problem), the metacognitive intervention may be more likely to shift content-specific metacognition but might have less of an impact on the learner’s overall metacognitive awareness. While some studies focus on shifting metacognition in a specific domain (e.g., see Hill et al., 2014; Dahlberg et al., 2019), others studies conceptualize metacognition more broadly and aim to shift participants’ general ability to reflect on their learning. In the current study, given that the course content and specific problem-solving strategies vary depending on the courses that student LAs support (and our LAs support a variety of courses), we examined metacognitive changes that are not tied to a specific domain.
There are a number of different ways of assessing broad or domain-general metacognition. Some studies have used self-report measures (e.g., Sperling et al., 2004; Young and Fry, 2008) or qualitative coding of written reflections (e.g., Huvard et al., 2020). However, questionnaires are some of the most common methods of measuring metacognition (Dinsmore et al., 2008), attributable at least in part to the practicality of their use (Berger and Karabenick, 2016). These questionnaires ask participants to evaluate, for instance, the extent to which they are aware of what they have learned versus what they need to study more, or whether they have skills to appropriately troubleshoot when they face difficulties. Questionnaires like the Metacognitive Awareness Inventory (MAI; Schraw and Dennison, 1994) are not as closely tied to problem solving or learning in a particular subject area as other self-report measures like the Metacognitive Activities Inventory (which looks at problem solving for chemistry problems; MCAI; Cooper and Sandi-Urena, 2009). Thus, the MAI allows for a more global assessment of how reflective the learner is irrespective of the context.
Due to its broad applicability and ease of administration, the MAI developed by Schraw and Dennison (1994) has become one of the more popular self-report measures of metacognitive awareness in educational settings. It assesses two aspects of metacognition: knowledge of cognition and regulation of cognition. The Knowledge of Cognition scale assesses the learner’s knowledge of strategies and skills to appropriately solve problems. In contrast, the Regulation of Cognition scale assesses whether learners can monitor their progress and allocate attentional resources appropriately. Higher scores on the MAI have been associated with greater use of learning and study strategies (Sperling et al., 2004), improved test performance (Schraw and Dennison, 1994; Zulkiply et al., 2008), and higher course grades and cumulative grade point average (GPA; Young and Fry, 2008).
Across a number of studies designed to improve student metacognition, as indexed by the MAI, evidence for the effectiveness of interventions is mixed. For example, in one intervention, students completed various “exam wrappers,” a type of post-exam activity. Some wrappers included metacognitive reflection (e.g., How did your actual score compare to how you thought you did on the exam after taking it?), and others did not. Overall, students’ MAI scores increased from the beginning to the end of the term. However, the magnitude of this change did not differ based on the type of wrapper completed (Soicher and Gurung, 2017). Other studies have been able to successfully move MAI scores (e.g., Terlecki and McMahon, 2018; Alt and Raichel, 2020). For instance, Terlecki and McMahon (2018) found that enrollment in an interactive metacognition course was associated with a significant improvement in MAI scores over the course of a term. In contrast, enrollment in courses in cognition or introductory psychology, which included some topics related to memory and problem solving, did not show any improvement.
In the current investigation, the MAI was administered at the beginning and end of each quarter to determine how the LA experience may impact scores. Given that the pedagogical seminar explicitly discusses metacognition, and LAs are asked to reflect on their use of problem-solving strategies to help support their peers’ learning, the LA program may improve LAs’ general metacognitive abilities.
Assessing Gains in Motivation
In addition to evaluating whether LAs show improvements in metacognition, we evaluated whether participating in the LA program might increase students’ interest and drive to succeed in STEM. There is a growing body of evidence to suggest that student motivation is associated with better grades (Lin et al., 2003; Glynn et al., 2011) and greater persistence in STEM (Simon et al., 2015). Although higher motivation seems to lead to better academic outcomes, the source of that motivation might also play a role in facilitating success. Some measures, like the Science Motivation Questionnaire (SMQ-II; Glynn et al., 2011), attempt to measure the extent to which a student’s desire to succeed in STEM is motivated by specific internal (e.g., intrinsic motivation, self-efficacy, self-determination) or external components (e.g., career motivation, grade motivation). STEM majors tend to score higher in all five components compared to non–STEM majors (Glynn et al., 2011), but there is some indication that more internally driven components, such as higher self-efficacy, are better predictors of future academic success (Robbins et al., 2004; Richardson et al., 2012; Austin et al., 2018). There is also some evidence suggesting that the source of motivation can vary depending on a student’s demographic characteristics (Glynn et al., 2009; Kassaee and Rowell, 2016; Young et al., 2018). For example, some studies have found that women tend to score lower than men on the self-efficacy scale and higher on the self-determination scale (Glynn et al., 2009; Young et al., 2018; however, see Kassaee and Rowell, 2016, which found no gender differences).
Given the association between motivation and success in STEM, examining the effects of educational interventions on students’ motivation is of primary interest. In prior work, motivational measures are typically not themselves the targets of change but are instead used to predict which students will gain the most from an education intervention (Goldschmidt and Bogner, 2016; Hibbard et al., 2016; Schumm and Bogner, 2016). The interventions that do directly target improvements in motivation as an outcome often consist of short programs or classroom activities designed to engage students or spark their interest in STEM (Marth and Bogner, 2017; Linnenbrink-Garcia et al., 2018; Evans et al., 2022; Heim and Holt, 2022). In these studies, participants are typically asked to complete the motivation measures before and after the intervention (Marth and Bogner, 2017; Evans et al., 2022; Heim and Holt, 2022), or motivation is compared between groups that experienced the intervention versus those that did not (Kassaee and Rowell, 2016; Olimpo et al., 2016).
The findings of these intervention studies are mixed. Some interventions, like taking undergraduate biology students to the zoo, have demonstrated long-term increases in self-efficacy and decreases in grade-based motivation (Heim and Holt, 2022). Other interventions have shown temporary, but not long-term changes. For example, one study found that giving sixth graders an outreach module about bionics temporarily increased ratings on items that addressed their intrinsic motivation and self-efficacy (Marth and Bogner, 2017). However, not all interventions seemed to impact attitudes (Cleveland et al., 2017; Edwards et al., 2021). Some interventions that have successfully shifted attitudes share a number of characteristics, including occurring over multiple weeks or months (Muis et al., 2010; Feldon et al., 2018; Covert et al., 2019; Karpudewan and Chong, 2020; Dixon and Wendt, 2021), and being active or experiential in nature, such as a summer science program, online laboratory activity, or game-based course (Linnenbrink-Garcia et al., 2018; Covert et al., 2019; Srisawasdi and Panjaburee, 2019; Karpudewan and Chong, 2020). Given that the LA program occurs over the course of a quarter (10 weeks) and requires LAs to be actively engaged, we believe that the program has the potential to positively impact LAs’ motivation to succeed in STEM. Although motivation has not been assessed directly in this population, qualitative analyses of statements made by LAs during teaching reflections, interviews, and applications to serve as an LA in subsequent semesters suggest that their experiences in the program made them feel more confident and competent in physics (Close et al., 2016). LAs also report higher interest in the subject matter after participating in the program (Otero et al., 2010). It is possible that these positive attitudes toward STEM may also be associated with greater motivation to succeed in STEM courses.
The Current Study
In the current investigation, we used a pretest–posttest design to explore how participating in the LA program may benefit LAs. We evaluate changes in both first-time LAs and in LAs who participated in the program for a second quarter. As the LA program at our university is large and LAs assist in a variety of courses, our data represent an overall LA experience—not one that relies on the idiosyncrasies of a particular course. Given this variety of experiences, we are well positioned to assess the broad impact of being an LA on metacognitive awareness and motivation to succeed in STEM. The current study addressed the following two questions:
Does the LA program make LAs more effective learners? In particular, can it lead to changes in metacognitive awareness (measured using the MAI)?
Does the LAs’ motivation to succeed in STEM (measured using the SMQ) change over the course of their enrollment in this program?
METHODS
LAs apply to serve in specific courses, and individual course instructors have different criteria for screening and selecting LAs. Over the 2019–2020 school year, an average of 632 LAs applied to the program each quarter; some of these students had participated in the program before and others had not. Of those who applied, 70.2% were accepted into the program, and the majority (84.9%) of those who were accepted enrolled (total program enrollment in Fall 2019 = 341 students, Winter 2020 = 404 students, Spring 2020 = 385 students). All undergraduates participating in the LA program were asked to complete a survey that included the MAI and SMQ measures at the beginning and end of each quarter. The methodology of this study and linking our student data to demographic data from the registrar’s office was approved by the UCLA Institutional Review Board (IRB no. 19-001995).
Participants
In total, 505 students served as LAs for the first time in the 2019–2020 academic year. Of those, 443 students completed the survey before and after their first quarters in the program. Fifty-eight students were excluded from the final sample; 50 because they only completed one of the two surveys and eight because they could not be identified or linked to the course roster. In the final data set (N = 443), 11 students responded to either the pre or post survey more than once. In these cases, we used the first set of responses. Based on demographic data obtained from the registrar, the students in the data set were predominately female (60.2%)1 and were admitted into UCLA as freshman (94.0%). About a quarter of students were first-generation college students (23.4%)2, and 16.3% self-identified as Black/African American, Latinx/Hispanic, or Native American/Alaskan Native3 (see Table 1 for demographic information). Most students in this sample were admitted to UCLA in Fall 2017 (31.9%) or Fall 2018 (44.4%), meaning that most students were in their second or third year at UCLA. The distribution of participants included in the data set by subject area is shown in Table 2. Of the 443 first-time LAs, 175 also completed the measures again at the end of their second quarter participating in the program. Students received a small amount of course credit in exchange for completing the surveys.
N (%)a | |
---|---|
Year at UCLA | |
First | 27 (6.3%) |
Second | 192 (44.4%) |
Third | 138 (31.9%) |
Fourth | 71 (16.4%) |
Fifth | 4(0.9%) |
Admit type | |
Freshman | 406 (94.0%) |
Transfer | 26 (6.0%) |
Gender | |
Male | 172 (39.8%) |
Female | 260 (60.2%) |
Identified as Black/African American, Latinx/Hispanic, or Native American/Alaskan Native | |
Yes | 68 (16.3%) |
No | 348 (83.7%) |
First-generation college student | |
Yes | 101 (23.4%) |
No | 324 (75.0%) |
Unknown/Other | 7 (1.6%) |
Subject area | Fall 2019 | Winter 2020 | Spring 2020 |
---|---|---|---|
Life sciences | 71 | 42 | 36 |
Chemistry | 61 | 39 | 36 |
Physics | 25 | 17 | 12 |
Computer science | 12 | 10 | 15 |
Mathematics | 16 | 8 | 14 |
Psychology | 7 | 9 | 7 |
Other STEMa | 2 | 4 | n/a |
Total | 194 | 129 | 120 |
Measures
MAI.
In this study, we administered the shortened 19-item version of the MAI developed by Harrison and Vallin (2018), which had two subscales: Knowledge of Cognition (eight items) and Regulation of Cognition (11 items). As in the longer 52-item version (Schraw and Dennison, 1994), the Knowledge of Cognition subscale assesses the extent to which students are aware of their own thought processes and contains items such as “I am aware of what strategies I use when I study.” The Regulation of Cognition subscale assesses students’ ability to allocate resources to cognitive tasks and contains items such as “I change strategies when I fail to understand.” Participants responded to each question on a five-point Likert scale from 1 (not at all typical of me) to 5 (very typical of me). The items on the Knowledge (range: 8–40 points) and Regulation (range: 11–55 points) subscales were summed separately and were combined to create a composite MAI score (range: 19–95 points). Higher scores indicate a greater degree of metacognitive awareness. As in previous work (Harrison and Vallin, 2018), the composite MAI had good internal consistency in our sample (Cronbach’s alpha for pre data = 0.898; Cronbach’s alpha for post data = 0.914). Internal consistency was similarly high for each of the subscales (pre data: Cronbach’s alpha for Knowledge = 0.862; Regulation = 0.819; post data: Cronbach’s alpha for Knowledge = 0.870; Regulation = 0.853). Harrison and Vallin (2018) found that the shorter 19-item two-factor version of the MAI had the best fit to their data collected from an undergraduate sample. In our sample, confirmatory factor analysis4 indicated that a two-factor model did not fit our data particularly well (according to the recommendations of Hu and Bentler, 1999); thus, we were cautious in our interpretation of the separate subscales (pre data: comparative fit index (CFI) = 0.856, Tucker-Lewis index (TLI) = 0.837, root mean square error of approximation (RMSEA) = 0.079; post data: CFI = 0.877, TLI = 0.861, RMSEA = 0.079).
SMQ.
The SMQ-II (Glynn et al., 2011) is a 25-item scale designed to measure the degree to which students’ motivation to learn science is driven by five dimensions: Intrinsic Motivation, or learning for its own sake (e.g., “I am curious about discoveries of STEM”), Career Motivation (e.g., “Understanding STEM will benefit me in my career”), Self-Determination, or a sense of responsibility for their own learning (e.g., “I put enough effort into learning STEM”), Self-Efficacy, or confidence in learning science (e.g. “I believe I can master STEM knowledge and skills”), and Grade-Based Motivation (e.g., “I like to do better than other students on STEM tests”).5 Students indicated how much each statement applied to them on a five-point Likert scale from 0 (never) to 4 (always). Each subscale contains five items total and has a possible score of 0–20. A higher score on each subscale indicates a greater motivation from that source (i.e., scoring higher on the Intrinsic Motivation subscale indicates greater motivation to learn science for its own sake). All five subscales had good internal consistency in our sample (pre data: Cronbach’s alpha for Intrinsic Motivation = 0.792, Career Motivation = 0.788, Self-Determination = 0.805, Self-Efficacy = 0.866, Grade-Based Motivation = 0.831; post data Cronbach’s alpha for Intrinsic Motivation = 0.858, Career Motivation = 0.868, Self-Determination = 0.865, Self-Efficacy = 0.903, Grade-Based Motivation = 0.860). The five-factor model fit relatively well to our pre data set (CFI = 0.905, TLI = 0.892, RMSEA = 0.062) and our post data set (CFI = 0.900, TLI = 0.887, RMSEA = 0.077). The fit of the five-factor model to our data was similar to Glynn et al. (2011; i.e., Glynn’s CFI = 0.91, RMSEA = 0.07).
Procedure
At the beginning of each quarter, LAs were asked to complete a survey containing the MAI and the SMQ. In their first quarter as an LA, students attended a 10-week pedagogy seminar. The major topics included: asking open questions to help students build understanding, fostering collaboration and growth mindset, recognizing and working to counteract systemic issues in education, and metacognition. Although the seminar is large (∼180 students), there is an emphasis placed on active learning. As part of the seminar, LAs are given opportunities to role play and practice applying the teaching strategies that they are learning about.
Notably, the seminar included one explicit lesson about metacognition. In this lesson, students were introduced to a metacognitive problem-solving process modeled after Ambrose et al. (2010; see Figure 1). The LAs discussed how they could apply this method to their own experiences and in their LA classrooms.

FIGURE 1. Diagram used to explain the metacognitive problem-solving process during the lesson on metacognition. This figure was adapted from Figure 7.1 of Ambrose et al. (2010).
In some courses, LAs supported students in labs, and in others, they assisted with learning activities that were part of the lecture. LAs may have also had the opportunity to work with students during office hours. Finally, all LAs completed written reflections each week. The reflection topics varied, but in general, they were asked to reflect on their experiences as LAs and the topics that they discussed in the seminar course.
LAs were asked to complete a survey containing the MAI and the SMQ again at the end of each quarter.
Analyses
Changes in MAI and SMQ scores were assessed from the beginning to the end of students’ first quarter in the program using repeated-measures analyses of variance (ANOVAs) and t tests conducted using SPSS v. 28. For the SMQ, because there are multiple subscales, t tests were Bonferroni corrected to adjust for the fact that there were multiple comparisons. Similar analyses were conducted to examine changes in MAI and SMQ scores from the end of LAs’ first quarter to the end of their second quarter in the program.
RESULTS
First-Quarter LAs
Metacognition.
A repeated-measures ANOVA was used to compare LAs’ total MAI scores at the beginning and end of their first quarter participating in the program. Given that there may have been differences in LAs’ experiences in Fall and Winter (where instruction was in person) compared to Spring (which was online), Quarter (Fall, Winter, Spring) was included in the ANOVA as a between-subjects factor. Overall, we observed an increase in MAI scores after participating for the first time in the program, F(1, 440) = 22.42, p < 0.001, ηp2 = 0.05. There was no difference in MAI scores across quarters, F(2, 440) = 1.73, p = 0.179, ηp2 = 0.01, and no interaction between Quarter and MAI scores, F(2, 440) = 2.14, p = 0.119, ηp2 = 0.01, indicating that the magnitude of the change in MAI scores that occurred from pre to post did not vary significantly across quarters.
Overall, MAI scores increased by 1.92 points (out of a possible 95 points) after students’ first quarter in the LA program (see Figure 2). In line with Harrison and Vallin (2018), we also evaluated the change in each of the two subscales individually. Here, we found that both the Knowledge of Cognition scores (mean difference = 0.55, SD = 4.23), t(442) = 2.73, p = 0.007, d = 0.13, and Regulation of Cognition scores (mean difference = 1.37, SD = 5.42), t(442) = 5.34, p < 0.001, d = 0.25, increased from the beginning to the end of the quarter. It does not seem to be the case that the increases observed in MAI scores were driven primarily by changes in one or two items, as the mean scores of 15 out of the 19 items increased. Numerically, the largest mean increases were observed on the following three items: “I ask myself if I learned as much as I could have once I finish a task” (mean increase = 0.25 points, SD = 1.18), “I know when each strategy I use will be most effective” (mean increase = 0.21 points, SD = 0.93), and “I use the organizational structure of the text to help me learn” (mean increase = 0.20 points, SD = 1.06). Multiple aspects of the program could have led LAs to indicate that these (or other) items were more typical of them. The LA program focuses heavily on reflection; each week, students are asked to reflect on what they learned in the seminar and on their experiences working with students. The act of continuously reflecting on one’s own learning and the learning of others may have helped LAs to see themselves as people who typically assess their own learning at the end of each task.
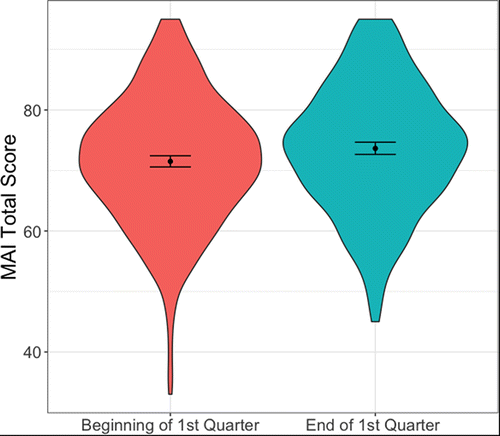
FIGURE 2. MAI total scores at the beginning and end of students’ first quarter working as LAs. Error bars represent 95% confidence intervals.
Items that specifically pertain to applying appropriate problem-solving strategies could be influenced by the training that LAs receive to support students during class time. The strategies LAs are given are not discipline specific, but are general strategies that could be applied to any problem or question, such as guiding the learner to make connections to the lecture material or reflect on prior knowledge that could be applied to the current problem (or the types of problems they have previously seen). The LA program may be particularly effective in moving these kinds of items, because not only do LAs explicitly learn about these metacognitive strategies, they also practice helping other students apply these strategies to solve problems in class each week.
STEM Motivation.
To assess whether participating in the LA program led to differences in students’ STEM motivation, a series of paired-sample t tests6 were performed to compare students’ pre and post scores on each SMQ subscale (i.e., Intrinsic Motivation, Career Motivation, Self-Efficacy, Self-Determination, Grade-Based Motivation; see Table 3). LAs reported higher intrinsic motivation and self-efficacy at the end of the quarter compared to the beginning of the quarter. Scores on the Career Motivation and Self-Determination subscales also tended to increase; however, neither change reached significance. Students also showed a numerical decrease in their grade-based motivation.
Pre first quarter M (SD) | Post first quarter M (SD) | t | p | d | |
---|---|---|---|---|---|
Intrinsic Motivation | 15.80 (2.72) | 16.18 (2.96) | 3.33 | <0.001 | 0.16 |
Career Motivation | 17.39 (2.39) | 17.46 (2.74) | 0.65 | 0.517 | 0.03 |
Self-Determination | 16.23 (2.72) | 16.36 (2.95) | 1.13 | 0.260 | 0.05 |
Self-Efficacy | 14.53 (3.33) | 15.09 (3.52) | 4.36 | <0.001 | 0.21 |
Grade-Based Motivation | 17.37 (2.83) | 17.17 (2.97) | −1.90 | 0 .058 | 0.09 |
Post first quarter M (SD) | Post second quarter M (SD) | t | p | d | |
---|---|---|---|---|---|
Intrinsic Motivation | 16.10 (2.95) | 16.49 (2.59) | 2.01 | 0 .046 | 0.15 |
Career Motivation | 17.46 (2.84) | 17.52 (2.73) | 0.31 | 0.759 | 0.02 |
Self-Determination | 16.48 (2.99) | 16.69 (2.76) | 1.02 | 0.310 | 0.08 |
Self-Efficacy | 15.11 (3.37) | 15.58 (3.09) | 2.16 | 0.032 | 0.16 |
Grade Motivation | 17.01 (3.11) | 17.13 (2.83) | 0.62 | 0.539 | 0.05 |
An exploratory post hoc analysis examined whether being an LA improved motivation for particular groups of students who, based on previous work, might be at higher risk for leaving STEM fields (in particular female students and students from underrepresented groups; e.g., see Chen, 2013). To assess this, we conducted a multivariate analysis of variance (MANOVA) with the difference in each SMQ subscale from pre to post included as dependent variables. Gender and race/ethnicity7 were included as independent variables. We found no significant differences in the amount of change observed in each of the five subscales depending on LA gender, F(5, 409) = 1.45, p = 0.205, ηp2 = 0.02, or whether the LA identified as either Black/African American, Latinx/Hispanic, or Native American/Alaskan Native, F(5, 409) = 0.60, p = 0.704, ηp2 = 0.01. Given previous work suggesting that there might be demographic differences in SMQ scores (e.g., Glynn et al., 2011), we also explored whether there were any baseline differences in SMQ scores in our sample before program participation. The results of a MANOVA predicting pre-SMQ subscale scores revealed a main effect of gender, F(5, 409) = 11.65, p <0.001, ηp2 = 0.13, but not race/ethnicity, F(5, 409) = 1.13, p = 0.343, ηp2 = 0.01. Separate univariate ANOVAs on the outcome variables indicated that there were baseline differences in self-efficacy, F(1, 413) = 31.82, p <0.001, ηp2 = 0.07, with female students reporting lower self-efficacy before participating in the program compared to male students. Taken together, these findings suggest that although participating in the LA program may lead to overall improvements in intrinsic motivation and self-efficacy, the program does not seem to have a larger impact on the SMQ scores of female students or students who identified as Black/African American, Latinx/Hispanic, or Native American/Alaskan Native.
Returning LAs
Metacognition.
Given that many students are involved in the LA program for more than one quarter, we were interested in examining whether there were longer-term changes in MAI and SMQ scores. A total of 175 of the 443 LAs who completed pre and post measures in their first quarter in the program also completed the same survey at the end of their second quarter. We conducted a repeated-measures ANOVA with MAI scores at three time points: the beginning of their first quarter, the end of their first quarter and the end of their second quarter. There was a significant main effect of experience in the program, F(2, 348) = 9.80, p <0.001, ηp2 = 0.05. Bonferroni-corrected pairwise comparisons indicated that there were increases in MAI scores from the end of the first quarter to the end of the second quarter (mean difference = 1.41, p = 0.046). There was also an increase from the beginning of the first quarter to the end of the second quarter (mean difference = 2.66, p < 0.001). Note that in this subset of the original sample, the change in MAI scores from the beginning to the end of the first quarter increased but did not reach statistical significance (mean difference = 1.25, p = 0.103). Overall, these findings suggest that metacognitive awareness continued to improve after serving as an LA for a second quarter. This increase occurred even though students did not take the pedagogy seminar again. It is possible that other aspects of the LA experience, for example, scaffolding students’ learning during the lectures/labs, could have helped to foster these continued gains in metacognitive awareness.
Importantly, it does not seem to be the case that the LAs who chose to continue in the program for a second quarter were different in terms of their MAI scores compared with the students who left the program after their first quarter.8 The initial MAI scores of LAs who continued into their second quarter (M = 72.98, SD = 9.77) were similar to those who only participated for one quarter (M = 71.11, SD = 11.60), t(299) = 1.52, p = 0.131, d = 0.18. We also did not observe differences in post–first quarter MAI scores, t(299) = 1.17, p = 0.244, d = 0.14, or in the difference between pretest and posttest in the first quarter, t(220.31) = 0.39, p = 0.700, d = 0.05.9 Numerically, the LAs who did not participate again actually showed slightly larger gains in metacognitive awareness across their first quarter as an LA (1.67 points vs. 1.25). In addition to students who graduated or did not have the opportunity to continue (as the Spring quarter was their first time participating in the program), there are several logistical reasons why first-time LAs may have not continued on with the program (e.g., they may not have had room in their academic schedules).
STEM Motivation.
Using the same subset of 175 LAs, we assessed whether there were continued changes in students’ STEM motivation across their second quarter as an LA. To assess this, we conducted a series of paired-sample t tests10 to compare SMQ subscale scores (i.e., Intrinsic Motivation, Career Motivation, Self-Efficacy, Self-Determination, Grade-Based Motivation) from the end of LAs’ first quarter to the end of their second quarter (see Table 4). Paired-sample t tests revealed no significant differences. However, we observed numeric gains in the same subscales (i.e., Intrinsic Motivation and Self-Efficacy) that showed changes across the first quarter. These results suggest that gains from the first quarter in the program are sustained across the second quarter but do not substantially increase. Similar to our analysis of MAI scores, we might wonder whether the students who continued in the program were more motivated than those who did not. Here, we do not see any differences in the motivation of these two groups before (all |t| < 1.12, p > 0.262) or after (all |t|< 1.41, p > 0.161) their first quarter in the program.
DISCUSSION
Learning assistant programs improve outcomes for students enrolled in LA-supported courses (Otero et al., 2010; Talbot et al., 2015; White et al., 2016). However, few studies have examined the impact that participating in an LA program has on the LAs themselves. After students’ first quarter participating in a large, multi-course LA program, we observed small but promising improvements in metacognitive awareness, intrinsic motivation, and self-efficacy. Metacognitive awareness continued to increase after students’ second quarter in the program, while gains in motivation were maintained. Given these results, LA programs have the potential to bolster success in STEM for both the students enrolled in LA-supported courses and the LAs themselves.
The current investigation takes a broad approach to studying the benefits of being an LA by following a large cohort of students who supported a variety of courses. Given the inherent variability in the courses and types of activities that LAs assisted with, the active ingredient that led to the observed changes in motivation and metacognition is not necessarily clear. In fact, it is difficult to imagine that one specific activity could have driven these results. Instead, we propose that the gains we observe likely result from multiple aspects of students’ experiences in this program. The present findings align with other interventions showing that explicit instruction in metacognition in a term-length course can improve metacognitive awareness (Terlecki and McMahon, 2018). Similar to programs that have increased students’ metacognition (Sandi-Urena et al., 2011; Santangelo et al., 2021), the LA program occurred over the course of a quarter and is a highly interactive experience. We also assume that students’ role in preparing to teach and support their peers (see Fiorella and Mayer, 2013) may have also contributed to these gains. Finally, the act of writing weekly reflections on their experiences as LAs might have led to beneficial gains in metacognition but also might have increased LAs’ interest and motivation to succeed in STEM. Although students were not instructed to specifically write about the utility of the course material or in-class lab activities they supported, previous work has suggested that utility-based reflections can lead to improvements in student motivation and retention in STEM courses (Canning et al., 2018; Erickson et al., 2021). Future work is needed to tease apart the unique contributions of different components of the students’ experiences in this program.
It is particularly promising to observe that metacognitive awareness continues to increase across LAs’ second quarter in the program. We also see that the changes in motivation (i.e., intrinsic motivation and self-efficacy) are sustained across students’ second quarter. This is particularly impressive, given that student motivation typically decreases over the course of the academic term (Young et al., 2018). Given the nature of our data, we cannot rule out the possibility that the continued growth could also be attributed to changes that occur as students’ progress through their degrees. To rule out this possibility, we would need to study a sample of similarly motivated non-LAs longitudinally, a difficult task given the highly selective nature of the program. Additional work is needed to examine whether the gains observed here in metacognition and motivation are sustained even after students leave the program.
Limitations
Although we observe improvements in MAI scores, similar to other educational interventions (e.g., interventions aimed at fostering a growth mind set to improve academic achievement; Sisk et al., 2018), the size of these effects tends to be small (for a discussion, see Kraft, 2020). On average, we observe a 1.9-point change on the MAI scale, which would indicate that LAs are endorsing one or two items as being “more typical of them” as learners after participating in the program.
It is important to consider that there may be limitations to using self-report questionnaires to study metacognition (for a discussion, see Boekaerts and Corno, 2005; Cromley and Azevedo, 2006). One potential issue with this approach is that it relies on the learners’ ability to reflect on their use of strategies, which may be largely unconscious (Veenman et al., 2006). It is plausible that the metacognitive processes of more advanced learners are more automated, and thus they may be less aware of their use of these strategies. It is also difficult to ascertain how small (1 or 2 point) increases in MAI scores might translate into “real-world” changes in behavior. We know from previous work that MAI scores do correlate with academic outcomes like course grades (r = 0.19) and GPA (r = 0.23); however, these correlations are relatively small (Young and Fry, 2008).
Even though we used the MAI, a broad measure of metacognition, it is possible that students’ metacognitive abilities were primarily growing in the specific subject area covered by their LA assigned courses. That is, when answering questions on the MAI, they could have been thinking of themselves as a “physics student” or “biology student.” Most studies do not combine broader measures of metacognitive awareness (like the MAI) with measures that are specific to course content (i.e., the strategies being used to solve particular types of problems). This type of research is necessary to begin to understand the links between domain-general and domain-specific metacognitive awareness and the degree to which domain-specific experiences impact the way learners think about their learning more broadly.
In prior work and in the present data set, motivation and MAI scores are treated as separate measures. However, we acknowledge that there is some overlap between these constructs (e.g., Sperling et al., 2004). In our sample, MAI scores before participating in the program are correlated with students’ Intrinsic Motivation (r = 0.42), Career Motivation (r = 0.38), Self-Efficacy (r = 0.55), Self-Determination (r = 0.57), and Grade-Based Motivation (r = 0.17). Successful learners likely possess both the motivation and the cognitive skills to succeed. An important contribution of this work is showing that programs that train students to work with peers can actually support students’ growth in both of these areas. However, given that the students who choose to enroll in our LA program are already highly motivated and highly reflective students to begin with, many participants were already using responses at the top of the scales. In comparison to Glynn et al.’s (2011) sample of STEM majors, our sample is almost 1 point higher on average on each SMQ subscale on the pre–first quarter survey. Thus, in most areas, there might have been less room to “grow” compared with other studies. Although male and female students demonstrated similar overall motivation, female LAs did, as reported in previous work (Glynn et al., 2009; Young et al., 2018), begin the LA program with lower self-efficacy than male LAs. However, unlike researchers examining other types of experiences that strengthen students’ science identity (e.g., having the opportunity to develop and test their own hypotheses; Starr et al., 2020), we did not find evidence that participating in the LA program led to a larger motivational boost for women or students from underrepresented groups. It follows that we might see even larger improvements if an LA program were implemented in a sample that had lower baseline motivation and metacognitive awareness scores.
Instructional Implications
As the goal of the LA program is to support the learning and success of undergraduate STEM students, it is important to consider the potential benefits to students who offer their time and energy to serve as LAs. Our findings suggest that the LA program does have a positive impact on these students by supporting the development of their metacognitive awareness and improving their motivation to succeed in STEM. Although we were unable to assess whether the changes we observed were associated with other future outcomes, MAI scores have been positively associated with GPA and course grades in other studies (Young and Fry, 2008). We are hopeful, therefore, that the learning strategies developed in the LA program have broader impacts on student LAs as they progress toward their degrees.
Many of the individual items on the MAI align with the broader problem-solving and inquiry-based skills that STEM education aims to foster, such as learning how to solve a problem, how to search for resources when you get stuck, and how to ask questions to help further understanding. Although we did not directly manipulate aspects of the LA program to determine which components led to these changes, our findings highlight the potential importance of emphasizing problem-solving strategies in the LA seminar and having students reflect on their experiences applying these techniques when working with their peers.
CONCLUSION
The current domain of research presents rich possibilities for assessing the outcomes of LA program participation. Longer-term changes to other meaningful outcomes such as success in future STEM courses and retention in STEM majors should be of prime interest.
FOOTNOTES
1 Demographic data were obtained from the registrar’s office. As we did not survey students directly, we are limited in how we can report the data based on the way the questions were asked on the admission survey. On the admission survey, students were asked to identify their gender as male or female. Unfortunately, this does not allow us to report the percentage of students who would have answered this question differently if other options were provided to them. Demographic data could not be obtained for 11 students.
2 First-generation college students are defined as students whose parent(s) did not complete a bachelor’s degree based on registrar data.
3 The data we obtained from the registrar indicate the percentage of students who identified as either Black/African American, Latinx/Hispanic, or Native American/Alaskan Native. We do not have access to the exact breakdown of how many students belong to each group, due to the small sizes of some of the groups. This information could not be obtained for 27 students whose ethnicity/race was unstated or unknown.
4 Confirmatory factor analysis was conducted using the Lavaan package in R (Rosseel, 2012).
5 In order to make the SMQ items applicable to all LAs (some of which were LAing in Engineering and Math courses) the word “science” (i.e., I am curious about the discoveries of science) was replaced with “STEM” for all SMQ Items.
6 To correct for multiple comparisons, the alpha level was Bonferroni adjusted. Tests in which p < 0.01 were considered statistically significant.
7 In the data we obtained from the registrar, race/ethnicity was a dichotomous variable that categorizes the sample into two groups: students who identify as either Black/African American, Latinx/Hispanic, or Native American/Alaskan Native and students who did not. We do not have access to the exact breakdown of how many students belong to each ethnicity/race group, due to the small sizes of some of the groups.
8 To compare students who “continued” to those who “did not,” we excluded the first-time Spring quarter LAs who did not have the opportunity to continue in the program during the 2019–2020 academic year. We also excluded those who did continue as LAs in Winter 2020 or Spring 2020 but did not complete the survey post second quarter.
9 Levene’s test for equality of variances indicated that equal variances could not be assumed. A Welch’s t test is reported here.
10 To correct for multiple comparisons the alpha level was Bonferroni adjusted. Tests where p < 0.01 were considered statistically significant.
ACKNOWLEDGMENTS
The research team would like to thank all the instructors and teaching assistants who have supported the Learning Assistant Program at UCLA and Casey Shapiro at the UCLA Center for the Advancement of Teaching for facilitating access to registrar data.