How Four Scientists Integrate Thermodynamic and Kinetic Theory, Context, Analogies, and Methods in Protein-Folding and Dynamics Research: Implications for Biochemistry Instruction
Abstract
To keep biochemistry instruction current and relevant, it is crucial to expose students to cutting-edge scientific research and how experts reason about processes governed by thermodynamics and kinetics such as protein folding and dynamics. This study focuses on how experts explain their research into this topic with the intention of informing instruction. Previous research has modeled how expert biologists incorporate research methods, social or biological context, and analogies when they talk about their research on mechanisms. We used this model as a guiding framework to collect and analyze interview data from four experts. The similarities and differences that emerged from analysis indicate that all experts integrated theoretical knowledge with their research context, methods, and analogies when they explained how phenomena operate, in particular by mapping phenomena to mathematical models; they explored different processes depending on their explanatory aims, but readily transitioned between different perspectives and explanatory models; and they explained thermodynamic and kinetic concepts of relevance to protein folding in different ways that aligned with their particular research methods. We discuss how these findings have important implications for teaching and future educational research.
INTRODUCTION
Recent calls for educational reform in the life sciences have repeatedly encouraged a greater focus on scientific competencies, including the modeling and analysis of complex systems, and the use of analytical and scientific reasoning in ways that are authentic and relevant to current research (Howard Hughes Medical Institute and Association of American Medical Colleges [HHMI–AAMC], 2009; American Association for the Advancement of Science, 2011). This includes a call for greater integration of the physical and mathematical sciences into life sciences like biochemistry to support a deeper student understanding of fundamental scientific principles (National Research Council, 2003; HHMI–AAMC, 2009). In this way, students can develop the competence to address some of the many cutting-edge research questions pursued in the industrial, pharmaceutical, and medical fields that require application of biochemical knowledge and research methods to processes governed by thermodynamic and kinetic principles.
Toward partially addressing these calls for reform, the purpose of the present study was to investigate how selected experts, working at the cutting edge of protein folding and dynamics, use thermodynamics and kinetics with analytical and scientific reasoning and modeling to explain their research. This will, in turn, permit us to pursue our longer-term goal of using such expert data to inform the development of teaching activities in this cognitively demanding but crucial area of undergraduate biochemistry that is central to our understanding of many areas of biochemistry in general. Indeed, in so doing, we would hope that we would go a long way toward addressing the various well-documented conceptual difficulties that students exhibit with thermodynamics and kinetics in biochemistry (e.g., Sears et al., 2007; Wolfson et al., 2014), let alone other science contexts such as chemistry (see reviews by Bain et al., 2014; Bain and Towns, 2016), physics (e.g., Dreyfus et al., 2012, 2013), and engineering (e.g., Meltzer, 2007; Haglund et al., 2015). In the case of biochemistry, the thermodynamics and kinetics of complex, dynamic biochemical processes tend to be difficult for students to understand, a situation that can be exacerbated by the often confusing symbolic language, mathematical descriptions or models, and information-rich visualizations used to represent such processes (e.g., Liu et al., 2016). Thus, we believe that it is crucial to characterize how practicing scientists integrate theoretical and experimental knowledge of biochemical processes, like protein folding and dynamics, as only then will we be better prepared to help students master this complex topic, which is both an integral part of modern undergraduate biochemistry curricula and relevant to current research.
There are five major philosophical models of scientific explanations relevant to research and practice in science education (Braaten and Windschitl, 2011), but for the purposes of this study we broadly define “explanation” to include descriptions of observable phenomena; theoretical accounts of how phenomena progress according to any of the philosophical models; and/or the process of clarifying ideas, reasoning, and findings regarding a phenomenon (Achinstein, 1983; Salmon, 1989; Knorr-Cetina, 1999). Some suggest that the model of scientific explanation that is most appropriate depends on the purpose(s) of an investigation and its explanatory aims (Van Fraassen, 1980; Craver, 2006; Brigandt, 2010, 2013). For instance, a researcher may provide a statistical–probabilistic explanation relating the occurrence of a disease to trends in environmental factors in order to make health recommendations. Although the underlying cause is not mentioned, the aim of the investigation is a predictive tool, so a mathematical account “suffices” as an explanation. In the life sciences, historical reconstructions and examinations of scientific discourse have enhanced our understanding of scientific explanation, especially of mechanistic processes where explanations specifically seek to establish causal links between agents and events (Machamer et al., 2000; Darden, 2008; Bechtel and Richardson, 2010). A growing number of problems in the life sciences also address emergent phenomena—like protein folding and dynamics—where the overall behavior of the system emerges from underlying random processes rather than a regular sequential mechanistic process (Chi et al., 2012). We aim to further characterize how scientists explain phenomena and their study, with a long-term goal of using the findings to inform the development of more authentic undergraduate life science educational materials to foster the integration of theoretical and experimental knowledge, the understanding of biochemical research methods, and the application of physical principles in the life sciences.
The idea of using expert knowledge to inform student learning is key to the philosophy underpinning this study. Not only is scientific research the primary source of scientific knowledge, but given the sophisticated nature of scientific problems, the study of expert scientific thinking can offer valuable insight into the higher-order cognitive processes educators desire to develop in their students. Research has, for example, shown that scientists employ distant analogies as explanatory devices (Dunbar, 2000) and that analogical reasoning is a crucial cognitive skill for expert biochemists, likely because biochemistry depends heavily on understanding the abstract world of molecular structures and processes (Anderson and Schönborn, 2008; Schönborn and Anderson, 2008, 2009). Previous studies have used information gleaned from the study of expert knowledge and reasoning practices to develop classroom activities, resources, and/or guidelines for connecting levels of biological organization (Van Mil et al., 2013, 2016), developing representational competence in chemistry (Kozma and Russell, 1997), and supporting students in monitoring their explanations of biological mechanisms (Trujillo et al., 2016a). Trujillo et al. (2015, 2016a,b) provide a detailed example of how knowledge from case studies of expert scientists can be brought into the classroom. Recognizing that science educators would benefit from a clear model of how biologists explain cellular and molecular mechanisms, Trujillo et al. (2015) asked several expert biologists to explain sequential causal mechanisms relevant to their research. They found that those scientists consistently interwove discussion of research methods (M), analogies (A), social or biological context (C), and descriptions of how (H) a phenomenon operates in their explanations of molecular mechanisms and used these themes to develop the MACH model of mechanistic explanations (Trujillo et al., 2015). An iterative design-based process was then used to adapt, test, and modify the MACH model to improve its function as an educational tool to help students construct explanations of biological mechanisms (Trujillo et al., 2016a,b).
Although the MACH model helped students identify and incorporate its constituent components in explanations of mechanisms, Trujillo et al. (2016a) noted that students struggled to make connections between the MACH components and frequently overlooked research methods. The original MACH model does not describe how the components connect, or whether there is any pattern or sequence to their use. Driven by the overarching research question “How do experts explain their research related to protein folding and dynamics?,” the present study used the MACH model as a guiding framework for data collection and analysis. The similarities and differences that emerged from analysis of interviews with four experts led us to make the following claims:
All four experts integrated their theoretical knowledge and their research context, methods, and analogies when they explained how protein-folding phenomena operate (MAtCH model, Figure 1), in particular by mapping phenomena to theoretical mathematical models.
All four experts explored different processes depending on their explanatory aims, but readily transitioned between different perspectives and explanatory models.
All four experts explained thermodynamic and kinetic concepts of relevance to protein folding in different ways that aligned with their particular research methods.

FIGURE 1. The MAtCH Model. A simplified pattern of integration and connection between the MAtCH components was reflected in interviews of four experts who explained their protein-folding and dynamics research. The connections indicate a pattern, but the ways in which each connection was made differed for each scientist as highlighted in their research profiles. Please note that there is no specific direction or sequence, nor is there complete separation of components. The arrows are double-headed to reflect how the four experts moved back and forth between the components in their explanations, with the words near the arrows creating a sentence when read clockwise. This was done to simplify the relationships between components without making the diagram overly complex.
On the basis of these claims, we propose a revised version of the MACH model that includes the central role of theoretical knowledge. We offer the MAtCH model as a framework that can be used to analyze expert practice and to inform instruction.
METHODS
Selection of Participants
A pool of expert participants from various science departments at a large midwestern public research university were chosen purposefully based on two criteria used for theoretical sampling (Patton, 2002). Participant selection involved analyzing experts’ research profiles to determine whether their current published research 1) is related to protein folding or dynamics and 2) considers kinetic and/or thermodynamic data. By protein folding and dynamics, we mean the physical processes by which a protein changes its three-dimensional structure, including both global (whole-structure) and local (single-atom and partial-structure) deviations in position over time. Once identified, the participants (N = 4) were approached and asked to participate in an approximately hourlong, semistructured interview about their research. These participants will hereafter be referred to as “experts” or “expert scientists,” and pseudonyms will be used to protect their identities. The current research was performed under the approval of the Purdue University Institutional Review Board (protocol #1511016694).
Development and Description of Interview Protocol
The MACH model (Trujillo et al., 2015) was used to structure the interview protocol to focus on aspects previously identified as prevalent in experts’ explanations. In the MACH model, M is operationally defined as the methods of research, including the experimental procedures, techniques, or instruments used to generate data that inform the explanation; A refers to the analogies that help make sense of the mechanism, including formal analogies, representations, and/or narratives; C encompasses the social or biological context that connects the explanation to an important situation in which it can be applied; and H describes how the entities of the phenomenon interact to produce changes of state, activities, and spatial and temporal organization involved with understanding how the phenomenon operates. With this guiding framework, the interview protocol was separated into artificial “phases” that began with a general question regarding the experts’ research but then focused on probing the context and experimental methods used by these experts. Several probes were designed to ask experts whether and how they thought about specific thermodynamic concepts typically covered in undergraduate chemistry (e.g., entropy, free energy). As representations are also an integral part of scientific work and communication (e.g., Kozma, 2003), the interview also prompted participants to draw or show any representations they felt would be useful to gain additional insight into their mental models.
The initial interview protocol was piloted with graduate students who were members of the research labs run by potential research experts. Pilot interviews were audio/video-recorded, and a record of protocol modifications with evidence and reasoning for each modification was updated after each pilot interview. This process allowed the interviewer (K.A.J.) to test, and if necessary improve, various phrasing and to become more familiar with the interview protocol. For the main portion of the final interview protocol, the participants were asked to explain their research as they would to a colleague or a scientist in a related or similar field. At the end of the interview, with considerations of future educational activities in mind, participants were also asked to explain their research and protein folding in general as they would to a junior- or senior-level undergraduate student. Both types of data were collected to obtain a fuller characterization of these experts’ explanations of protein folding and dynamics, including accessing any potential pedagogical content knowledge. The purpose and methods of the study were explained to the experts before their participation in the study. Semistructured interviews were employed, as they allow an interviewer to explore individuals’ ideas at great depth and to probe for additional details or clarifications in order to come to a shared understanding, just as might happen in a conversation between two investigators.
Data Processing and Analysis
Interviews lasted between 1 and 1.5 hours and were audio/video-recorded. As expert use of representations was of interest, the production of representations or use of any computer-based representations was also video-recorded. Interviews were transcribed verbatim, and then portions of the text were aligned with provided representations by reviewing the video recordings. All drawing steps during the production of representations, gestures indicating parts of representations, and captured air gestures were described and inserted into the interview transcript. In this paper, only verbal data and a sample of the representations are examined. Gestures will be the target of future work. Interview transcripts were inductively analyzed (Lincoln and Guba, 1985, p. 203) to identify common concepts, representational modes, and analogies. The first round of analysis of the interview transcripts produced a master list of quotations that contained references to general concepts, and these were then sorted into a number of emerging categories. As the category descriptions crystallized, categories with fewer quotations/excerpts were removed or merged into other larger categories. Representations were analyzed to describe all the modes of representation used by the experts. Interviews were then analyzed for analogies, which were similarly sorted into emerging categories and then aligned with the previously identified concept categories. This process resulted in the identification of the unique ways these four experts think about thermodynamic and kinetic concepts given their research goals and methods (claim 3), as well as similarities in how they applied knowledge of scientific theories to their research (claim 1). Several excerpts and representations from the interview transcripts were selected to create “expert research profiles” to showcase the unique way each expert approached his or her research. The excerpts were coded with the MACH components, using the operational definitions set forth by Trujillo et al. (2015) described above in the Introduction. As an example, if the expert referenced the use of an experimental procedure, technique, instrument, or data, this was coded as “M.” Initial case analyses were sent to the respective experts to check whether their thoughts were represented accurately. Two of the participants (John and Gertrude) responded, and sentences were revised per their suggestions. A constant comparison method in combination with MACH coding allowed us to characterize similarities and differences in how the four experts transitioned between the MACH components and their theoretical knowledge (claim 1), and how their research goals and methods influenced their explanations (claims 2 and 3). The patterns that emerged from this process are described in the Results and Discussion section.
RESULTS AND DISCUSSION
Analysis of the interviews revealed that the MACH model components feature prominently in all four expert explanations, with experts frequently connecting and integrating the components. Furthermore, each expert’s explanation revealed clear connections between the MACH components and his or her knowledge of scientific theories. The amount of integration between the MACH components and theory made it difficult to organize the interview data in an easily understandable sequence. It became evident during analysis that all four experts integrated research context, methods, analogies, and how the phenomenon operates with their theoretical knowledge when explaining their research projects (claim 1). All four cases demonstrate this complex integration of components, but a general pattern of connections between the MACH components and theoretical knowledge emerged from the data. This pattern led us to propose a modified MACH model, or MAtCH model (Figure 1), which incorporates a new component, “theory.” By “theory,” we refer to the experts’ knowledge of overarching scientific explanations and models (e.g., collision theory or mathematical models of reaction kinetics) used by these experts when talking about their research. We situated the theory component at the center of the MAtCH model, because theoretical knowledge underpinned each of the MACH components and was used by the experts to mediate between the components. As the reader will see, the experts’ use of theoretical knowledge was often implicit or tacit in their explanations, but at other times they made it explicit. We have left the “t” in lowercase to emphasize the foundational role of theoretical knowledge in each of these components and explanations. For reader convenience, we first present a diagram of the MAtCH model (Figure 1), after which we use our analysis to illustrate how the data support its structure.
The structure of the MAtCH model will be used to introduce each of the expert research profiles, starting from their research context (C) and moving clockwise to first describe the entities they consider (H) and the methods by which they are measured (M) in their efforts to develop narrative or representational models (A) of a phenomenon. Although the research described in this paper could be considered very complex, we believe that following the order of the MAtCH model in Figure 1 allows us to make sense of these experts’ explanations. In the same way that students might use the MAtCH model to follow a simplified story of complex research, this model is used here to guide a description of each scientists’ research project while maintaining the connections between the MACH components and the theoretical knowledge the scientists used. For enhanced readability, the original MACH components will be indicated with the appropriate letter in parentheses in the analysis.
Throughout our analysis, we will also highlight the different ways in which the experts demonstrate the inseparability of the components of the MAtCH model in explanations of their research. We will indicate where the experts use knowledge of scientific theories and models (the “t” in MAtCH) to mediate between the MACH components, particularly by mapping phenomena to mathematical models. By this, we mean the way these experts interpret symbols, theoretical concepts (often represented symbolically), or formulas (A) through knowledge of physical systems such that they represent entities, states, processes, and/or measurable variables (H/M). Furthermore, we will use these four cases to illustrate how the experts explain thermodynamic and kinetic concepts in different ways closely aligned with the research methods they employ (claim 3). In this section, we present all four cases separately. The last two cases (Gertrude and William) are summarized, and full analyses can be found in the Supplemental Material. We then return to our three main claims in the Summary and Conclusions section, where we briefly compare the experts’ explanations, reflecting on their similarities (claim 1) and differences (claims 2 and 3).
Beaker Elucidates Enzyme Mechanisms
Beaker and his research group focus on how enzymes recognize substrates in order to design drugs and enzymes (C). One of their broad aims is to understand how a protein recognizes and catalyzes a reaction with a substrate (H). Because their focus is on understanding mechanisms (H), they collect data on structure and structural movement through techniques like x-ray crystallography, site-directed mutagenesis, and stroboscopic methods (M). These data (M) are used to map out the positions and movements of specific amino acid residues or protein domains in the active site along a reaction trajectory to propose a mechanism (H/A). In his discussion, Beaker focuses mainly on structural relations like proximity, orientation, and angle (H), consistently using theoretical knowledge of mathematical models of reaction kinetics, steric effects, and interactions to interpret data (M) and to explain the organization and activities of entities in the proposed mechanism (H/A). Beaker’s first excerpt in Figure 2a showcases how he uses theoretical knowledge to mediate between the H and M components in the MAtCH model to propose a mechanism via a narrative (A). We can also see how Beaker assigns meaning to mathematical models and symbols (A) during this process.
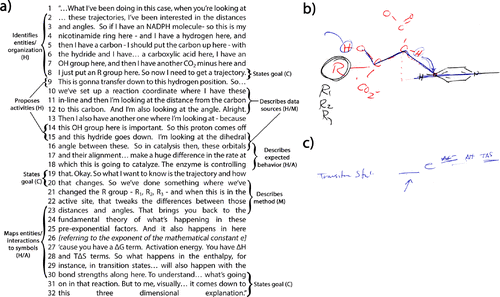
FIGURE 2. Beaker draws and explains the structural data he collects and interprets to determine reaction mechanisms. Beaker first constructs a representation of a protein active site with several residues (drawn in red) and a ligand (NADPH, black ring to the right) pictured in b. The line bisecting the ring of the NADPH molecule serves as a reaction coordinate. In a, Beaker mentions his research goal is to understand the trajectory of a reaction, and this remains implicit as he discusses research methods until it is mentioned again. He describes some of the activities that will take place along that trajectory, for example, circling two hydrogens in b in blue and using a line or arrow to show their movement. Beaker describes the types of data he will collect so that he can model the reaction trajectory, such as the dihedral angle indicated in b by the blue line tracing from the carbon on the nicotinamide ring to the carbon with the hydroxyl group or distances indicated by the dotted red lines. He uses theoretical knowledge of orbital alignment and reaction kinetics to support his use of research methods focused on determining angles and distances between the entities in b. Beaker also explains how the modification of R groups (black R is changed to R1, R2, etc., at the left side of b) will affect those distances and angles, and thus the rate of catalysis, which will enable him to better model the reaction trajectory. He explicitly relates all of these data back to theoretical knowledge and mathematical models of kinetics and thermodynamics, represented by formulas such as the one in c. He explains how distance and angle are included in pre-exponential factors (the underlined area indicated by an arrow in c), and how enthalpy (ΔH) and entropy (ΔS) are included as factors that affect activation energy in the exponent of e.
In his excerpt, Beaker starts by describing the organization of residues in the active site and the NADPH molecule (H, lines 2–8). He represents each of these physical entities and their organization in a drawing (A, Figure 2b). Then Beaker connects the H and M components of the MAtCH model as he describes what he measures about these particular entities (i.e., distance, angle; H/M, lines 10–12, 15–16). At this point, Beaker enters a cycle wherein he uses his theoretical knowledge to constantly mediate among the H, M, and A components of the MAtCH model in his efforts to understand the reaction mechanism (C, lines 8, 19–20). In his narrative (A), he proposes activities for some of the entities (H, lines 9, 14–15) and interprets data (M) in light of his theoretical knowledge about orbitals, reaction kinetics, and the importance of alignment to make a general claim regarding how he expects the system will behave (H/A, lines 16–18). After making this claim, Beaker restates what he wants to measure about the system (H) and describes a specific R group modification method for doing so (M, lines 20–23). As stated elsewhere in his interview, these data will allow him to construct a model of the active site and the reaction trajectory (H/A), furthering his understanding of the reaction mechanism (C, lines 30–32). As part of this cycle, Beaker uses his theoretical knowledge to explicitly connect the measurable and molecular worlds through the interpretation of mathematical models of reaction kinetics and thermodynamics as represented by formulas (A, lines 23–30). He does this by assigning meaning to the mathematical models by mapping entities and interactions (H) to particular symbols (A; e.g., lines 23–25; see Figure 2c, where alignment information is represented in pre-exponential factors).
Although Beaker mentions thermodynamic quantities in the excerpt, as a result of his focus on elucidating mechanism, he does not assign much significance to thermodynamic values. He states later that this is because they only indicate that something has happened, but not what or how. Therefore, it seems appropriate that Beaker focuses on collecting data (M) that will inform causal mechanistic explanations, and he thinks about the theoretical concepts of enthalpy and free energy in ways important to mechanisms by considering bond and interaction strengths (H). We can see evidence for this at the end of the excerpt (lines 21–23) as well as elsewhere in the interview when Beaker uses a dose of ibuprofen for treating a headache as a formal analogy (A) to explain the difference in ∆G values of different states (see the Supplemental Material).
Throughout his interview, Beaker consistently makes similar connections between theoretical thermodynamic and kinetic concepts and the interactions of entities (H). He does this by transitioning between narrative about generic models based on his knowledge of scientific theories and mathematical models, and more specific models (A) of interacting entities and their organization in a system (H). One such example is found in the next excerpt we discuss. This particular excerpt was chosen because Beaker devoted a significant amount of his discussion to the importance of spatial organization (H) and reaction kinetics (M) in elucidating enzyme mechanisms (C). To contextualize this excerpt, Beaker was claiming that there are very few examples of how enzymes work in detail, that is, their motions, distances, and angles along a reaction trajectory (H/M). In his opinion, this is partly because enzyme mechanisms have typically been studied using indirect methods (M) and partly because scientists over the years have reinterpreted and “rediscovered” the original model Linus Pauling proposed (Pauling, 1946)—that enzymes work by binding to the reaction transition state. In the excerpt in Figure 3a, Beaker essentially makes an argument for the concepts of proximity, orientation, and complementary binding (H) underlying Pauling’s original model through the use of a representation and narrative of a hypothetical two-substrate reaction mechanism (A).

FIGURE 3. In a, Beaker explains rate enhancement in an enzyme active site. Beaker begins by citing the most basic conditions for a reaction according to theory: bringing two reactants, like A and B in the blue circles in b, into proximity. Using a generic form of a rate law shown in c, Beaker assumes 1 M and 55 M concentrations for reactants A and B to illustrate that bringing reactants into close proximity provides a rate enhancement that is negligible in comparison to data for enzymes. Beaker then uses the rate law in c to estimate rate enhancement after the addition of multiple other reagents (red circles H, OH, and M in b) at 55 M each to again illustrate that rate enhancement is a negligible 105 or 106 in comparison with enzymatic data at 1012. Thus, enzyme rate enhancement cannot be due to concentration alone. Using theoretical knowledge of factors that increase reaction rate, like probability, proximity, and orientation represented by mathematical formulas elsewhere (e.g., Figure 2c), he proposes a model in which the cartoon enzyme in b uses its upper arm to bring the reactants together and appropriately orient them (where the darkened blue triangles on reactants A and B in b represent the structural parts that must be aligned). Beaker uses a bar magnet analogy to explain how the cartoon enzyme uses electrostatic forces to aid the alignment of the reactants. Thus, he uses this excerpt to explain that enzymatic rate enhancement is ultimately the result of purposeful spatial organization by the enzyme leading to specific orientations and interactions.
In this excerpt, Beaker once again uses his theoretical knowledge to mediate between the H, M, and A components of the MAtCH model as part of a cycle. He begins by connecting the H and A components as he describes the organization of a variety of entities (H, lines 5–8) in a hypothetical two-substrate reaction mechanism (A; see also Figure 3b). He then uses his theoretical knowledge of mathematical models of reaction kinetics, represented by a rate law equation (A; see Figure 3c), to model this hypothetical reaction and to illustrate that concentration alone cannot account for the observed enhancement of enzymatic rate from 105 or 106 to 1012. Because data on observed rates (M) cannot be mapped onto such a simple mathematical model, the equation is insufficient to represent reality (A, lines 8–11). Beaker then uses theoretical knowledge to propose that if, however, the function of the model enzyme is to bring the appropriate substrates into proximity with the appropriate orientation/alignment in order to react (H), as suggested by transition-state theory and mathematical models like that represented by the Arrhenius equation (A), then he has a reasonable model of the system (H/A, lines 11–22). In this process, Beaker again uses theoretical knowledge to connect the measurable and molecular worlds, namely by assigning meaning to mathematical models of reaction kinetics by mapping entities and interactions (H) to equations and symbols (A). There are additional instances in his interview when Beaker makes similar connections. For example, Beaker indicates that he always thinks about the Henderson-Hasselbalch equation (A) and pKa values when considering an active site, because the ionization state, and thus the structure, of certain residues can differ (H) depending on the pH (i.e., entities have variable properties). Beaker also uses theoretical knowledge of mathematical models to relate the energetics of steric hindrance, interaction strength, and structure (H) with functionality (C). For example, by determining the actual distance between residues (M), he can use mathematical models of electrostatics (A) to reason about why the system behaves a certain way (H). All of the these considerations provide him with rich data that he can use to inform enzyme and drug design. We see in the next case that John similarly assigns meaning to mathematical models of reaction kinetics to think about both his research methods and how protein-folding phenomena operate.
John Investigates Protein Stability with Proteolysis Kinetics
John is interested in how globular proteins lose their structure in order to understand more about protein rigidity and longevity and to engineer more robust proteins for function in harsher conditions or for longer shelf-lives (C). John’s research group investigates how partially unfolded nonnative protein conformations that are in equilibrium with native proteins lose their structure (H). In his interview, John focuses on the use of proteolysis kinetics (M) to measure how often a protein loses its structure (H). If, for example, the addition of a mutation changes which region of a protein is digested or alters the rate of proteolysis (M), this suggests that the mutation has changed the relative stability of the partially unfolded forms of the protein (H). From this kinetic data (M), John derives change in free energy values to estimate the relative stabilities of folded and partially unfolded proteins (H) and maps such results onto different representations (A). In the excerpt in Figure 4a, John provides a simplified narrative (A) of his proteolysis kinetics method, in which we can see how he uses kinetic theory to integrate the H and M components of the MAtCH against the backdrop of several related representations (A; Figure 4b). We also see evidence of how John assigns meaning to mathematical models by linking symbols (A) to entities or measurable variables (H) and of his unique understanding of thermodynamic and kinetic concepts.

FIGURE 4. John outlines his method to determine the energy difference between folded and partially unfolded proteins. John first describes two processes undergone by proteins in his proteolysis kinetics method: the folding–unfolding equilibrium of native folded protein (N) to cleavable partially unfolded protein (C), and the proteolysis of the cleavable form. He represents these processes with cartoons and several constants at the top of b. The size of the equilibrium arrows represents the relative populations of each form, while the unidirectional arrow represents the irreversible proteolysis reaction. John explains that they monitor the proteolysis reaction that produces fragments (later measured by gel electrophoresis) and use these data to determine Kunf, the equilibrium constant for unfolding; kint, the intrinsic rate constant for proteolysis; and then the product of these two variables, which represents the overall proteolysis rate, kp. kint is approximated using the unstructured peptide or a generic peptide substrate if the sequence is unknown. Toward the end of the excerpt in a, John provides an example of how digestion rates relate to relative values of variables, providing examples of small Kunf values on the right (10−5 and 10−6) of b. The series of mathematical formulas in b shows how John uses theoretical knowledge to relate kinetics and kinetic data to thermodynamics to calculate ∆G and to numerically describe the susceptibility/stability of the protein.
In the excerpt in Figure 4, John uses his theoretical knowledge of kinetics and equilibrium to repeatedly cycle through the H, M, and A components of the MAtCH model. He seamlessly moves between a description of the interacting entities and their activities in his method (H/M), his data measuring that process (M), and a mathematical model of the system as represented through a series of equations (A; see Figure 4b). John first draws connections between the H and M components. He describes the dynamic equilibrium that naturally exists between the native and nonnative conformations of a protein (an entity with variable states, H, lines 1–4, 7–10), and intersperses this description with a discussion of how proteolysis occurs (H/M, lines 4–5, 10–15) and how his method gives several kinetic values (M, lines 15–21). Each of these values represents a different process in the system (H/M, lines 15–16, 18–21; also see Figure 4b). We can see that John assigns meaning to mathematical models by using his theoretical knowledge of kinetics and equilibrium to map processes in the system (H) to particular symbols (A) and to connect their relative measured values (M) to what they imply about the susceptibility or stability of the protein (H, lines 21–26). This enables John to use these equations (A) to mediate between the interacting molecular entities of the folded–unfolded–digested protein system (H) and the measurable world of data (M). It is also significant to note that John closely intertwines kinetic and thermodynamic theoretical concepts during his explanation. This unique integration is critical to how John relates the variable states of protein molecules to the abstract idea of their relative stability (H). The excerpt in Figure 5a provides additional evidence of the unique way John does this.

FIGURE 5. John describes how he relates protein movement to free energy. John describes how a protein will “jiggle,” relating the concept of free energy to the time it takes for it to “jiggle” in or out of a particular conformation. He also states that the time a protein spends in a particular form and the frequency at which a protein changes form are representative of the difference in free energy between conformations. This difference determines the populations of the conformations. In an earlier part of the interview, John used the kinetic barrier diagram in b to similarly relate speed of protein folding to the concept of free energy. ∆G° represents the difference in free energy between the unfolded (U) and native (N) protein conformations as environmental conditions change. ∆G‡ canonically represents activation energy. For this diagram, John explains that the time it takes for the protein to fold (the kinetics), which he represents with an arrow over the top of the diagram, determines the height of the barrier (the energy difference). The excerpt in a and the picture in b serve as further evidence of how John closely intertwines kinetic and thermodynamic concepts in a way that aligns with his experimental methods.
Throughout his interview, John talks about how proteins “jiggle” or have a “jiggling time,” which he relates to free energy (A, lines 1–6, 13–14). To John, the frequency at which a protein loses its structure and/or its longevity in a particular form appear to be physical manifestations of free energy (lines 6–15). John also interweaves frequency, time, and relative population of protein conformations (lines 16–17 in this excerpt) with the concept of free energy through statements like “This is a rare conformation so its free energy is much higher,” or “How frequently that would happen… Is it one of one million? Or one of ten thousand?” This temporal way of thinking about free energy (A; see also Figure 5b) aligns with John’s use of proteolysis kinetics (M) to estimate ∆G values. It is not apparent whether John’s conception of free energy is influenced by the methods he uses or whether he chose those methods because of his understanding of thermodynamics and kinetics. Thus, the kinetic data that John collects allow him to better understand the energetics of partial unfolding in proteins in order to engineer improved proteins. In the following case, we see how Gertrude uses a fundamentally similar method to study the stability of protein drugs.
Gertrude Investigates Protein Drug Shelf-Life
Gertrude is interested in the physical and chemical modification processes undergone by lyophilized (i.e., freeze-dried) protein drugs in order to improve drug formulations and enhance shelf-life (C). These drug formulations include excipients, which are inactive substances that serve as vehicles for delivering drugs or other active ingredients. Her research group considers the extent to which protein drugs unfold and how they aggregate when they are unfolded or partially unfolded (H). The degree of unfolding is determined by hydrogen–deuterium exchange (HDX): lyophilized protein powders are exposed to deuterium vapor and the resulting peptide mass is measured with a mass spectrometer (M). These data are then used to create representations (A) reflecting deuterium incorporation, indicating what regions of the protein drugs remain protected during unfolding (H). Gertrude’s case provides a clear example of the presence and integration of the MACH model components and the implicit role of theory in her explanations (see the Supplemental Material for full analysis).
Gertrude makes distinct connections between the data collected (M), how they are represented (A), what entities and interactions are described in the system (H), and what that implies about functionality (C). As she cycles through components in her discussion, she explicitly and implicitly employs theoretical knowledge of protein structure, inter- and intramolecular interactions, and equilibrium. For example, she explains how an increase in mass via HDX (M) allows her to measure the exposure/protection of regions of protein structure (H), mapping data directly onto three-dimensional representations of protein structure (A). These data can then be correlated with a drug’s stability as a dry solid (C). Gertrude also examines the interactions of protein drugs and their organization in space and over time (H), employing her theoretical knowledge to suggest a hypothetical model (in narrative form) of what may occur in a protein–excipient system (H/A). During this process, she integrates knowledge of a suggested “hydrogen bond replacement theory” from her field and connects her hypothetical model of the protein–excipient system (H/A) to her research goal of predicting good excipients (C). Gertrude uses her theoretical knowledge to closely relate HDX (M) to the scale of unfolding and interactions between entities in the phenomenon (H) through a narrative story (A).
Gertrude also uses representations (A) as backdrops during her discussion. For example, her research group also investigates protein aggregation, because proteins that become partially unfolded after lyophilization have a tendency to form aggregates (H) when they are reconstituted and potentially cause immune responses in patients (C; e.g., see Ratanji et al., 2013). The kinetics and equilibria underlying the episodic incorporation of deuterium into the partially unfolded proteins are particularly important, as the amount of deuterium that is incorporated over time (M) reflects how fast residues become buried in the aggregated form and where residues are buried (i.e., the aggregation interface; H). As before, theoretical knowledge plays a critical role in this process by allowing Gertrude to mediate between the representation (A) of the measureable world of HDX data (M) and the molecular world of interacting entities (H). Gertrude’s research enables her to more quickly make inferences regarding which peptide drug formulations will have longer shelf-lives through the application of HDX methods. We can see in the following case how William’s efforts similarly aim to improve predictions but address an entirely different research problem.
William Simulates Protein Dynamics to Improve Drug Metabolism Prediction
William’s work focuses on incorporating protein dynamics into computational models (M/A) in order to improve predictions about where drug candidates are metabolized and by which enzymes, so as to aid the development of more metabolically stable drugs (C/H). Unlike the other experts interviewed, William’s goal is the development of a predictive method to model possible drug and protein movements and interactions (M/A), which is validated and trained using experimental site metabolism data (M). The end product of his research—a process incorporating a variety of techniques like molecular dynamics simulations, molecular docking, and statistical techniques (M/A)—can then be used to produce data of its own (M). By considering protein dynamics (which he defines as the trajectories of atoms and residues in a protein [H]), he can produce an ensemble of protein structures to represent the multitude of possible conformations and average them to suggest the most likely preferred conformation (M/A). This conformation can then be used in the simulated docking of drug candidates to make predictions (M/A). Because of his research goal, and the computer model-based nature of his research, the H, M, and A components are completely integrated in William’s discussion and his understanding of thermodynamics similarly appears to intertwine or align with his simulations (A). The MAtCH model allows us to make sense of this complexity by focusing on the connections (see the Supplemental Material for full analysis).
In his interview, William describes how the structural components of proteins might change their spatial organization to accommodate drug compounds (H). He argues that, because alternative structural states (i.e., dynamics) can affect the prediction of a compound’s distance in relation to the catalytic center (M/H), including dynamics in simulations (M/A) is critical to improving the predictive capabilities of current methods (C). William’s tacit use of theoretical knowledge allows him to productively mediate between the measurable world (M) and what it implies about the molecular world of (simulated) protein structures and their interactions (H/A). William’s discussion shows that he relates residue flexibility to protein dynamics and that he also has a unique way of assigning meaning to theoretical thermodynamic concepts. William’s understandings of enthalpy, entropy, and free energy appear to align with his simulations (M/A) and are mapped to entities, interactions, and states of a protein system (H). For example, he makes the concept of entropy tangible as “How much an object is moving. How dynamic it is…” (i.e., structural flexibility) and he connects it to temperature and the velocity of particles (H). He describes enthalpy as internal or potential energy but also associates it with the sum of interactions and interaction strength (H). William states that both entropy and enthalpy must be considered to determine the actual preferred state of the system and explains how, in his simulations (A), temperature can be “turn[ed] on” to allow protein dynamics (entropy), and the resulting different states have different kinds of interactions (enthalpy; H). William explains that, if protein dynamics are ignored, “you don’t have entropy, you’re not calculating ∆G’s,” and the result is incorrect predictions for ligand binding (M/A) and unreliable predictions about drug candidates (C).
Throughout his discussion, William assigns meaning to mathematical models by mapping entities, interactions, and variable states (H) to particular symbols in formulas and graphs (A). At one point, William discusses the difficulties his students seem to have interpreting data (M). He explains how, to him, a change in free energy on a graph (A) reflects underlying changes in structural movement and/or the formation of new interactions (H) in the simulation (A). It also indicates he must look at the simulated protein system (A) to interpret the possible structural cause (H/A) of the data (M). According to William, while producing a numerical or graphical output is doable for students in his lab, interpreting and making connections between the data (M) and the underlying (simulated) physical causes (H/A) are not as obvious. Thus, a combination of experimental and simulated data enables William to improve current methods used to predict the metabolism of drug candidates.
SUMMARY AND CONCLUSIONS
The present study explored how four scientists integrate thermodynamic and kinetic theories, analogies, and research goals and methods in explanations of research projects related to protein folding and dynamics. What differentiates our study from extant accounts of expert explanatory practices is that it compares how several experts understand their work in the context of their research goals and methods as they work on projects at the intersection of physical and biological sciences. Within this context, our study attends to the structure of these experts’ explanations, as well as the central and underlying role of thermodynamic and kinetic theories that are typically covered at the undergraduate level. Current research has begun to characterize components of explanations but does not examine how data from particular research contexts are incorporated as evidence with the intent to inform instruction. Four explanations of research projects were analyzed, ranging in context from enzyme mechanism elucidation (Beaker), to globular protein stability (John), to protein drug shelf-life (Gertrude), and protein dynamics simulations (William). From these data we make the following claims, which we briefly discuss below:
All four experts integrated their theoretical knowledge and their research context, methods, and analogies when they explained how protein-folding phenomena operate (MAtCH model, Figure 1), in particular by mapping phenomena to theoretical mathematical models.
All four experts explored different processes, depending on their explanatory aims, but readily transitioned between different perspectives and explanatory models.
All four experts explained thermodynamic and kinetic concepts of relevance to protein folding in different ways that aligned with their particular research methods.
Claim 1: All four experts integrated their theoretical knowledge and their research context, methods, and analogies when they explained how protein folding phenomena operate, in particular by mapping phenomena to theoretical mathematical models. Experts’ common integration of the MACH components and theoretical knowledge in their explanations led us to propose the MAtCH model (Figure 1). For the purpose of simplifying our data analysis, we attempted to separate the experts’ explanations into the individual components, though in reality there was no clear separation of these components, nor was there any specific sequence in which the components were used by each expert. We found that, by attempting to separate the experts’ explanations into the MACH components, we were able to track the complex connections between what they study (H), how they study it (M/A), why it is important (C), and the theoretical knowledge (t) underpinning the components according to how the experts mediated among them. Whereas the original MACH model identified components of expert explanations of cellular and molecular mechanisms, the MAtCH model provides a framework that can be used to recognize the role of theory in tying the components together in explanations of research. Overall, we found that these experts address the social or biological importance of their research in their opening statements and do not appear to immediately move from statements of research goals (C) to experimental methods (M), but rather do this by way of interacting entities (H) or models of entities involved (A). In constructing their explanations, the experts consistently use knowledge of scientific theories and mathematical models to cycle between the how, methods, and analogy components, integrating that knowledge with experimental data (M) and various models of reality in narrative and representational forms (A) to discuss the interacting entities of the phenomenon (H). For example, both John’s and Gertrude’s research methods rely on knowledge of mathematical models of kinetics and equilibrium, and this knowledge allows them to relate their methods (M) and representations of data (M/A) to specific interacting entities (H) involved in those processes. Theoretical knowledge and mathematical models in particular are key to how these experts mediate between a molecular-level description of a phenomenon (H) and the measurable world of data and data representations (M/A). To do this, the experts map entities, states, interactions, and processes (H) to formulas (A) representing mathematical models via measurable variables (M). For instance, Beaker connects the collision of entities to variables in rate laws and the Arrhenius equation, whereas William connects particle movement and protein flexibility to entropy and temperature. As Schuchardt and Schunn (2016) suggest, and as our case studies support, it is the context behind the mathematical representation that determines whether it is seen as a model of a phenomenon or a calculated procedure. The integrated nature of the MAtCH components suggests that explaining how a phenomenon operates (H) in practice may be inseparable from how we measure it (M) and the theories (t), mathematical concepts, and analogies (A) we bring to bear on it (see also Boumans, 1999).
Claim 2: All four experts explored different processes depending on their explanatory aims, but readily transitioned between different perspectives and explanatory models. Analyzing the explanations according to the MAtCH model also helped us consider how scientists’ research goals influenced their methods and types of explanations. We found that, despite all four experts addressing research problems involving protein folding and dynamics, they did so in different ways and for different reasons. Differences in research goals (C) led the experts to explore different types of processes (H) and to collect data (M) for different explanatory aims (Brigandt, 2013). We found that the experts considered protein folding and dynamics from both emergent and sequential perspectives, depending on their research goals. Beaker, the only expert who was chiefly concerned with mechanism in our study (C), mainly focused on methods to observe and perturb a system in order to seek underlying cause–effect relationships (causal explanation) and describe the order of events in an enzyme mechanism (a sequential process). The other three experts—John, Gertrude, and William—focused their discussion on describing causal relationships in emergent processes (H) or methods (M) based on emergent processes, making inductions from trends in data (statistical–probabilistic explanation). The latter is a decidedly different research goal (C) from establishing causation. For example, John and Gertrude used proteolysis kinetics and HDX, respectively, to make inferences about structural stability. Seeking the underlying causes of events was not their predominant research goal, possibly because their projects focused on emergent processes, which cannot be reduced into sequences of subevents. While John, Gertrude, and William, like Beaker, described causal relationships among entities, properties, and interactions for emergent processes, they did so without suggesting a cause–effect chain of events. Instead, they described the actors (entities) and their roles (interactions) without an order to events, as one would expect in a narrative. They made references to multiple states of the system. Furthermore, all four experts had instances in which they transitioned between statistical–probabilistic and causal explanations, or between describing sequential and emergent processes as part of explaining their methods (M) or the phenomena (H) they study. We believe this highlights that these experts used and combined a variety of explanatory models; which model is employed in a particular instance depends on the nature of the process being explained and the explanatory aims of the research. John, for example, offered a sequential–causal explanation to describe his proteolysis kinetics method (M), but his description of the equilibrium between folded and cleavable forms of a protein (H) reflected the “collective summing” characteristic of emergent processes (Chi et al., 2012). In regard to his research goals (C), he focused on what kinetic data (M) imply about protein stability (H) rather than on establishing causation, which is characteristic of a statistical–probabilistic explanation (Braaten and Windschitl, 2011). As another illustration, Beaker referenced diffusion and collision frequency (emergent processes) in determining reaction rate, but such processes are secondary to the importance of proximity and orientation (H) in determining a mechanism of enzyme catalysis (sequential process). In a sense, the emergent processes operated at a hierarchical level (Machamer et al., 2000) below where Beaker’s research goals (C) and methods (M) were concerned, but he pulled them into his explanation where appropriate.
Talanquer (personal communication) offers another perspective on this, suggesting that there are three levels to mechanistic explanation: the macroscopic–phenomenological, particulate–mechanistic, and particulate–structural, and it is possible for explanations to be hybrids of more than one level. From the perspective of the MAtCH model, the explanations here suggest something similar: experts interweave discussion of measurable (M) system behavior (macroscopic–phenomenological) with discussion of collisions and forces (particulate–mechanistic) and interactions or properties resulting from structure (particulate–structural; H), and do so for both sequential and emergent processes. For example, in one of his excerpts, Beaker explained how the (measurable) enhancement of a reaction rate by an enzyme (macroscopic–phenomenological) cannot be explained entirely by frequency of collisions (particulate–mechanistic) but must consider how structures in the active site orient reactants in close proximity (particulate–structural). The properties of entities, or the “particulate–structural level,” were repeatedly highlighted in these experts’ explanations as they used structure or structural properties to make predictions even when they did not have a particular mechanism in mind, regardless of whether they were considering the phenomenon from an emergent or a sequential perspective. For example, William and Beaker discussed the significance of entities’ properties (e.g., charged, hydrophobic) on interactions in the system. Whether they focused on emergent or sequential processes, structure appears to be a powerful predictive tool for these experts.
Claim 3: The four experts explained thermodynamic and kinetic concepts of relevance to protein folding in different ways that were aligned with their different research methods. The data also revealed that the experts explained thermodynamic and kinetic concepts in multiple, functionally useful ways, closely aligned with their research methods. Beaker remarked that thermodynamic data do not provide mechanistic information about how something occurred, only that something may have changed, so he devotes less attention to thermodynamics. Even so, Beaker’s discussions of entropy and enthalpy reflect a focus on structure and mechanism: enthalpy is connected to interactions, and entropy is connected to the movement of molecules from a more organized or restricted state to one of greater disorder (e.g., the displacement of water from an active site). John’s aim was to measure a thermodynamic property (free energy), but he used kinetics-based methods that led him to consider free energy and stability from a temporal perspective. John was interweaving frequency, time, and population by discussing the frequency at which a protein “jiggles” into partially unfolded conformations or its longevity in a particular conformation. He avoided breaking free energy into enthalpy and entropy components, because he considered it too difficult to compare their magnitudes. On the other hand, William looked at entropy and enthalpy separately in developing simulations. He connected enthalpy to interactions and made entropy tangible as flexibility or particle movement, which can be “turned on” through temperature. Given the practical and descriptive orientation of her research, Gertrude devoted little attention to thermodynamic variables but directly connected the idea of stability to measurements of mass (i.e., amount of deuterium incorporation) and rigidity to the extent of hydrogen-bonding interactions. This relates to how she represented her HDX data. Gertrude, John, and William’s explanations of thermodynamic concepts particularly show how they integrated theoretical knowledge with their research methods and data representations so intricately that they cannot be isolated from one another. We believe this further supports the integrated nature of the MAtCH components. It also suggests that theoretical concepts of significance to the study of protein folding and dynamics can be explained in many different ways and with a basis in authentic research methods. Rather than a single definition of entropy or free energy, there are multiple practical definitions, each of which emphasizes different aspects of a phenomenon and varies in degree of usefulness depending on the research context. This aligns with Brigandt’s (2013) remark that scientific models and explanations—and we add analogies—are not all-purpose tools but serve specific purposes and explanatory aims. These experts provided other verbal and visual analogies that will be the focus of later studies.
Limitations
As with any qualitative study, there are important limitations to consider. First, the original intention was for participants in this study to address the interviewer as a colleague in a similar or related field, but this was difficult, and the authors acknowledge that the explanations provided to the interviewer were directed more at the level of a graduate student with some knowledge of the field. However, this was actually advantageous, as the semistructured nature of the interviews still allowed the participants and interviewer to develop a mutual understanding of the research at a level that shows application of thermodynamic and kinetic concepts students would learn in undergraduate science courses. This serves the long-term goal of this research. Furthermore, while these results only represent the ideas and work of a small sample of four experts currently conducting research related to protein folding and dynamics and therefore cannot be generalized across all experts in this area, the results do provide an opportunity for a deeper analysis of expert explanation than would be obtainable through a larger sample size study. The authors would argue that, while the specifics would change from research project to research project, it is probably commonplace for experts to integrate components of explanations (as per Figure 1) and shift between types of explanatory approaches and perspectives when appropriate. Similarly, while we cannot claim from this study that the ways these experts think about thermodynamic and kinetic concepts are shared by other individuals working on similar research projects, the findings do indicate that experts’ ideas may align with their research methods.
Implications for Instruction
Given the previously stated pedagogical importance of protein folding and dynamics to the undergraduate curriculum and current research, we suggest that these findings can inform the following:
Development of educational materials to support students’ ability to use research methods, data, and theoretical knowledge to explain protein-folding phenomena;
Use of mathematical models in biochemistry courses; and
Examples or case studies based on the expert research described in this paper, including a range of ways to conceptualize thermodynamic and kinetic concepts used in protein-folding and dynamics research.
These pedagogical implications are discussed in greater detail in the following paragraphs.
First, findings can inform the development of educational materials to support students’ ability to use research methods, data, and theoretical knowledge to explain protein-folding phenomena. The cases here suggest that the blending of MACH components guided by theoretical knowledge (i.e., MAtCH) is critical to research projects of social impact. We believe that the findings of this study highlight the importance of bringing both research contexts (C) and methods (M) into the science classroom to provide a more holistic and practical understanding of natural phenomena and the process by which they are understood. Students are often not prompted to consider or integrate the MAtCH components in their course work. Although the original MACH model was used to help undergraduates think about components of mechanistic explanations (Trujillo et al., 2015, 2016a), students still struggled to make connections between the MACH components—especially between the phenomenon (H) and how it is measured (M)—which resulted in disjointed explanations (Trujillo et al., 2016a,b). While we did not investigate student learning in this study and therefore cannot make any claims regarding the use of the MAtCH model in the classroom, we found it was helpful for making sense of the complex interconnected components and theoretical knowledge important to complex cutting-edge research projects. Similarly, we believe that instructors can use the MAtCH model as a tool to design or modify curricula for life science courses to create contextualized content with activities and assessments structured to emphasize the MAtCH components and their connections. By using the MAtCH model to systematically check for the presence of components and connections, instructors can critique course objectives and materials based on expert practice, thus identifying strengths and limitations or gaps in coverage, so that they may make informed decisions regarding design and implementation to ensure that the curricula expose students to more authentic and practical science. By emphasizing the components and connections, our objective is to help students not only gain knowledge of procedures and data-processing techniques (M), but also to enable them to use underlying theoretical knowledge to develop models and representations of a system (A) and to discuss data (M) in terms of what they measure about the interacting entities of the system (H) as well as the social or biological implications (C). As an illustration, we employed the MAtCH model to briefly review and suggest possible modifications for three protein-folding and dynamics educational materials published this year (Helgren and Hagen, 2017; Lipchock et al., 2017; McLaughlin, 2017; see Supplemental Table S1). Lipchock et al. (2017), for example, provide a 10-week research-like laboratory module in which students use various techniques to explore the effect of mutagenesis on enzyme structure and function using protein tyrosine phosphatase 1B (PTP1B). Evaluation of the materials using MAtCH suggests a strength of the module is its in-depth discussion and use of different techniques (M) that involve or result in a variety of representations (A). However, the module does not explicitly help students interpret their data and/or data representations in terms of the interacting entities of the system (M/H, A/H). To address this, prompts like the following could be included in the module:
What information about PTP1B can be obtained from your stained gel? What cannot? (A/H)
Compare and contrast the methods used in this project with other methods for studying protein structure and dynamics. What can each of those methods tell you about the protein you are studying? What can they not tell you? (M/H)
Modified prompts like these, which elicit more integration of the MAtCH components, may enhance student learning by supporting meaningful interpretation of (multiple) representations, by scaffolding discussion of data in terms what they measure about a system so that they can be used to develop a model, and by directing students’ attention to the limitations of methods and representations, thereby supporting the development of a more authentic understanding of scientific practice. By including more opportunities for students to integrate MAtCH components (such as the M/H and A/H connections above) instructors can encourage students to think in ways that are more similar to experts in the field.
Our second implication concerns the instruction and use of mathematical models in biochemistry courses. Previous research has found that many students seem to engage with thermodynamic and kinetic formulas solely as algorithmic exercises (e.g., Carson and Watson, 2002; Hadfield and Wieman, 2010; Bektas¸li and Çakmakci, 2011). Students can demonstrate mathematical proficiency without conceptual understanding and often struggle to interpret physical meaning from mathematical expressions and/or to produce mathematical expressions from physical situations (e.g., Thompson et al., 2006; Hadfield and Wieman, 2010; Becker and Towns, 2012). As Bain et al. (2014) point out, if educators expect students to develop an understanding of thermodynamics through mathematical relationships and representations, they must be taught what those mathematical concepts mean in a thermodynamics context. Too often mathematics in science becomes a summary of data or a calculated procedure that is manipulated, with little link to scientific phenomena or processes (Schuchardt and Schunn, 2016). The findings here underscore the importance of mapping entities, interactions, and processes to mathematical formulas and symbols in scientific practice. The MAtCH model demonstrates that, to address current scientific research problems, one must be able to use mathematical models to mediate between methods, data, and ever-developing models of interacting entities in a phenomenon. The findings provide several examples of how mathematical models related to thermodynamics and kinetics serve as key theoretical tools for interpreting data and reasoning about complex processes. We believe the MAtCH model can be used by instructors to reflect on how they might better connect mathematical models to scientific phenomena and research methods in the life sciences.
The third pedagogical implication of this study is a broader range of ways for educators to conceptualize thermodynamic and kinetic concepts used in protein-folding and dynamics research, including how they may be integrated with each other and with research methods. We believe that, if educators intend to support students in understanding scientific practice and knowledge, it is necessary to develop educational materials that scaffold the integration of research methods and conceptual knowledge in the ways that expert scientists do. In the traditional biochemistry classroom, thermodynamics and kinetics are taught separately, with little emphasis on experimental methods and significant focus on calculation and interpretation of various plots (e.g., Lineweaver-Burk plots). Contrary to this, the experts in our study used a variety of analogies and employed unique descriptions of theoretical concepts to explain their research. We believe our findings support the argument Haglund (2012) provides in his work on entropy: that instead of abandoning several distinct meanings for a single “scientifically correct” concept, educators should take into account “the perceptual embodied nature of our cognition [and] the pragmatic, contextual circumstances in which any act of reasoning is performed.” He notes that different models can highlight different aspects of a phenomenon to create richer descriptions and allow for varying degrees of idealization within different knowledge traditions. Not only do the experts provide examples with language and analogies that at times seem hardly “scientific” at all—for example, using analogies like electrostatics as magnets and free energy as “jiggling,” which could be powerful tools for instructors—but the heterogeneity in these experts’ conceptions demonstrates that context and pragmatics have a notable influence on reasoning and explanation. The apparent alignment between these experts’ conceptions and their research methods indicates that research methods can directly influence the ways in which these scientists think about phenomena, implying that understanding research practice may be an important part of functional scientific knowledge. Therefore, it may be useful to incorporate several case studies based, for example, on the four experts’ research projects described in this study, in order to make the thermodynamics and kinetics of protein folding and dynamics more tangible to the learning of biochemistry.
Implications for Future Research
Frameworks to evaluate scientific explanations began, in part, with consideration of how expert scientists work and communicate, and it is critical to continue investigating how experts explain complex research projects and processes so that these can be better communicated to students. We identify at least two main avenues for future research. First, this study offers only a preliminary characterization of several experts’ explanatory practices connected to specific phenomena. Significantly more work is required to untangle the complexity inherent to explanations of scientific research projects in order to develop pedagogical strategies and materials that help students integrate course content with practice (e.g., understanding research methods or connecting experimental findings to processes governed by theories that students are learning in the classroom). A second major avenue for future research concerns the critical role of analogical models in scientific communication and reasoning. Past research has shown that the interpretation of models can be extremely difficult for students and can lead to a range of conceptual difficulties that impact learning, especially when students must interpret representations of theoretical concepts (Schönborn et al., 2002; Schönborn and Anderson, 2006). As with mathematical formulas, students can demonstrate competence at answering graph-related questions, but without understanding or referencing its meaning in the natural world (e.g., Bowen et al., 1999). By characterizing how experts use analogical models to explain protein folding and dynamics in a research context, such studies may inform the design of educational materials aimed at scaffolding the development of students’ explanatory skills in this cutting-edge area of biochemistry.
ACKNOWLEDGMENTS
We especially thank the pilot and expert participants of our study for their time and willingness to share with us their knowledge and insights into their research areas, as well as members of our VIBE research group for their contributions to the progress of the study. We also thank our reviewers for their excellent feedback, which we believe greatly contributed to the quality of our article. This work was partially supported by the ACE-Bio project (NSF grant 1346567) and the BASIL project (NSF grant 1503798). Any opinions, findings, and conclusions or recommendations expressed in this material are those of the authors and do not necessarily reflect the views of the National Science Foundation.