The Case for Biocalculus: Improving Student Understanding of the Utility Value of Mathematics to Biology and Affect toward Mathematics
Abstract
The next generation of life science professionals will require far more quantitative skills than prior generations. Calculus is important for understanding dynamical systems in biology and, therefore, is often a required course for life science students. However, many life science students do not understand the utility value of mathematics to biology. Therefore, according to expectancy-value theory, life science students may experience lower motivation, which can impact their performance in a calculus course. This study examines how two different biocalculus courses, which integrated calculus and biological concepts and successfully halved the rates of students earning a D, F, or withdrawing (DFW), affected life science students’ utility value, interest, and overall attitudes toward mathematics. Using pre and post surveys, we found that students’ interest in mathematics increased by the end of the semester, and they demonstrated a more sophisticated understanding of how mathematics is used in biology. Students whose attitudes toward mathematics improved primarily attributed these changes to a better understanding of the utility of mathematics to biology, feelings of competence in mathematics, or rapport with the instructor. Thus, communicating the utility value of mathematics to biology through integrated mathematics–biology courses can contribute to improved attitudes toward mathematics that can impact students’ motivation and performance.
INTRODUCTION
Due to an increasing demand for quantitative skills among life scientists, undergraduates in biology today need to demonstrate competency in a variety of quantitative skills (National Research Council [NRC], 2003; Steen, 2005; Association of American Medical Colleges–Howard Hughes Medical Institute [AAMC-HHMI], 2009; American Association for the Advancement of Science [AAAS], 2011). These skills include the ability to create and interpret graphs, the ability to statistically analyze data, and the ability to mathematically model systems (NRC, 2003; AAMC-HHMI, 2009; AAAS, 2011). Because biological systems are dynamic, calculus plays a key role in the modeling of biological systems. For example, calculus is used in population growth models to account for complex demography (Ellner and Rees, 2006), in Susceptible-Infectious-Removed (SIR) models to understand disease spread (e.g., Buceta and Johnson, 2017), and in tumor growth and metastasis models (e.g., Bilous et al., 2019). Therefore, many life science students are required to take a calculus course to satisfy their major or pre-med requirements.
However, life science students are often perceived to be math averse and, therefore, may be less than enthusiastic about taking a calculus course. Although recent work did not demonstrate particularly poor motivational attitudes among life science majors toward the use of mathematics in biology courses, it did reveal a tremendous amount of variation in these attitudes (Andrews and Aikens, 2018). For example, Andrews and Aikens (2018) found that, although the average score for students’ interest in using mathematics to understand biology was 4.5 on a scale from 1 to 7, 1 SD around the mean encompassed values from 2.8 to 6.2. Additionally, one-third of life science students reported high cost (anxiety and effort) of using mathematics in biology courses. Thus, a significant portion of life science students likely enter a calculus course with little interest and some anxiety. Compounding the problem, if calculus courses do not connect the concepts to biological applications, life science students may complete the course without an understanding of why calculus is even required for their major or pre-professional program, making the course feel like a hurdle to overcome rather than a valued learning experience.
Integrated science, technology, engineering, and mathematics (STEM) courses can improve life science students’ affect toward other STEM disciplines. For example, life science students in Introductory Physics for Life Science (IPLS), a course designed to relate principles of physics to biological concepts, reported an increase in their interest in physics at the end of the semester (Crouch et al., 2018). Students who have taken IPLS report that the biological applications are interesting and relevant to their future careers (Meredith and Bolker, 2012; Crouch et al., 2018). In particular, students report that the physics problems related to biological concepts they have previously encountered in their biology classes are the most interesting, because such problems allow them to see the connections between physics and their biology course work (Geller et al., 2018). Therefore, one approach to improving life science students’ attitudes toward mathematics, and calculus in particular, is to teach calculus through an integrated biocalculus course in which calculus concepts are applied to biological problems.
Recognizing the value of teaching calculus within a biological context, a number of biocalculus textbooks and courses have been developed (e.g., Adler, 2012; Comar, 2013; Uhl and Holdener, 2013; Bodine et al., 2014; Neuhauser and Roper, 2018). However, few studies have examined the extent to which an integrated biocalculus course can improve life science students’ attitudes toward mathematics. In this study, we report on students’ affective outcomes after participating in a biocalculus course designed to intentionally integrate calculus concepts into biological problems. We use expectancy-value theory as a framework for understanding students’ affective experiences, focusing on utility value, as described in the following section.
Theoretical Framework
Expectancy-value theory connects students’ personal values and self-beliefs to their motivation and achievement. Specifically, it posits that students’ expectations of success on a task and their personal values for a task will affect their persistence on a task, particularly challenging tasks, and thus their performance on a task (Eccles et al., 1983; Wigfield and Eccles, 2000). Students who believe that they can successfully complete a task are more likely to persist on a task to completion. However, simply believing a task can be successfully accomplished does not ensure students will persist on a task; they must also find some personal value in that task. Task values include intrinsic value (interest and enjoyment), utility value (usefulness of the task for a future goal), attainment value (importance of doing well on a task for one’s identity), and cost (negative aspects of engaging in a task; Wigfield and Eccles, 2000).
Utility value represents students’ perceptions of the importance or usefulness of a task for their future goals (Eccles et al., 1983). It has been shown to be positively correlated with student performance in a variety of contexts (Zusho et al., 2003; Cole et al., 2008; Hulleman et al., 2008). Additionally, studies in which students’ utility value is experimentally enhanced through a utility-value intervention have found increases in performance compared with a control group of students (Hulleman and Harackiewicz, 2009; Hulleman et al., 2010). The utility-value intervention also has been shown to narrow achievement gaps between underrepresented and overrepresented groups in science (Harackiewicz et al., 2016). Therefore, there is strong empirical evidence that students’ perceptions of the usefulness of the material they are learning in their class can play an important role in their course performance.
Experimental research using utility-value interventions has also documented increases in students’ interest in a topic as a result of the intervention (Hulleman and Harackiewicz, 2009; Hulleman et al., 2010). Hidi and Renninger (2006, pp. 113) describe the development of individual interest, an “enduring predisposition to reengage particular content over time,” as resulting from knowledge, positive feelings, and value. Thus, recognizing the value of course material in their lives can contribute to the development of students’ interest in the subject matter (Hulleman et al., 2010). Interest is an important predictor of academic achievement (Schiefele et al., 1992) and academic choices, such as courses taken or major (Harackiewicz et al., 2002). For example, interest in mathematics has been found to predict both grades in mathematics and the number of mathematics courses taken during high school (Simpkins et al., 2006). It has also been argued that developing students’ interest should be a goal in and of itself, beyond its role in motivation, because interest is fundamental to students’ happiness and well-being (Harackiewicz and Hulleman, 2010).
Redesigning calculus courses to enhance the utility value of mathematics for biological applications may be one way to increase life science students’ interest in mathematics and, ultimately, positively influence their motivation and performance in calculus courses. Cognitive psychologists have demonstrated that students are unable to transfer skills between contexts (Gick and Holyoak, 1983; NRC, 2000). Therefore, it is not surprising that when mathematics and biology are not explicitly linked in a curriculum, students have a difficult time envisioning the connection (Arnett and Van Horn, 2009). However, when students’ learning experiences integrate the two disciplines, they are more likely to report that mathematics is important to biology (Arnett and Van Horn, 2009; Thompson et al., 2010). Additionally, placing mathematics in a genuine, real-world context in an interdisciplinary science and mathematics course has led to increases in both students’ perceptions of the importance of mathematics to biology and their enthusiasm for mathematics (Matthews et al., 2010).
Research Objectives
This study examines changes in students’ utility value, interest, and overall attitudes toward mathematics in two distinct biocalculus courses at two different institutions: the University of Portland (UP) and Unity College (Unity). At UP, the impetus to create a biocalculus course arose out of the observation that biology students were demonstrating generally lower performance in the standard calculus course than their other STEM counterparts and poor quantitative reasoning skills in subsequent courses. Therefore, creating a calculus course that emphasized the relevance of mathematics to biology seemed like a possible avenue to more deeply engage students and improve their performance. Unity designed and implemented a biocalculus course to foster integration between disciplines as part of its larger mission in sustainability science education and transdisciplinary problem solving. The development and structure of both biocalculus courses is described in detail in Diaz Eaton and Highlander (2017).
In the study by Diaz Eaton and Highlander (2017), they provided evidence that a carefully designed biocalculus course can improve student performance and retention. Compared with students taking a standard calculus course the same semester, students in the biocalculus course at UP demonstrated higher performance on a common quiz at the end of the semester, despite these students initially having significantly lower performance on a common precalculus quiz at the beginning of the semester. Additionally, rates of students earning a D, F, or withdrawing (DFW rates) were about 50% lower in the redesigned biocalculus courses at both Unity and UP compared with previous years when life science students were in traditional, non-biology calculus courses. In this paper, we build upon those results and show such a course can also improve students’ attitudes toward mathematics by demonstrating the relevance of calculus to biological problems. Specifically, we asked the following questions: 1) To what extent do students’ perceptions of the usefulness of mathematics for their major and their career change after taking a biocalculus course? 2) To what extent does students’ interest in mathematics change after taking a biocalculus course? 3) To what extent do students have more sophisticated views of the utility of mathematics to biology after taking a biocalculus course? 4) What aspects of the biocalculus courses caused students to have generally more positive attitudes about mathematics after taking the course? To answer these questions, we conducted pre and post surveys across multiple sections of the biocalculus classes and analyzed both Likert-type items and open-response items.
METHODS
Setting and Participants
The study was conducted from 2011 to 2014 in biocalculus courses developed at UP and at Unity. A full description of the courses, the development, and the context can be found in Diaz Eaton and Highlander (2017). Unity is a small liberal arts college offering only environmental-related majors. At the time the study was conducted, students who took Calculus I typically did so as a major program requirement for earth and environmental science and wildlife biology. Due to the small size of the college, typically one section per semester had been offered. The biocalculus course that was created to fulfill Calculus I requirements has a mixture of lecture, guided-inquiry worksheets, writing, and projects. Modeling (Diaz Eaton et al., 2019), Excel, metacognition, and communication skills (Diaz Eaton and Wade, 2014) were emphasized, and proofs of major theorems and symbolic calculations “by hand” were de-emphasized.
UP is a small, private Catholic university, with professional schools in business, education, engineering, and nursing and a liberal arts core in the College of Arts and Sciences. Although the UP Biology Department elected in 2010 to remove the calculus requirement for life science majors, many pre-med students were still interested in taking calculus. Therefore, a biocalculus course was created for these students with input from biology faculty. The course included all of the standard Calculus I topics, but with a focus on the applications to modeling biological phenomena, using Fred Adler’s (2012)Modeling the Dynamics of Life: Calculus and Probability for Life Scientists. The course was taught using a more traditional, lecture-based approach, with an emphasis on collaborative problem solving and modeling activities inside and outside class. Students were also required to submit regular journal assignments that asked them to reflect on what they were learning and how, including which concepts they were struggling with and why, and what study methods they were implementing. There were two sections of the course offered each year.
At both institutions, the instructors of the course (C.D.E. and H.C.H.) administered a mathematics attitude survey, modified from Richard Schori’s survey for the Oregon Collaborative for Excellence in the Preparation of Teachers (Schori, 2015). Pre and post surveys were given in class on the first and last days of class to assess any changes in student attitudes toward mathematics. This study was approved by the Institutional Review Board (IRB) at Unity (UCIRB 2012-05) and by the IRB at UP (UPIRB 2011).
Surveys at Unity were collected over four semesters (each semester from Fall 2012 to Spring 2014) and over five different sections of the course (two sections offered in Fall 2012). Surveys at UP were collected over five semesters (each semester from Fall 2011 to Spring 2013 and Spring 2014) and over six different sections of the course (two sections offered in Spring 2014). Only students who completed both the pre and post surveys, whose data were able to be matched between the pre and post surveys, and who were life science majors or pre-med students (four students were not life science majors or pre-med) were included in the analyses. Students were instructed to write individualized codes on each pre and post survey that consisted of a numerical sequence of their birth months, birth dates, and the last two digits of their social security numbers to be used to match the pre and post survey data. Many students at Unity inconsistently reported the coding prompt, making some pre and post data matching impossible. In particular, data from only two students in one Fall 2012 section and one student in another Fall 2012 section could be matched. Because this represented an extremely low response rate for these sections, data from Fall 2012 at Unity were not included in the analyses. The average class size of the biocalculus course at Unity was approximately 14 students, and the average class size of the biocalculus course at UP was approximately 20 students. Response rates, calculated as the number of life science students with pre–post data that could be matched divided by the total number of students in the class section, ranged from 58 to 83% at Unity and 65 to 88% at UP. A total of 32 students from Unity and 87 students from UP are included in the analyses. However, two students were dropped from the quantitative analyses, because they did not report demographic information included as variables in the model-selection process in those analyses. Participant demographics are shown in Table 1.
UP | Unity | Total | |
---|---|---|---|
Gendera | |||
Male | 29 | 8 | 37 (31%) |
Female | 57 | 24 | 81 (68%) |
Year in school | |||
First-year | 44 | 16 | 60 (50%) |
Sophomore | 26 | 4 | 30 (25%) |
Junior | 9 | 7 | 16 (13%) |
Senior | 8 | 5 | 13 (11%) |
Self-reported final GPAb | |||
2.00–2.99 | 4 | 6 | 10 (8%) |
3.00–3.24 | 12 | 6 | 18 (15%) |
3.25–3.49 | 22 | 8 | 30 (25%) |
3.40–3.74 | 24 | 4 | 28 (24%) |
3.75–4.00 | 24 | 8 | 32 (27%) |
Measures
Each survey included 25 Likert-type items about students’ mathematics attitudes (five-point scale from “strongly disagree” to “strongly agree”), as well as demographic items (e.g., major, year in school, gender, and self-reported grade point average [GPA]), and short, open-response items (see Supplemental Material for the full pre and post survey). The Likert-type items measuring attitudes were not constructed as scales, which necessitated individual analyses of each item rather than summing or averaging scores across items. For the purposes of this study, we only analyzed a subset of Likert-type, demographic, and open-response items related to our research questions. Among the Likert-type items, we analyzed three items that represented utility value: 1) Mathematics is important for my chosen profession (career utility value 1), 2) The skills I learn in this class will help me in my career after college (career utility value 2), and 3) The skills I learn in this class will help me in other classes for my major (major utility value). We also analyzed one item that represented interest: Mathematics is enjoyable and stimulating to me.
We included the following demographic variables in our analyses: gender (male or female), self-reported GPA at the end of the semester, and year in school (first-year, sophomore, junior, or senior). For GPA, students chose the range in which they believed their GPAs fell: 2.00–2.99, 3.00–3.24, 3.25–3.49, 3.50–3.74, or 3.75–4.00. Thus, GPA is a categorical variable with five levels. Although students reported their GPAs in both the pre and the post survey, many of the students were taking the biocalculus course during their first-year Fall semester. Thus, we chose to use self-reported GPA on the post survey in our analyses because it was not clear to us what a GPA at the beginning of a student’s first semester in college represented.
For the open-response items, we qualitatively analyzed responses to two questions: 1) In your opinion, is the knowledge of mathematics beneficial to biologists? Why or why not? 2) Do you feel that your attitude toward and/or beliefs about mathematics has changed over the course of this semester? Why or why not? If you answered “yes,” what has most influenced any changes in your attitude or beliefs about mathematics? The first question was included on both the pre and post survey, and we used this question to address our third research question related to students’ sophistication in their understanding of the utility value of mathematics for biology. The second question was only on the post survey. We used this question to address our fourth research question, in which we aimed to identify the factors that contributed to a positive change in students’ attitudes.
Data Analysis
Quantitative Analysis: Likert-Type Items.
We used ordinal mixed-effects regression models with an adaptive Gauss-Hermite quadrature approximation (10 quadrature points) to determine whether students’ utility value and interest, as measured by the Likert-type items, significantly changed from the beginning to the end of the course. Ordinal regression is more appropriate for Likert-type items than linear regression, because the numbered responses represent ordered categories. Unlike the difference between integers, where the linear distance between 1 and 2 is the same as between 2 and 3, differences between ordered categories may not be equal. In other words, the difference between strongly disagree and disagree may not be the same “distance” as the difference between disagree and neutral (Theobald et al., 2019). We conducted four separate regressions in which each of the four Likert-type items, representing utility value or interest, served as a dependent variable. Time (categorical variable that is either “pre” or “post”) was included as a predictor variable in all regressions. The time variable is important in repeated-measures mixed-effects models, because it represents differences in scores between the time points (i.e., change in scores). In our model, “pre” was the reference level for the time variable, so the regression output for the time variable indicates the degree to which post scores are different from pre scores. We used model selection to determine whether other predictor variables should be included as fixed effects in each regression. All analyses were conducted in R v. 3.5.0 (R Core Team, 2018) using the ordinal package (Christensen, 2018).
We used mixed-effects models in order to include repeated measures of each student as a random effect. Additionally, we tested whether class section necessitated inclusion as a random effect in each model by calculating the intraclass correlation coefficient (ICC) and by comparing Akaike information criterion values corrected for small sample sizes (AICc) between full and reduced models (Theobald, 2018). ICC is a ratio of between-group variance (i.e., between–class section variance) to total variance, and is calculated for a regression model that includes only the random effect (Theobald, 2018). Small ICC values (<0.05) suggest little variance between groups, and thus a random effect accounting for group variation may not be needed in the model (Theobald, 2018). ICC values for class section in our regression models ranged from 0 to 0.06. For each attitude item, we also compared the AICc value for a full regression model with class section as a random effect to the AICc value for a reduced regression model without class section as a random effect (Theobald, 2018). Lower AICc values indicate a model with better fit, but AICc values within 2 indicate models with similar fit (Burnham and Anderson, 2002). When the difference in AICc values between the full and reduced model was within 2, we kept the simpler model (i.e., the reduced model). For all four of our regressions, the reduced model had a lower AICc value than the full model with class section as a random effect. Moreover, not including class section in the regression models did not change the significance of any predictor variables in our final model. Therefore, although some of the ICC values were slightly above 0.05, we chose to use simpler models in our analyses by not including class section as a random effect.
For each regression model, we also used model selection to determine which predictor variables to include in the model. The full model included categorical main effects, interaction terms, and student as a random effect:
Dependent variable ∼ time (pre or post) + institution (UP or Unity) + gender + final GPA + year in school + time*institution + time*gender + time*final GPA + time*year in school + (1|student)
We compared AICc values among the full model and all possible nested models that included time and student using the dredge function in the package MuMIn (Bartón, 2018). We examined all models with AICc values within 2 of the best models and chose the simplest of these models to run as our final model. In evaluating the significance of the predictor variables in our final models, we adjusted our alpha level using a Bonferroni correction to account for multiple hypothesis tests. Across the four regression models, we conducted hypothesis tests on seven predictor variables, so our alpha was 0.007. Using an alpha of 0.007 as a threshold for significance allows for conservative tests, such that any predictor variable with a p value less than 0.007 is likely not a result of a type I error (false positive).
In ordinal regression, the odds ratio calculated from the regression coefficient represents the odds of increasing from one level of the dependent variable to a higher level of the dependent variable. A major assumption of ordinal regression is that the odds are the same for all levels of the dependent variable. In other words, the odds of moving from the lowest level to a higher level are the same odds as moving from the second-lowest level to a higher level. This is known as the proportional odds assumption. We tested whether our data fit the proportional odds assumption by modeling time and institution as nominal effects, which relaxes the assumption that the odds of each of the variables are the same among all levels (Christensen, 2018). We then used likelihood ratio tests to compare the models including time and institution as nominal effects with our ordinal model in which we assumed proportional odds. For all four models, the likelihood ratio tests indicated the model assuming proportional odds was a better fit, indicating our models met the assumption of proportional odds.
Qualitative Analysis: Open-Response Items.
Responses to the open-response questions were analyzed using deductive and inductive coding. A set of codes were established a priori for whether students thought mathematics was beneficial (three codes: beneficial, not beneficial, not sure) and for whether students’ attitudes changed after taking the biocalculus course (three codes: attitude improved, attitude stayed the same, attitude worsened). However, we used inductive coding, in which we derived codes based on the data in students’ open-response answers (Saldaña, 2016), to code why students reported mathematics was beneficial to biology and why their attitudes changed.
The two questions were analyzed separately, each following the same general procedure for initial coding. First, a subset of 20 responses to the question were coded independently by two researchers (M.L.A. and a biology education graduate student for the first question; M.L.A. and an undergraduate researcher in biology education for the second question). The researchers met and discussed the codes, came to consensus on the codes, and created an initial codebook. The researchers then independently coded another 20 responses to the question and through discussion came to consensus on the codes. They revised the codebook as necessary, either by adding new codes or by revising the definitions, inclusion criteria, or exclusion criteria of the original codes. When codes were added or revised, the researchers reread previously coded responses and recoded as necessary. This iterative cycle of independently coding 20 responses, coming to consensus on codes, revising the codebook, and recoding as necessary was repeated until all responses had been coded.
For the first open-response question asking students whether and how mathematics is beneficial to biologists, we first coded whether students said mathematics was beneficial, mathematics was not beneficial, or they were not sure if mathematics was beneficial. For those students who expressed that mathematics was beneficial, we then coded the explanations they gave for why mathematics was beneficial. We did not code explanations for why mathematics is not beneficial, because only four students, between the pre and post surveys, reported that mathematics was not beneficial, representing a very small sample size on which to base conclusions. We assessed the reliability of our codes by giving 30% of the pre survey and 30% of the post survey responses to H.C.H. to be independently coded. We used Holley and Guilford’s (1964) G index of agreement as a measure of interrater reliability (IRR) due to the high frequencies of some codes and the low frequencies of other codes. When codes demonstrate distributional skew, either because they are used extremely frequently or very rarely, Cohen’s kappa can be quite low even with high interrater agreement (Xu and Lorber, 2014). The G index of agreement is less sensitive to distributional skew in the codes, but still takes into account chance agreement (it assumes an equal probability of choosing a code; Xu and Lorber, 2014). All codes had a G greater than 0.80, and only two codes had a G < 0.90. Because we had generated a large number of codes, many of which were found in only a handful of responses, we condensed some of the initial codes into broader categories (Saldaña, 2016). As codes were clustered into categories, all responses within each code were reread to ensure each coded segment reflected the meaning of the broader category. The codebook with the initial codes, categories, and definitions of the codes and categories is included in the Supplemental Material.
For the second open-response question, which asked students if their attitudes had changed and why, we first coded whether students’ attitudes had improved, worsened, or stayed the same. If students’ attitudes stayed the same, we coded whether they had positive or negative mathematics feelings. If students’ attitudes improved or worsened, we coded reasons for these changes. We assessed the reliability of our codes by giving 30% of the student responses (found only on the post survey) to C.D.E. to be independently coded. One code had a G = 0.76, but all other codes had a G > 0.80 (and only one code had a G >0.80 but <0.90). At the end of coding, we had generated 15 codes, only seven of which applied to students whose attitude improved, which is the focus of our study; therefore, we did not condense codes into broader categories. The codebook with the initial codes and definitions of the codes is included in the Supplemental Material.
RESULTS
Model selection resulted in slightly different models for each measure of utility value (Supplemental Table S1), but in no case did utility value significantly change from the beginning to the end of the semester (Supplemental Table S2). Only one of the career utility-value items (Mathematics is important for my chosen profession) demonstrated significant institutional differences, with UP students reporting overall lower scores on this item compared with Unity students; B = −1.69 (±0.56), p = 0.002. Gender was included in the best model explaining major utility value, but it was not significant according to our adjusted alpha value.
In contrast to the results for the utility-value items, students at both Unity and UP reported a significant increase in their interest in mathematics by the end of the semester; B = 1.02 (± 0.29), p = 0.0004 (Supplemental Table S2). The final model for interest included both time and institution as predictors. Overall interest gains were similar between institutions (0.40 at Unity and 0.33 at UP), although Unity had overall lower (though not statistically significant lower) interest scores than UP (Figure 1).
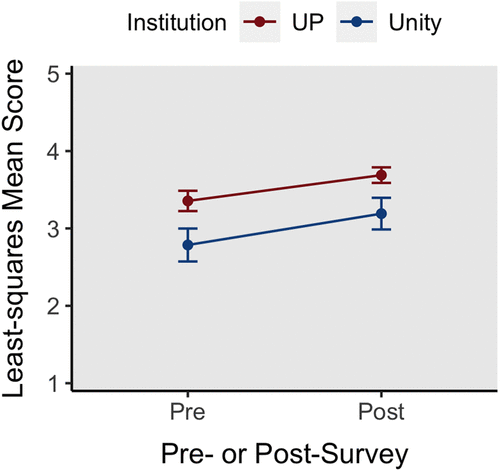
FIGURE 1. Least-squares means of pre and post scores for the interest survey item: Mathematics is enjoyable and stimulating to me. Error bars represent ± SE.
Qualitative Results: Why Is Mathematics Beneficial to Biology?
In response to the open-ended question asking students whether mathematics is beneficial for biology and why, 94% (111 out of 118 total responses on this question) of students stated that mathematics was beneficial for biology in the pre survey, and 99% (117 out of 118 total responses on this question) of students stated that mathematics was beneficial for biology in the post survey. In the pre survey, 3% of students reported mathematics was not beneficial to biology, and another 3% of students reported they were unsure whether mathematics was beneficial to biology or not. However, only one student (1%) reported mathematics was not beneficial in the post survey.
Students provided a variety of reasons for why mathematics is beneficial to biology that revealed a range of sophistication in understanding the role of mathematics in biology. We describe the seven major categories that emerged from the open-response data: Broad Utility, Biology Utility, Chemistry Utility, Calculations, General Analytical Skills, Science Process Skills, and Specific Applications (Table 2; Figure 2A). Percentages reported are based on the number of students who reported mathematics was beneficial.
Category | Definition |
---|---|
Broad Utility | Student expresses that mathematics is useful for daily life or science broadly but does not specifically reference biology. |
Biology Utility | Student expresses that math is useful for understanding biology concepts, understanding a broad subdiscipline of biology, or doing biology experiments or research. However, student does not detail how mathematics is specifically used in biology or biology research. |
Chemistry Utility | Student expresses that math is generally useful for understanding chemistry broadly or the chemistry that is related to biology. |
Calculations | Student expresses that mathematics is useful for doing calculations or making estimations in biology. |
General Analytical Skills | Student expresses that mathematics is useful for general problem-solving, critical-thinking, or reasoning skills. |
Science Process Skills | Student expresses that mathematics is useful for specific skills associated with doing science research. |
Specific Applications | Student expresses that mathematics is important for doing specific functions or solving specific biological problems. |
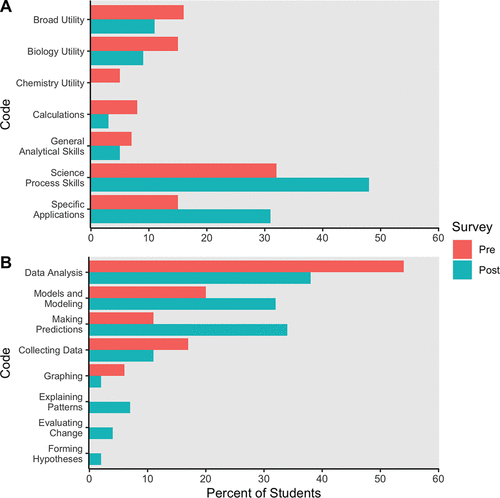
FIGURE 2. (A) Frequency of categories describing why mathematics is beneficial to biology among individuals who reported mathematics to be beneficial to biology (n = 111 in pre survey; n = 117 in post survey). (B) Frequency of specific science process skill codes (from initial coding) among individuals whose responses to why mathematics is beneficial to biology were categorized under Science Process Skills (n = 35 in pre survey; n = 56 in post survey). Multiple categories or specific science process skills may have been coded per student, so total percentage sums to greater than 100%.
The Broad Utility and Biology Utility categories represent vague responses for why mathematics is beneficial for biology. Responses in the Broad Utility category did not contain reasons why mathematics was important specifically for understanding biology; rather, students reported that mathematics was broadly important for daily life or for understanding science in general. Overall, these responses indicated that students believed mathematics was important, but they did not demonstrate an explicit connection between mathematics and biology. On the other hand, the Biology Utility category contained student responses in which students stated mathematics was important for broad understanding of biological concepts or processes or for doing biological research but did not clarify in what ways mathematics might be important. Responses did not identify how mathematics may be used in biology, biological concepts or processes for which mathematics may be particularly useful, or any specific practices of biological research that use mathematics, any of which would indicate a deeper understanding of the connection between the disciplines. Overall, the lack of specificity in responses coded as Biology Utility suggests a less sophisticated understanding of the role of mathematics in biology. Together, the Broad Utility and Biology Utility categories encompassed 31% of student responses (16% Broad Utility, 15% Biology Utility) on the pre survey, but only 20% of student responses (11% Broad Utility, 9% Biology Utility) on the post survey (Figure 2A).
A small percentage of students (5%) reported on the pre survey that mathematics was useful for understanding chemistry or for specific chemistry applications, such as titrations (Figure 2A). Most of these responses reflect students’ perceptions that mathematics is indirectly used in biology through chemistry applications. No students reported mathematics was useful for understanding chemistry on the post survey.
Some students were much more specific in their responses, indicating that mathematics was useful for specific skills used in biology (Figure 2A). A small percentage of students indicated that mathematics was important for calculations (pre survey: 8%; post survey: 3%). Many more students indicated that mathematics was useful for more higher-order cognitive skills. Some students responded that mathematics was useful for critical thinking, problem solving, or logical reasoning (pre survey: 7%; post survey 5%). These responses were coded as General Analytical Skills, because they referred to a general set of higher-order cognitive skills identified as important to biology. In contrast, other students reported mathematics was useful for specific skills in reference to conducting biological research, which we have categorized as Science Process Skills. Science Process Skills include collecting data, analyzing data, using or creating models, and making predictions. The number of students reporting a science process skill as a reason why mathematics is beneficial to biology increased from 32% in the pre survey to 48% in the post survey. Among the students who reported a science process skill on the pre survey (n = 35), 54% reported that mathematics was useful for data analysis in biology research. However, among the students who reported a science process skill in the post survey (n = 56), almost the same percentages of students reported that mathematics was useful for making predictions (34%), using or creating models (32%), and analyzing data (38%) (Figure 2B).
Finally, 15% of students on the pre survey indicated a specific biological example in which mathematics was useful; this increased to 31% of students on the post survey (Figure 2A). Most of the examples were related to population growth and dynamics, though drug dosage, game theory, epidemics, and carbon dating were also mentioned by students.
Qualitative Results: Has Your Attitude toward Mathematics Changed? How and Why?
Of the 117 students who wrote responses that could be coded, 47% of students reported that their attitudes toward mathematics improved by the end of the semester, 44% of students reported their attitudes toward mathematics had not changed, and 9% of students reported that their attitudes toward mathematics had worsened. Of the 51 students whose attitudes stayed the same, 31% of students had positive mathematics attitudes, 41% of students had negative mathematics attitudes, and the remaining students did not indicate whether they had positive or negative mathematics attitudes.
Students whose attitudes toward mathematics improved over the semester reported three primary reasons for this change: the relevance and applicability of the material to biology, an ability to comprehend the material, and the professor’s teaching style (Table 3 and Figure 3). Other codes were used only once or twice in student answers and are not discussed here (see Supplemental Material for complete codebook).
Code | Definition |
---|---|
Relevant or applicable | Students’ attitudes improved because they found the material or class to be relevant to their interest or major and/or applicable to their field of study. |
Ability to comprehend the material | Students’ attitudes improved because they felt like they understood the concepts, how to approach the problems, and/or materials presented in class. |
Professor | Students’ attitudes improved because of something about the professor and the way the professor teaches. |

FIGURE 3. Frequency of predominant codes for why students’ attitudes toward mathematics improved among individuals who reported their attitudes improved (n = 55). Multiple reasons may have been coded per student, so total percentage sums to greater than 100%.
The most common reason reported for a positive change in attitudes was understanding the relevance of mathematics (45% of students whose attitudes improved). Some students emphasized how much they preferred learning about the applications of the calculus concepts rather than simply memorizing mathematical equations. Therefore, explicitly showing students how mathematics could be used within a biological context, that is, demonstrating the utility of mathematics to the field of biology, positively changed how students viewed mathematics.
Another common reason reported for improved attitudes was students’ ability to understand the calculus (35% of students). In some cases, students explicitly mentioned previous struggles with calculus. Students’ responses did not indicate that the restructuring of the calculus course into a biocalculus course was the reason for their ability to understand the material. Rather, it seemed to be related to the instructional style. Regardless, understanding the material appeared to increase students’ perceived competence in their mathematical skills, which promoted a more positive attitude toward mathematics.
Finally, 35% of students whose attitude improved indicated that something about the way the professor taught the course was the underlying reason. This code was found for both instructors in this study, indicating it was not just one particular instructor’s unique teaching methods. Rather, this code included a variety of aspects of an instructor’s teaching style that promoted a positive learning environment. Students mentioned things such as patience, dedication to the students, and the creation of a supportive learning environment as being critical to their improved attitudes. Therefore, student–instructor rapport and classroom climate may be important factors to consider when examining students’ attitudes about a subject.
DISCUSSION
Life science majors and pre-medical students are often required to take a calculus course, yet many calculus courses do not make explicit, authentic connections between calculus and biology concepts. This can deter students from developing an understanding of the relevance of mathematics to biology and an interest in mathematics, which has been shown to affect student performance. We sought to understand the extent to which two redesigned biocalculus courses for life science students, in which calculus concepts were explicitly rooted in biological problems, would improve students’ utility value and interest in mathematics, and what components of the course were critical for improving overall attitudes toward mathematics. Figure 4 summarizes the relationships we found (solid arrows), as well as relationships we posit based on theory and results from other studies (dashed arrows). Our data suggest that integrating biology and calculus, such that the relevance of calculus to biological contexts is emphasized, can improve students’ understanding of the utility of mathematics for biology, which can promote positive overall attitudes toward mathematics among life science students. Additionally, although we could not test this here, we hypothesize that the observed increase in students’ interest in mathematics was mediated, at least partially, by their more sophisticated understanding of the relevance of mathematics to biology. Our data also demonstrate that students’ perceived competence at mathematics and the rapport they have with the instructor are also important factors that contribute to improved attitudes toward mathematics. Thus, cultivating positive attitudes toward mathematics goes beyond simply demonstrating the relevance of mathematics to biology, but also encompasses instructional techniques and behaviors that cultivate feelings of competence and rapport, such as clarity in explanations, opportunities for students to succeed, enthusiasm for the subject, and approachability.

FIGURE 4. Factors that contribute to improved affect toward mathematics among life science students in biocalculus courses. Solid arrows represent relationships supported by the results of this study. Dashed arrows represent relationships supported by theory and/or other studies. The arrow from perceived competence to another arrow represents moderation.
Situating Calculus in a Biological Context Improves Students’ Understanding of the Utility of Mathematics to Biology
The biocalculus courses were designed to demonstrate the utility of mathematics to biology through problems that used a biological context. For example, at UP, where most students enrolled in biocalculus were interested in careers in medicine, students developed discrete-time dynamical systems to model the change in chemical concentration within the human lung when an individual breathes in the surrounding air containing a fixed concentration of the chemical. Upon model development, they then used various graphical and analytical tools from calculus to study the resulting equilibrium concentration within the lung. At Unity, where a significant number of students were wildlife biology majors, students worked on deriving a recommendation for a land trust’s lake by using discrete-time models to allow for population growth, stocking, and harvesting. In this project, they were asked to consider additional constraints beyond known biology such as reproductive rate—for example, the target permit numbers for tourism and planning, the amount of investment capital the trust had to invest in stocking, and environmental variability. They then had to write a report of findings to the land trust with a final recommendation.
Because of the applied nature of these courses, we predicted a shift in utility-value scores from the pre survey to the post survey, but these predictions were not borne out by the quantitative data. Examining the pre survey data, we found that students’ utility-value scores were high; approximately 75% of students chose 4 or 5 on a five-point Likert scale for each of the three utility-value items, indicating most students agreed or strongly agreed that mathematics was important for biology before even taking the biocalculus course (Supplemental Figure S1). Therefore, there was little room for improvement in scores on the post survey, and ceiling effects may explain why we did not see a significant increase. Other studies have reported similarly high values for life science students’ perceptions of the importance of mathematics to biology, either before engaging in a quantitative biology curriculum (Karsai et al., 2015; Hoffman et al., 2016) or in a control group of students who did not engage in a quantitative biology curriculum (Thompson et al., 2010), suggesting that most life science majors recognize, at least superficially, the importance of mathematics to the biological sciences.
The qualitative data provided deeper insight into students’ utility value of mathematics for biology by asking them how they perceived mathematics to be useful to biology. This question illuminated gaps in students’ understanding of the connection between mathematics and biology that the quantitative data were unable to demonstrate. Notably, on the pre survey, only 45% of students described mathematics as useful for a specific science process skill and/or a specific application in biology, meaning that 55% of students who indicated mathematics was beneficial for biology did not connect mathematics in a meaningful way to the process of biological research or to the understanding of biological phenomena. However, by the end of the course, the percentage of students who cited a science process skill and/or a specific application that requires mathematical knowledge increased to 68%. Together, the quantitative and qualitative data suggest that biology education successfully inculcates in students the idea that mathematics is important for biology, but there is room for improvement in teaching students how mathematics is actually used in biology. Integrated mathematics–biology courses such as biocalculus are one way to effectively fill this knowledge gap in students. These data also point to the limitations of using quantitative measures of utility value and raise the question of the extent to which students need to understand how a task is useful to their future goals for it to meaningfully affect their academic performance and choices.
Among the science process skills, data analysis was most commonly cited in students’ responses in both the pre and post surveys. It is not surprising that many students think of data analysis when they think of mathematics in the biological sciences. Biology labs often incorporate data collection and interpretation into their curricula, and if students have read primary literature for their courses, then they have likely encountered statistics. Additionally, at Unity, most students take statistics before Calculus I. One successful outcome of the biocalculus courses was to broaden students’ perspectives on the way mathematics is used in biology. On the post survey, “using and creating models” and “making predictions” were cited almost as many times as “data analysis” (Figure 2B). Because of the courses’ emphasis on mathematical modeling, students gained a greater understanding and appreciation of the role of modeling in the biological sciences, which is a core competency for life science students (AAAS, 2011). Exposing students to a wide array of uses of mathematics within biology can enrich students’ views of how mathematics enhances understanding in the biological sciences.
Understanding of the Utility of Mathematics to Biology and Perceived Competence Promote Positive Attitudes toward Mathematics
It was encouraging that almost half of the students taking the biocalculus courses reported more positive overall attitudes toward mathematics by the end of the semester. Recognizing the relevance and utility of mathematics to biology was one of the main drivers of these improved attitudes (Figure 3). Many students reported that they had developed a better appreciation of or respect for mathematics after seeing how applicable and important it was to biological problems. These results are similar to those found in other integrated STEM courses, such as the IPLS courses (Meredith and Bolker, 2012; Crouch et al., 2018). Although overall attitudes toward mathematics have been found to only weakly correlate to achievement in mathematics (Ma and Kishor, 1997), it seems plausible that improved attitudes may positively affect how students engage with mathematics in their life science courses and careers. As pointed out by Wilkins and Ma (2003, pp. 52): “A person’s mathematical disposition related to her or his perceptions about and attitude toward mathematics may be as important as content knowledge for making informed decisions in terms of willingness to use this knowledge in everyday life.”
Although some students reported on the open-response question that the application of calculus to biological problems made the mathematics more interesting, we cannot make a causal connection between students’ enhanced views of the utility of mathematics for biology and their increase in interest in mathematics as demonstrated through the quantitative data. However, studies have established that utility-value interventions can result in an increase in interest in the topic (Hulleman et al., 2008, 2010; Hulleman and Harackiewicz, 2009). However, this relationship appears to be moderated by students’ perceived competence and whether the utility value is directly communicated or self-generated. When the utility value of a topic to a student’s career is directly communicated, those with lower perceived competence may actually report lower interest in the subject (Canning and Harackiewicz, 2015). In contrast, students with higher perceived competence report greater interest in the task or subject because it reinforces the importance of something at which they are already competent (Canning and Harackiewicz, 2015; Durik et al., 2015). This aligns with Hidi and Renninger’s (2006) concept of interest development, in which positive emotions, such as those generated by feelings of competence, in conjunction with values drive the development of individual interest. Interestingly, in our study, students’ confidence in their ability to understand the calculus concepts and do the problems was an important factor in improving their overall attitudes toward mathematics. Therefore, increasing students’ ability beliefs in mathematics through instruction may be critical for leveraging the utility value communicated through the curriculum of a biocalculus course. Future studies should aim to explore more deeply the relationships among utility value, interest, and perceived competence in integrated STEM courses.
Improving students’ perceptions of the usefulness of mathematics for biology and their interest in using mathematics within a biological context is important for promoting positive student outcomes related to quantitative biology. Many studies have found that students’ utility value or interest in a topic predict, either directly or indirectly, their academic performance (Durik et al., 2006; Simpkins et al., 2006; Cole et al. 2008; Hulleman et al., 2008). Additionally, studies in which interventions have been employed to increase students’ utility value or interest in a topic have led to increases in student performance compared with control groups lacking the intervention (Hulleman and Harackiewicz, 2009; Hulleman et al., 2010; Canning et al., 2018). In this study, we did not collect individual student grades, so we are unable to examine the extent to which changes in interest or students’ understanding of the utility value of mathematics to biology affected student achievement. However, comparing DFW rates in the biocalculus courses at Unity and UP to DFW rates in the standard, non-biology calculus courses that preceded these courses at these institutions demonstrated that DFW rates were halved after implementation of the biocalculus curriculum (Diaz Eaton and Highlander, 2017). Additionally, students in the biocalculus course at UP had significantly higher final common quiz scores than students who were taking the traditional calculus course that same semester (Diaz Eaton and Highlander, 2017). It is important to point out, though, that perceived competence, which is empirically similar to expectancies of success in expectancy-value theory, is also posited to predict performance (Wigfield and Eccles, 2000). Because this was a prevalent code among students whose attitude improved, we cannot discount the idea that changes in students’ perceived competence in the subject matter, rather than utility value or interest, may have factored into the observed changes in DFW rates and quiz scores. Utility value and interest have also been found to predict students’ academic choices, such as course enrollment choices or intentions to remain in a STEM major (Durik et al., 2006; Simpkins et al., 2006; Canning et al., 2018). Future studies should examine whether changes in students’ interest or understanding of the relationship between mathematics and biology arising from integrated mathematics and biology courses increase their likelihood of taking additional quantitatively focused biology courses.
Instructor–Student Rapport Affects Students’ Attitudes toward the Content
Although not related to the redesign of the calculus courses, the positive influence of the instructor on students’ attitudes toward mathematics must be noted. This result aligns with findings from the social psychology and instructional communication literature on the relationship between instructor behaviors and student affective and cognitive learning outcomes. Students’ affective learning outcomes, or their attitudes toward the instructor and content of the course, have been shown to be affected by instructor immediacy (Rodriguez et al., 1996; Witt et al., 2004) or behaviors that enhance psychological perceptions of liking and closeness (Mehrabian, 1971). More recently, the importance of instructor–student rapport, or a perception there is a mutual, trusting, prosocial bond between the instructor and student, to learning outcomes has been emphasized (Frisby and Martin, 2010; Frisby and Housley Gaffney, 2015). Instructor–student rapport is a perception that results from instructors’ behaviors, such as immediacy, and may therefore serve as a link to explain how instructor behaviors affect student learning outcomes (Frisby and Martin, 2010; Frisby and Housley Gaffney, 2015). Responses from our study coded as “Professor” contain descriptions of the professor that correspond to many of the components that have been used to measure instructor–student rapport, such as helpful, enthusiastic, encouraging, and enjoyable (Wilson et al., 2010). These responses suggest an element of instructor–student rapport was important for improving student attitudes toward the mathematics content. This is an important consideration that should be factored into future studies examining changes in student attitudes of course content.
Limitations and Future Directions
We implemented a pre–post study design that did not have a control group and cannot state with certainty that all changes observed between the pre and post surveys were due to participation in the biocalculus course. In particular, the increase in interest and the change in sophistication of students’ responses about how mathematics is beneficial to biology could be due to general maturation or a shift that would have naturally been seen over this time period regardless of the biocalculus course (Shadish et al., 2002). Alternatively, we cannot rule out the possibility that the observed changes may have been a result of other experiences the students had over the course of the semester, perhaps in other courses or in a research experience. Additionally, changes in interest may have simply resulted from changes in students’ perceived competence in calculus, rather than from the integrated nature of the course. However, the responses obtained when we queried students directly about the impact of the course on their overall attitudes suggests that at least some change in interest in mathematics is due to the integration of the disciplines. Moreover, many of the responses on the post survey question asking students why mathematics was beneficial for biology referenced specific applications that had been covered in the biocalculus course (e.g., population growth, modeling the concentration of a chemical in a lung over time), suggesting the course content was indeed influencing their views on how mathematics is used in biology. Nevertheless, a control section, in which calculus was taught in a traditional manner to biology students, would have strengthened our conclusions about the effect of integrating mathematics and biology on students’ attitudes toward mathematics.
Additionally, the data collected were from primarily female students in biocalculus courses at two small, predominantly white institutions with two instructors, which limits the generalizability of the data. Importantly, gender and first-generation college status have been found to affect students’ interest in mathematics or their perceptions of the utility of mathematics to their careers (Gaspard et al., 2015; Andrews and Aikens, 2018). We included gender (as a binary variable) in our analyses and did not find it to be a statistically significant predictor of attitudes or of change in attitudes. However, there are two caveats to this finding: 1) model selection results suggested the presence of gender differences in students’ scores for major utility value, but our sample size (overall sample size and sample size of male students) may not have been large enough to detect a statistically significant difference using a Bonferroni-corrected alpha value; and 2) our sample size may not have been large enough to detect an interaction between time and gender, which would indicate changes in attitudes from pre to post differ between the genders. We did not collect demographic data about first/continuing-generation or race/ethnicity from students. Therefore, we recommend that additional studies examine change in affect in a broader diversity of students to more fully understand how attitudes change in different students. Moreover, we recommend examining biocalculus courses at a wide variety of institutions to understand how institutional context may influence changes in affect. For example, class size may affect instructor–student rapport, which may affect the extent to which students’ attitudes change. Studies conducted across diverse contexts with diverse students will ultimately allow for the identification of the critical elements of a biocalculus course necessary to engender positive affect toward mathematics.
Implications for Mathematics and Biology Educators
Understanding biological concepts relies on an understanding of chemistry (e.g., metabolic pathways), physics (e.g., physiological mechanisms), and mathematics (e.g., modeling communities or ecosystems), yet life science students often lack an understanding of how these disciplines are connected to biology and are less enthusiastic about taking these required courses. Our results provide empirical evidence that student attitudes toward mathematics improved after completing a course that highlighted the relevance and applicability of calculus to biology. Creating an integrated mathematics–biology course, such as a biocalculus or biostatistics course, can be one way to achieve this goal. Furthermore, a biocalculus course can contribute to the development of three of the core competencies outlined in Vision and Change (AAAS, 2011) for life science students: the ability to use quantitative reasoning, the ability to use modeling and simulation, and the ability to tap into the interdisciplinary nature of science. Based on reflection as instructors, and to help guide other instructors, we suggest a focus on the following three key items to nurture positive student attitudes toward mathematics: 1) interdisciplinary curricula and conversations, 2) authentic problems for the audience, and 3) intentional metacognition interventions.
Interdisciplinary Curricula and Conversations.
For those interested in how to develop an interdisciplinary biocalculus course, we refer readers to Diaz Eaton and Highlander (2017). However, we recognize that creating a separate biocalculus course may be beyond the scope of what most departments can do. Therefore, we recommend that both mathematics and biology instructors incorporate examples and problems into their current courses that draw upon the other discipline. Importantly, many curricula have already been developed for that purpose. The QUBES website (www.qubeshub.org) has a repository of quantitative biology curricular activities, and CourseSource (coursecourse.org) has quantitative activities listed under some of the learning goals in the Science Process Skills Learning Framework. Duane Nykamp at the University of Minnesota has developed freely available materials, including videos, projects, and in-class worksheets, for a flipped-format biocalculus course (Nykamp, 2020). These materials have been used heavily at UP since Spring 2014. Curricular resources have also been described in a number of papers (e.g., Jungck et al., 2010; Thompson et al., 2010; Schultheis and Kjelvik, 2015; Hoffman et al., 2016). However, even though we are mathematical biologists by training, our work in connecting mathematics and biology for students has been deeply enhanced by ongoing interdisciplinary conversations with our biology colleagues and vice versa (Diaz Eaton et al., 2019). To guide broad collaborative discussions between life scientists and mathematicians, we recommend the special issue “Interdisciplinary Conversations” published in Problems, Resources, and Issues in Undergraduate Mathematics Education (Ganter et al. 2019a,b).
Authentic and Relevant Problems.
Critically, when integrating mathematics and biology to enhance life science students’ understanding of the connection between the disciplines, thought must be given to incorporating authentic biological problems. Authentic biological problems do not just use mathematics to solve a problem situated within a biological context, but rather integrate mathematics into a biological problem in a way that allows the student to come to a greater understanding of a biological phenomenon or process (Watkins et al., 2012). Developing authentic biological problems that integrate mathematics requires expertise from both disciplines and will benefit from time invested in collaborative relationships. To guide the creation and revision of authentic problems, we recommend using the framework presented by Svoboda Gouvea and colleagues (2013), which can be used to reflect on how the disciplines interact in the problems.
It is also important to consider how to make the problems relevant to the interests of the students. Biology students have diverse interests, ranging from cellular and molecular mechanisms to physiology and medicine to ecology and evolution. Therefore, an understanding of what specific interests the students in a class have can be used to design relevant biocalculus problems. For example, C.D.E. realized that medically oriented time-of-death problems used in the exponential growth and decay section were met with reluctant engagement by her predominantly wildlife biology–focused students. But a colleague in conservation law stopped her in the hallway one day excitedly to tell her about using the same technique and tables to discern the time of death for a deer in a suspected poaching. C.D.E. was able to share this story with the students, relating a newly revised and contextualized problem to concepts learned in other classes and touted by an expert in their desired career, and they anecdotally seemed more interested and engaged (e.g., see Geller et al., 2018, which supports this anecdotal evidence). Both H.C.H. and C.D.E. also reflect that conversations with junior and senior biology majors in the class can be similarly helpful in recognizing key linkages between classes and impressing upon younger students the importance of these topics.
Metacognition and Utility-Value Interventions.
Both H.C.H and C.D.E. employed metacognitive interventions at least every other week. For example, H.C.H. regularly shared relevant articles with students and asked them to reflect on the articles in their journals. In one such journal assignment, the prompt was as follows:
Read the article posted on Moodle entitled “The ‘Gift’ of Mathematics in the Era of Biology” and provide a one-paragraph summary of the article. Topics you may want to address include the following:
What is BIO 2010? What did it urge colleges to do?
What are some similarities they mention between math and biology?
What was most surprising in this article?
Were there parts of the article you didn’t understand or terms you didn’t know? If so, include these in your summary.
C.D.E. presented students with a variety of prompts to encourage reflection. For example, one prompt asked students to read excerpts of news articles and discuss how one excerpt related to the class. Interested readers can find the full set of writing prompts that C.D.E. used on QUBES (Diaz Eaton, 2020).
Utility-value interventions are motivation interventions aimed specifically at boosting students’ utility value. They are relatively easy to implement; students simply write about how what they are learning in class is relevant to their lives (e.g., see Canning and Harackiewicz, 2015). Several studies have demonstrated the positive effect of a utility-value intervention on student interest and performance, though these effects appear to primarily occur in students with lower perceived competence (Hulleman and Harackiewicz, 2009; Hulleman et al., 2010). Recent work suggests that the timing of the utility-value intervention is important to consider but that three utility-value interventions over the course of a semester, with written feedback, are ideal, because they allow students multiple opportunities to make connections between the topics and their lives (Canning et al., 2018).
CONCLUSION
Our results demonstrate that integrated biocalculus courses can improve life science students’ attitudes toward mathematics by explicitly demonstrating the relevance of mathematics to biological problems. Importantly, by the end of the course, many students had a more sophisticated understanding of how mathematics is used in the biological sciences, and their responses represented a broader perspective on the role mathematics can play in the biological sciences. Therefore, adding a biology-oriented calculus option for life science students can improve students’ understanding of the connection between the disciplines, ultimately improving student motivation for learning and their performance. Recognizing that transforming disciplinary courses into interdisciplinary courses is a significant undertaking, we recommend that instructors of both mathematics and biology courses initiate a dialogue to select and/or design problems that can be included in both types of courses, ensuring they are biologically authentic. Further research on integrated mathematics–biology courses among more diverse student populations and institutions will be invaluable for identifying the instructional and curricular aspects of integrated courses that are critical for improving student motivation and learning. Additionally, documenting longer-term outcomes of integrated mathematics–biology courses, such as how students engage with mathematics in their future courses, will be essential for understanding the extent to which these courses induce long-term change in students’ epistemological beliefs about the role of mathematics in the biological sciences.
ACKNOWLEDGMENTS
We thank Richard M. Schori for allowing us to modify and use the attitude survey that he originally developed. We thank Alexis White, Kieu Tran, Kenny Nguyen, and Alexander Kulacki for their assistance with data entry and analysis. We also thank Erin Bodine, Suzanne Lenhart, and Lou Gross and Princeton University Press for the use of the draft versions of their textbook, Lou Gross for feedback on an earlier draft of this paper (which was eventually split into Diaz Eaton and Highlander, 2017, and this paper), and two anonymous reviewers for their helpful feedback.