Undergraduate GPA Predicts Biochemistry PhD Completion and Is Associated with Time to Degree
Abstract
There is interest in admission criteria that predict future success in biomedical graduate school programs, but identifying predictors of PhD attainment is inherently complex. In particular, high noncompletion rates of PhD programs have long been recognized as a major crisis. Here, we present a quantitative analysis of the PhD students enrolled in the Department of Biochemistry and Biophysics at Texas A&M University between 1980 and 2010. The input variables included sex, country of citizenship, undergraduate grade point average (GPA), and Graduate Record Examination (GRE) scores (Verbal and Quantitative Reasoning). Only GPA was a significant predictor of PhD completion based on logistic regression. We also examined associations involving nonbinary measures of success (PhD duration, first author, and total number of publications) among students who completed a PhD. GPA was again associated with the PhD duration. No enrollment variable was strongly associated with publication output. Despite potential limitations, this analysis is the first to suggest an undergraduate GPA association with PhD completion in life sciences. These results from a large state university in a predominantly rural area expand the range of programs from which such analyses have been reported.
INTRODUCTION
PhD Program Completion and Duration and Their Significance
The PhD is an advanced research degree offered after several years of study to students admitted to PhD programs of accredited institutions of higher education. The National Center for Science and Engineering Statistics within the National Science Foundation (NSF) conducts regular surveys of earned PhD degrees (https://ncses.nsf.gov/pubs/nsf21308). In 2019, U.S. institutions awarded 55,703 PhD degrees, of which 42,980 were in science and engineering. In life sciences alone, 9842 PhD degrees were awarded in 2019. The sheer numbers and commitments at multiple levels associated with the overall enterprise of doctoral education motivate analyses of factors that determine outcomes of PhD programs (Weiner, 2014).
The ultimate goal in graduate education is to recruit, retain, and graduate students, producing well-trained individuals for future careers in highly specialized and advanced fields. This goal is undermined by the high noncompletion rate of students enrolled in PhD programs. There are arguments that some degree of noncompletion in PhD programs may not necessarily be a problem, as some doctoral students decide that a PhD will not provide them with the necessary training for their optimal career paths (Cassuto, 2013). Nonetheless, the fraction of PhD candidates that do not complete their degrees seems excessive. For example, PhD completion in 6 years or fewer averaged 49.0% among 1168 biological and health sciences PhD programs in a National Research Council (NRC) report (Ostriker et al., 2015). Overall, PhD completion was 62.9% for life sciences in a Council of Graduate Schools report in 2008 (Sowell et al., 2008). To make matters worse, among students who do eventually complete their PhDs, degree completion often takes exceedingly long. These problems are significant for several reasons. There are substantial financial costs to all parties involved (institutions, faculty, and students). PhD noncompletion or longer times to degree also negatively impact the graduate program because of a perceived loss in academic reputation. Not completing the PhD could lead to a sense of failure and lost opportunity for the students. It is noteworthy that >40% of doctoral students consistently report signs of psychological and mental health issues associated with their studies (see Sverdlik et al., 2018; https://gradresources.org/research). Consequently, the high noncompletion rate of PhD programs has long been recognized as a major crisis (Mooney, 1968; Lovitts and Nelson, 2000; Caruth, 2015).
Frameworks of Different Approaches to Analyzing PhD Program Outcomes
To evaluate predictors of graduate program outcomes, one could ask at least two broad questions: Is it the graduate program that “makes” a successful student? If so, then identifying and restructuring the problematic components of graduate programs ought to improve completion rates and time to degree. Such program components include an overall collegial environment that promotes social interaction among students and faculty, generating synergies that stimulate students’ research and scholarly productivity (Weidman and Stein, 2003). Alternatively, is it the “quality” (however one defines it) of the students at admission that ultimately makes a program successful? In that case, identifying and applying such admission criteria may improve outcomes. It is only logical that the two scenarios are not exclusive of each other, and one could easily imagine both student- and institution-related factors contributing to degree noncompletion or long times to degree (Breneman et al., 1976).
The problem of PhD noncompletion has attracted interest for decades, especially after World War II and the passage of the National Defense Education Act in 1958, which provided unprecedented federal support in fellowships and loans to PhD students to bolster education in the areas of science, mathematics, and modern foreign languages. A landmark study in the 1960s (Mooney, 1968) looked at 3542 PhD candidates, who from 1958 to 1960 received the prestigious Woodrow Wilson National Fellowship. Around 20% of these highly qualified students did not complete their PhDs (Mooney, 1968). Similar conclusions were reached 30–40 years later (Baker, 1998; Wendler et al., 2010), showing that a PhD dropout rate of about 25% persisted among students awarded very competitive graduate research fellowships, such as those from the Graduate Research Fellowship Program of the NSF.
Surveys of PhD candidates who did not complete their degrees point to various reasons for leaving graduate school, including dissatisfaction with the program and personal reasons (at the top of the list was “change to family status”; Wendler et al., 2010). On the other hand, surveys of PhD completers listed major factors contributing to their success: financial support, mentoring and advising, and family support (Sowell, 2009). Other contributing factors included social environment and peer group support, program quality, and career guidance (Sowell, 2009). Hence, while some specific personal problems for some students may be unaddressable by institutions, other problems leading to noncompletion could be solved by institutional interventions (providing adequate funding and support, better mentoring, etc.). The theoretical framework of our analysis does not involve any features of the institution or the graduate program. Instead, we deal exclusively with student-related characteristics at admission and examine whether any of these are associated with successful outcomes.
Measuring PhD Program Outcomes in Life Sciences and Their Association with Admission Variables
Analyses of quantifiable student admission variables assume that one or more of these variables holds explanatory and predictive value for student performance in the future. From a large pool of applicants, one could then select the students most likely to succeed (Weiner, 2014; Park et al., 2018). Importantly, however, there has never been a randomized trial, affording the strongest statistical reliability, to test the power of any putative admission predictors to forecast future success in a graduate program. Nonetheless, several analyses have sought to examine variables considered for admission to a life sciences PhD program and their correlation with outcomes.
The outcomes evaluated most commonly are easy to collect, including how long it takes to complete the PhD (years to degree [YTD]) and the number of publications authored (or coauthored) by students, although various programs may implement additional performance metrics (Petersen et al., 2018; Sealy et al., 2019). For example, a study of 280 students enrolled in a PhD program at the University of North Carolina in 2008–2010 found that higher ratings from recommendation letters correlated with publication output, mainly when the student in question was the lead author (Hall et al., 2017). The North Carolina study and two others from Vanderbilt University (Moneta-Koehler et al., 2017; Sealy et al., 2019) did not find any correlation between PhD productivity outcomes and Graduate Record Examination (GRE) test scores. On the other hand, a Boston University School of Medicine study found that undergraduate grade point average (GPA) and to a lesser extent GRE scores were associated with a higher performance during the PhD (Park et al., 2018). Overall, these studies emphasized predictors of productivity and performance during a PhD in life sciences and focused less on PhD completion itself.
PhD completion rates were explicitly examined in other reports that did not focus on life science PhD programs. A multicenter study of 1805 students enrolled in science, technology, engineering, and mathematics (STEM) PhD programs in 2000–2005 looked at PhD completion and found that GRE scores were poor predictors (Petersen et al., 2018). A study of about one-eighth of PhD students enrolled in physics graduate programs in 2000–2010 sought to identify typical admissions criteria correlated with completing a PhD (Miller et al., 2019), reporting that undergraduate GPA predicts for PhD completion. Still, the analysis and interpretation of that study have been debated (Weissman, 2020).
Other studies using large aggregate data sets from multiple programs have reported that standardized tests (including the GRE) are valid predictors of student success across fields (Kuncel and Hezlett, 2007). However, there has been no systematic, randomized trial for any predictors (e.g., GRE scores, GPA), so potential biases in the available observational studies are often difficult to identify or overcome. For example, while most institutions keep data for their enrolled students, there are often no data for all their applicants. There are also range restrictions if students are already selected based on a predictor. Then, the observed correlation in the range-restricted sample will be lower than if data from the entire range were analyzed (Weissman, 2020). Indeed, in a notable study looking at GRE scores collected but not used, thus avoiding restriction of range, the GRE was a valid predictor of student success in a psychology PhD program (Huitema and Stein, 1993). It has also been pointed out that splitting the individual components of the GRE, which are usually correlated with each other, inflates their variance and lowers the net effect of the GRE as a predictor in subsequent regression models (Weissman, 2020). Additional problems involving endogenous selection biases may lead to erroneous conclusions about causality when linking predictors to outcomes (Elwert and Winship, 2014). Despite the inherent limitations and potential artifacts in examining predictors of graduate student success, the interest in this area remains intense.
Student Identities and Their Role in PhD Program Outcomes
Two other parameters we sought to evaluate, sex and citizenship status, have also been analyzed in previous studies.
Women are underrepresented in many STEM fields. The situation worsens during career advancement, with women progressively abandoning science careers more frequently than men do, a phenomenon known as the “leaky pipeline” (Berryman, 1983; Blickenstaff, 2005). Analyzing gender in PhD success can be highly complex, confounded by heterogeneous and large gender differences in interest across STEM fields (Su and Rounds, 2015). Such interest differences are profound in some fields (e.g., in engineering), but insignificant in other ones (e.g., life sciences; Su and Rounds, 2015).
Several studies report no or minor gender disparities in PhD completion. For example, gender was not associated with degree completion in some PhD programs in the United Kingdom (Seagram et al., 1998; Wright and Cochrane, 2000; Park, 2005), Canada (Sheridan and Pyke, 1994), or the United States (Wao and Onwuegbuzie, 2011), including MD–PhD programs (Jeffe et al., 2014). On the other hand, a large aggregate study from the Council of Graduate Schools (King, 2008) suggested that women may have lower PhD completion rates. Likewise, an extensive survey of students awarded prestigious NSF predoctoral fellowships reported that female students were slightly less likely to complete a PhD in natural sciences and engineering than male students were (Baker, 1998). But when adjusted for differences in the students’ academic background, degree completion was unrelated to gender, and “determinants of progression to and completion of the doctorate by women and minorities are largely the same as those of Caucasian males” (Baker, 1998).
Nonetheless, even in the same institution, disparities among different programs lead to differences in outcomes during PhD studies. In most, but not all, STEM programs at the University of California–Berkeley, students belonging to groups that are underrepresented in STEM fields and, to a lesser and less consistent degree, female students were not encouraged to publish and had fewer opportunities to present their research (Mendoza-Denton et al., 2017). It has also been reported that female PhD candidates are more likely to complete their degrees in departments with higher proportions of female faculty and work with female thesis advisors than male advisors (Main, 2018). While a gender gap in PhD completion seems to be narrowing, and in some fields significantly so (e.g., in life sciences; Baker, 1994), there is continuing interest in the role of gender in PhD outcomes.
Citizenship (domestic vs. international) and success in PhD programs is important to study, because the growth of graduate programs has often been accompanied by an increase in the fraction of international students, especially in the United States. By 2000, 30% of all PhD degrees from U.S. institutions were awarded to international students (Hoffer et al., 2001). Studies from the United Kingdom (Park, 2005) and New Zealand (Spronken-Smith et al., 2018) have reported higher PhD completion rates for international students, a result that was also reported in the large study from the Council of Graduate Schools in the United States (King, 2008). However, a study of doctoral candidates in Canada reported that it takes significantly longer for international students to complete their PhDs (Sheridan and Pyke, 1994). Given that international students make up a significant portion of the PhD student body, it is necessary to continue examining the outcomes of this demographic group.
Features and Motivation of This Study
We analyzed the available data for students enrolled in the Graduate Program in the Texas A&M University Department of Biochemistry and Biophysics (TAMU-BCBP) toward a PhD in biochemistry over 30 years, from 1980 to 2010. This retrospective study tested whether any available information collected at admission could predict successful PhD outcomes. We examined associations between enrollment variables (sex, citizenship status, GRE test scores, undergraduate GPA) and PhD completion. We also looked into variables typically associated with productivity during the PhD, such as YTD and number of publications authored by students. We found that the undergraduate GPA was a significant predictor for PhD completion and PhD duration.
Our study is significant for several reasons: First, the sample size (several hundred students) is considerable for such a study of a single program. Because it spans three decades, it is likely robust to year-to-year variations in the cohorts of admitted students. Second, it is inherently “normalized” for institutional variables that are often hard to capture, because we focused on a single program. Third, previous aggregate studies from multiple institutions and programs have pointed to “student quality” as a predictor of PhD completion (Baker, 1998; Kuncel and Hezlett, 2007; Miller et al., 2019). To our knowledge, however, this is the first time a clear association between undergraduate GPA and completion of a PhD in a given life sciences program has been reported. Despite its potential limitations (e.g., no data for the total applicant pool, possible unknown biases in the enrolled students), this study adds to the growing body of analyses of attainment levels in doctoral programs.
METHODS
Institutional Profile and Student Admission Data
Texas A&M is a large, public research university, reporting $1.131 billion in research expenditures for the 2020 fiscal year to the NSF for the Higher Education Research and Development survey. In the Fall of 2020, there were 3988 PhD candidates at the main campus in College Station, TX, enrolled on a full-time basis. Among those, 2929 were STEM students, of which 234 were from underrepresented groups, 921 were women, and 1768 were international students.
The graduate program in the TAMU-BCBP is an NRC-ranked program (Ostriker et al., 2015). The NRC report used data collected from June 2006 until 2010, which overlapped with the last years of this study. TAMU-BCBP was among 158 programs in biochemistry, biophysics, and structural biology included in the NRC analysis. TAMU-BCBP had a ranking similar to biochemistry PhD programs at other land-grant universities in the 2011 report. For example, among these 158 programs, the regression (R) and survey (S) NRC rankings (for a description, see Ostriker et al., 2015) were: 60–99 (the rankings were a range) and 52–124 for TAMU-BCBP; 34–63 and 59–120 for the Biochemistry program at the University of Illinois–Urbana Champaign; 67–116 and 75–142 for the Biochemistry and Molecular Biology program at the University of Florida, respectively (Ostriker et al., 2015). Likewise, the average PhD completion percentage in 6 years or fewer was 45.4% for TAMU-BCBP in the NRC report, against 46.16% for the average of all 158 programs. Finally, the median graduation time was 6 years for TAMU-BCBP and 5.73 years for the 158 programs in biochemistry, biophysics, and structural biology in the NRC analysis (Ostriker et al., 2015).
TAMU-BCBP admits students only toward a PhD degree. The demographic composition of the program in terms of the proportion of women and international students fluctuates from year to year, but no significant shifts are discernible. Though the program does not offer direct admissions for the MS degree, eligible students who elect to withdraw from the PhD program may continue toward an MS degree. For this analysis, students who received an MS were in the noncompletion group. All PhD students admitted to TAMU-BCBP received full financial support through teaching or research assistantships until they completed their studies. All students enrolled from 1980 to 2010 had either completed their PhDs or are no longer in the program. Prior studies of life science PhD programs included in their analyses cohorts of enrolled students still in the program before completing their PhDs (Hall et al., 2017; Moneta-Koehler et al., 2017; Sealy et al., 2019). To accurately analyze PhD completion rates, we did not include any students enrolled since 2011, because some are still in the program. We also excluded any enrolled students who transferred to another institution, because we had no data about their progress toward a PhD.
Profile of Enrolled Students
Our “input” group included 459 students enrolled from 1980 to 2010. The input group consisted of 178 female and 281 male students; 279 were U.S. citizens, and 180 were international students. Although the enrolled international students were from 30 countries, two-thirds were from China and India (Figure 1A). Likewise, although the domestic students (U.S. citizens) were from 37 different states, more than half were from Texas (Figure 1B).
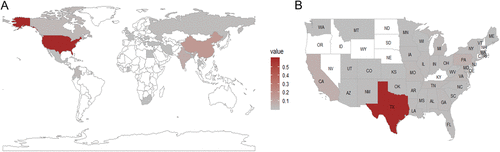
FIGURE 1. Geographic distribution of students enrolled in the TAMU-BCBP PhD program. The values on the maps represent fractions of students based on country of citizenship (A) or of U.S. citizens based on state residency (B). There were no students from Hawaii or Alaska, but three students from Puerto Rico were included in the analysis (not shown on the map in B). The maps were generated with the choropleth R language package. The value scale is the same for both panels.
Academic Measures
In this analysis, we used official GRE scores for Verbal (GRE-V) and Quantitative Reasoning (GRE-Q), as reported by the GRE proprietor Educational Testing Services (ETS), directly to TAMU-BCBP. Because the scoring system changed over the years, older scores were converted to the latest scale based on the concordance tables available from ETS. The Texas A&M Office of Admissions had already adjusted GPAs from non-U.S. institutions. Briefly, if institutions use different scales to report grades (e.g., a 0–10 scale), each grade from the native scale is converted to the respective grade on a 4.0 scale. The calculated GPAs for all enrolled students (U.S. citizens and international students) use only the last 60 credit hours from undergraduate study or the last 30 credits for the students joining the program with an MS degree. The calculated GPAs also exclude large credit items from internships and other projects. These converted GPAs were reported to our department, and we used them in this study.
Student Publications
To tabulate the number of the first author and total publications authored by each student in the data set, we queried PubMed (https://pubmed.ncbi.nlm.nih.gov) manually and with a Python-based “web-crawler” script that included as search terms each student’s name, the name of the corresponding PhD thesis advisor, and “Texas A&M” as affiliation. We included items published after the students graduated and left the program in this analysis.
Statistical Analysis
In all analyses, we used R language functions, as described in detail in each case. Binary variables were converted to [0,1] outcomes (female = 1, male = 0; U.S. citizen = 1, non-U.S. citizen = 0; success [PhD completion] = 1; noncompletion = 0).
RESULTS
GPA and GRE Scores of Enrolled Students
For most but not all of the enrolled students, admission “metrics” available to us were undergraduate GPA and GRE scores (GRE-V and GRE-Q). Scores from the writing component of the GRE were not available. This analysis did not include other information that likely played a role during the admission process, such as reference letters, prior research experience, or interview outcomes. Either the data were not available or were not collected uniformly throughout the period of the study. We note that the TAMU-BCBP PhD program did not have minimum admission cutoffs for undergraduate GPA or GRE scores during the years covered in this analysis. Cumulatively, for all students enrolled in the 30-year period we examined, the summary statistics of the sample are shown in Table 1.
GPA | GRE-V | GRE-Q | |
---|---|---|---|
Average | 3.368 | 154.470 | 154.688 |
Median | 3.380 | 154 | 153 |
SD | 0.394 | 7.066 | 6.693 |
Range | 1.730 | 40 | 26 |
Skewness | −0.302 | −0.262 | 0.277 |
Kurtosis | −0.500 | 0.127 | −0.965 |
The distributions of the GPA, GRE-V, and GRE-Q scores are shown in Figure 2. We also examined the extent to which these values correlate with one another, as shown in Figure 2. We used the nonparametric Spearman coefficient (rho) to gauge correlations, because the distributions appear to deviate from normality (Table 1 and Figure 2). The undergraduate GPA was not correlated at all with either GRE-V (rho = 0.034, p > 0.05) or GRE-Q (rho = 0.051, p > 0.05) scores (Figure 2). However, the two GRE components were significantly correlated (rho = 0.45, p < 0.001). Weissman pointed out that treating the Verbal and Quantitative Reasoning GRE components as separate variables could artificially reduce their predictive power (Weissman, 2020). To retrieve the full impact of the GRE, we followed the approach described by Weissman, summing the two scores after “giving them equal weight by dividing each by its range in the sample” (Weissman, 2020, p. 1). The distribution and correlation of this combined GRE score with GRE-V, GRE-Q, and GPA are also shown in Figure 2. The correlation between GPA and the single or combined GRE was still insignificant (p > 0.05).

FIGURE 2. Distributions and correlation matrix of GPA and GRE scores of enrolled students. At the plots along the diagonal are the histogram distributions of each variable: GRE.V, GRE Verbal; GRE.Q, GRE quantitative reasoning; GRE, combined GRE; GPA). The red lines indicate the corresponding density functions. Below the diagonal are scatter plots between every pair of the variables, with Loess nonparametric regression curves in red. Above the diagonal are the Spearman correlation coefficients for each pairwise comparison, with the font size indicating the magnitude of the correlation. The associated p values are indicated with three red asterisks (p < 0.001 in the cases shown) if the correlation is significant. The correlation matrix was drawn with the function chart.Correlation in the PerformanceAnalytics R language package.
Finally, the annual breakdown of GPA and GRE scores shows a substantially broad range among the enrolled students each year (Figure 3). For example, the GPAs in 2002 ranged from 2.3 to 4.0 (Figure 3, top).

FIGURE 3. Year-by-year enrollment metrics of the TAMU-BCBP PhD program. GPA (top panel), GRE-Q (middle panel), and GRE-V (bottom panel) values are on the y-axis for each student enrolled in the year shown on the x-axis. The crossbar in each box represents the median. The whiskers of each box were drawn at 1.5 times the interquartile range.
Predictors of PhD Completion
Of the 459 enrolled students described earlier, 309 completed their PhDs. The percentage of students completing their PhDs at TAMU-BCBP (67.3%) was above the average of 62.9% for life sciences reported by the Council of Graduate Schools in 2008 (Sowell et al., 2008). We then used logistic regression to look at the relationship between the various predictor variables at enrollment and the binary outcome of PhD completion or not (Sheather, 2009). The predictor variables were categorical (sex, citizenship) or continuous (GPA, GRE-V, GRE-Q, or the composite GRE). At first, only 322 students were included in this analysis, because values were missing for the other 137 students. We did the logistic regression analysis in two ways: using the GRE Verbal and Quantitative Reasoning components as separate variables or combining them as described above. We present the results in each scenario.
GPA Is a Better Predictor of PhD Completion Than Either the Verbal or the Quantitative Reasoning Component of the GRE.
Keeping the Verbal and Quantitative Reasoning GRE components separate, we used the following generalized linear model function: logit←glm(success ∼ sex + citizen +GRE-V +GRE-Q + GPA, data = admit, family = “binomial”), where “success” is PhD completion, evaluated against the indicated five predictor variables we used. The deviance residuals of the model fit were: Min = −1.8346, 1Q = −1.2835, Median = 0.7729, 3Q = 0.9492, Max = 1.3387. We asked whether the model with predictors fits significantly better than a null model. The difference between the residual deviance for the model with predictors and the null model was 13.67396, obtained with the function: with(logit, null.deviance − deviance). For 5 degrees of freedom (obtained with the function: with(logit, df.null − df.residual)), the associated p value was 0.01781863 (calculated with the function: with(logit, pchisq(null.deviance − deviance, df.null − df.residual, lower.tail = FALSE)). Hence, our logit model as a whole fits significantly better than an empty model. The results, including the coefficients, SEs, the z-statistic, and the associated p values, are shown in Table 2. Finally, the odds ratios and their confidence intervals were obtained with the function: exp(cbind(OR = coef(logit), confint(logit))), and they are also shown in Table 2. Only GPA was significantly associated with PhD completion (p = 0.00138), while sex, citizenship, GRE-V, and GRE-Q scores were not.
Variable | Coefficient | SE | z-statistic | p value | Odds ratio | CI(2.5%) | CI(97.5%) |
---|---|---|---|---|---|---|---|
Sex | −0.1548 | 0.2471 | −0.6260 | 0.5312 | 0.8566 | 0.5277 | 1.3928 |
Citizenship | 0.5155 | 0.2876 | 1.7920 | 0.0731 | 1.6745 | 0.9550 | 2.9576 |
GRE-V | −0.0110 | 0.0193 | −0.5710 | 0.5680 | 0.9890 | 0.9519 | 1.0272 |
GRE-Q | 0.0366 | 0.0235 | 1.5610 | 0.1186 | 1.0373 | 0.9909 | 1.0866 |
GPA | 1.0040 | 0.3138 | 3.1990 | 0.0014 | 2.7292 | 1.4870 | 5.1062 |
Because the logistic regression coefficients give the change in the log odds of the outcome, we conclude that for every 1-unit increase in the GPA, the odds of PhD completion (vs. noncompletion) increased by 2.73 times, with confidence intervals (CIs) at 2.5% and 97.5% being 1.49 and 5.10, respectively (see Table 2). After GPA, the predictor variable that showed some association with PhD completion was citizenship status (a U.S. citizen was more likely to complete the PhD). Still, that association did not reach statistical significance (p = 0.07309). Conducting the analysis using only GPA as the predictor variable showed a significant association (p = 0.0108), with a similar odds ratio of 2.60, CI(2.5%) = 1.260864432, and CI(97.5%) = 5.5242183.
We note that we used scale-adjusted GPAs for international students (see Methods). Hence, we also examined only the enrolled students who were U.S. citizens to see whether the association between GPA and PhD completion was still present in this subgroup. We repeated the logistic regression analysis for 185 students with U.S. citizenship, for whom we had complete values for all the input variables (GPA, GRE scores, sex). Again, only GPA was a significant predictor (p = 0.00693), with an odds ratio even higher than the one we obtained from the whole group (U.S. citizens and noncitizens) of enrolled students (odds ratio = 3.33; CI(2.5%) = 1.41; CI(97.5%) = 8.18). Hence, our conclusion that GPA is a significant predictor of PhD completion was not affected by adjusting the GPA scales for international applicants by the admissions office at Texas A&M University. Similarly, we also repeated the logistic regression analysis for the smaller group of 137 international students, for whom we had complete values for all the input variables (GPA, GRE scores, sex). The leading predictor was by far the GPA, albeit, the association was not below the α level of 0.05 (p = 0.0572) with this smaller sample.
GPA Is Still a Better Predictor of PhD Completion Than the Composite GRE.
Because the scores in the two GRE components correlated with each other (see Figure 2), it is possible that treating them as independent predictor variables would bias the regression model due to variance inflation (Weissman, 2020). We used the variance inflation factor (VIF) to detect multicollinearity, which measures if and how strongly predictor variables are correlated. VIF values start from 1 with no upper limit, and they are usually of concern when they are greater than 4 or 5 (Marquardt, 1970). The VIFs for the model described were calculated using the car R language package’s vif(logit) function. They were quite low for all predictor variables: 1.032649 (sex), 1.432603 (citizenship), 1.364613 (GRE-V), 1.806020 (GRE-Q), and 1.035231 (GPA). Hence, variance inflation problems in our regression model were probably minimal, if any.
Nonetheless, given the correlation between the GRE components (we also noted that GRE-Q had the highest VIF value among all predictors), we performed the logistic regression with the combined GRE scores instead of the separate Verbal and Quantitative Reasoning components. The deviance residuals of the model fit were: Min = −1.8440, 1Q = −1.3138, Median = 0.7892, 3Q = 0.9409, Max = 1.3784. The difference between the residual deviance for the model with predictors and the null model was 12.32798. For 4 degrees of freedom, the associated p value was 0.0150. Hence, the logit model as a whole, in this case, also fits significantly better than an empty model. The results are shown in Table 3, calculated with the same functions described in Table 2. The VIF values were slightly lower in this model: 1.029759 (sex), 1.202063 (citizenship), 1.172618 (GRE), and 1.026475 (GPA). The Akaike information criterion (AIC) values of the two models were nearly identical: AIC = 415.65 when keeping GRE-V and GRE-Q separate and 415.00 when combined. Overall, the data strongly suggest that combining the GRE components did not significantly improve the model and did not change the conclusion that, among the enrollment metrics available to us for the data set we examined, the undergraduate GPA has predictive value for PhD completion.
Variable | Coefficient | SE | z-statistic | p value | Odds ratio | CI(2.5%) | CI(97.5%) |
---|---|---|---|---|---|---|---|
Sex | −0.1691 | 0.2463 | −0.6870 | 0.4922 | 0.8444 | 0.5210 | 1.3703 |
Citizenship | 0.3829 | 0.2630 | 1.4560 | 0.1454 | 1.4665 | 0.8765 | 2.4631 |
GRE | 0.3644 | 0.3358 | 1.0850 | 0.2778 | 1.4397 | 0.7469 | 2.7947 |
GPA | 0.9773 | 0.3116 | 3.1370 | 0.0017 | 2.6574 | 1.4535 | 4.9469 |
Logistic Regression with Missing Data.
We also performed logistic regression using all the student data at admission (n = 459 students), including student data missing one or more of the admission variables we considered. The fractions of students for whom we had no data for the GRE (7.6% of total), the GPA (12.9%), or both (9.4%) are shown schematically in Supplementary Figure 1A. We used two different approaches to impute missing data.
First, we used predictive mean matching as an imputation method, implemented with the mice R language package (van Buuren and Groothuis-Oudshoorn, 2011), with the following function: tempData ← mice(admit, m = 5, maxit = 50, meth = ‘pmm’, seed = 500). After a maximum of 50 reiterations, we generated five different data sets with imputed data. The distributions of each imputed data set against the actual observations are shown in Supplementary Figure 1B. To evaluate convergence, we plotted the mean and SD of each variable stream against the iteration number, using the function: imp ← mice(admit, seed = 62006, maxit = 30, print = FALSE); followed by plot(imp). From the plots shown in Supplementary Figure 1C, it appears that convergence is achieved very quickly, with the different streams freely intermingling with one another, without showing any definite trends. Next, we fit a model to each of the imputed data sets and then pooled the results, with the following function from the mice package: modelFit ← with(tempData, glm(success ∼ sex + citizen + GRE + GPA)). Note that we used the combined GRE components as an input variable. We obtained the summary of this procedure with the following function: summary(pool(modelFit)); the results are shown in Table 4. Only GPA was a significant predictor of PhD completion (p = 0.0203; see Table 4).
Variable | Coefficient | SE | p value | Odds ratio |
---|---|---|---|---|
Sex | −0.0438 | 0.0449 | 0.3296 | 0.9571 |
Citizenship | 0.0448 | 0.0483 | 0.3551 | 1.0458 |
GRE | 0.0950 | 0.0723 | 0.1937 | 1.0997 |
GPA | 0.1951 | 0.0759 | 0.0203 | 1.2154 |
Second, we imputed missing data with a methodology that maximizes the observed likelihood, implemented with the R language package misaem (Jiang et al., 2020). We again used the combined GRE components in this approach, with the following function: miss.logit ← miss.glm(success ∼ sex + citizen + GRE + GPA, data = admit, seed = 500), to obtain the coefficient estimates and SEs shown in Table 5. Again, only GPA was a significant predictor of PhD completion (p = 0.0027; calculated from the ratio of the regression coefficient over the SE), with an odds ratio of 2.4 (see Table 5). The next best predictor was the combined GRE, but the effect was not statistically significant (p = 0.1343; see Table 5).
Variable | Coefficient | SE | p value | Odds ratio |
---|---|---|---|---|
Sex | −0.1885 | 0.2133 | 0.3793 | 0.8282 |
Citizenship | 0.2282 | 0.2286 | 0.3186 | 1.2563 |
GRE | 0.4812 | 0.3208 | 0.1343 | 1.6180 |
GPA | 0.8739 | 0.2895 | 0.0027 | 2.3962 |
Overall, our data strongly suggest that, for the admission variables we examined and in each of the different ways we performed the logistic regression, GPA was the only significant predictor variable for PhD completion.
Duration of PhD and Publication Output
We then asked whether any of the enrollment variables are associated with productivity during the PhD. For this analysis, we focused on the 309 students who completed their PhDs. As productivity metrics, we used the number of years it took to complete the PhD (YTD) and the publication output (see Methods). The year-by-year breakdown of YTD for TAMU-BCBP is in Figure 4. The publication output was the number of first-author papers and the total number of papers authored by each student during the PhD work. Publication of graduate work is often required for graduation, introducing selection bias. Hence, we also examined the distribution of papers/year. The summary statistics for all these variables are in Table 6.

FIGURE 4. Duration of PhD among students who completed it. The YTD is on the y-axis for each student enrolled in the year shown on the x-axis. The crossbar in each box represents the median. The whiskers of each box were drawn at 1.5 times the interquartile range.
Papers (total) | Papers (1st author) | YTD | Papers/year | GRE-V | GRE-Q | GPA | |
---|---|---|---|---|---|---|---|
Average | 3.612 | 1.767 | 6.565 | 0.588 | 154.697 | 155.057 | 3.419 |
Median | 3 | 2 | 6.330 | 0.474 | 155 | 154 | 3.440 |
SD | 3.161 | 1.560 | 1.233 | 0.599 | 6.992 | 6.615 | 0.383 |
Range | 31 | 12 | 7.000 | 7.159 | 37 | 26 | 1.730 |
Skewness | 2.816 | 1.555 | 0.783 | 4.832 | −0.206 | 0.268 | −0.364 |
Kurtosis | 18.317 | 5.958 | 0.965 | 46.667 | −0.068 | −1.049 | −0.384 |
To gauge overall relationships between the enrollment variables (GPA and GRE scores) and productivity during the PhD, we used the rank-based, nonparametric Spearman test. All the distributions of these variables and their correlations are in Figure 5. We note that YTD is negatively correlated (p < 0.001) with publication output, both for the first author (rho = −0.25) and total (rho = −0.21) papers. There was no association between enrollment variables and publication output. However, there was a negative correlation between YTD and GPA (rho = −0.23) and between YTD and GRE-Q scores (rho = −0.21). Interestingly, while the GRE-Q score did not predict whether a student will complete the PhD, for the students who do complete it, this score is associated somewhat with how long it will take the student to finish the degree. Nonetheless, albeit statistically significant (p < 0.001), these associations were not very strong (rho = |0.20–0.25|; see Figure 5). Furthermore, there was no association between any of the metrics at enrollment and the overall productivity normalized for PhD duration (papers/year; see Figure 5).

FIGURE 5. Distributions and correlation matrix of enrollment and productivity metrics among students who completed their PhDs. The matrix was plotted as described in Figure 2.
We explored these relations further in linear regression models, accommodating missing data. The structure of the missing data, with the fractions of students who obtained their PhDs but for whom we had no data for the GRE components or the GPA, are shown schematically in Supplementary Figure 2A. We then used the two different approaches described earlier to impute missing data.
First, with the mice R language package, we used the function tempData ← mice(phd, m = 5, maxit = 50, meth = ‘pmm’, seed = 500) to generate five distinct data sets with imputed data. The density plots of those data sets against the actual observations are shown in Supplementary Figure 2B, and the corresponding convergence plots are presented in Supplementary Figure 2C. To find associations between any of the admission variables and YTD, we then used the following function: modelFit ← with(tempData, lm(YTD ∼ sex + citizen + GRE.V + GRE.Q + GPA)). The results are summarized in Table 7. GPA was the variable most significantly associated with YTD (p = 0.0105; see Table 7). Interestingly, domestic U.S. citizens have significantly longer times to degree (p = 0.0497; see Table 7). Hence, while international students are not more likely to complete their PhDs (see Tables 2 and 3), it appears that international students who complete their PhDs finish their studies in less time. The Quantitative Reasoning component of the GRE was somewhat associated with shorter YTD, but in this model, the association was not statistically significant (p = 0.08; see Table 7). There was also no significant association between sex and PhD duration (Table 7).
Variable | Coefficient | SE | p value |
---|---|---|---|
Sex | 0.1595 | 0.1429 | 0.1810 |
Citizenship | 0.3472 | 0.1749 | 0.0497 |
GRE-V | 0.0056 | 0.0119 | 0.6375 |
GRE-Q | −0.0244 | 0.0139 | 0.0812 |
GPA | −0.7392 | 0.2525 | 0.0105 |
Second, we used the misaem R language package to analyze the data from the 309 students who completed their PhDs. We tested whether any of the admission variables are strongly associated with YTD, with the following function: miss.linear ← miss.lm(YTD ∼ sex + citizen + GRE-V + GRE-Q + GPA, data = phd). The coefficients and SEs of the linear model are provided in Table 8. GPA was again the variable most significantly associated with YTD (p < 0.00001). As with the Spearman associations, the Quantitative Reasoning component of the GRE was associated with shorter YTD (p < 0.05; see Table 8). As with the previous linear model, another variable associated with YTD was citizenship, with domestic U.S. citizens having longer times to degree (p < 0.05; see Table 8). Finally, there was no association between sex and PhD duration (Table 8).
Variable | Coefficient | SE | p value |
---|---|---|---|
Sex | 0.1743 | 0.1391 | 0.2112 |
Citizenship | 0.3269 | 0.1586 | 0.0401 |
GRE-V | 0.0047 | 0.0106 | 0.6578 |
GRE-Q | −0.0270 | 0.0128 | 0.0357 |
GPA | −0.8048 | 0.1769 | <0.00001 |
In conclusion, as with PhD completion, our data obtained with multiple approaches argue strongly that GPA is also significantly associated with how long it takes to complete the PhD.
Potential Limitations of This Study
Before discussing the results we presented, we outline some limitations of this type of study. As pointed out in detail before (Weissman, 2020), significant caveats could limit the value of the admission metrics we and others have used (e.g., GPA, GRE) to predict outcomes in doctoral education. Our data argue against variance inflation being a source of bias (Tables 2 and 3), but other variables could be. A potential limitation is the involvement of a downstream variable that affects the measured outcome. If such a variable is causally affected by two or more input predictor variables (including unknown predictors), then a “collider” bias may be in play (Elwert and Winship, 2014). Sampling biases often underlie such collider effects. For example, in the original demonstration of the phenomenon by Berkson (1946), collider bias yielded a spurious negative association between inflammation of the gallbladder and diabetes among hospital patients, but such an association is absent in the general population.
We cannot be sure that collider effects are absent from our analysis. Narrowing the slice of the pool of students taken into consideration increases the chances that correlations between input predictors and outcomes are suppressed (Hall et al., 2017; Moneta-Koehler et al., 2017; Sealy et al., 2019). To identify such effects, one would need data from all the students who applied to the program and those accepted, not just those enrolled, which we analyzed here. Unfortunately, many programs, including ours, do not keep these records. GRE scores may also be significant predictors for PhD completion, but our analysis may have failed to reveal that due to range restriction. We note, however, that even if range restriction lowered the predictive value of the GPA in our study, GPA was still a significant predictor in every test we performed.
Nonetheless, while enrollment variables, such as undergraduate GPA, may be good predictors of PhD completion and duration, factors different from those we examined here may also determine productivity during the PhD. As noted in the Council of Graduate Schools report (Sowell et al., 2008), besides selection and admissions factors, other possible determinants of PhD outcomes include mentoring, the overall environment of the program, structure of the curriculum, research experience at the advisor’s laboratory, and opportunities for professional development. Finally, although all TAMU-BCBP students receive financial support, in individual cases where that support is mostly in teaching assistantships, those students might not progress as fast as they could in their research projects.
There are strong arguments about GRE’s utility and whether its use is harmful in selecting PhD students for admission. A large aggregate study across different fields and institutions argued that GRE scores are good predictors of successful PhD outcomes (Kuncel and Hezlett, 2007). However, it has also been argued that high GRE scores do not necessarily reflect academic ability but rather systemic privilege, placing groups of students who are underrepresented in PhD programs at a disadvantage (Moneta-Koehler et al., 2017; Miller et al., 2019; Sealy et al., 2019; Wilson et al., 2019). Because we had no measure of the students’ socioeconomic status, our study is not controlled for such disparities.
We also point out that the measure of sex that was available and we used in our analysis (male and female) may not necessarily represent students’ gender identities. Hence, this study does not evaluate a range of identities that do not correspond to established ideas of male and female.
Overall, although our conclusions are strongly backed by the analyses we performed, our study may be limited by unknown sampling biases, collider effects, inadequate student classifications, and uncontrolled disparities among students.
DISCUSSION
Why Did GPA Not Feature Prominently as a Predictor of PhD Completion in Life Science Programs Previously?
Among similar analyses of PhD programs in life sciences, our study placed a higher emphasis on PhD completion as an outcome rather than on various performance metrics during the PhD. Because the studies from the University of North Carolina (Hall et al., 2017) and Vanderbilt University (Moneta-Koehler et al., 2017; Sealy et al., 2019) included students still in the programs, it may have been more difficult to detect a link between PhD completion and undergraduate GPA or other enrollment variables. The multicenter study of PhD completion rates in STEM fields did not include the GPA in the input variables, and the authors focused instead on GRE scores (Petersen et al., 2018). We note, however, that GPA was a component of student “quality” shown in the large aggregate study of NSF fellows to be associated with PhD completion (Baker, 1998).
What about the little or no correlation of outcomes with GRE scores reported by the single-institution studies of life science programs mentioned earlier (Hall et al., 2017; Moneta-Koehler et al., 2017; Sealy et al., 2019)? We note that a Boston University School of Medicine graduate program study detected a weak association between GRE scores and performance during the PhD (Park et al., 2018). We also detected an association between GRE Quantitative Reasoning (math) scores and PhD duration (Figure 5 and Table 8). However, these associations with YTD notwithstanding, there were no correlations with the “normalized” productivity metric of papers/YTD (Figure 5). As we discussed earlier, the GRE has been associated with strong biases. We note that many PhD programs abandoned the GRE as a requirement for admission in recent years. Since 2019, TAMU-BCBP has also dropped the GRE requirement for admission.
Unlike the GRE, the GPA is a metric measured across many subjects, over a relatively long period, not through a single exam. Hence, it should not be surprising that it may be a valuable predictor of future success in a graduate program. Nonetheless, although undergraduate GPA may be a strong predictor of PhD completion, we note that the student with the lowest GPA in our data set completed the PhD in the low interquartile range, with three first-author publications and one more article where the student was not the first author. Furthermore, we caution that some of the same reasons that introduce bias into GRE scores may also similarly affect the GPA. For example, students of higher socioeconomic status may be able to afford extra tutoring to improve their undergraduate performance.
Why Other, Perhaps More Holistic Measures of Academic Quality Were Missing from Our Analysis
Our analysis was retrospective. We did not include other enrollment variables incorporated in other studies (e.g., research experience, ratings from recommendation letters, the applicants’ undergraduate institutions), because we did not have usable data spanning the study period. Such metrics undoubtedly offer additional information to evaluate each applicant. However, some standardization needs to be in place for these metrics to be effective in data analyses. For example, the National Institutes of Health T32 ranking matrix of research experience used in the University of North Carolina study (Hall et al., 2017) seems an appropriate, uniform way to record PhD applicants’ prior research experience at admission.
Regarding metrics of PhD productivity, our study dealt only with broad, easily quantifiable ones, such as PhD duration and publication output. Other studies included various performance evaluations (e.g., fellowship acquisition, faculty surveys) in their analyses (Hall et al., 2017; Moneta-Koehler et al., 2017; Sealy et al., 2019). Again, we did not have such data spanning the period of the study. Nonetheless, PhD duration and publication output are likely to capture PhD productivity to a significant degree. It has been reported that publication success 10 years after students obtained their PhDs correlates positively with how many papers they published during their PhDs (Laurance et al., 2013). As we mentioned, it is also likely that variables unrelated to student admission (e.g., the graduate program’s structure, research environment at the thesis advisor’s laboratory) play a major role in PhD productivity. For example, since 2010, the YTD at TAMU-BCBP has been reduced substantially by restructuring the program and implementing policies to improve YTD.
How Representative Is TAMU-BCBP among Graduate Programs, and How Generalizable Could Our Findings Be?
Based on the NRC rankings we described earlier, it is likely that our conclusions may apply to a reasonably broad range of biochemistry graduate programs in public, research-intensive universities. Nonetheless, with more than 1000 PhD programs in biological and life sciences, a few select studies are unlikely to capture the full spectrum of determinants in doctoral education. We also note that some features of the students at TAMU-BCBP did not mirror those of the doctoral student body of its home university. For example, female or international TAMU-BCBP students were each 39% of the cohort we analyzed. But as of last year, women accounted for 31% and international students for 60% of doctoral STEM students at the main campus of Texas A&M University. Large, aggregate multi-institutional studies are robust due to their much greater sample size. But it is also vital to maintain and analyze program-specific data to capture discipline-specific or other effects evident at individual programs. More studies like the one we presented here and those reported previously would be helpful, including as many metrics as possible from programs with varied student and institutional profiles.
Conclusions and Practical Implications
For admission committees at individual departments evaluating applicants, our study suggests that GPA can be a valid predictor of future success. However, it may not be prudent to use any single variable to exclude students from admission. Instead, it may be wise to adopt a multi-tier admission model, wherein students ranked high on metrics such as GPA may be advanced to an accelerated admissions review, as proposed previously (Wilson et al., 2019). That no student is excluded from the next tier of detailed review before admission seems to be a reasonable approach during graduate admissions.
For graduate program directors, because our data show that the admissions metrics we considered are weak at best in predicting YTD and productivity during the PhD, the most reasonable approach would be to focus on interventions that prior research has advocated (e.g., see Sowell et al., 2008), including the following: mentoring, building a supportive environment within the program (both among students and between students and faculty), changing curricula to facilitate graduation, and creating opportunities for professional development.
At the institutional level, it is clear that applying a consistent collection of information at admission across different programs, and maintaining the data from all applicants (instead of just those enrolled), will help tremendously with future statistical analyses. Furthermore, expanding the list of typical metrics (e.g., GPA, GRE scores, or demographics) would be valuable. Institutions could implement a normalized ranking system of recommendation letters or research experience (e.g., following the NIH T32 system, as mentioned earlier). Those data would provide more rounded evaluations of individual applicants in a way that make the data amenable to future analyses to identify additional predictors of future success.
ACCESSING MATERIALS
The Human Research Protection Program (HRPP) at Texas A&M University determined (IRB2021-0252Ml; reference number 121906) that this research meets the criteria for exemption, in accordance with 45 CFR 46.104. However, under the Family Educational Rights and Privacy Act (FERPA), we cannot make the data set publicly available. If others wish to re-examine and analyze the data, please contact Michael Polymenis (polymenis@tamu.
ACKNOWLEDGMENTS
There was no financial support for the research being reportedin this article. MP is supported by grant GM123139, from the National Institutes of Health. The authors thank the anonymous reviewers for their many suggestions, which substantially strengthened the conclusions and improved the article.