Longitudinal Education and Career Outcomes of a Cancer Research Training Program for Underrepresented Students: The Meharry-Vanderbilt-Tennessee State University Cancer Partnership
Abstract
This study examined longitudinal education and career outcomes of the Meharry-Vanderbilt-Tennessee State University Cancer Partnership, the longest-running National Cancer Institute (NCI) Comprehensive Partnerships in Advancing Cancer Health Equity (CPACHE) program site in the United States. Degree completion rates were calculated and progression along the entire postsecondary “pipeline” was quantified for 204 participants recruited between 2011 and 2020. For participants who had entered the workforce, career outcomes were also analyzed. Relative to comparison data, participants completed degrees and progressed through the higher education “pipeline” to earn advanced degrees at remarkably high rates; the majority entered careers in which they support or conduct cancer research. The latter is important, because most participants identify with demographic categories currently underrepresented in the cancer research workforce. This article makes two contributions to knowledge on research training programs for underrepresented students: 1) it quantifies participants’ progression along the entire postsecondary education pipeline as well as into the workforce, and 2) it identifies points where participants are most prone to exit the pipeline rather than progress. We identify two types of exits—permanent and temporary—and offer empirically supported operational definitions for both. Evaluators may find the quantitative model and/or definitions useful for analyzing similar programs.
INTRODUCTION
Cancer Health Disparities and the Cancer Research Education “Pipeline”
Racial disparities in incidence, diagnosis, treatment, and survival of many forms of cancer are well documented in health literature. Although the landscape and patterns of cancer health disparities have changed over time, it remains the case, for example, that 5-year relative survival is lower for Black than White patients for most cancers at each stage of diagnosis (DeSantis et al., 2016). One recognized barrier to progress in achieving more equitable cancer health outcomes is lack of racial diversity in the cancer research workforce (Springfield et al., 2020). While Black Americans make up 13.4% of the U.S. population (U.S. Census Bureau, 2019), they comprise only 6% of the medical and life sciences research workforce (Pew Research Center, 2021), a portion of which is engaged in cancer research. Conducting independent biomedical research requires advanced training and knowledge that can typically be gained only through graduate education in doctor of medicine (MD), doctor of philosophy (PhD), doctor of public health (DrPH), or some master’s of public health (MPH) degree programs. Thus, increasing racial diversity in the cancer research workforce is related to changes in educational outcomes for underrepresented (UR) students (i.e., Black, Hispanic/Latino, Native American, Pacific Islander, and Native Hawaiian students) in the biomedical and life sciences. Indeed, increasing the prevalence of UR students in cancer research education and training programs is an essential strategy for reducing cancer health disparities, per the National Cancer Institute (NCI), the American Cancer Society, the American Academy for Cancer Research, the Institute of Medicine (IOM), and the National Science Foundation (NSF; IOM, 2010; NSF, 2011; Polite et al., 2017).
Like racial disparities in cancer health outcomes, racial disparities in educational outcomes are complex and persistent in the United States. The underlying causes of unequal educational outcomes for UR students relative to well-represented (WR) students (i.e., Asian and White students) are beyond the scope of this paper but certainly include intersecting political, economic, social, and environmental factors. Prior research indicates that disparities in educational outcomes differ not only among racial and ethnic groups but also among fields of study, notably science, technology, engineering, and mathematics (STEM) versus non-STEM fields (Higher Education Research Institute, 2010). For example, national data consistently show that:
UR students enroll in STEM undergraduate degree programs at rates that do not reflect their representation in the college-age population (National Academy of Sciences, National Academy of Engineering, and Institute of Medicine, 2011).
Fewer UR students eventually complete STEM undergraduate degrees relative to WR students (Higher Education Research Institute, 2010).
When UR students do complete STEM undergraduate degrees, they take longer on average than WR students to do so (Higher Education Research Institute, 2010; National Academy of Sciences, National Academy of Engineering, and Institute of Medicine, 2011).
After completing STEM undergraduate degrees, fewer UR students enroll in and complete STEM graduate degrees relative to WR students (Sowell et al., 2015; Council of Graduate Schools, 2019).
These data indicate that nonentry, noncompletion, delayed completion, and selective attrition along the education “pipeline” all affect the size of the STEM-educated UR candidate pool from which future biomedical and cancer researchers can be drawn. Because earning an advanced degree requires a student to have first earned an introductory degree (e.g., a bachelor’s degree), relatively low rates of entering and completing early-pipeline STEM degree programs contribute directly to UR students earning even fewer advanced degrees. In other words, because underrepresentation and undercompletion occur early in the pipeline for UR students, they also grow in magnitude over time (Ginther et al., 2012). Throughout this paper, we use the STEM education pipeline as a proxy for the cancer research education pipeline, both because there has been limited research that specifically investigates the cancer research education pipeline and because the types of degrees most common to aspiring cancer researchers (e.g., biomedical and life sciences) are recognized STEM fields (National Center for Education Statistics [NCES], 2019).
The Pipeline as Analytic Model
Pathways through higher education are frequently described with pipeline metaphors (e.g., Lee et al., 2017; Toldson, 2018; Foroozesh et al., 2019). The pipeline metaphor implies 1) the existence of established, common entry points for students rather than porous boundaries; 2) progression contingent on having passed through a prior “step” or place in the pipeline; and 3) the presence of a destination or end goal. Pipeline metaphors for education also frequently draw on the highly intuitive descriptive concept of “leaking.” An education pipeline “leak” describes an interim point along the pipeline where students are prone to exit rather than progress. For example, some students leave the pipeline after completing bachelor’s or master’s degrees, and these students are generally entering the workforce in lieu of pursuing an MD or PhD.
We would like to acknowledge two important critiques of pipeline models for educational pathways. First, conditioning progress on having completed a prior “step” in the pipeline may be fraught with assumptions about learning that are not only incorrect but also harmful to some students (see Hill, 2019). At the level of individual STEM degree programs—particularly within degree programs where course work may be heavily sequenced with prerequisites—this may be especially true (see Fries-Britt et al., 2010 and Gasiewski et al., 2012). Second, behaviors that are identical from the perspective of a pipeline model may have meaningfully different explanations and implications for equity in education. For example, time off between degree programs might be explained by taking time off to complete a prestigious scholarship or immersive experience (e.g., Fulbright or Peace Corps), to care for a child or family member (Nevill and Chen, 2007), to pursue full-time employment to support family finances (Nevill and Chen, 2007), or to work to save money to pay for tuition and fees (Cochran et al., 2017). These reasons for leaking from the pipeline potentially have very different effects on education and career trajectories, yet they are indistinguishable in a pipeline model.
Despite these important criticisms, scholarship and interventions designed to improve representation in STEM fields have continued to use the pipeline metaphor in describing the target of their efforts, and we find the metaphor to be analytically useful. Specifically, investigating the degree to which students complete each step in the pipeline and transition between steps allows STEM education researchers to quantify inequalities in representation and participation and to identify targeted points for intervention.
Outcomes and Scope of Prior Pipeline Intervention Evaluations
Pipeline interventions tend to focus on a single point in the pipeline (e.g., providing research experiences to undergraduate science students; Lopatto, 2004), on a single transition point in the pipeline (e.g., STEM bachelor’s enrollment to completion; Eagan et al., 2010), or on a relatively narrow range of transition points (e.g., PhD completion or postdoc experience to workforce entry; Gibbs et al., 2014). Pipeline intervention outcomes that are often measured for undergraduates include grade point average (GPA; Toven-Lindsey et al., 2015), self-efficacy and science identity (Findley-Van Nostrand and Pollenz, 2017), degree completion (Eagan et al., 2010), interest in or intention to enroll in a STEM graduate degree program (Lopatto, 2004), matriculation to a STEM graduate program (Doerschuk et al., 2016; Ford et al., 2016), and intention to pursue a science research career (Schultz et al., 2011; Hernandez et al., 2017). In our literature review, we noted that studies that measure progression beyond the undergraduate level are much less common than studies that measure intention to progress beyond the undergraduate level.
Pipeline intervention outcomes that have been measured for PhD completers and postdocs include knowledge of career options and clarity about career pathways (Gibbs et al., 2015), interest in faculty careers (Gibbs et al., 2014), interest in non-research careers (Gibbs et al., 2014), and entering faculty positions (Rybarczyk, 2016; Eisen and Eaton, 2017). Studies that report late-pipeline outcomes tend to focus exclusively on the terminal stages of the pipeline rather than, for example, tracking students into these stages from earlier points. One exception to this is Thompson et al. (2019), a study of another Comprehensive Partnerships in Advancing Cancer Health Equity (CPACHE) site. Those authors reported that nearly 80% of former program participants who have entered the workforce (n = 170) are employed in the biomedical sciences or in positions related to biomedical sciences (Thompson et al., 2019, p. 7). This figure includes former undergraduate as well as graduate participants, hence the study measures progression into the workforce from both early and late pipeline points. Thompson et al. also report graduate degree completion rates for both undergraduate and graduate students, but some of these rates exceed 100% and are therefore difficult to interpret.
Studies that focus on one point (or a relatively narrow range of points) in the pipeline are less able to assess the longer-term impacts of programs relative to those that are more expansive in scope. Similarly, studies that measure intention to progress in the pipeline do not assess actual behavior, which is important for many aspects of evaluation. In this study, we assessed the long-term outcomes of the Meharry-Vanderbilt-TSU Cancer Partnership’s (MVTCP) research education initiatives with respect to their impact on the cancer research education pipeline. The MVTCP supports research education at the high school, undergraduate, master’s, professional (e.g., MD, DDS), doctoral (PhD), and postdoctoral levels and has tracked students throughout its 20-year existence. The design and longevity of the MVTCP program are unusual for a pipeline intervention program and provided the opportunity to 1) measure trainees’ progression along the entire postsecondary education pipeline, 2) measure their success within programs, and 3) measure their success between programs. Measuring actual progression rather than intention to progress is a strength of our design.
Research Questions
This study was motivated by three research questions related to the longitudinal education and career outcomes of MVTCP participants:
How do MVTCP participants’ transitions along the education pipeline compare with those of students in similar degree programs, especially at key transition points defined by 1) earning degrees and 2) enrolling in advanced degree programs?
At which points along the pipeline are participants prone to exit rather than progress? What proportion of these exits are permanent and what proportion are temporary?
What proportion of MVTCP participants choose careers related to cancer research?
METHODS
This study relied on administrative data combined with data obtained from public sources (e.g., LinkedIn). Accordingly, it was granted a non-research determination through the Vanderbilt University Institutional Review Board.
Description of Program and Recruitment
The MVTCP is the longest-running CPACHE program site in the United States. CPACHEs are supported through U54 cooperative grants and P20 grants administered by the NCI and are designed to improve cancer health equity by recruiting UR students and faculty into cancer research. The goals of the CPACHE program are to increase the cancer research and cancer research education capacity of institutions serving underserved health disparity populations and UR students, to increase the number of UR students and investigators engaged in cancer research, to improve cancer center effectiveness in developing and sustaining research programs focused on cancer health disparities, to increase the number of investigators and students conducting cancer health disparities research, and to develop and implement cancer-related activities that benefit the surrounding underserved communities (NCI, n.d.). Despite the CPACHE program requirement that all sites allocate substantial resources to cancer research education, relatively little has been published on the education-specific outcomes of these sites (Behar-Horenstein et al., 2020)
Meharry Medical College (hereafter Meharry), a historically Black university (HBCU), and the Vanderbilt Ingram Cancer Center, a comprehensive cancer center at a predominantly White institution, were selected as a CPACHE seed site in 2001 and have remained continuously funded since that time. For the first two cycles (i.e., 10 years) of the grant, the Meharry-Vanderbilt site supported a limited number of MD and PhD students whose outcomes have been reported elsewhere (Adunyah et al., 2010). In 2011, the site incorporated a second Nashville-based HBCU, Tennessee State University (TSU), thus becoming the Meharry-Vanderbilt-TSU Cancer Partnership (MVTCP). The program currently supports students at each level of the education pipeline from high school to medical residency training and/or postdoctoral fellowships. Moreover, the site prioritizes the following participants: students from two local high schools that primarily serve UR students, undergraduate students from TSU, master’s students from all three institutions, professional doctorate students (e.g., MD, MD/PhD) from Vanderbilt and Meharry, and PhD students from TSU and Meharry.
Each institution has somewhat different methods for recruiting and admitting students to participate in their MVTCP-supported cancer research opportunities. In general, however, institutions conduct outreach to all eligible students at each stage of the process (e.g., flyers sent home with high school juniors and seniors enrolled in science courses; announcements made to all medical students entering research rotations), and eligible students are invited to apply. Students are assessed based on overall GPA, science GPA, and a statement of interest. High school and undergraduate students are also required to submit letters of recommendation. Selection criteria include academic talent and interest in cancer research. Sites do not necessarily prioritize students who already have experience with research or a background in cancer-related work. Acceptance rates range from highly selective (∼5% for high school students) to somewhat more generous (50–60% for medical students). All participants receive structured research experiences that are commensurate with their levels of education. High school and undergraduate students receive additional support in the form of summer research experiences, communities of practice, workshops grounded in social cognitive career theory, and mentoring by a cancer researcher at one of the three MVTCP institutions.
Importantly, the partnership among the three institutions creates an environment in which students have access to the complementary strengths and resources of each institution. Furthermore, each institution is simultaneously pursuing other opportunities aimed at supporting UR students, and these opportunities are leveraged to further the goals of the MVTCP. To provide two examples, Meharry is a longtime awardee of the National Institute on Minority Health and Health Disparities’ Research Centers in Minority Institutions funding, which it has used to expand its basic science infrastructure, lab space, instrumentation, and faculty expertise, as well as robust community engagement resources and strategies. Investment in the research and educational environment leads to better outcomes for all students, including those supported through the MVTCP. Likewise, Vanderbilt has ongoing and successful efforts to increase the number of UR graduate students at Vanderbilt, making it one of the top producers of Black PhD-level scientists in the country. Although Vanderbilt doctoral students are not eligible for direct MVTCP support, expanded racial diversity among doctoral students and corollary commitments among the principal investigators in their labs means, among other things, that MVTCP-supported high school and undergraduate students who are placed in these labs experience more racial congruence with their teachers and mentors. Full descriptions of the partnership model can be found elsewhere (Adunyah et al., 2010; Pal et al., 2022).
Reconstruction of Missing Values
Participant data before 2019 contained a substantial number of missing values across primary variables of interest, including most demographic variables. For example, race was initially missing for 43.9% of participants, gender was missing for 39.5%, current position was missing for 88.3%, and program entry year was missing for 31.8%. The first author (M.L.M.) reconstructed missing participant data (to the extent possible) using institutional records to identify race and gender, grant reports to identify year of entry into the program, and web sites like LinkedIn, Google, and Doximity to identify current positions. Recognizing that some information found online would not be up-to-date, attempts were made to triangulate information found online using multiple online sources. The percentage of missing values in the initially reconstructed data ranged from 7.6% (program entry year) to 30.5% (ethnicity). The first and second authors (M.L.M. and S.V.S.) subsequently conducted a second round of reconstruction in which former participants and/or their MVTCP mentors were emailed and asked for specific information. Missing data for program-related variables (e.g., start year) and current workforce positions were obtained for all participants during the second round of reconstruction.
Missing values for race, gender, and ethnicity that could not be reconstructed were imputed with multiple imputation using the MICE package in R v. 4.0.4. Multiple imputation is appropriate when data are missing at random (MAR; Enders, 2010) and can produce accurate results even when the MAR assumption is tenable (Enders, 2017). A definitive test of MAR is not possible, but we used a series of chi-square tests to test for significant relationships between observed, nonmissing variables and each of the variables with missing values (i.e., race, gender, and ethnicity). In each case, one or more significant relationships were found (p < 0.01), suggesting at least a partially systematic relationship between measured variables and the probability of missing values for race, gender, and ethnicity. A total of 20 imputed data sets were generated based on the guidelines for selecting imputation numbers in Graham et al. (2007). Imputed values for race were generated with a polytomous regression function; values for gender and ethnicity were generated with a logistic regression function.
Description of Participants
Since 2011, 204 participants have been recruited to the MVTCP program. The number of students who have participated over time and overall program growth are shown in Figure 1. The decline in 2020–2021 can be explained primarily by COVID-related lab closures as well as the cutoff point for data that were included in our analysis (early 2021).

FIGURE 1. Number of participants recruited annually over time.
Demographic data were collected with voluntary preparticipation surveys. When self-reporting race, participants could choose not to answer the question or select one or more of the following categories: American Indian or Alaskan Native, Asian, Black or African American, White, and other. A total of eight participants self-reported more than one race and were combined into the single category “multiracial.” When self-reporting Hispanic/Latino/Latina identity (i.e., ethnicity), participants could choose not to answer or select “yes” or “no.” When self-reporting gender, participants could choose not to answer or select “female” or “male.” For the 189 participants who self-reported both race and gender, demographic characteristics are summarized in Table 1.
Meharry | TSU | Vanderbilt | Total: n (%) | ||
---|---|---|---|---|---|
Female | AsianBlack or African AmericanMultiracialOtherWhite (Hispanic/Latinx)White (non-Hispanic/Latinx)Subtotal: | 142101247 | 048421762 | 311001015 | 4 (3%)91 (73%)6 (5%)2 (2%)2 (2%)19 (15%)124 (66%) |
Male | AsianBlack or African AmericanMultiracialOtherWhite (Hispanic/Latinx)White (non-Hispanic/Latinx)Subtotal: | 729010643 | 26100312 | 11101610 | 10 (15%)36 (55%)2 (3%)1 (2%)1 (2%)15 (23%)65 (34%) |
Institution and sample totals: | 90 | 74 | 25 | 189 |
Table 2 shows counts and percentages of participants by type of degree in progress upon entry to the program; undergraduate participants are further categorized by field of study. Overall, 7.8% of participants began as high school students, 26.0% as undergraduates, 3.9% as master’s students, 33.8% as professional doctorate students, 17.6% as research doctorate students, 9.3% as postdoctoral researchers, and 1.5% as medical residents. The majority of participants (94%) who entered as professional doctorate students were enrolled in MD programs.
Meharry | TSU | Vanderbilt | |
---|---|---|---|
High school | NA | 16 | NA |
Undergraduate | NA | 53 | NA |
Biology/biological sciences | NA | 17 | NA |
Biochemistry/chemistry | NA | 13 | NA |
Biology and chemistry | NA | 4 | NA |
Unknown | NA | 19 | NA |
Master’s | 0 | 7 | 1 |
Professional doctorate | 53 | 0 | 16 |
Research doctorate | 25 | 9 | 2 |
Resident | 1 | NA | 2 |
Postdoc | 12 | 0 | 7 |
Total | 91 | 85 | 28 |
Classifying Participant Outcomes
At the broadest level of classification, participants fall into two categories: those still in the education pipeline and those who have left the pipeline and entered the workforce. To answer our third research question, “What proportion of MVTCP participants choose careers related to cancer research?,” we needed to categorize participants’ positions in the workforce. Because there is no standardized definition of “a career in cancer research,” two authors (D.R.M. and M.M.W.) independently coded the positions of participants who progressed through the pipeline in its entirety before entering the workforce (n = 38) using the following scale: “strongly related to cancer research,” “somewhat related to cancer research,” “not related to cancer research,” and “don’t know.” The authors agreed on 16 out of 30 positions for which they both provided a rating (Cohen’s kappa = 0.527). Although this value suggests only moderate agreement, it is worth noting that most disagreements arose from one rater using “don’t know” much more frequently than the other (in 13 out of 30 vs. 2 out of 30 cases). To settle these “disagreements,” a third author (S.V.S.) rated the positions and effectively served as a tiebreaker. Positions deemed “strongly related to cancer research” included assistant professors of oncology/hematology, medical liaisons of oncology/hematology, and program directors at the NCI. Positions deemed “somewhat related to cancer research” included pharmacologists and project coordinators at agencies that conduct some cancer research. Positions deemed unrelated to cancer research included senior scientists at organizations that do not conduct cancer research and secondary education teachers.
For participants who entered the workforce directly after completing bachelor’s degrees (n = 4), master’s degrees (n = 5), professional doctorates (n = 4), and PhDs (n = 3), consensus on workforce categorization was reached through discussion alone. Positions deemed “strongly related to cancer research” included clinical research associates at facilities that conduct cancer research and program management positions at cancer centers. Positions deemed “somewhat related to cancer research” included clinical lab assistants at facilities that conduct some cancer research and program directors at foundations associated with cancer research. Positions deemed unrelated to cancer research included dentists, pharmacists, and university administrators.
STATE TRANSITION MODEL
State transition models (STMs) are widely used in public health and disease management programs (Siebert et al., 2012), in part because of their ability to represent outcomes as a set of states between which transitions may occur as a condition of time or some other variable. While STMs are frequently probabilistic, they may also be descriptive (i.e., based on observed outcomes). We chose to use a descriptive STM for the MVTCP program evaluation, which allowed us to quantify outcomes across a related sequence of states (e.g., across the postsecondary education pipeline) as well as into a “final” state (e.g., performing work that is or is not related to cancer research). The model aggregates information about individual participants’ progression through these states into program-level transition rates between each set of adjacent states. Transition rates are valuable longitudinal evaluation statistics for the following reasons:
Several transition points in the pipeline are recognized educational milestones (e.g., the transition from enrolling in a bachelor’s degree program to completing a bachelor’s degree). When aggregated across participants, these data provide important program-level information, namely degree completion rates for participants. Degree completion rates for other samples of students are frequently reported by the U.S. Department of Education, the NSF, and other stakeholders in higher education. As one measure of program efficacy, we compare MVTCP participants’ degree completion rates with those of select samples from these publicly available sources.
Not all participants eventually earn advanced degrees or choose careers in cancer research. Relatively low transition rates between adjacent states point to precise locations of program-specific pipeline “leak points” and “delay points.” These points, in turn, indicate key areas for evaluators to explore further with qualitative and/or mixed-methods research.
Relatively high transition rates between adjacent states indicate areas where the program is effective at supporting and preparing students for further study or workforce entry. These also indicate key areas for evaluators to explore, as findings may be transferable to similar programs or other CPACHE sites.
“Delay points” are a descriptive concept that, to the best of our knowledge, has not been used in the empirical literature on pathways through higher education. An education pipeline “delay point” is an interim point in the pipeline where students are prone to experience delays in progression. A delay can occur while a student is enrolled in a program (i.e., needing more time than expected to complete a degree) or between programs (i.e., “time off” between completion of one degree and enrollment in another). The latter type of delay can be distinguished from leaking only as a function of time: A temporary exit or hiatus from the pipeline is a delay, while a permanent exit is a leak. Both can be observed and distinguished in a sufficiently longitudinal analysis like this one.
Figure 2 shows a diagram of the MVTCP state transition model. Boxes with solid borders are the set of mutually exclusive and exhaustive states that “typical case” MVTCP participants can occupy at a given time; boxes with dashed borders are pipeline entry points; horizontal blue arrows represent the ability to transition between states in the indicated direction; red arrows represent points where students leave the pipeline; bold values are computed transition rates; circled numbers are counts of participants who currently occupy a state and are not yet deemed able to have progressed to the next sequential state.

FIGURE 2. State transition model diagram.
Model Formulation
Transitions rates are defined for all states as:
where whether a participant “could have progressed” into the state beyond her current state is a function of how long she has been in her current state. For example, participants were allowed 5 years to complete a bachelor’s degree; if a student was observed to be enrolled in a bachelor’s degree program for more than 5 years, she was deemed someone who could have progressed into the next state of “completed bachelor’s degree.” In this example, the participant’s lack of progression would contribute +1 to the denominator of the transition rate between “enroll in STEM bachelor’s program” and “complete STEM bachelor’s program.”
Participants were allowed 2 years to complete high school, 5 years to complete bachelor’s degrees, 3 years to complete master’s degrees, 5 years to complete MDs, 5 years to complete DDSs, 6 years to complete PhDs, 10 years to complete MD/PhDs, 12 years to complete DDS/PhDs, 8 years to complete residencies (or residencies followed by fellowships), and 5 years to complete postdoctoral training. Except for the allowances for MD/PhD and high school students, each value incorporates a 1-year buffer beyond the minimum amount of time generally needed to complete the degree at MVTCP institutions.
Degree completion allowances are not based on the year that participants began their individual degree programs, though this would have been preferred. Neither our original nor our reconstructed data contain the beginning and ending enrollment year for each degree earned by each participant, many of whom have completed multiple degrees since their involvement in the program. As a proxy for the year that participants began the degree with which they entered the program, we used the year that participants entered the program itself, which is known for all participants. Participants’ initial degree completion allowances were added to this known time point; as participants entered subsequent degree programs, additional time was added to the same time point. For example, if an undergraduate student was recruited to the program in 2015, she would be allowed five years (i.e., until 2020) to complete this initial degree; if she was not observed to complete this degree by 2021, she would be deemed someone who “could have transitioned” to the next state, and her lack of transition would be reflected with a decrement to the transition rate between entering and completing bachelor’s degrees. If the participant completed a bachelor’s degree and then entered an MD program, she would be allowed until 2025 to finish the MD (2015 + 5 years for bachelor’s completion + 5 years for MD completion); if she had not finished the MD program by 2026, her lack of progression would be reflected as a decrement to the transition rate between entering and completing MDs.
This method of determining when each participant is considered able to have transitioned beyond her current state means that some participants are permitted more than the stated time allowances to transition. For example, if an undergraduate entered the program as a sophomore rather than as a freshman in 2015, she would still be allowed 5 years to complete her degree, despite being likely to graduate by 2019. If this participant ultimately did not finish her bachelor’s degree, her lack of progress would not be reflected as a decrement to the transition rate between entering and completing a bachelor’s degree until 2021 regardless of when she effectively left her program. This lack of precision affects only the denominator of the transition rates and applies only to cases in which participants entered the MVTCP program with advanced standing in their individual degree programs (e.g., as second-, third-, or fourth-year students).
Selection of Comparison Data
As one measure of program efficacy, we compare MVTCP participants’ degree completion and enrollment rates to those of select samples from publicly available sources. Given the number of different types of degrees earned by participants, no single source of comparison data was sufficient to meet our purposes. Instead, we carefully chose comparison data from reputable sources, prioritizing information that is 1) based on student populations or nationally representative samples and 2) recently collected. The most common sources of comparison data for degree completion rates are studies completed or funded by the NSF and the National Institutes of Health (Eagan et al., 2010) or the Council of Graduate Schools (Sowell et al., 2015; Council of Graduate Schools, 2019). The most common source of comparison data for degree enrollment rates is the NCES. Data that most closely resemble the fields of study chosen by MVTCP participants were selected as comparison points. Finally, residency “match” rates were used to approximate residency “entry” rates, because MD students generally have one opportunity per year to match and are thus assumed to enter residency assignments if matched. For only one group of participants—postdocs—were we unable to find relevant comparison data.
Comparison data are not used to infer estimates of program effects but as descriptive benchmarks for participants’ outcomes. We compute and describe the differences between participant outcomes and each comparison data point, including differences by race or gender in cases where granular comparison data by race or gender were available. Limitations of this method are discussed in Lackof Control Group. Though they do not produce causal estimates of program effectiveness, benchmark descriptors are nevertheless inherently useful for program evaluation.
RESULTS
Degree and Training Program Completion Rates
The education pipeline shown in Figure 2 contains six “completion” states: complete bachelor’s, complete master’s, complete professional doctorate, complete PhD, complete residency (or residency followed by fellowship), and complete postdoctoral training. Each of these completion states has an associated transition rate that describes MVTCP participants’ progression into the associated state. In other words, these six transition rates are synonymous with completion rates for the type of degree or training experience they describe.
The answers to our first research question, “How do MVTCP participants’ transitions along the pipeline compare with those of others, especially at key points defined by earning degrees?,” are mapped in Table 3, with MVTCP participants’ degree completion rates in the left column and comparison data in the right column.
MVTCP participants | Comparison data |
---|---|
STEM bachelor’s degree completion rate: 100% | Life sciences bachelor’s degree completion rate (Eagan et al., 2010, p. 14):a 36.8% (6-year rate)Physical sciences bachelor’s degree completion rate (Eagan et al., 2010, p. 14):a 22.9% (6-year rate) |
STEM master’s degree completion rate: 100% | Biological and agricultural sciences master’s degree completion rate (Council of Graduate Schools, 2019, p. 22):b 62% (3-year rate), 69% (4-year rate) |
Professional doctorate completion rate: 98% | MD completion rate (AAMC, 2014, p. 1):c 94.1% (5-year rate) |
STEM PhD completion rate: 96% | Life sciences PhD completion rate (Council of Graduate Schools, et al., 2008, p. 17):d 53.7% (7-year rate), 62.9% (10-year rate) |
Residency completion rate: 100% | Internal medicine residency completion rate (Lipner et al., 2016, p. 378):e 95% |
Postdoc completion rate: 100% | Postdoc completion rate: Unknown |
The left column of Table 3 shows nearly perfect completion rates for MVTCP participants. Compared with values in the right column, MVTCP completion rates are striking, especially for undergraduate, master’s, and PhD students. While 100% of MVTCP undergraduates complete their degrees, only around one-third and one-fifth of a nationally representative sample of undergraduates in life and physical sciences programs finish these degrees (36.8% and 22.9%, respectively; Eagan et al., 2010, p. 14). MVTCP participants who enroll in PhD programs complete their degrees at a much higher rate (96%) than other students in doctoral life sciences programs (62.9%); MVTCP students who enroll in master’s program also complete their degrees at substantially higher rates (100%) than other master’s students in similar programs (69%). Ninety-eight percent of MVTCP professional doctoral students and 100% of medical residents complete their programs, rates that exceed the comparison data by four and five percentage points, respectively.
Degree and Training Program Completion Rates by Race and Gender.
Degree completion rates of nearly 100% across the sample imply degree completion rates of nearly 100% for UR and female students within the sample. These can be compared with the following data for other UR students:
24.7% (6-year STEM bachelor’s completion rate; Eagan et al., 2010, p. 18)
51.5% and 57.5% (3- and 4-year STEM master’s completion rates; calculated based on data in Council of Graduate Schools, 2019)
79.2% (5-year MD completion rate; Smedley et al., 2001, p. 333)
52 and 63% (7- and 10-year life sciences PhD completion rates; Sowell et al., 2015, pp. 15, 22)
38.1% (6-year STEM bachelor’s completion rate; Eagan et al., 2010, p. 19)
63 and 69% (3- and 4-year STEM master’s completion rates; Council of Graduate Schools, 2019, p. 22)
∼91.3% (5-year MD completion rate; Association of American Medical Colleges, 2007, p. 1)
48 and 56% (7- and 10-year life sciences PhD completion rates; Council of Graduate Schools, 2008, p. 63)
MVTCP UR and/or female participants clearly outperform students of similar demographic characteristics with regard to completing degrees. We note, however, that the most demographically relevant comparison group for most MVTCP participants is not “UR or female” but “UR and female,” as most participants identify as both UR by race and as female. We discuss this limitation in Results Do Not Explore the Intersection of Race and Gender.
Degree and Training Program Enrollment Rates
The pipeline shown in Figure 2 contains eight “entry” states, all following completion of a prior degree. The eight entry states are: enter STEM bachelor’s, enter STEM master’s, enter professional doctorate (directly from undergraduate), enter STEM PhD (directly from undergraduate), enter professional doctorate (from master’s), enter STEM PhD (from master’s), enter residency, and enter postdoctoral training. We followed the NCES taxonomic system for fields of study when determining which programs to consider STEM, and only one participant (a high school participant who majored in finance as an undergraduate) failed to progress based on not having enrolled in a STEM program. Progression into each “entry” state has an associated transition rate that describes MVTCP participants’ progression through the associated section of the pipeline. In other words, these eight transition rates are synonymous with program-level “enrollment” rates for the type of degree or training experience they describe.
Table 4 provides answers to part 2 of our initial research question, “How do MVTCP participants’ transitions along the pipeline compare with those of comparison data, especially at key points defined by enrolling in advanced degrees?”
MVTCP participants | Comparison data |
---|---|
STEM bachelor’s enrollment rate: All participants: 94% | Intention to enroll in Science and Engineering bachelor’s degree (National Science Board, 2016, Appendix Table 2-16):a All: 44.6% |
STEM master’s enrollment rate: All participants: 31% | Master’s enrollment rate after completing biological or physical sciences bachelor’s degree (NCES, 2013):a All: 25.1% |
Professional doctorate enrollment rate (after bachelor’s completion): All participants: 31% | Professional doctorate enrollment rate after completing biological or physical sciences bachelor’s degree (NCES, 2013):a All: 17.2% |
PhD enrollment rate (after bachelor’s completion): All participants: 25% | PhD enrollment rate after completing biological or physical sciences bachelor’s degree (NCES, 2013):a All: 9.7% |
Residency entry (i.e., “match”) rate: All participants: 96% | Residency entry (i.e., “match”) rate (National Resident Matching Program, 2019):b All: 94.9% |
Postdoc entry rate: All participants: 100% | Postdoc entry rate: Unknown |
MVTCP participants enrollment/entry rates exceed those of all comparison data except MD completers entering residency programs, in which case the difference is approximately one percentage point. Most striking are the differences between MVTCP and comparison data enrollment in MD and PhD programs. Enrollment in either of these advanced degree programs is uncommon in the comparison data (17.2% + 9.7% = 26.9%). Among MVTCP participants, enrollment in an MD or PhD program following bachelor’s completion occurs in the majority of cases (31% + 25% = 56%). More precisely, participants enroll in MD programs at nearly twice the rate (31%) of comparison data (17.2%) and enroll in PhD programs at more than twice the rate (25%) of comparison data (9.7%). It is also notable that the program produces no fewer master’s enrollees than the national average despite these remarkably high MD and PhD enrollment rates. Altogether, MVTCP participants who complete bachelor’s degrees go on to enroll in some kind of graduate program at a rate of 87% (31% + 25% + 31% = 87%).
Degree and Training Program Enrollment Rates by Race and Gender.
As shown in Table 5, UR participants enroll in subsequent education/training programs at rates that are higher than or equal to WR participants at five out of ten points along the pipeline; female participants transition at rates that are higher than or equal to male participants at almost every point along the pipeline. The overall trend of Table 5 suggests that MVTCP participants who identify as UR and/or female transition from completing degree programs into subsequent degree and training programs more frequently than they exit at every point in the pipeline except for those who complete master’s degrees.
UR enrollment rate | WR enrollment rate | Female enrollment rate | Male enrollment rate | |
---|---|---|---|---|
Bachelor’s (from high school) | 100% | 75% | 100% | 67% |
Overall graduate school enrollment (from bachelor’s) | 92% | 66% | 93% | 60% |
Master’s (from bachelor’s) | 38% | 0% | 37% | 0% |
Professional doctorate (from bachelor’s) | 31% | 33% | 30% | 40% |
PhD (from bachelor’s) | 23% | 33% | 26% | 20% |
Overall graduate school enrollment (from master’s) | 33% | 50% | 43% | 0% |
Professional doctorate (from master’s) | 0% | 0% | 0% | 0% |
PhD (from master’s) | 33% | 50% | 43% | 0% |
Residency (from MD) | 95% | 100% | 96% | 96% |
Postdoc (from PhD) | 100% | 100% | 100% | 100% |
Pipeline Exits after Completing Degrees
A partial answer to our second research question, “Where along the pipeline are participants prone to exit rather than progress?” is contained in the results for degree completion rates: completion rates of 96–100% indicate that participants are not prone to exit the pipeline while enrolled in their degree programs (i.e., they do not switch to non-STEM programs or “drop out”). An additional answer is contained in Figure 2. More specifically, when MVTCP participants do leave the pipeline, it is in one of two places: after completing bachelor’s degrees and (much more frequently) after completing master’s degrees. Exits that occur between degree programs are calculated with enrollment rates: Subtracting the observed enrollment rate for a degree program from 100% quantifies the percentage of participants who fail to enroll in the program (i.e., who exit the pipeline immediately after completing the degree associated with the prior state).
After completing bachelor’s degrees, participants enroll in master’s programs at a rate of 31%, in professional doctoral programs at a rate of 31%, and in PhD programs at a rate of 25%. These disaggregated enrollment rates sum to 87% hence, 13% (100%−87% = 13%) of bachelor’s completers leave the pipeline after earning bachelor’s degrees.
After completing master’s degrees, participants enroll in MD programs at a rate of 0% (i.e., no master’s completer has ever been observed to enroll in an MD program) and in PhD programs at a rate of 38%. The overall percentage of master’s completers who leave the pipeline after earning master’s degrees is thus 62% (100%−38% = 62%). This is the highest exit rate anywhere along the pipeline.
Pipeline “Leak Points” and “Delay Points.”
While pipeline exit points can be identified with transition rates, transition rates alone do not provide enough information to distinguish between two types of exit points: leak points and delay points or permanent versus temporary exits. (“Delay points,” as noted in Results, can also refer to delayed progression through a degree program, but as no MVTCP participant has ever been observed to complete a degree in more time than our comparison data suggest is reasonable, we focus below on the type of delay point characterized by “time off” between degrees.) Distinguishing leaks from delays requires a time-based definition. However, because permanent exits would be nearly impossible to observe in practice, a proxy-based definition is needed. To the best of our knowledge, no empirical definitions of education pipeline leaks and delays are currently available in the literature. We propose two new definitions below that allow us to answer part 2 of our second research question, “What proportion of pipeline exits are permanent and what proportion are temporary?”
As shown in Figure 2, 16 MVTCP participants have completed their bachelor’s degrees and not yet progressed into graduate programs. Four of these sixteen are deemed participants who “could have progressed” based on the definition provided in Model Formulation, while the remaining 12 are not yet deemed able to have progressed. For this part of the analysis, we sought to reclassify these participants’ lack of progression as “permanent” or “temporary.” To do so we analyzed the distribution of their graduation years, as these provide reasonable evidence of intentions to progress further in the pipeline eventually.
Gaps in the distribution of graduation years for non-progressors speak to empirical thresholds that may distinguish “permanent” exits from “temporary” ones. In other words, gaps for non-progressors indicate entire “cohorts” of participants who have all returned to the pipeline following an exit, or, less probably, who all remained in the pipeline, never exiting. The distribution of graduation years for non-progressors contains one such gap: participants who graduated between 2016 and 2017. We propose that this “gap” plausibly reflects a moderate-to-high rate of participants who exited and later re-entered the pipeline. We can thus examine the cohorts on either side of this gap as a way of distinguishing participants who are unlikely to re-enter the pipeline from those who will.
Participants on the far side of the gap are those who graduated between 2013 and 2015. We define these graduates as having “leaked” or (most likely) having left the pipeline permanently. A “leak” is thus defined as a continuous absence of 6+ years from the pipeline based on the length of time these participants have been absent from higher education (2021−2015 = 6). Few participants who have leaked from the pipeline are expected to re-enter. We base our definition of “delay points” on participants on the near side of the gap who graduated between 2018 and 2019. A “delay point” or temporary exit is thus one that is characterized by an absence of more than 2 but less than 6 years from the pipeline (2021−2019 = 2). A number of these participants are expected to re-enter the pipeline in time. Participants who have been absent from the pipeline fewer than 2 years are not yet considered to have exited according to the above criteria.
Applying our definitions of “leaks” and “delays” to the MVTCP analytic sample yields the following:
7% (3/44) of participants permanently left the pipeline after completing their bachelor’s degrees
14% (6/44) of participants temporarily left the pipeline after completing their bachelor’s degrees)
7% (1/14) of participants permanently left the pipeline after completing their master’s degrees
43% (6/14) of participants temporarily left the pipeline after completing their master’s degrees
Career Outcomes
The answer to our third and final research question, “What proportion of MVTCP participants choose careers related to cancer research?,” is summarized in Figure 3.
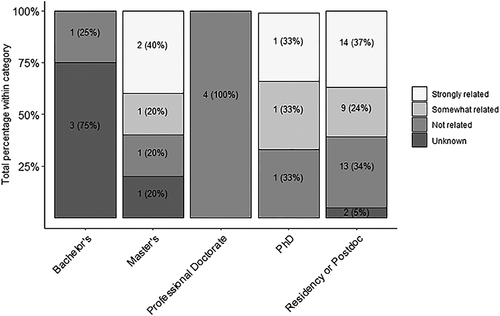
FIGURE 3. Classification of chosen careers by last degree or training program completed.
Among participants who progressed through the pipeline in its entirety (i.e., who completed residencies or postdocs before entering the workforce), the distribution of career outcomes is: 61% chose careers strongly or somewhat related to cancer research, 34% chose careers unrelated to cancer research, and 5% have careers that we are unable to categorize. Of those with cancer-research related careers, 74% are UR students and 61% are female.
Subgroups of participants who left the pipeline immediately after earning bachelor’s, master’s, professional doctorate, or PhD degrees are too small to interpret individually. However, among those whose current workforce positions are known, many—42% (five out of 12)—have chosen careers that are related or strongly related to cancer research. Master’s completers who do not go on to earn professional doctorates or PhDs make a special contribution to this value, as 75% (three out of four) whose workforce positions are known have opted for careers in cancer research. Participants who entered the workforce immediately after earning professional doctorates made the smallest contribution to this value, as none (zero out of four) has ever been observed to enter a career related to cancer research. (However, this result seems reasonable given that all four of these participants earned PharmD or DDS degrees.) Of the 42% of participants who chose cancer-research related careers immediately after earning their degrees, 83% are UR students and 75% are female.
DISCUSSION
Degree Completion and Enrollment Rates over Time
Regardless of the type of degree(s) they pursue during or after their involvement, MVTCP participants complete these degrees at rates of 96–100%. In Table 3, we map each MVTCP degree completion rate to a comparable benchmark rate represented by a large and/or nationally representative sample of students in similar degree programs. Results suggest that MVTCP participants complete their degrees at remarkably high rates at each step in the postsecondary pipeline. MVTCP bachelor’s completers also enroll in MD or PhD programs at remarkably high rates. As Table 4 indicates, just over half of bachelor’s completers (56%) enter one of these two types of programs, making matriculation into doctoral programs the program-level norm for bachelor’s completers. An additional 31% of bachelor’s completers enroll in master’s programs, a rate that exceeds the rate of similar students in a nationally representative sample. We posit that MVTCP participants’ unusually high degree completion and enrollment rates can potentially be explained in one of two ways: Either the program recruits participants who would be capable of these outcomes on their own, or, more likely, it recruits highly motivated and talented young scientists who are then provided with opportunities and environments that effectively reduce barriers to these outcomes. Given how otherwise uncommon such outcomes are, as suggested by multiple sources of comparison data, the latter explanation seems the most probable.
Another notable characteristic of MVTCP participants’ degree completion rates is observed by viewing Table 3 not as a series of side-by-side comparisons but as a comparison between columns. MVTCP degree completion rates are not only high but consistently high across the entire pipeline (i.e., from the top to the bottom of the column). Comparison data exhibit a much different trend, with low completion rates characterizing the early stages of the pipeline (i.e., top of the column) and moderate to high rates characterizing the terminal stages of the pipeline (i.e., bottom of the column).
These results may be conceptually related to the hypothesis that increasing the prevalence of UR students in the cancer research workforce depends on two necessary (but not sufficient) conditions: 1) increasing the proportion of UR students who are retained early in the pipeline and 2) increasing the proportion of UR students who progress through the pipeline. Consistently high degree completion and enrollment rates across the pipeline suggest that these conditions are already observed among the program population. Because the MVTCP program intervenes at multiple levels (e.g., individually focused cancer research experiences, transinstitutional investments in infrastructure to support cancer research education), additional research is needed to better understand how and why the MVTCP affects student outcomes. Further work will be facilitated by knowing the actual rates at which participants progress through the pipeline rather than the rates at which they intend(ed) to progress. In this way, our results contribute to the extant body of literature on pipeline interventions, especially interventions that measure observed outcomes.
We believe our results are an important contribution to the pipeline intervention evaluation literature not only as quantitative descriptions, but also because they capture a program-level trend across the pipeline in its entirety. That is, we believe our modeling technique itself is an important contribution to the literature on pipeline intervention evaluations. In contrast, our review of prior studies suggests that the majority of pipeline evaluations report outcomes for a single transition point (e.g., STEM bachelor’s degree completion).
Location and Duration of Pipeline Exits
Though we used program-level data and thus a relatively small number of observations to propose empirical definitions for “temporary” and “permanent” exits from the pipeline, these definitions are also supported in data from a nationally representative sample. The NCES Baccalaureate and Beyond (B&B) study followed a cohort of undergraduate seniors (n = 11,200) for 10 years after they earned their degrees and found that 39% had enrolled in master’s, professional doctorate, or research doctorate programs by the end of the study. Students who re-entered the pipeline had an average “gap” of 2.69 years (SD = 2.66) between bachelor’s completion and graduate school enrollment (NCES, 2003). Assuming these re-entry times were normally distributed, 49.5% would have occurred between 2 and 6 years after bachelor’s completion and thus would have been correctly classified as “temporary” exits using our proposed definition. In contrast, 10.8% of re-entries would have occurred between 6 and 10 years after graduation. These students would have been considered “permanent” exits at the time they re-entered the pipeline using our definition and hence would have been misclassified. In short, our proposed definitions of pipeline leak points and delay points may be externally valid for bachelor’s completers.
A significant difference between the B&B and MVTCP bachelor’s completers is that most of the B&B sample did not go on to enroll in graduate school, while the majority of MVTCP participants did. Hence, in retrospect, any observed exits among the B&B sample would best be predicted to be “permanent.” Among the MVTCP population, for whom graduate school matriculation is much more common than not, distinctions between permanent and temporary exits are likely to be more meaningful. For example, an intervention designed to facilitate pipeline retention for MVTCP bachelor’s completers might focus on minimizing time off between degrees, a goal that could be directly informed by distinguishing between temporary and permanent exits. The relevant target for such an intervention would be all participants who have been absent from the pipeline fewer than 6 years; the purpose would be to prevent these temporary exits from becoming permanent.
Overall, pipeline exits following master’s completion are much more common (62%) than exits following bachelor’s completion (13%). Indeed, exits following master’s degree completion are the highest of any along the pipeline. This suggests that the largest MVTCP-specific “obstacle” to pipeline progression is related either to entering professional doctorate and/or PhD programs following master’s completion or to the experience of earning a master’s degree itself. The former explanation would ostensibly be explained by the selectivity of professional doctorate and PhD programs, though this seems unlikely, given that master’s degrees should be an advantage to applicants. An alternative explanation is that master’s students become discouraged from pursuing MDs and PhDs while enrolled in their programs or are subsequently unwilling to take on additional debt; in both cases, lack of progression is related to the experience of earning a master’s degree. Yet another potential explanation is that low enrollment in professional doctorate and PhD programs following master’s completion is not an “obstacle” at all but rather a choice: participants who choose to enroll in master’s programs or who enter the program while completing these degrees could be doing so to build their résumés and/or to gain pre-workforce experience rather than to prepare for more advanced degree programs. These very different but plausible explanations point to the need for evaluators to explore this result further, as more substantive findings could inform the way that program experiences are structured to better serve master’s students and/or to promote greater pipeline retention.
Choosing Cancer Research Careers
The majority of MVTCP participants choose careers related to cancer research. Moreover, the majority of those who choose careers related to cancer research are UR and/or female, an outcome that contributes directly to the CPACHE goal of increasing the number of UR professionals in the cancer research workforce. Chosen cancer research careers include both “support” roles and direct investigation roles, adding racial and gender diversity across the cancer research workforce continuum in perhaps unexpected ways. Master’s completers who do not go on to earn professional doctorates or PhDs are nevertheless clearly motivated to pursue careers related to cancer research, which they are most likely to do via research support roles.
In addition to all of the results related to our research questions presented earlier, we noted several trends of interest that may be used to guide future studies. Briefly, most program participants are female despite relative gender parity at the institutions involved, leading us to wonder why the program is more successful at recruiting female than male students. Second, having quantified the rates at which participants do and do not progress into 1) advanced academic programs and 2) cancer research careers, we would like to explore the underlying reasons for these phenomena, as this information could inform future student and program success. Understanding the specific attributes of MVTCP research education experiences that contribute to student success will serve the same broad program goal. Finally, although we explored student entry into cancer-related professions, we did not explore the degree to which their work is focused on health disparities, another program goal. These observations all serve as potential directions for future research.
Limitations
This study has modest limitations, including lack of a control group for benchmarking program outcomes and difficulty directly comparing some outcomes to available comparison data. These limitations are addressed in the following sections.
Lack of Control Group.
Studies that report outcomes of research and/or STEM training programs for UR students are generally designed in one of three ways:
Participant outcomes are sometimes measured with pre and post surveys but essentially stand alone without reference to an external comparison group (e.g., Lopatto, 2004; Strayhorn, 2010; Doerschuk et al., 2016; Ford et al., 2016; Gotian et al., 2017; Thompson et al., 2019)
Outcomes are compared with an unmatched comparison group (e.g., Eisen and Eaton, 2017)
Outcomes are compared with a matched comparison group (e.g., Schultz et al., 2011; Thompson et al., 2016).
We chose the second type of design and, like Eisen and Eaton (2017), mostly compare participant outcomes to data aggregated across large samples. Problems with this design choice are obvious and previously noted by Lopatto (2004): The MVTCP program selects the most promising applicants to participate, and students who self-select into the applicant pool are likely to be more motivated to pursue advanced degrees and/or cancer research careers before participation. Selection effects may therefore underlie MVTCP participants’ success and create an upward-performance bias relative to the comparison data. We would like to note clearly that we are not using the comparison data to produce causal estimates of the program’s effects but rather to benchmark program outcomes more generally. General benchmarks, in turn, can serve multiple purposes in program evaluation. For example, large and positive outcome differences between participants and students in the comparison data suggest that the program is effective at selecting legitimately talented young scientists from the applicant pool. As selecting the “right” participants is an integral part of program performance, this information is inherently useful to us as evaluators.
Results Do Not Explore the Intersection of Race and Gender.
We do not report results for participants by race and gender (intersection) but rather by race and gender separately. Results calculated within levels of both variables would be based on small numbers of participants, particularly at the nonlinear transition points shown in Figure 2. Results reported for race or gender (e.g., Table 5) may thus be somewhat misleading, because all participants belong to both a racial and gender group, hence it is not clear from Table 5 whether race may confound the results for gender or vice versa. Future evaluations may include more granular demographic analysis of participants, especially over time as the number of program alumni grows.
CONCLUSION
This study makes contributions to the literature on research training programs for UR students as well as the literature on program evaluation. Results suggest that education and career outcomes for MVTCP participants differ substantially from the general student and workforce populations. For example, participants complete degrees at rates near 100%, they matriculate into advanced degree programs at remarkably high rates, and the majority decide to pursue careers in which they support or conduct cancer research. These outcomes, in turn, directly support CPACHE program goals related to diversifying the cancer research workforce. This study’s design leverages conceptual strengths of the pipeline metaphor for postsecondary education but has also sought to improve on this metaphor by distinguishing between permanent and temporary exits from the pipeline. Such distinctions may be useful for types of programs in which participants are expected to progress through a series of related states over an extended time horizon. While such programs certainly include research training programs for UR students, they are not necessarily limited to these.
ACKNOWLEDGMENTS
The authors would like to thank Beth Shinn and Kimberly Dahlman for detailed feedback on preliminary drafts, Jon Caulkins for modeling assistance, and two anonymous CBE–-Life Sciences Education reviewers for incisive feedback. We would also like to acknowledge Ann Richmond for her many years of leadership and advocacy for the MVTCP program as well as its students. We would like to thank the National Institutes of Health/National Cancer Institute for support of our Meharry-Vanderbilt-Tennessee State University Cancer Partnership (U54CA163066, U54CA163069, and U54CA163072).