Forming Groups in a Large-Enrollment Biology Class: Group Permanence Matters More than Group Size
Abstract
Active-learning pedagogies often require group work. We tested aspects of forming groups in a nonmajors Biology class. We asked whether large or small groups affected student learning outcomes and attitudes towards working in groups. We placed students in groups of three or six and students stayed in their groups for the term. We measured learning outcomes using a pre/postassessment as well as two-stage exams. Attitudes towards working in groups were measured using a previously published pre/post survey and an exit survey. We found that students in large groups did better on group exams and large groups had higher highest scores on the individual part of two-stage exams. Group size had no effect on students’ postassessment scores or attitudes towards working in groups. We next assigned students to permanent or nonpermanent groups. We used the same metrics as the group size experiment. Students in permanent groups had higher group exam scores and better attitudes towards working in groups. Group permanence had no effect on students’ postassessment scores. Students preferred working in permanent groups due to positive group interactions that developed over the quarter. Optimal group size and permanence are likely context-specific and dependent on the types of group work used in class.
INTRODUCTION
Active-learning pedagogies are increasingly being used in undergraduate science classes due to mounting evidence that active learning increases student achievement (Handelsman et al., 2004; Ruiz-Primo et al., 2011; Freeman et al., 2014). In a meta-analysis of 225 papers describing active learning in undergraduate classrooms, Freeman et al. (2014) found that students in classes using active learning have significantly better performance on tests compared with students in lecture-based classes (average effect size of 0.47), and significantly lower failure rates (22% compared with 34%). They also found that classes in which a larger fraction of class time was spent on active learning had larger effect sizes, suggesting that “more is better.” Their results prompted Freeman and his colleagues to suggest moving onto “second generational” studies that focus on comparing different active-learning techniques in order to determine which practices are most effective and the best way to implement them, and how much active learning needs to be implemented to produce positive results.
Active-learning pedagogies often have a social component, requiring students to engage with science concepts through interactions with their instructor and one or more peers. Students can work in temporary, informal groups for short periods of time such as when students discuss a question or concept with a neighbor during think-pair-share. In classes that incorporate multiple structured assignments throughout the term, students often work in formal groups that are larger than two students, more fixed, and longer lasting (Tanner et al., 2003; Hodges, 2018; Wilson et al., 2018). There is evidence that working in groups increases student achievement in college classrooms. In a meta-analysis of undergraduate science, math, engineering, and technology classes, Springer et al. (1999) found that students working in groups had greater learning gains, better attitudes toward learning, and increased persistence in class work compared with students in more traditional classes that lacked group work. Student learning is enhanced when groups are structured to be cooperative and student success depends on the success of others in the group (Johnson et al., 2014). In a study directly comparing working in groups to working individually, Linton et al. (2014) found that students who worked in cooperative groups performed better on higher-level exam questions compared with students who completed the same work individually. Strategies for structuring cooperation within groups include ensuring that student success is tied to group success, giving students time to discuss and exchange ideas, holding students accountable for their part in reaching group goals, defining cooperative behaviors to help students with the social aspects of group work, and allowing time for group reflection (Tanner et al., 2003). Task complexity is also important; tasks should be sufficiently complex to warrant group work (Kirschner et al., 2011; Scager et al., 2016)
While the importance of cooperative groups seems clear, there is less consensus about other aspects of forming formal groups. For example, there is conflicting evidence about whether groups that are homogeneous or heterogeneous for student ability are more effective, and the type of group that is best for a high-achieving student may be different than for a low-achieving student (Heller and Hollabaugh, 1991; Lou et al., 1996; Jenson and Lawson, 2011; Baer, 2003; Miller et al., 2012). In a meta-analysis of studies from elementary through postsecondary classrooms, Lou et al. (1996) concluded that forming homogenous groups by student ability was better overall, although the results were different for students of different ability: mid-ability students learned more in homogenous groups, while low-ability students learned more in heterogenous groups and there was no difference for high-ability students. We recently tested whether homogeneous or heterogeneous groups were more effective in our Introductory Biology course (Donovan et al., 2018). Using a pretest, we categorized students as low-, mid-, or high-performing in biology then assigned them to either homogeneous or heterogeneous competence groups. We found that low-competence students performed better on course exams and on a comprehensive postassessment when they were in heterogeneous groups (i.e., groups with higher competence peers), while mid- and high-competence students did equally well in homogeneous and heterogeneous groups. Students of all competence levels had better attitudes towards group work in heterogeneous groups. Therefore, heterogeneous competence groups appear to be most effective in our context.
There is also conflicting evidence for how big formal groups should be. Hunkeler and Sharp (1997) found that groups of four had higher grades than groups of three in a senior Engineering course. A meta-analysis conducted by Lou et al. (1996) found that group size significantly affected student learning, with smaller groups (three to four students) producing larger effect sizes than larger groups (five to seven students). However, a subsequent meta-analysis with a larger data set did not find a significant group size effect (Lou et al., 2000). Proponents of team-based learning think that groups should be relatively large (five to seven students) to provide for a diversity of learners with different perspectives (Michaelsen et al., 2014).
Study Rationale
In our class, we have historically assigned students to groups of six. This decision was based on group size recommendations for team-based learning (Michaelsen et al., 2014) as well as the constraints of teaching a large class in a lecture hall with fixed seating. Groups of six produce fewer total groups than groups of three or four, and students can still interact with one another by sitting in two groups of three in consecutive rows. However, we questioned whether smaller groups might be better for students because larger groups might allow some students to put in less effort than others, a phenomenon known as social loafing (Latané et al., 1979).
Little is known about whether permanent groups support better learning and attitudes about working in groups. Permanent groups are considered important for team-based learning (Michaelsen et al., 2014) and problem-based learning (Mazur, 1997) because it is thought that students need time to develop relationships that will lead to productive group work. McConnell (2006) structured permanent groups of computer science students, arguing that students need time to get to know each other to work effectively, although this was not formally tested in the classroom. Students in peer instruction classrooms, however, often engage in activities that have them work with whoever is sitting closest, which can enhance learning when compared with less-structured classrooms (Crouch and Mazur, 2001; Hodges, 2018). In a study directly testing permanent and nonpermanent groups, Zhang et al. (2017) found that students working in permanent groups in a peer instruction classroom had a greater shift toward expert-like attitudes about physics and learning about physics compared with students in changing groups, and especially when compared with the attitudes of students working independently in a traditional lecture course.
To add to the body of work assessing the impacts of group structure on student success and group dynamics, we conducted two experiments in a nonmajors Introductory Biology course. In the first experiment, we investigated the extent to which group size affects content acquisition and attitudes towards working in groups. We placed students in heterogeneous competence groups of three or six people and measured content acquisition using a pre/postassessment given to individual students and group exams. We measured attitudes towards working in groups using a previously published survey and exit polls. In the second experiment, we investigated the extent to which group permanence affects content acquisition and attitudes towards working in groups. We placed students in groups that either stayed together for the quarter or groups that changed twice, and we used the same measurement instruments as in the first experiment.
METHODS
Our study was approved by the Human Subjects Review Committee at Western Washington University (IRB# EX 18-127).
Study Context
Our study was conducted in several sections of a highly structured, large-enrollment (approximately 200 students), nonmajors Biology class. We used multiple student-centered pedagogies and students worked in instructor-formed groups that were heterogeneous for student performance because heterogeneous groups support better content acquisition for low-performing students in our class (Donovan et al., 2018). Students prepared for content modules (there were six in the 10-week class) by watching short online videos, completing reading assignments, filling out reading-watching guides, posting points of confusion to a discussion board, and taking a preclass quiz. Students sat in assigned seats with their groups when they were in class and engaged in activities such as group worksheets, jigsaws, modeling, ABCD questions, and just-in-time lectures covering the muddiest points to help students move to a higher level of content understanding (Allen and Tanner, 2005). After every two modules, students took a two-stage exam (Zipp, 2007; Gilley and Clarkston, 2014; Nicol and Selvaretnam, 2021) where students first answered exam questions individually, then as a group.
We conducted our experiment on group size in two sections of the class during Fall quarter 2018. The same instructor (G.L.C.) taught both sections. Before class, students were purposefully assigned to heterogeneous groups of three or six, using GPA and self-reported competence in biology to group them. We also balanced groups so that women and Black, Indigenous, and People of Color (BIPOC) students had allies in their group. The experimental group sizes were chosen because the fixed seating in our classroom allowed for a group of three to sit together in a row and a group of six to sit with three members in one row and three in the next row, allowing group members to turn around for group work. Both sections of the class had both small and large groups. There were 31 small groups and 16 large groups in each section, so the number of students in small and large groups was relatively equal (187 students in small groups and 177 students in large groups). Students were not told that there were different group sizes within the classroom, however they were likely aware of this. To minimize their awareness, we placed all small groups on one side of the classroom and all large groups on the other, with the sides switched in the two different sections. Students stayed in their groups for the duration of the quarter.
We conducted our experiment on group permanence during Fall quarter 2019 and winter quarter 2020. The same instructor (G.L.C.) taught the same nonmajors Biology class described above, and she taught two sections of the class each quarter. Before class, students were assigned to heterogeneous groups of six, using GPA and self-reported competence in biology to group them. Groups were balanced for gender and BIPOC status. In one section, students stayed in their groups for the entire quarter (permanent groups). In the other section, students changed groups after each module exam, resulting in students being members of three different groups during the quarter (nonpermanent groups). Students were carefully assigned to nonpermanent groups such that they worked with different people each time and such that the groups were heterogeneous for perceived competence each time. We replicated the experiment in two consecutive quarters to account for the different times of day the two sections were taught, such that the permanent and nonpermanent sections were each taught at both times. Data from the two quarters were combined for analysis.
Data Sources
Content Knowledge Measurement.
We measured student content acquisition in two different ways. We administered a pre- and postassessment, which consisted of 26 multiple choice questions modified from concept inventories (Klymkowsky & Garvin-Doxas, 2008; D’Avanzo et al., 2010; Nadelson and Southerland, 2010; Fischer et al., 2011), supplemented by 10 questions written by us to cover the range of concepts that would be addressed in the class, for a total of 36 questions (see Supp Mat Pre-assessment in Supplementary Materials). The students took the preassessment on the first day of class. These questions were then integrated into module exams (which also contained additional questions) throughout the quarter as a postassessment. When assessing content acquisition using pre/postassessments, it is common to administer a preassessment before a module and a postassessment after the module is taught (e.g., Knight et al. 2008, Hoffman et al. 2016, Booth et al. 2021). Due to time constraints, we administered all the questions at the beginning of the course, then the same questions pertaining to specific module content were administered as a postassessment at the end of the modules. These postassessment questions were supplemented by other questions for more comprehensive module exams (for each module exam, 13 questions were added to 12 postassessment questions to form a 25-question module exam). Module exams were taken after every two modules covering different material. They were two-stage exams, in which students took the exam individually for an individual score, then they took the same exam as a group for a group score. This provided us with group exam scores and the highest individual score within a group. Thus, we measured content acquisition two ways: comparing preassessment score with scores from only postassessment questions on module exams for each individual student (providing a single postassessment score) and comparing group exam scores from each of the three module exams.
Student Attitudes Measurement.
We used the student attitudes toward group environments (SAGE; Kouros & Abrami, 2006) to measure attitudes about group work. The SAGE is a five-point Likert survey that measures student attitudes about four constructs of working in groups: Quality of product and process (i.e., “When I work in a group I do better quality work.”), Peer support (i.e., “When I work in a group I am able to share my ideas.”), Student interdependence (i.e., “Everyone’s ideas are needed if we are going to be successful.”), and Frustration with group members (i.e., “I become frustrated when my group members do not understand the material.”). Students took the SAGE online at the beginning and then again at the end of the course.
We conducted a confirmatory factor analysis (CFA) to determine how well the SAGE constructs were measured in our student population. We used the pre-SAGE data from the group permanence study because the sample size was large enough to support a robust CFA (n = 586 students; Knekta et al. 2019). We performed a four-factor CFA because Kouros and Abrami (2006) had developed the instrument under a well-developed theoretical framework and had conducted an exploratory factor analysis on a population similar to ours (high school and college students). Before conducting the CFA, we analyzed our data to determine whether they were appropriate for CFA following the guidelines of Knekta et al. (2019). We then conducted a multigroup CFA and determined whether students in different treatments initially responded to SAGE questions in a similar manner by assessing measurement invariance between students in the two treatments (permanent groups and nonpermanent groups). Details of these analyses can be found in the Supplemental Material.
On the last day of the course, in addition to the SAGE, we administered an exit survey. In the group size experiment, the survey consisted of 11 questions about how students felt their group functioned, with a focus on aspects of group size (e.g., hearing and seeing group work, feeling included in group activities, and feeling accountable for coming to class). These questions were answered on a five-point Likert scale. In the group permanence experiment, students completed a self and peer evaluation, which included an open-ended question about preference for permanent or nonpermanent groups and reasons for that preference.
Student Demographics.
To determine whether students from different demographic groups were affected differently by group size or group permanence, we gathered demographic data for each student from the registrar. These data included binary gender (female or male, as designated by the only information provided by the registrar at the time of our study), BIPOC status (BIPOC students were designated as not Caucasian nor Asian, per the criteria used by our university for broader reporting), and first-generation status (neither parent completed a university degree, per the criteria used by our university for broader reporting). We recognize that binary gender does not capture all students, and regret that we were constrained by the information provided to us at the time. Due to the low number of BIPOC students in our study, and at our university in general, we did not try to investigate the intersectionality of demographic groups (i.e., BIPOC women). Overall GPA was also obtained for each student. In addition, we recorded student absences as a measure of student accountability.
Hypothesis Testing
Our research questions, null hypotheses, and full models used to test specific hypotheses are summarized in Table 1.
Research Question | Null Hypothesis | Initial Full Model |
---|---|---|
RQ1: Do students in large groups have different content acquisition compared with students in small groups? | H1a: No difference in postassessment scores between students in large and small groups. | post ~ pre + group size + GPA + 1|group + 1|section |
H1b: No difference in postassessments scores between women, BIPOC students, and/or first-generation students in large and small groups. | post ~ pre + group size * demographic + GPA + 1|group + 1|section | |
H1c: No difference in group module exam scores between students in large and small groups. | student group score ~ group size * exam number + GPA + 1|student ID + 1|group + 1|section | |
H1d: No difference in highest individual score within a group between large and small groups. | highest ind score ~ group size + exam + 1|section | |
H1e: No difference in group scores based on highest individual score within a group. | group score ~ highest ind score + exam + 1|section | |
RQ2: Do students in large groups have different attitudes towards working in groups compared with students in small groups? | H2a: No difference in attitudes towards working in groups between students in large and small groups. | Post-SAGE construct ~ pre-SAGE construct + group size + GPA + 1|group + 1|section |
H2b: No difference in attitudes towards working in groups of women, BIPOC students, and/or first generation students in large and small groups. | Post-SAGE construct ~ pre-SAGE construct + group size * demographic + GPA + 1|group + 1|section | |
RQ3: Do students in permanent groups have different content acquisition compared with students in nonpermanent groups? | H3a: No difference in postassessment scores between students in permanent and nonpermanent groups. | post ~ pre + group permanence + GPA + 1|group + 1|section |
H3b: No difference in postassessments scores between women, BIPOC students, and/or first generation students in permanent and nonpermanent groups. | post ~ pre + group permanence * demographic + GPA + 1|group + 1|section | |
H3c: No difference in group module exam scores between students in large and small groups. | student group score ~ group permanence * exam number + GPA + 1|student ID + 1|group + 1|section | |
H3d: No difference in highest individual score within a group between permanent and nonpermanent groups. | highest ind score ~ group permanence + exam + 1|section | |
H3e: No difference in group scores based on highest individual score within a group. | group score ~ highest ind score + exam + 1|section | |
RQ4: Do students in permanent groups have different attitudes towards working in groups compared with students in nonpermanent groups? | H4a: No difference in attitudes towards working in groups between students in permanent and nonpermanent groups. | Post-SAGE construct ~ pre-SAGE construct + group permanence + GPA + 1|group + 1|section |
H4b: No difference in attitudes towards working in groups of women, BIPOC students, and/or first generation students in permanent and nonpermanent groups. | Post-SAGE construct ~ pre-SAGE construct + group permanence * demographic + GPA + 1|group + 1|section |
Because we were interested in how individual students performed in the class, and because students in groups are not independent of each other, we used multilevel models (MLM) to test for differences in assessment scores and SAGE constructs between students in different types of groups (Theobald, 2018). As an overview of our analyses (specific models are described below and in Table 1), models of pre- and postassessment scores (36 questions) were estimated using the lmer function, with the lme4 package in R (Bates et al., 2015). Models of SAGE constructs were estimated using the clmm function, with the ordinal package in R (Christensen, 2018) to account for Likert-scale data generated by the SAGE survey (Theobald et al., 2019). To test whether the outcome variable of interest was affected by group type, we used a model selection procedure recommended by Zuur et al. (2009). Generally, we first fit the most complex model that only contained fixed effects and that tested the specific hypothesis of interest. Then we fit the same complex model separately with all combinations of the random effects, looking for the most parsimonious best-fitting model. After the most parsimonious random effects were determined, models containing those random effects were fitted and, using backwards model selection, we found and reported the most parsimonious model with random and fixed effects.
To examine whether group type (size or permanence, depending on the experiment) affected content acquisition using the pre- and postassessment data, we first modeled individual postassessment score as the dependent variable, with individual preassessment score, group type, and GPA as fixed effects. We included GPA to partially control for characteristics of students in different sections, as recommended by Theobald and Freeman (2014). With this starting model, we then modeled all combinations of random effects (section and group) to determine the most parsimonious random effects. When those were determined, we used backwards model selection to determine which fixed effects (individual preassessment score, group type, and GPA) remained in the most parsimonious model. This allowed us to test hypotheses H1a and H3a (Table 1).
To examine whether students from different demographic groups (binary gender, BIPOC status, and first-generation status) were affected differently by group type, we first modeled postassessment score as the dependent variable, with preassessment score, group type, the demographic variable, and GPA as fixed effects, including an interaction between group type and the demographic variable. We then used backwards model selection to determine which combinations of random effects and then fixed effects produced the most parsimonious model. This allowed us to test hypotheses H1b and H3b (Table 1).
To examine whether group type affected students’ group module exam scores, we first modeled each student’s group exam scores as the dependent variable, with group type, exam number, and GPA as fixed effects, including an interaction between group type and exam number. Because each student had multiple group exam scores (one for each exam), student ID was included as a random effect to account for the repeated measures. Group and section were also included as noninteracting random effects. We checked for a ceiling effect by evaluating the model using censored regression. This allowed us to test hypotheses H1c and H3c (Table 1).
To further examine the effect of group type on group module exam scores, we investigated whether groups of different types (large or permanent) had higher highest individual module exam scores within a group (the highest individual score in each group from the individual part of the two-stage exam), and whether the highest individual score predicted the group score. We first modeled the highest individual exam score as the dependent variable, with group type and exam as fixed effects. Section was included as a random effect in these models. We next examined whether the highest individual score in the group predicted the group exam score by modeling group exam score as the dependent variable, with highest individual score and exam as fixed effects. Section was also included as a random effect in these models. This allowed us to test hypotheses H1d, H1e, H3d, and H3e (Table 1).
To examine whether group type affected student attitudes about group work, we performed similar MLM analyses as those described above, with student SAGE responses as the variables of interest. Student SAGE responses from the beginning of the course, and then again from the end of the course, were summed for each construct of the survey, providing pre- and post scores for each construct. Constructs consisted of eight to 15 questions, each answered on a five-point Likert scale, so summed constructs ranged from nine to 74. Summed scores allowed us to compare results about attitudes towards working in groups from both studies because the SAGE was implemented the same way in both studies (Widaman and Revelle 2023). In addition, factor loadings from the CFA for the constructs indicated that using an unweighted score (a summed score as opposed to a score estimated by weighting responses by factor loadings) was reasonable (Supplemental Material). We conducted the model selection process as described above, using the post scores for each SAGE construct as the dependent variable and the pre score, group type, and GPA as fixed effects. Group and section were included as random effects. We also conducted analyses, similar to those above, to investigate whether students from different demographic groups had different attitudes about group size. This allowed us to test hypotheses H2a, H2b, H4a, and H4b (Table 1).
To analyze responses from the open-ended question about preference for permanent or nonpermanent groups in the group permanence experiment, three coders (G.L.C., D.A.D., and a student research assistant) first developed a coding scheme based on responses we thought students would give. All three of us then coded 20 student responses each from permanent and nonpermanent groups and revised our coding scheme based on actual student responses. D.A.D. and the student researcher next used the revised coding scheme to code 50 more responses from each group type and discussed them to reach consensus. The two of us then individually coded the rest of the responses and the final coding included 160 overlapping responses, which we used to calculate inter-rater reliability (Supplemental Table S1). This analysis informed our test of hypothesis H4a (Table 1).
RESULTS
SAGE CFA
The preSAGE data from the group permanence study were appropriate for CFA. In addition, the confirmatory factor analyses of the data indicated that the four SAGE constructs were modeled reasonably well in our student population and that the constructs were modeled similarly between students in permanent and nonpermanent groups. Details of these analyses can be found in the Supplemental Material. Because our data indicated good fit with the SAGE instrument and because there were no theoretical reasons to remove any of the items, we used the SAGE as originally developed in our experiments.
Group Size
In general, group size did not have a significant effect on students’ individual content postassessment score; group size was not retained in the most parsimonious model (Table 2). Group size was also not retained in the best-fit models of individual scores that tested for a disproportionate benefit of group size on BIPOC students, female students (binary gender), and first-generation students (Table 2; Supplemental Table S2). In short, group size did not impact individual content acquisition nor did students from minoritized groups in STEM appear to be affected differently by group size. Women and BIPOC students, however, performed worse on the postassessment compared with men and students who were not BIPOC (Table 2).
Best-fit model | Estimate ± SE | t or z value* | |
---|---|---|---|
Individual Content Assessment | post ∼ pre + GPA | ||
intercept | 4.85 ± 1.16 | 4.20 | |
preassessment | 0.58 ± 0.06 | 10.21 | |
GPA | 3.41 ± 0.32 | 10.53 | |
Gender | post ∼ pre + gender + GPA | ||
intercept | 4.51 ± 1.16 | 4.43 | |
preassessment | 0.56 ± 0.06 | 9.91 | |
gender (ref: female) | 0.93 ± 0.43 | 2.15 | |
GPA | 3.51 ± 0.32 | 10.89 | |
BIPOC status | post ∼ pre + BIPOC + GPA | ||
intercept | 5.12 ± 1.16 | 3.89 | |
preassessment | 0.58 ± 0.06 | 10.34 | |
BIPOC status (ref: not BIPOC) | −1.11 ± 0.50 | 2.22 | |
GPA | 3.38 ± 0.32 | 10.49 | |
Students’ Group Exam Scores | group score ∼ group size + exam + 1|group | ||
intercept | 85.85 ± 1.47 | 58.35 | |
group size (ref: large) | −3.50 ± 1.73 | 2.06 | |
exam number (ref: one) | |||
two | −4.89 ± 0.59 | 8.29 | |
three | −8.57 ± 0.59 | 14.54 | |
Highest Individual Exam Score within a Group | high score ∼ group size + exam | ||
intercept | 86.34 ± 1.34 | 64.57 | |
group size (ref: large) | −4.32 ± 1.27 | 3.12 | |
exam number (ref: one) | |||
two | −8.55 ± 1.47 | 5.81 | |
three | −10.99 ± 1.48 | 7.44 | |
Group Exam Scores | group score ∼ high score | ||
intercept | 26.41 ± 3.23 | 8.19 | |
highest individual score | 0.69 ± 0.04 | 16.61 | |
SAGE Quality of Product | postQual ∼ preQual | ||
intercept | 17.09 ± 2.42 | 7.05 | |
preQuality | 0.72 ± 0.05 | 15.62 | |
SAGE Peer Support | postPeer ∼ prePeer + GPA + 1|group | ||
prePeer Support | 0.24 ± 0.03 | 7.85 | |
GPA | 0.45 ± 0.17 | 2.73 | |
SAGE Interdependence | PostInt∼ preInt + 1|group | ||
preInterdependence | 0.26 ± 0.03 | 9.93 | |
SAGE Satisfaction with Group | postSat ∼ preSat + 1|group | ||
preSatisfaction | 0.26 ± 0.03 | 9.12 |
Despite no impacts on individual scores, students in large groups had higher group exam scores compared with students in small groups (Table 2). Overall, students in small groups earned 82.3 ± 9.0% of the points on group exams while students in large groups earned 85.4 ± 7.1% (Table 3). This result is likely linked to our finding that large groups had significantly higher highest individual scores within the group compared with small groups (Table 2, Figure 1) and highest individual exam score significantly predicted group score (Table 2, Figure 2). For most groups, the group score was higher than the highest individual score within the group (Figure 2).
Small groups | Large groups | |||||
---|---|---|---|---|---|---|
n | Prescore | Postscore | n | Prescore | Postscore | |
Group exam scores | 62 | – | 82.3 ± 9.0 | 32 | – | 85.4 ± 7.1 |
Individual content assessment | 174 | 38.9 ± 11.6 | 65.0 ± 14.2 | 165 | 38.9 ± 8.9 | 63.2 ± 15.7 |
Individual SAGE constructs | 161 | 153 | ||||
Quality of product | 3.42 ± 0.61 | 3.60 ± 0.68 | 3.50 ± 0.57 | 3.67 ± 0.60 | ||
Peer interaction | 3.69 ± 0.47 | 4.00 ± 0.50 | 3.79 ± 0.46 | 4.04 ± 0.51 | ||
Interdependence | 3.81 ± 0.42 | 3.81 ± 0.45 | 3.88 ± 0.35 | 3.77 ± 0.45 | ||
Frustration (Satisfaction) | 3.05 ± 0.50 | 3.38 ± 0.59 | 3.09 ± 0.48 | 3.49 ± 0.52 | ||
Individual exit survey items | 178 | 165 | ||||
Group function on | ||||||
worksheets | – | 4.16 ± 0.80 | – | 4.23 ± 0.71 | ||
group tests | – | 4.36 ± 0.73 | – | 4.49 ± 0.58 | ||
During group tests | ||||||
hear reader | – | 4.59 ± 0.64 | – | 4.30 ± 0.87 | ||
see questions | – | 4.56 ± 0.69 | – | 4.17 ± 0.86 | ||
Feel included on | ||||||
worksheets | – | 4.42 ± 0.76 | – | 4.27 ± 0.86 | ||
group tests | – | 4.55 ± 0.68 | – | 4.45 ± 0.73 | ||
Feel accountable | – | 4.21 ± 1.01 | – | 4.15 ± 0.93 |

FIGURE 1. (A) Highest individual exam scores within a group compared with group scores over the three module exams for students in large and small groups. Students in large groups had higher group exam scores and also had higher highest individual exam scores. (B) Highest individual exam scores within a group compared with group scores over two module exams for students in permanent and nonpermanent groups. Students in permanent groups had higher group exam scores but the highest individual exam score was not affected by group type. The third module exam was not included in the analyses due to an abrupt change to online classes and the subsequent loss of the third group exam.
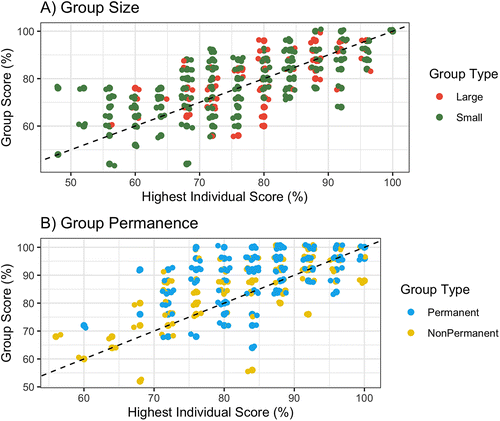
FIGURE 2. Highest individual exam scores compared with group scores for the module exams for (A) large and small groups and (B) permanent and nonpermanent groups. The dashed lines are where highest individual score equals group score. Thus, all points above the line represent groups where the group score was higher than the highest individual score within the group. Because many points overlapped, the data have been randomly “jittered” around the actual value so different groups can be visualized.
Group size did not affect students’ attitudes towards working in groups. Group size was not retained in any of the best-fit models for the four SAGE constructs (Table 2), nor was it retained when the different demographic factors were added to the models (Supplemental Table S2). Overall, students rated group work positively, as demonstrated by mean scores above 3.0 for all SAGE constructs (Table 3). Students in both group sizes rated the dynamics of their group favorably on the exit survey items. They could see tests and worksheets, could hear their group members, felt included, and felt accountable for coming to class (Table 3). Recorded absences supported their perceived accountability. The mean number of absences over 10 weeks was only 1.96 for students in large groups and 1.72 for students in small groups.
Group Permanence
In general, group permanence did not have a significant effect on students’ individual content acquisition. Group permanence status was not retained in the most parsimonious model of factors affecting postassessment score (Table 4). There were also no significant interactions between group permanence and binary gender, BIPOC status, or first-generation status, indicating that students from these minoritized groups in STEM did not appear to be affected differently by group permanence (Supplemental Table S3). Women and first-generation students, however, performed worse on the postassessment compared with men and students who were not first-generation (Table 4).
Best-fit model | Estimate ± SE | t or z value* | |
---|---|---|---|
Individual Content assessment | post ∼ pre + GPA + (1|section) | ||
intercept | 5.54 ± 1.05 | 5.27 | |
preassessment | 0.54 ± 0.05 | 12.10 | |
GPA | 3.58 ± 0.29 | 12.50 | |
Gender | post ∼ pre + gender + GPA + (1|section) | ||
intercept | 5.14 ± 1.05 | 4.88 | |
preassessment | 0.52 ± 0.05 | 11.42 | |
gender (ref: female) | 1.07 ± 0.35 | 3.06 | |
GPA | 3.70 ± 0.29 | 12.92 | |
First generation status | post ∼ pre + first.gen + GPA + (1|section) | ||
intercept | 6.38 ± 1.09 | 5.84 | |
preassessment | 0.53 ± 0.05 | 11.68 | |
first gen status (ref: not first gen) | −0.93 ± 0.38 | 2.48 | |
GPA | 3.47 ± 0.29 | 12.01 | |
Students’ Group Exam Scores | group score ∼ permanence*exam + GPA + 1|section + 1|student | ||
intercept | 86.64 ± 1.79 | 48.49 | |
permanence (ref: nonpermanent) | 0.92 ± 2.04 | 0.46 | |
exam number (ref: one) | |||
two | −5.30 ± 0.59 | 9.00 | |
GPA | 1.51 ± 0.36 | 4.22 | |
group permanence*exam | |||
permanent groups; exam two | 2.09 ± 0.82 | 2.54 | |
Highest Individual Exam Score within a Group | high score ∼ 1|quarter | ||
intercept | 86.23 ± 2.92 | 29.56 | |
Group Exam Scores | group score ∼ high score | ||
intercept | 34.32 ± 4.44 | 7.73 | |
highest individual score | 0.65 ± 0.05 | 12.47 | |
SAGE Quality of Product | PostQual ∼ preQual + (1|group) | ||
preQuality | 0.18 ± 0.01 | 15.67 | |
BIPOC status | PostQual ∼ preQual + BIPOC + (1|group) | ||
preQuality | 0.18 ± 0.01 | 15.73 | |
BIPOC status (ref: not BIPOC) | −0.38 ± 0.18 | 2.15 | |
SAGE Peer Support | Post-Peer ∼ prePeer + permanence + (1|group) | ||
prePeer Support | 0.23 ± 0.02 | 9.81 | |
permanence (ref: nonpermanent) | 0.44 ± 0.17 | 2.68 | |
BIPOC status | PostPeer ∼ prePeer + permanence + BIPOC + (1|group) | ||
prePeer Support | 0.24 ± 0.02 | 9.97 | |
permanence (ref: nonpermanent) | 0.43 ± 0.17 | 2.61 | |
BIPOC status (ref: not BIPOC) | −0.50 ± 0.18 | 2.78 | |
SAGE Interdependence | PostInt∼ preInt + permanence + (1|group) | ||
preInterdependence | 0.29 ± 0.02 | 14.47 | |
permanence (ref: nonpermanent) | 0.66 ± 0.18 | 3.64 | |
SAGE Satisfaction with group | PostSat ∼ preSat + permanence + GPA | ||
intercept | 12.65 ± 1.16 | 10.91 | |
preSatisfaction | 0.59 ± 0.04 | 15.57 | |
permanence (ref: nonpermanent) | 2.23 ± 0.29 | 7.55 | |
GPA | −0.51 ± 0.22 | 2.37 | |
BIPOC status | PostSat ∼ preSat + permanence + BIPOC + GPA | ||
intercept | 12.94 ± 1.16 | 11.14 | |
preSatisfaction | 0.59 ± 0.04 | 15.71 | |
permanence (ref: nonpermanent) | 2.21 ± 0.29 | 7.54 | |
BIPOC status (ref: not BIPOC) | −0.82 ± 0.35 | 2.37 | |
GPA | −0.57 ± 0.22 | 2.65 |
That said, students in permanent groups had higher group exam scores and the interaction between permanence and exam number was retained in the best-fit model (Table 4). In this case, students in permanent groups performed disproportionately better on the second exam, which was more difficult than the first exam as indicated by lower scores compared with exam one in both experiments in this study. The third group exam was disrupted by the abrupt shift to online classes at the end of Winter 2020, so it was not included in this analysis. Overall, students in permanent groups earned 89.8 ± 6.3% of the points on the two group exams and students in nonpermanent groups earned 87.6 ± 5.5% (Table 5). Unlike group size, group permanence did not significantly predict the highest individual exam score within a group, although the highest individual exam score still significantly predicted group exam score (Table 4, Figure 1). As with the group size experiment, the group score was higher than the highest individual score within the group for most groups (Figure 2).
Permanent groups | Nonpermanent groups | |||||
---|---|---|---|---|---|---|
n | Prescore | Postscore | n | Prescore | Postscore | |
Group exam scores | 63 | – | 89.8 ± 6.3 | 64 | – | 87.6 ± 5.5 |
Individual content assessment | 317 | 40.4 ± 10.3 | 67.6 ± 15.3 | 311 | 38.7 ± 11.1 | 66.4 ± 14.8 |
Individual SAGE constructs | 300 | 286 | ||||
Quality of product | 3.5 ± 0.6 | 3.7 ± 0.6 | 3.5 ± 0.6 | 3.6 ± 0.6 | ||
Peer interaction | 3.7 ± 0.5 | 4.0 ± 0.5 | 3.7 ± 0.4 | 3.9 ± 0.4 | ||
Interdependence | 3.9 ± 0.4 | 3.8 ± 0.4 | 3.8 ± 0.4 | 3.7 ± 0.4 | ||
Frustration (Satisfaction) | 3.0 ± 0.5 | 3.4 ± 0.5 | 3.0 ± 0.5 | 3.1 ± 0.5 |
Students in permanent groups had better attitudes towards working in groups, as measured by the SAGE constructs, compared with students in nonpermanent groups. Students in permanent groups were more Satisfied (less frustrated) with their group members, had greater sense of Interdependence, and perceived better Peer support, as group permanence was retained in the best fit models for all these constructs (Table 4, Figure 3). The construct Quality of work, however, was not significantly affected by group permanence.

FIGURE 3. Change in raw means of the four SAGE constructs for students in permanent and nonpermanent groups. Error bars represent standard error. Change in Peer Interactions, Interdependence, and Frustration (Satisfaction) were significantly greater for students in permanent groups compared with students in nonpermanent groups.
BIPOC students differed in their attitudes towards group work compared with white and Asian students, although group permanence was not a significant factor in these differences (interactions between BIPOC status and group permanence were not retained in the best-fit models). BIPOC students perceived less Peer support, reported lower Quality of their work, and were less Satisfied with their group (Table 4). There were no effects of gender or first-generation status on attitudes towards group work.
When asked about their preference for permanent or nonpermanent groups, 88.5% of students in permanent groups reported preferring permanent groups, while 7.3% preferred to change groups (Figure 4). Of students in nonpermanent groups, 36.6% reported preferring to stay in one group, while 49.8% preferred to change groups. The open-ended explanations for preferences of students in both group types yielded six common reasons: students either liked or disliked their group, students in permanent groups were more likely to report positive interactions, there were startup costs and increased logistics when groups changed, groups could be dysfunctional, students liked getting different ideas and perspectives by changing groups, and change was inherently good or bad (Supplemental Table S1). The most common reason for preferring permanent groups was that students felt more comfortable sharing ideas and asking questions when they were in groups with people that they knew well (positive group interactions; Table 6). Students also cited startup costs and the logistics of forming new groups as reasons they preferred to remain in permanent groups. Some students in permanent groups who preferred changing groups reported that their groups got too comfortable with each other, which increased off-task behavior (group dysfunction). A common reason for preferring to change groups was that students liked meeting new people and getting different perspectives on course material. Students also worried about getting stuck in a group they didn’t like. All of these results are presented in Table 6.

FIGURE 4. Percent of students from permanent and nonpermanent groups reporting preference for staying in the same group for the entire class or changing groups twice during the class.
Students in permanent groups | Students in nonpermanent groups | |||
---|---|---|---|---|
Preference | Stay(n = 277) | Change(n = 23) | Stay (n = 113) | Change (n = 154) |
Reason for preference | ||||
Liked/disliked group (%) | 11.2 | 17.4 | 24.8 | 29.2 |
Positive group interactions (%) | 58.5 | 0 | 51.3 | 1.3 |
Startup costs/logistics (%) | 20.9 | 0 | 30.1 | 1.3 |
Group disfunction (%) | 0 | 26.1 | 0.9 | 16.2 |
Different ideas & perspectives (%) | 0.4 | 52.2 | 0.9 | 45.4 |
Change is good/bad (%) | 0.4 | 8.7 | 2.7 | 15.6 |
DISCUSSION
Overall, we found group permanence affected learning outcomes more than group size in our nonmajors Introductory Biology class: students had higher group exam scores and more favorable views toward working in groups when in permanent groups. Students in larger groups of six also had higher group exam scores compared with students in groups of three, but group size did not affect student attitudes toward working in groups. Neither group permanence nor group size affected individual exam scores (Tables 2 and 4).
Group Size
We found that students in large groups performed better on the group part of two-stage exams compared with students in small groups. Two-stage, or collaborative, exams occur when students first take the exam individually, then again as a group (Zipp, 2007; Gilley and Clarkston, 2014; Nicol and Selvaretnam, 2021). Thus, students are able to pool knowledge during the group exam, usually resulting in higher exam scores compared with the individual exam (Rao et al., 2002). There are two possible explanations for the result that our students in large groups outperformed students in small groups. First, simply by probability, large groups were more likely to have high performing students, which contributed positively to the group score. This possibility is supported by large groups having higher highest individual exam scores within the group (Table 2; Figure 1), which means they were more likely to have students who did very well on the individual exam compared with small groups. Another possibility, that is also supported in the literature (e.g., Smith et al., 2009, 2011) is that knowledge was being pooled in groups and large groups had more students working on the problems, thus were able to solve the problems with more ideas. This possibility is supported by our result that mean group exam scores were higher than mean highest individual scores for both large and small groups (Figure 1). In support of this possibility, Smith et al. (2009) found that when students discuss a clicker question after answering individually, discussion leads to increased understanding of the topic, even when none of the students initially answered the question correctly. In a subsequent study, Smith et al. (2011) found that students of all ability groups benefited from peer discussion combined with instructor’s explanation when answering clicker questions. It is interesting in our study that the difference between the mean highest individual score and the mean group score tended to increase over the course of the quarter, suggesting that students were pooling knowledge more effectively after they had worked together for several weeks (students were in permanent groups during the group size experiment). Indeed, in other contexts, two-stage exams have been demonstrated to help develop positive student relationships (Sandahl, 2010) as well as increase individual knowledge and retention (Gilley and Clarkston, 2014; Cooke et al., 2019).
In the literature, the effects of group size on individual content acquisition are conflicting and likely context specific. Our finding that group size did not affect individual content assessment scores (Tables 2 and 3) is consistent with recent meta-analyses. Apugliese and Lewis (2017) found no statistical difference in chemistry understanding for high school and college students in groups of four or less students compared with groups of five or more students. Chen and Yang (2019) conducted a meta-analysis on studies of project-based learning compared with formal lecture in primary, secondary, and university classes and found that group size was not a significant moderator for academic achievement. However, other studies have found that group size affects student achievement. For example, Heller and Hollabaugh (1991) found that physics students in groups of three and four made fewer mistakes when solving complex problems compared with students in groups of two. Hunkeler and Sharp (1997) found that students in larger groups had higher grades compared with students in smaller groups in a senior Engineering course.
Our finding that group size did not affect students’ attitudes towards working in groups (Tables 2 and 3) was not consistent with a recent meta-analysis on student satisfaction in flipped classrooms, which typically employ substantial group work. Strelan et al. (2019) found that students were more satisfied with the course when group size was less than five compared with larger groups. Chou and Chang (2018) also found that undergraduate engineering students were more satisfied with content acquisition, learning performance, and skill development when they were in smaller groups. One reason that students might prefer small groups is that working with a smaller number of peers could encourage individual group members to be more active within the group, which might increase individual learning gains and decrease social loafing (Chidambaram and Tung, 2005). Small groups might also promote higher levels of engagement due to the closer proximity of group members. In our study, however, group size did not affect students’ attitudes towards working in groups; students in large and small groups had similar perceptions about the quality of their work, interactions with their peers, and satisfaction with their groups. Our results are similar to those of Bacon et al. (1999) who also found no effect of group size on students’ experiences in a Master’s in Business Administration program.
We did not find evidence of social loafing in large groups, which could explain why we did not find an effect of group size on student attitudes towards working in groups. In the exit survey, students in both small and large groups reported that they felt accountable for coming to class because they were part of a group and they felt included in all aspects of the group work (Table 3). Individual posttest scores were the same for students in small and large groups and in the exit survey, students in both group sizes reported that they could adequately see group worksheets and tests to participate, and they could hear their peers during group work, indicating that individuals in both small and large groups were equally engaged.
It is likely that different group sizes are optimal for different contexts. For example, different group sizes are recommended for different types of group work: team based-learning recommends five to seven students, problem-based learning recommends five to eight or more students, and cooperative learning recommends two to four members (Michaelsen et al., 2014). In addition, the physical setting could influence the effectiveness of different group sizes. Active-learning classrooms with moveable chairs and students facing each other might support success for larger groups compared with fixed seating lecture halls. Physical space can influence student success, with students in active-learning classrooms outperforming students in traditional lecture spaces in some studies (Brooks, 2011; Cotner et al., 2013). Even within a single space, the physical arrangement of students can influence contributions to students and to groups. For example, Heller and Hollabaugh (1991) observed more off-task behavior when students were sitting side by side compared with when students were facing each other in a lecture hall.
Group Permanence
Students in permanent groups had higher group exam scores and there was an interaction between group exam score and exam, with students in permanent groups performing disproportionately better on the second exam (the third exam was not included in this analysis due to missing data; Table 4, Figure 1). This result was not explained by a difference in the highest- individual score within a group, as was the case in the group size experiment, because group type was not a significant predictor of highest individual score. Instead, it appears that students in permanent groups worked together more effectively to achieve higher scores, especially as the quarter progressed. As with the group size experiment, mean group exam scores were higher than mean highest individual scores within a group, indicating that students were pooling knowledge during the second part of the two-stage exams.
The conclusion that students in permanent groups worked more effectively together is further supported by our evidence that students in permanent groups had better attitudes towards working in groups compared with students in nonpermanent groups. Our SAGE results indicated that students in permanent groups were more Satisfied with their group members, had a greater sense of Interdependence, and had better Peer-interactions (Figure 3). On the exit survey, 88.5% of students in permanent groups in our study preferred permanent groups and one of the main reasons was positive group interactions (Table 6). When asked why they preferred permanent groups, many of our students described better knowledge of their group’s strengths and weaknesses. For example, in response to the question “Do you prefer to stay in the same group for the entire quarter or to change groups? Please explain.” One student responded:
Stay in the same groups the entire quarter. We got along better as time went on and began to understand each other’s (sic) strengths and weaknesses.
and another said
Same group. Because we were all in the same group we got to know each other and got to know each other’s work skills, so we felt like we could rely on each other’s knowledge more because we trusted each other.
Although there is a paucity of research directly testing the impacts of group permanence in undergraduate STEM classrooms (as noted by Hodges, 2018), there is growing evidence that productive discourse and coconstruction of content understanding, which may occur more often in permanent groups, are important for positive group function and content acquisition. In a study investigating the quality of discussion in a class using team-based learning pedagogies, which rely on permanent groups, Leupen et al. (2020) found that higher-order discussion, which included conceptual explanations, re-evaluations, and coconstruction, occurred most often when discussion was centered on complex questions that scored high on Bloom’s taxonomy. The authors suggested that high quality discussion is more likely when students have had time to build positive group dynamics, although they did not test this. Bierema et al. (2017) found that students often coconstructed models they were developing to understand biological concepts and this was important for the successful practice of modeling. In their analysis of student discourse during clicker questions, Knight et al. (2013) found that students coconstructed knowledge in over 75% of recorded discussions while using higher-level reasoning skills. They also found that instructor cues were important for facilitating productive discussion.
There is also evidence that comfort in groups is important for positive group function and content acquisition and our data suggest that permanent groups increase student comfort level. Theobald et al. (2017) found that students who were comfortable with their groups performed significantly better on a posttest compared with students who reported less comfort. They also found that students who reported having a friend in the group were 5.25 times more likely to report being comfortable in their group. On the open-ended exit survey in our study, many of our students in permanent groups described their comfort level increasing as the term progressed such that they felt more comfortable interacting with their peers and asking questions in their group. In response to the open-ended question described above, one student responded:
Same group, we were able to build rapport, and felt comfortable sharing any confusion, concerns, etc. that we had regarding class material, etc. Don’t think I would have the same amount of comfortableness/openness (sic) if I were to be in different groups.
In our study, most students in nonpermanent groups preferred to change groups (Figure 4), although 36.6% of students in nonpermanent groups would have preferred to stay in one group. The main reason students gave in favor of nonpermanent groups was that they would be exposed to other students with different ideas and perspectives. On the open-ended survey, students described enjoying meeting new people and getting fresh perspectives about the content they were learning. For example, on the question about preference for permanent or nonpermanent groups, one student answered:
I prefer to change groups because it gives me a chance to meet new people and to see new ways people learn so I can apply it to my learning skills.
Another reason students reported preferring nonpermanent groups is that they might get stuck in a group with bad social dynamics if groups were permanent. A policy we have implemented to help mitigate this fear is that students can submit a request to change groups. We also wonder if the high level of preference for non-permanent groups among this treatment is that students prefer what they know. In other words, in both nonpermanent and permanent groups, more students preferred the group type they were in. However, there was more preference for “difference” among the students in nonpermanent groups: 36% of students in nonpermanent groups would have preferred permanent groups whereas only 7% of students in permanent groups would have preferred nonpermanent groups.
Effects on Students from Different Demographic Groups
Neither group size nor group permanence had differential effects on women compared with men, BIPOC students, or first-generation students. None of the final models exploring individual content assessment scores or attitudes towards working in groups retained an interaction between the demographic factor and group type (Tables 2 and 4).
In our studies, however, BIPOC students reported less Peer support and were less Satisfied with their groups, regardless of group type, in the group permanence study but not the group size study. This difference in results may be due to the number of students involved in each experiment. The group permanence study had approximately twice the number of student participants becuase it was conducted over two quarters and included four sections of the course. BIPOC students accounted for only 26% of student enrollment at Western Washington University (WWU) during the time of the study (data retrieved from the WWU Office of Institutional Effectiveness on May 23, 2022) and 22% of students in both our studies identified as BIPOC. Thus, doubling the sample size would increase our ability to detect a signal.
Our results add to growing evidence that social identity can impact a student’s experience in group work. Eddy et al. (2015) found that gender, and to some extent race/ethnicity, influenced the roles students preferred to play during group discussion. They also found that international students and Asian Americans were more likely to report a dominator in their group. This latter finding was also a result in Theobald et al.’s (2017) study on comfort in groups.
Implications for the Instructor
Our data do not strongly support the use of groups of one size over the other. Given that, we recommend group sizes that are convenient; in our context that is larger groups due to group composition and instructor workload. It was challenging to create heterogeneous performance groups, which are beneficial to low-performing students in our context (Donovan et al., 2018). There were not enough high-performing students for all our small groups, so some low-performing students did not work with high-performing peers. Groups with six members, on the other hand, were easier to form, allowed for fewer groups in the classroom, and increased the odds that each group had a high-performing student. This was important on group exams on which larger groups performed better, likely due to having members with high scores on the individual part of the two-stage exams. Smaller groups also created space and access issues in a classroom with fixed auditorium seating. Generally, group work has worked best for us in classrooms where there are more seats than students; we use classrooms that either seat 425 or 297 for a 200-person class. When there were twice as many small groups, there wasn’t adequate aisle access for the instructor to reach all groups. There was also twice as much formative assessment feedback and group test grading associated with doubling the number of groups. This increased workload does not feel warranted given the results of this study.
Our data also suggest that permanent groups are better for student learning, as students in permanent groups scored higher on group exams, particularly those that were harder and later in the quarter. Students also preferred permanent groups across several measures of satisfaction. One important caveat is that some students would really rather switch groups, so instructors might consider having this as an option. Permanent groups also decrease course logistics because group formation only has to happen once. Nonpermanent groups, that switched twice, increased instructor workload by tripling the time spent on group-formation tasks, although this cost would be less for instructors who allow students to self-select their own groups.
Considering all the evidence, we suggest forming permanent larger groups to reduce instructor workload, while still maintaining the benefits of students working in groups.
ACKNOWLEDGMENTS
We thank the many WWU Biology 101 students who participated in this research. We also thank undergraduate researcher Erika Francoeur for help coding open-ended questions. Members of the Biology Education Research Group at the University of Washington provided helpful guidance on different stages of this project.