Factors Influencing Instructors’ Adoption and Continued Use of Computing Science Technologies: A Case Study in the Context of Cell Collective
Abstract
Acquiring computational modeling and simulation skills has become ever more critical for students in life sciences courses at the secondary and tertiary levels. Many modeling and simulation tools have been created to help instructors nurture those skills in their classrooms. Understanding the factors that may motivate instructors to use such tools is crucial to improve students’ learning, especially for having authentic modeling and simulation learning experiences. This study designed and tested a decomposed technology acceptance model in which the perceived usefulness and perceived ease of use constructs are split between the teaching and learning sides of the technology to examine their relative weight in a single model. Using data from instructors using the Cell Collective modeling and simulation software, this study found that the relationship between perceived usefulness–teaching and attitude toward behavior was insignificant. Similarly, all relationships between perceived ease of use–teaching and the other variables (i.e., perceived usefulness–teaching and attitude toward behavior) became insignificant. In contrast, we found the relationships between perceived ease of use–learning and the other variables (i.e., perceived usefulness–teaching, perceived usefulness–learning, and attitude toward behavior) significant. These results suggest that priority should be given to the development of features improving learning over features facilitating teaching.
INTRODUCTION
Computational modeling and numerical simulations have played an increasingly important role across diverse learning environments, including science education (e.g., life and medical sciences topics like cell biology and anatomy), mathematics education (e.g., geometry), and engineering education (e.g., structural design) (Smetana and Bell, 2012); a wide range of applications have been propelled by increases in computational power and the availability of large-scale data sets. The COVID-19 pandemic has brought these activities to the forefront, with the public at large exposed to and discussing mathematical models of the disease epidemiology, the impact of the virus on physiology, and vaccine efficacy. Modeling and simulations are considered core elements for not only postsecondary undergraduate biology education guidelines (AAAS, 2011) but also the Next Generation Science Standards for K–12 students (Achieve, 2013). Across the past few decades, education researchers have learned much more about effective and equitable teaching and learning (National Academies of Sciences, Engineering, and Medicine, 2018). For example, educational technology to aid students in doing biological modeling and simulations can enable equitable teaching by providing personalized instruction, access to educational resources, and opportunities for collaboration and engagement; this can help level the playing field for students who may not have access to high-quality educational resources and opportunities and foster a sense of community and belonging (Culp et al., 2005; Thomas, 2016). Indeed, the success of biology education reform increasingly relies on the availability, usability, and educator adoption of computational modeling and simulation technologies. Following recognition that computational modeling and simulation are skills needed for students to deepen their understanding of complex biological processes covered in life sciences courses, many modeling and simulation tools have been developed and made available to facilitate the development of those skills (Helikar, 2021).
Multiple technologies have been used for various educational purposes, including biological education, such as Web platforms, robots, social networking tools, mobile devices, machine learning–based education applications, learning analytics, and virtual reality technologies (Crompton et al., 2018; Crompton and Traxler, 2018; Luo et al., 2019a,b; Brown et al., 2020). However, the development and availability of educational tools are not a sufficient foundation for the transformative adoption of new technologies and related pedagogical changes. Educational technologies are of limited use if they are not broadly adopted and consistently used by instructors to impact students’ learning at scale. Hence, continuous efforts to engage more instructors and keep their interest are essential for new technologies to reach students and impact their learning. At the same time, the development of new technologies for teaching often outpaces research investigating the effectiveness of these technologies in equitably supporting student learning. Although many studies have been conducted to develop and expand our understanding of how educational technologies impact learning (Hrastinski and Keller, 2007; Ross et al., 2010; Barbera et al., 2015; Baydas et al., 2015; Tang and Tsai, 2016), more research is needed for current technological advances, in particular covering interdisciplinary domains such as computational modeling and simulation in the life sciences. Understanding the factors that affect instructors’ adoption of technologies is essential as the field considers how to engage students more actively in learning how to model biological processes.
Many models and theories have been introduced to explain technology adoption and use in general (Venkatesh et al., 2003). They have been applied in investigating factors that influence the adoption and continued use of educational technologies by instructors (Friedrich and Hron, 2011; Abdullah and Ward, 2016; Panigrahi et al., 2018; Kaushik and Verma, 2019; Scherer et al., 2019; Alghazi et al., 2020; Liu et al., 2020; Al-Nuaimi and Al-Emran, 2021; Granić, 2022). Foundational models and theories include the theory of reasoned action (Fishbein and Ajzen, 1975; Fishbein, 1979), technology acceptance model (TAM; Davis, 1989), theory of planned behavior (TPB; Ajzen, 1991), diffusion of innovations theory (DIT; Rogers, 2003), and self-determination theory (Deci and Ryan, 2013), among others. More recent models have attempted to advance or extend TAM and TPB, apply DIT, introduce factors from related models or theories, or investigate additional or alternative belief factors. However, few, if any, studies on educational technology adoption have focused on computational modeling and simulation technologies. Furthermore, although many educational technologies propose features and present challenges for both instructors (teaching) and students (learning), past research has primarily focused on either the teaching or learning side of technology, rarely considering both sides of educational technology features at the same time. In most cases, students’ use of specific educational technologies depends on instructors’ adoption and deployment (especially in the formal education environment), and instructors consider their students when choosing to adopt and continue to use educational technologies. Hence, the research that considers one side of the spectrum (i.e., either teaching or learning) may provide limited insight for promoting the adoption and continued use of educational technologies.
This study attempts to address this need. To explore the factors that may motivate or better facilitate the use of modeling and simulation software in the classroom, we use the Cell Collective (Cell Collective, 2022), an interactive computational and modeling platform designed to deepen students’ understanding of biological processes and networks by building, simulating, and breaking computer models of such biological processes (Helikar, 2021; Helikar et al., 2012, 2015). This study encompasses two core constructs of TAM—perceived usefulness and perceived ease of use—considered both for teaching and learning as perceived by instructors. The results provide practical information about more efficiently (any resources—including financial, human, and time—required for developing educational technology) to developing or enhancing educational technology to promote instructors’ adoption of educational technology more efficiently.
METHODS
Decomposed Technology Acceptance Model
Past studies on educational technology adoption involved either instructors (e.g., Wong, 2016; Cheng, 2019; Huang and Teo, 2020; Islamoglu et al., 2021; Tang et al., 2021) or students (Joo et al., 2016; Yang et al., 2017; Eraslan Yalcin and Kutlu, 2019; Teo et al., 2019; Sun and Gao, 2020), not reflecting the unique situation in which an educational technology provide features for teaching and learning. Weighing the distinct sets of features is critical in deciding where to invest more resources. The few studies that have considered both sides added some measurement items for the learning side into the construct for the teaching side (Wang and Wang, 2009; Sánchez-Mena et al., 2019).
We employed the TAM to address this research gap. TAM was developed to explain how individual end-users accept and use information systems (Davis, 1989). Davis (1989) included the constructs of perceived usefulness (defined as the degree to which one believes that using a particular system would enhance one’s job performance) and perceived ease of use (defined as the degree to which one believes that using a particular system would be free of effort) as determinants of attitudes toward using information systems. The other main variables in TAM are attitude toward behavior (defined as an individual’s positive or negative feelings [evaluative affect] about performing the target behavior—use of Cell Collective in this study; Fishbein and Ajzen, 1975), behavioral intention to use, and actual use of information systems (refer to the Supplemental Material to see how these constructs are measured). Figure 1 is a graphic representation of TAM.
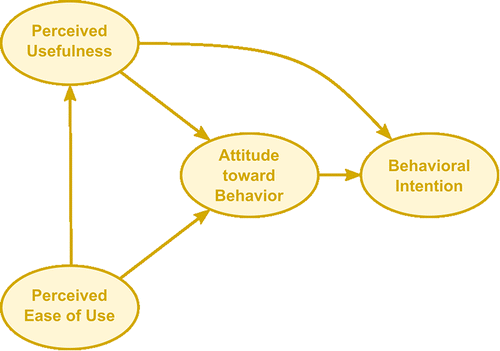
FIGURE 1. Original technology acceptance model (TAM).
Davis et al. (1989) empirically showed that TAM is more powerful in explaining behavioral intention to use technology. Many researchers have empirically tested the relationships between constructs employed in TAM (e.g., Adams et al., 1992; Hendrickson et al., 1993) and extended TAM to different settings (Agarwal and Prasad, 1999; Koufaris, 2002; Gefen et al., 2003; Amoako-Gyampah and Salam, 2004; Wixom and Todd, 2005; Venkatesh and Bala, 2008; Granić and Marangunić, 2019; Al-Emran and Granić, 2021), significantly contributing to developing technology adoption research. A number of studies using TAM indicate its popularity in the field of educational technology (Granić and Marangunić, 2019; Al-Emran and Granić, 2021).
We developed a decomposed TAM in which two core constructs of TAM—perceived usefulness and perceived ease of use—are duplicated to represent teaching and learning, resulting in the variables perceived usefulness–teaching, perceived usefulness–learning, perceived ease of use–teaching, and perceived ease of use–learning. Like the original TAM, the decomposed model hypothesizes positive relationships between perceived usefulness–teaching and attitude toward behavior (H1), between perceived usefulness–teaching and behavioral intention (H2), between perceived usefulness–learning and attitude toward behavior (H3), and between perceived usefulness–learning and behavioral intention (H4). It is reasonable to think that instructors would consider the impact on learning by their students when considering the usefulness of educational technology. Thus, our decomposed model also hypothesizes a positive relationship between perceived usefulness–learning and perceived usefulness–teaching (H5).
Like the original TAM, our decomposed TAM hypothesizes positive associations between perceived ease of use–teaching and attitude toward behavior (H6), between perceived ease of use–teaching and perceived usefulness–teaching (H7), between perceived ease of use–learning and attitude toward behavior (H8), between perceived ease of use–learning and perceived usefulness–learning (H9), between perceived ease of use–learning and perceived usefulness–teaching (H10), and between attitude toward behavior and behavioral intention (H11). Our 11 hypotheses are presented on the decomposed TAM in Figure 2.

FIGURE 2. Decomposed model of educational technology acceptance. Perceived usefulness and ease of use are considered separately for learning and teaching. “Hx” refers to the hypotheses listed in the text.
Measurement
Our questionnaire aimed to measure secondary and tertiary biology instructors’ perceived usefulness and ease of use for teaching and learning, their attitudes toward technology adoption behavior, and their behavioral intentions to use the modeling and simulation software tool Cell Collective in their life sciences courses. We used the research model’s constructs—the latent variables—to minimize measurement error from perception-based question statements and reduce their collinearity (Gefen et al., 2000). Our questionnaire’s scales are all drawn or adapted from existing instruments and follow standard practice (American Educational Research Association et al., 2014). Perceived usefulness scales include five items for teaching and four for learning, while perceived ease of use scales include five items for both teaching and learning; we adapted them from Adams et al. (1992), Davis (1989), Davis et al. (1989), and Moore and Benbasat (1991). The items were worded differently to reflect the teaching and learning context. For example, Moore and Benbasat (1991) have a question item stated as “using a PWS increases my performance,” but in this research, it was revised to “Using Cell Collective improves my teaching performance” to represent the teaching side of the technology and “Using Cell Collective improves my students’ learning performance” to represent the learning side of technology.
Attitude toward behavior has been a core construct in most technology acceptance models (Venkatesh et al., 2003). We adapted the attitude scale from these previous studies by adding the “teaching” context for instructors. Finally, we adapted items to measure behavioral intention from Taylor and Todd (1995) and Venkatesh et al. (2003) by adding “continue to use Cell Collective.” All constructs employed in the research model were modeled to be reflective (Chin, 1998; Diamantopoulos and Siguaw, 2006). We used a seven-point Likert scale to measure each item. The respondents were asked to rate each item or statement from 1 (strongly disagree) to 7 (strongly agree).
Cell Collective: The Technology in This Study
Cell Collective is a modeling and simulation software tool used in the life sciences (Cell Collective, 2022). It is research-grade technology to build scientifically authentic technical skills and makes computational modeling of complex biological processes accessible to users regardless of their prior modeling experience (Helikar, 2021; Helikar et al., 2012, 2015). The technology was originally designed for scientists to use in their work, but also has teaching features for instructors and learning features for students. Having software suitable for use in secondary and tertiary classrooms that is also used by active scientists allows instructors to more authentically engage their students in doing science. Cell Collective is a Web-based platform accessible from any browser, eliminating the need for users to install the software on local computers and allowing flexible implementation (on-campus/remote, lecture/laboratory, in class/homework). The models and lessons are customizable; components and relationships between components can be added, removed, or modified. During the course of developing and implementing the simulation and modeling lessons, research has shown mounting evidence of the effectiveness of simulation and modeling in promoting student learning (Bergan-Roller et al., 2018; Dauer et al., 2019; Clark et al., 2020; Helikar, 2021).
Data Collection
An online survey method was employed to collect data from instructors using Cell Collective. An online survey was conducted to collect cross-sectional research data. An email targeted all instructors who adopted Cell Collective when teaching biological networks. We sent the survey to 98 instructors, but two emails bounced due to invalid addresses, resulting in 96 effective survey invitations. We received 42 responses from 37 institutions (about 30% men, 70% women; an average age of 42.7 years; 17.6% professor, 20.6% associate professor, 38.2% assistant professor, 5.9% lecturer, and 2.9% teaching assistant), ending up with a response rate of 43.8%.
A critically related issue with sample size is whether it provides enough statistical power for hypothesis testing. Power analysis relies on effect size information. The recommended method for determining effect size is to identify the latent variable block of the research model that requires the largest multiple regression; for this, the larger of the following needs to be used: 1) the block with the highest number of formative indicators or 2) the dependent latent variable with the largest number of independent variables affecting it (Kock, 2021). Once the larger of the two options is identified, the effect size, Cohen’s f2, can be calculated using the R2 of the dependent latent variable. According to Cohen (1988), f2 values of 0.02, 0.15, and 0.35 represent the independent variable’s small, medium, and large impact on the dependent variable, respectively; the corresponding R2 for small, medium, and large effect size is 0.02, 0.13, and 0.26, respectively. The research model includes four dependent latent variable blocks: perceived usefulness–teaching, perceived usefulness–learning, attitude toward behavior, and behavioral intention. Table 1 shows R2, f2, the number of predictors, and the sample size required to test hypotheses for a statistical power of 0.8 for each of the dependent latent variable blocks drawing on the power analysis table adapted by Green (1991). According to Table 1, the minimum sample size to test hypotheses for a statistical power of 0.80 for all the dependent latent variable blocks in the research model is 39, and the number of respondents from the online user survey is 43. Therefore, we secured the recommended statistical power of 0.80.
Dependent latent variablea | R2 | Cohen’s effect size (f2) | Number of predictors | Minimum sample size |
---|---|---|---|---|
PU-T | 0.587 | 1.421 (large) | 3 | 35 |
PU-L | 0.447 | 0.808 (large) | 1 | 24 |
ATT | 0.398 | 0.661 (large) | 4 | 39 |
BI | 0.564 | 1.123 (large) | 3 | 35 |
Data Analysis
We performed structural equation modeling (SEM) to test the study’s hypotheses, because it offers an analytical ability to handle both latent and measured variables and allows for the simultaneous analysis of multiple relationships among variables, including direct, indirect, and mediated effects (Kaplan, 2009). The sample size was small, and the sample data in this research did not satisfy the multivariate normality requirement. These data and model characteristics led to a need to conduct partial least-squares SEM (PLS-SEM), which relies on a component-based estimation approach, instead of covariance-based SEM (CB-SEM). Despite certain disadvantages compared with CB-SEM (e.g., potentially biased parameter estimates, no global fit criteria provided), PLS-SEM can handle both data with multivariate nonnormality and a model with a small sample size (Chin, 1998). Estimation methods in CB-SEM—generalized least-squares and maximum likelihood—require normally distributed data. An alternative method—asymptotically distribution free—can be used to estimate parameters using nonnormally distributed data in CB-SEM, but it requires a considerable sample size (e.g., > 2500) and has limitations in handling missing data.
RESULTS
Measurement Model
We modeled all constructs to be reflective. We used the estimates to assess the measurement model in terms of instrument reliability, discriminant validity, collinearity, and predictive validity. Composite reliability and Cronbach’s alpha coefficients are measures of reliability, and average variances extracted (AVE) and full collinearity variance inflation factors (VIFs) were used to assess discriminant validity and overall collinearity, respectively (Kock, 2021). We show the construct coefficients of these assessment criteria in Table 2.
Constructa | Composite reliability | Cronbach’s alpha | Average variance extracted | Full collinearity VIF |
---|---|---|---|---|
PU-T | 0.948 | 0.930 | 0.785 | 2.212 |
PU-L | 0.910 | 0.867 | 0.718 | 2.691 |
PE-T | 0.909 | 0.874 | 0.669 | 1.860 |
PE-L | 0.955 | 0.940 | 0.809 | 3.081 |
ATT | 0.924 | 0.890 | 0.753 | 2.212 |
BI | 0.945 | 0.922 | 0.811 | 2.040 |
Composite reliability and Cronbach’s alpha are used to test internal consistency reliability, the degree to which responses are consistent across a set of question items within a single factor or construct. A measurement instrument can be regarded as having good reliability when the question items associated with each construct are understood in the same way by different respondents (Kock, 2021). Though there is no universal standard about how high composite reliability and Cronbach’s alpha should be, in general, an alpha coefficient greater than 0.9 can be considered “excellent,” a coefficient value greater than 0.8 is “very good,” and a value greater than 0.7 is “adequate” (Kline, 2005, p. 59). The Cronbach’s alpha coefficients for the constructs in this research are all greater than 0.8, indicating that the internal consistency reliability is significantly high (very good or excellent) in this study, as shown in Table 2.
Campbell and Fiske (1959) stressed the importance of using both discriminant and convergent validation techniques when assessing the validity of the measurement model; this recommendation is supported in more recent guidelines for constructing validity arguments for instruments (American Educational Research Association et al., 2014). Discriminant validity represents the degree to which a construct differs from the other constructs and can be tested by AVEs in conjunction with construct correlations. Construct correlations are shown in Table 2. The measurement model demonstrates acceptable discriminant validity, as all the correlation coefficients between paired constructs are less than the square root of AVE associated with each construct. Therefore, o0ur model exhibits strong discriminant validity for the questionnaire to measure instructors’ perceptions of the included scales.
Meanwhile, we achieve strong convergent validity evidence when all standardized factor loadings are greater than 0.7 and significant, when the AVE of each construct is greater than 0.5 (Fornell and Larcker, 1981), and when construct reliability or internal consistency reliability is achieved. As shown in Table 3, all AVEs are greater than 0.5. In addition, construct reliability or internal consistency reliability is demonstrated as acceptable by significantly high composite reliability and Cronbach’s alpha. Finally, Table 4 presents combined factor loadings and cross-loadings that provide evidence for the convergent validity of the measurement model in this research. Two criteria are recommended as the basis for concluding that a measurement model has acceptable convergent validity. First, the p values associated with the loadings should be less than 0.05. Second, the loadings should be equal to or greater than 0.7 (Chin, 1998) or 0.5 (Hair et al., 2009). As shown in Table 4, all factor loadings are both greater than 0.7, except for one item to measure the construct of perceived ease of use for students, and statistically significant (p < 0.001); therefore, our model demonstrates strong evidence for convergent validity of the questionnaire to measure our intended constructs.
Mean | SD | PU-T | PU-L | PE-T | PE-L | ATT | BI | |
---|---|---|---|---|---|---|---|---|
PU-T | 4.90 | 1.32 | 0.886 | |||||
PU-L | 5.65 | 1.07 | 0.658*** | 0.847 | ||||
PE-T | 5.18 | 1.02 | 0.379* | 0.554*** | 0.818 | |||
PE-L | 4.41 | 1.34 | 0.614*** | 0.633*** | 0.638*** | 0.899 | ||
ATT | 5.75 | 0.91 | 0.511*** | 0.595*** | 0.398** | 0.632*** | 0.868 | |
BI | 4.79 | 1.32 | 0.512*** | 0.346* | 0.351* | 0.612*** | 0.596*** | 0.901 |
PU-T | PU-L | PE-T | PE-L | ATT | BI | SE | p value | |
---|---|---|---|---|---|---|---|---|
PU1_T | 0.910 | −0.277 | 0.171 | −0.052 | −0.130 | 0.011 | 0.105 | <0.001 |
PU2_T | 0.897 | −0.162 | 0.040 | 0.045 | 0.214 | −0.012 | 0.106 | <0.001 |
PU3_T | 0.930 | 0.034 | −0.007 | −0.220 | −0.047 | 0.146 | 0.104 | <0.001 |
PU4_T | 0.775 | 0.469 | −0.225 | 0.176 | 0.198 | −0.234 | 0.112 | <0.001 |
PU5_T | 0.910 | 0.003 | −0.011 | 0.082 | −0.202 | 0.050 | 0.105 | <0.001 |
PU1_L | −0.057 | 0.893 | 0.142 | −0.133 | 0.178 | −0.089 | 0.106 | <0.001 |
PU2_L | −0.084 | 0.884 | −0.154 | −0.085 | 0.004 | −0.085 | 0.106 | <0.001 |
PU3_L | −0.130 | 0.865 | −0.079 | 0.001 | −0.151 | 0.017 | 0.107 | <0.001 |
PU4_L | 0.323 | 0.738 | 0.105 | 0.261 | −0.043 | 0.190 | 0.113 | <0.001 |
PE1_T | −0.107 | −0.023 | 0.752 | 0.242 | −0.083 | −0.112 | 0.113 | <0.001 |
PE2_T | −0.109 | 0.257 | 0.866 | −0.144 | 0.163 | −0.143 | 0.107 | <0.001 |
PE3_T | 0.352 | −0.346 | 0.705 | 0.030 | −0.054 | 0.174 | 0.115 | <0.001 |
PE4_T | −0.146 | −0.137 | 0.879 | −0.070 | 0.024 | 0.183 | 0.107 | <0.001 |
PE5_T | 0.063 | 0.183 | 0.873 | −0.020 | −0.071 | −0.086 | 0.107 | <0.001 |
PE1_L | 0.242 | −0.001 | −0.173 | 0.822 | 0.017 | 0.040 | 0.109 | <0.001 |
PE2_L | −0.055 | 0.070 | 0.090 | 0.917 | 0.027 | 0.056 | 0.105 | <0.001 |
PE3_L | −0.137 | 0.019 | −0.073 | 0.911 | −0.095 | −0.055 | 0.105 | <0.001 |
PE4_L | −0.124 | −0.110 | 0.144 | 0.905 | −0.094 | −0.003 | 0.106 | <0.001 |
PE5_L | 0.094 | 0.020 | −0.004 | 0.936 | 0.142 | −0.034 | 0.104 | <0.001 |
ATT1 | −0.150 | 0.250 | −0.013 | −0.072 | 0.910 | 0.003 | 0.105 | <0.001 |
ATT2 | −0.022 | 0.051 | −0.069 | 0.002 | 0.860 | −0.234 | 0.108 | <0.001 |
ATT3 | 0.322 | −0.226 | 0.311 | 0.008 | 0.802 | 0.031 | 0.110 | <0.001 |
ATT4 | −0.115 | −0.102 | −0.199 | 0.064 | 0.894 | 0.195 | 0.106 | <0.001 |
BI1 | −0.020 | −0.079 | 0.063 | 0.178 | −0.134 | 0.923 | 0.105 | <0.001 |
BI2 | −0.158 | −0.006 | −0.139 | 0.274 | 0.000 | 0.919 | 0.105 | <0.001 |
BI3 | −0.048 | 0.160 | −0.078 | −0.011 | −0.149 | 0.903 | 0.106 | <0.001 |
BI4 | 0.242 | −0.077 | 0.165 | −0.475 | 0.301 | 0.855 | 0.108 | <0.001 |
We conducted a Harmon one-factor test (Podsakoff et al., 2003) to see whether the measurement involved a common method bias issue. The covariance explained by one factor is 48.03%, indicating that the common method bias is not a serious concern (not a likely contaminant of the measurement). Full collinearity VIFs can also be used to conduct common method bias tests (Lindell and Whitney, 2001) that are more conservative than the traditionally used tests relying on exploratory factor analyses (Kock, 2021). Table 2 presents full collinearity VIFs for all constructs. These VIFs are estimated by a full collinearity test that enables the identification of both vertical and lateral collinearity (Kock, 2021). The full VIFs in Table 2 are all much less than 10, demonstrating no existence of multicollinearity in the measurement model.
Structural Model
Efron (1979) suggested using bootstrapping (a resampling technique) to determine the significance of path coefficients. We used bootstrapping with 100 resamplings. The results for the default (original) TAM’s average R2(ARS) were 0.332 (p < 0.01), with the average path coefficient (APC) being 0.398 (p < 0.01) and the average variation inflation factor (AVIF) being 1.299. Individual path coefficients between exogenous and endogenous constructs in the original TAM all turned out to be significant, as shown in Figure 3.

FIGURE 3. Results for the original TAM.
Meanwhile, the decomposed model (the research model in this study) explained more of the variance in the endogenous latent variables compared with the original TAM; the average R2(ARS) was 0.494 (p < 0.001), with the APC being statistically significant (β = 0.296, p < 0.01) and the AVIF belonging to an acceptable level (2.349). Individual path coefficients between exogenous and endogenous constructs are shown in Figure 4. The path coefficients from perceived usefulness–teaching to attitude (β = 0.18, p = 0.115) turned out to be insignificant, while the path from perceived usefulness–teaching to behavioral intention (β = 0.41, p < 0.01) was found to be significant, supporting hypothesis 2 but not hypothesis 1. The path coefficient from perceived usefulness–learning to attitude was found to be significant (β = 0.23, p < 0.05), supporting hypothesis 3, but the path to behavioral intention turned out to be insignificant (β = 0.05, p = 0.381), rejecting hypothesis 4. The path coefficient from perceived usefulness–learning to perceived usefulness–teaching was found to be significant (β = 0.51, p < 0.001), supporting hypothesis 5.

FIGURE 4. Results for the decomposed model of educational technology acceptance.
We found the paths from perceived ease of use–teaching to attitude toward behavior (β = 0.09, p = 0.271) and perceived usefulness–teaching (β = 0.05, p = 0.376) insignificant, failing to support hypotheses 6 and 7. In contrast, the path coefficients from perceived ease of use–learning to attitude toward behavior (β = 0.32, p < 0.05) and perceived usefulness–learning (β = 0.67, p < 0.001) turned out to be significant, supporting hypotheses 8 and 9. Furthermore, we also found the path from perceived ease of use–learning to perceived usefulness–teaching significant (β = 0.34, p < 0.01), supporting hypothesis 10. Finally, the path inherited from TAM from attitude toward behavior to behavioral intention was also significant (β = 0.42, p < 0.01), supporting hypothesis 11.
Table 5 shows the total effects of independent constructs on behavioral intention to continue using the technology in this research. All independent constructs but perceived ease of use turned out to have significant total effects on behavioral intention. Among these, the total effect of perceived ease of use for learning turned out to be the largest (effect size = 0.309).
Constructa | Effect | p value | SE | Effect size |
---|---|---|---|---|
PU-T | 0.492 | <0.001 | 0.113 | 0.235 |
PU-L | 0.474 | <0.001 | 0.113 | 0.147 |
PE-T | −0.024 | 0.428 | 0.134 | 0.008 |
PE-L | 0.553 | <0.001 | 0.110 | 0.309 |
ATT | 0.511 | <0.001 | 0.112 | 0.307 |
DISCUSSION
In many cases, students’ use of specific educational technology relies on instructors’ adoption and continued use of that technology. Therefore, to improve life sciences education through novel technologies, such as those enabling learning through computational modeling and simulation (like Cell Collective), motivating or facilitating instructors’ acceptance and use of educational technologies in their courses is crucial. The effort to motivate instructors or to facilitate their task begins with understanding the factors influencing their intention to adopt and continue using educational technologies.
The insights revealed by the original TAM (i.e., the model without the decomposition between learning and teaching) support the relationship between perceived usefulness and attitude toward educational technology. Various studies have consistently supported this relationship since the foundational research by Davis et al. (1989; e.g., Taylor and Todd, 1995; Wixom and Todd, 2005; Bhattacherjee and Sanford, 2006). The original TAM also supports the relationship between perceived usefulness and behavioral intention (Moore and Benbasat, 1991; Thompson et al., 1991; Adams et al., 1992; Compeau and Higgins, 1995; Igbaria et al., 1996; Gefen and Straub, 1997; Karahanna et al., 1999; Karahanna and Straub, 1999), indicating that functionality of educational technology plays a key role in instructors’ decisions to adopt and continue to use educational technology. However, in the decomposed TAM, in which the constructs of perceived usefulness and perceived ease of use are split into teaching and learning, the relationship between perceived usefulness–teaching and attitude became insignificant, while the path from perceived usefulness–learning to attitude still remained significant.
Similarly, all paths from perceived ease of use to the other constructs in the original TAM were supported as theorized. The relationships between perceived ease of use and perceived usefulness and between perceived ease of use and attitude have been found to be significant in the context of educational technology adoption (Scherer et al., 2019). However, in the decomposed TAM, in which the constructs of perceived usefulness and perceived ease of use are split into teaching and learning, all paths from perceived ease of use–teaching became insignificant. In contrast, all paths from added perceived ease of use–learning to the other constructs (i.e., attitude toward behavior, perceived usefulness–teaching, and perceived usefulness–learning) turned out to be significant. Presumably, these findings can be explained from the perspective of instructors’ professional ethics: to serve students (Professional Standards and Practices Commission, n.d.).
Our results have theoretical and practical implications. Theoretically, this study included, for the first time, separate constructs to study the impact of educational technology on learning and teaching in a single model, thereby enabling a comparison between the two sides (e.g., relative weights). This decomposed model of educational technology acceptance reflects the unique context in which instructors consider their students when determining the adoption and continued use of educational technology. The model shows how the relationships among the constructs in the original TAM changed when this unique context is reflected in the model. The comparisons between the original and decomposed models have significant implications for the development and improvement of educational technologies projects in contexts where instructors consider their students when deciding to adopt and continue to use such technology. In most cases, the resources for further development or improvement are limited, and such scarce resources must be used efficiently. Understanding the relatively more important factors should be the first step toward efficient spending. Our results suggest that prioritizing learning features (i.e., features improving learning by the students) over teaching features (i.e., features facilitating the teaching by instructors) is important to motivate instructors to accept and continue to use educational technology.
Despite the significant theoretical and practical implications, the sample size might limit the generalizability of our results. This study met the minimum sample size to secure statistical power of 0.8. However, future research needs to be conducted with a larger sample size to ensure greater statistical power and strengthen the external validity of the results. In this study, TAM was used to examine the relative weights of the constructs affecting the efficiency of instructors and students (i.e., perceived usefulness–teaching vs. perceived usefulness–learning; perceived ease of use–teaching vs. perceived ease of use–learning) through a survey involving instructors. Although what instructors think or feel is relevant to this study’s context (their assessments are based on the information from all sources, including observations of, interactions with, and feedback from students), future research should also consider collecting data directly from students to capture student perceptions of usefulness and ease of use and compare them with instructors’ perceptions of the learning side. For example, a gap between instructors and students in terms of perceived usefulness and ease of use might exist, and that information should be shared with instructors to address their concerns. Future research is warranted to investigate other factors (e.g., facilitating conditions) employed in other major technology acceptance models and theories (e.g., TPB, DIT) and thereby fill a knowledge gap in the literature.
CONCLUSION
Engaging students in modeling and simulation tasks in life sciences courses can improve student learning compared with students solely attending lectures. However, adopting instructional technology to support such tasks takes time (a precious resource for instructors) to develop expertise to use a particular instructional technology tool. Our study has practical implications in the context of limited resources available for creating or improving educational technology: Prioritize features improving the learning rather than features facilitating the teaching to motivate instructors to use the technology, so that they reach more students and help students improve their understanding of life science through interactive computational modeling and simulations.
ACKNOWLEDGMENTS
This research was supported by NSF IUSE grant no. 1915131. All findings and opinions are those of the authors and not necessarily of the funding agency.