Points of View: On the Implications of Neuroscience Research for Science Teaching and Learning: Are There Any?
NOTE FROM THE EDITOR
Points of View is a series designed to address issues faced by many people within the life sciences educational realm. We present differing points of view back to back on a given topic to stimulate thought and dialogue.
The focus of all contributed features and research articles in Issues in Neuroscience Education is the teaching and learning of neuroscience, from elementary school to graduate school audiences. However, neuroscience is unique as a branch of biology in that it includes the study of neuronal and brain mechanisms that may underlie learning. To highlight this unique position of neuroscience, we have chosen to focus this issue's Points of View on how research findings in the field of neuroscience may or may not have implications for the teaching and learning of science in general. We invited authors to address the following questions:
What are the current implications of neuroscience research, if any, for how to improve K–25+ science teaching and learning in schools and universities?
To what extent will neuroscience research into biological mechanisms of learning, memory, attention, and other brain functions inform educational practices and science teaching in the future?
INTRODUCTION
What, if anything, do teachers need to know about how the brain works to improve teaching and learning? After all, your plumber needs to know how to stop leaks—not the molecular structure of water. And we can learn how to use a computer without knowing how a computer chip works. Likewise, teachers need to know how to help students develop intellectually and learn—not necessarily how their brains work. Nevertheless, it is important for teachers to understand that what is being discovered about how brains work supports constructivist learning theory (Alexander and Murphy, 1999), which in turn supports inquiry-based teaching (American Association for the Advancement of Science, 1989; National Research Council, 1996, 2001; National Science Foundation, 1996). The goal of the present article is to explicate why this is so. Let's start with some basics.
SOME BASICS OF BRAIN DEVELOPMENT
The neocortex, which is the most recently evolved part of the brain, has a full complement of brain cells (neurons) at birth—some 100 billion. Yet, the most rapid growth of the neocortex takes place during the first 10 years of life. This growth is primarily because of the proliferation of dendrites, i.e., the branching projections that connect with and receive input, via synapses, from nearby neurons. Importantly, the number of dendrites varies depending on use or disuse. For example, the neurons in the brain area that deals with word understanding (Wernicke's area) have more dendrites in college-educated people than in people with only a high school education (Diamond, 1996). A classic study of the effect of disuse of neurons was conducted during the 1970s by Torsten Wiesel and David Hubel. They covered one of the eyes of newborn kittens at birth. When the covered eyes were uncovered 2 weeks later, they were unable to see. Presumably the lack of environmental input prevented the deprived neurons from developing normally. As Diamond (1996) put it, the phrase “use it or lose it” definitely applies in the case of neurons. Diamond adds, “No matter what form enrichment takes, it is the challenge to the nerve cells that is important. Data indicate that passive observation is not enough; one must interact with the environment.”
How does environmental interaction lead to an increase in the number of functional dendrites? According to neural network theory (Grossberg, 1982, 2005; Jani and Levine, 2000), dendrites become functional when neurotransmitter release rate increases at synaptic knobs. The increase in release rate makes signal transmission from one neuron to the next easier. Hence, learning is understood as an increase in the number of “operative” synaptic connections among neurons. That is, learning occurs when transmitter release rate at synaptic knobs increases so that the signals can be easily transmitted across synapses that were previously there, but inoperative. How, then, does experience strengthen connections?
HOW DOES EXPERIENCE STRENGTHEN CONNECTIONS?
Grossberg (1982, 2005) has proposed and tested equations describing the basic interaction of the key neural variables involved in learning. Of particular significance is his learning equation, which describes changes in transmitter release rate (i.e., Zij). The learning equation identifies factors that modify the synaptic strengths of knobs Nij. Zij represents the initial synaptic strength. Bij is a constant of decay. Thus, Bij Zij is a forgetting or decay term. S′ij[Xj]+ is the learning term as it drives increases in Zij. S′ij is the signal that has passed from node Vi to knob Nij. The prime reflects that the initial signal, Sij, may be slightly altered as it passes down eij. [Xj]+ represents the activity level of postsynaptic nodes, Vj, that exceeds the firing threshold. Only activity above threshold can cause changes in Zij. In short, the learning term indicates that for information to be stored in long-term memory (LTM), two events must occur simultaneously. First, signals must be received at Nij. Second, nodes Vj must receive inputs from other sources that cause the nodes to fire. When these two events drive activity at Nij above a specified constant of decay, the Zij values increase, and the network learns. For a network with n nodes, the learning equation is as follows:
For example, consider Pavlov's classical conditioning experiment in which a dog is stimulated to salivate by the sound of a bell. When Pavlov first rang the bell, the dog, as expected, did not salivate. However, upon repeated simultaneous presentation of food, which did initially cause salivation, and bell ringing, the ringing alone eventually caused salivation. Thus, the food is the unconditioned stimulus (US). Salivation upon presentation of the food is the unconditioned response (UCR). And the bell is the conditioned stimulus (CS). Pavlov's experiment showed that when a CS (e.g., a bell) is repeatedly paired with a US (e.g., food), the CS alone will eventually evoke the UCR (e.g., salivation). How can the US do this?
Figure 1 shows a simple neural network capable of explaining classical conditioning. Although the network is depicted as just three cells (A, B, and C), each cell represents many neurons of the type A, B, and C. Initial food presentation causes cell C to fire. This creates a signal down its axon that, because of prior learning (i.e., a relatively large Zcb), causes the signal to be transmitted to cell B. Thus, cell B fires, and the dog salivates. At the outset, bell-ringing causes cell A to fire and send signals toward cell B. However, when the signal reaches knob NAB, its synaptic strength ZAB is not large enough to cause B to fire. So the dog does not salivate. However, when the bell and the food are paired, cell A learns to fire cell B according to Grossberg's learning equation. Cell A firing results in a large S′AB and the appearance of food results in a large E[XB]+. Thus, the product S′AB[XB]+ is sufficiently large to drive an increase in ZAB to the point at which it alone causes node VB to fire and evoke salivation. Food is no longer needed. The dog has learned to salivate at the ringing of a bell. The key theoretical point is that learning is driven by simultaneous activity of pre- and postsynaptic neurons, in this case activity of cells A and B.
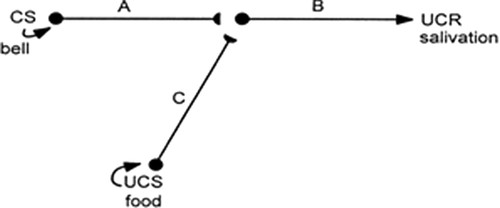
Figure 1. Classical conditioning in a simple neural network. Cells A, B, and C represent layers of neurons.
ADAPTIVE RESONANCE: MATCHING INPUT WITH EXPECTATIONS
Another key aspect of neural network theory explains how the brain processes a continuous stream of sensory input by matching sensory input with expectations derived from prior experience. Grossberg's mechanism for this, called adaptive resonance, is shown in Figure 2

Figure 2. Adaptive resonance occurs when a match of activity patterns occurs on successive slabs of neurons (after Grossberg, 1982; Carpenter and Grossberg, 2003).
The process begins when sensory input X(t) is assimilated by a slab of neurons designated as F(1). Because of prior experience, a pattern of activity, X1 then plays at F(1) and causes a firing of pattern X2 at another slab of neurons F(2). X2 then excites a pattern X on F(1). The pattern X is compared with the input following X1. Thus, X is the expectation. X will be X1 in a static visual scene and the pattern to follow X1 in a temporal sequence. If the two patterns match, then you see what you expect to see. This allows an uninterrupted processing of input and a continued quenching of nonspecific arousal. Importantly, one is only aware of patterns that enter the matched/resonant state. Unless resonance occurs, coding in LTM is not likely to take place. This is because only in the resonant state is there both pre- and postsynaptic excitation of the cells at F(1) (see Grossberg's learning equation).
Now suppose the new input to F(1) does not match the expected pattern X from F(2). Mismatch occurs and this causes activity at F(1) to be turned off by lateral inhibition, which in turn shuts off the inhibitory output to the nonspecific arousal source. This turns on nonspecific arousal and initiates an internal search for a new pattern at F(2) that will match X1.
Such a series of events explains how information is processed across time. The important point is that stimuli are considered familiar if a memory record of them exists at F(2) such that the pattern of excitation sent back to F(1) matches the incoming pattern. If they do not match, the incoming stimuli are unfamiliar and orienting arousal (OA) is turned on to allow an unconscious search for another pattern. If no such match is obtained, then no coding in LTM will take place unless attention is directed more closely at the object in question. Directing careful attention at the unfamiliar object may boost presynaptic activity to a high enough level to compensate for the relatively low postsynaptic activity and eventually allow a recording of the sensory input into a set of previously uncommitted cells.
HOW IS VISUAL INPUT PROCESSED IN DIFFERENT PARTS OF THE BRAIN?
As reviewed by Kosslyn and Koenig (1995), the ability to recognize objects visually requires participation of six major brain areas. As shown in Figure 3, sensory input from the eyes passes from the retina to the back of the brain and produces a pattern of electrical activity in the visual buffer (located in the occipital lobe). This activity produces a spatially organized image within the visual buffer. Next, a smaller region within the visual buffer (called the attention window) performs additional processing. The processed electrical activity is then simultaneously sent along two pathways on each side of the brain: two pathways run down (to the ventral subsystem in the lower temporal lobes), and two run up (to the dorsal subsystem in the parietal lobes). The ventral subsystem analyzes object properties, such as shape, color, and texture. The dorsal subsystem analyzes spatial properties, such as size and location. Patterns of electrical activity within the ventral and dorsal subsystems are then sent and matched to visual patterns stored in associative memory, which is located primarily in the hippocampus, the limbic thalamus, and the basal forebrain. If a good match is found (i.e., an adaptive resonance), the object is recognized and the observer knows the object's name, categories to which it belongs, sounds it makes, and so on.

Figure 3. Kosslyn and Koenig's model of the visual system consists of six major subsystems that spontaneously and subconsciously generate and test hypotheses about what is seen.
However, if a good match is not obtained, the object remains unrecognized and additional sensory input must be obtained. Importantly, the search for additional input is not random. Rather, stored patterns are used to make a second hypothesis about what is being observed, and this hypothesis leads to new observations and to further encoding. In the words of Kosslyn and Koenig, when additional input is sought, “One actively seeks new information that will bear on the hypothesis. The first step in this process is to look up relevant information in associative memory” (p. 57). This information search involves activity in the prefrontal lobes in an area referred to as working memory. Activating working memory causes an attention shift of the eyes to a location where an informative component should be located. Once attention is shifted, the new visual input is processed in turn. The new input is then matched to shape and spatial patterns stored in the ventral and dorsal subsystems and kept active in working memory. Again, in Kosslyn and Koenig's words, “The matching shape and spatial properties may in fact correspond to the hypothesized part. If so, enough information may have accumulated in associative memory to identify the object. If not, this cycle is repeated until enough information has been gathered to identify the object or to reject the first hypothesis, formulate a new one, and test it” (p. 58).
For example, suppose while driving your car you observe what seems to be a puddle of water in the road ahead. Thanks to connections in associative memory, you know that water is wet. So when you continue driving, you expect that your tires will splash through the puddle and get wet. But upon reaching the puddle, it disappears and your tires stay dry. Therefore, your brain rejects the puddle hypothesis and generates another hypothesis, perhaps a mirage hypothesis. The pattern of information processing involved in this example can be summarized as follows:
If … the object is a puddle of water,
and … you continue driving toward it,
then … your tires should splash through the puddle and they should get wet.
But … upon reaching the puddle, it disappears and your tires do not get wet.
Therefore … the hypothesis is not supported; the object was probably not a puddle of water.
In other words, as one seeks to identify objects, the brain generates and tests stored patterns selected from memory. Kosslyn and Koenig even speak of these stored patterns as hypotheses, where the term hypothesis is used in its broadest sense. Thus, brain activity during visual processing uses an If/then/Therefore hypothetico-deductive pattern. One looks at part of an unknown object and the brain spontaneously and immediately generates an idea of what it is—a hypothesis. Thanks to links in associative memory, the hypothesis carries implied consequences (i.e., expectations/predictions). Consequently, to test the hypothesis one can carry out a simple behavior to see whether the prediction does in fact follow. If it does, one has support for the hypothesis. If it does not, then the hypothesis is not supported and the cycle repeats.
IS AUDITORY INPUT PROCESSED IN THE SAME HYPOTHETICO-DEDUCTIVE WAY?
The visual system is only one of several of the brain's information processing systems. However, information seems to be processed in a similar hypothetico-deductive manner by other brain systems. For example, with respect to learning the meaning of spoken words, Kosslyn and Koenig (1995) state “Similar computational analyses can be performed for visual object identification and spoken word identification, which will lead us to infer analogous sets of processing subsystems” (p. 213).
Details of this hypothesized word recognition subsystem are not important. Rather, what is important is that word recognition, like visual recognition, involves brain activity in which hypotheses arise immediately, unconsciously, and before any other activity. In other words, the brain does not make several observations before it generates a hypothesis of what it thinks is out there. Instead, from the slimmest piece of input, the brain immediately generates an idea of what it “thinks” is out there. The brain then acts on that initial idea until subsequent behavior is contradicted. In other words, the brain is not an inductivist organ. Rather, it is an idea-generating and -testing organ that works in a hypothetico-deductive way. There is good reason in terms of human evolution why this would be so. If you were a primitive person and you look into the brush and see stripes, it would certainly be advantageous to get out of there quickly as the consequences of being attacked by a tiger are dire. And anyone programmed to look, look again, and look still again in an “inductivist” way before generating the tiger hypothesis would most likely not survive long enough to pass on his plodding inductivist genes to the next generation.
The important point is that learning does not happen the way you might think. Your brain does not prompt you to look, look again, and look still again until you somehow internalize a successful behavior from the environment. Rather, your brain directs you to look and, as a consequence of that initial look, the brain generates an initial hypothesis that then drives behavior, behavior that carries with it a specific expectation. Hopefully, the behavior is successful in the sense that the prediction is matched by the outcome of the behavior. But sometimes it is not. So the contradicted behavior then prompts the brain to generate another hypothesis and so on until eventually the resulting behavior is not contradicted. In short, we learn from our mistakes—from what some would call trial and error.
CAN NEURAL NETWORKS EXPLAIN HIGHER LEVELS OF REASONING AND LEARNING?
Research has shown that the previous neural network principles can be successfully applied to explain more complex learning. For example, Levine and Prueitt (1989) developed and tested a neural network model to explain performance of normal persons and those with frontal lobe damage on the Wisconsin Card Sorting Task. More recently, Jani and Levine (2000) developed a neural network model that simulates the learning involved in proportional analogy making. But what about more complex learning—learning involved in scientific discovery? Lawson (2002) applied neural network theory to do just that in the case of Galileo Galilei during his discovery of Jupiter's moons in 1610. Following Lawson (2002), let's turn to that report and analyze Galileo's reasoning in terms of If/then/Therefore reasoning and the previously introduced neural network principles.
In 1610 in his Sidereal Messenger, Galileo reported observations made by a new telescope of his invention. In the report Galileo claims to have discovered four “planets” circling Jupiter. As he put it: “I should disclose and publish to the world the occasion of discovering and observing four planets, never seen from the beginning of the world up to our times” (Galilei, 1610, as translated and reprinted in Shapley et al., 1954, p. 59).
Unlike most modern scientific papers, Galileo's report is striking in the way in which it chronologically reveals the steps in his thinking. Thus, it provides an extraordinary opportunity to gain insight into the thinking involved in an important scientific discovery. What follows is a brief recapitulation of part of that report followed by an attempt to fill in gaps in Galileo's reasoning as he interpreted his observations. Galileo's reasoning will then be modeled in terms of Kosslyn and Koenig's neural network principles. Let's start with Galileo's initial observations on January 7.
January 7
Galileo made a new observation on January 7 that he deemed worthy of mention. In his words, “I noticed a circumstance which I had never been able to notice before, owing to want of power in my other telescope, namely that three little stars, small but very bright, were near the planet (i.e., Jupiter).”
This statement suggests that Galileo's observation was immediately assimilated by a fixed star category. In other words, he knew from past experiences that some of the objects in the night sky were fixed stars (i.e., stars that were part of the unchanging celestial sphere). But Galileo's continued thinking led to some initial doubt as this following remark reveals: “… and although I believed them to belong to the number of the fixed stars, yet they made me somewhat wonder, because they seemed to be arranged exactly in a straight line, parallel to the ecliptic, and to be brighter than the rest of the stars, equal to them in magnitude.”
Why would this observation lead Galileo to somewhat wonder? Perhaps he was reasoning along these lines:
If … the three objects are fixed stars,
and … their sizes, brightness, and positions are compared with each other and to other nearby stars,
then … variations in size, brightness, and position should be random, as is the case for other fixed stars.
But … “they seem to be arranged exactly in a straight line, parallel to the ecliptic, and to be brighter than the rest of the stars.”
Therefore … the fixed-star hypothesis is not supported. Or as Galileo put it, “yet they made me wonder somewhat.”
January 8
The next night Galileo made another observation. Again, in his words: “… when on January 8, I found a very different state of things, for there were three little stars all west of Jupiter, and nearer together than on the previous night, and they were separated from one another by equal intervals, as the accompanying figure shows.”
The new observation puzzled Galileo and raised another question. Again, in Galileo's words: “At this point, although I had not turned my thoughts at all upon the approximation of the stars to one another, yet my surprise began to be excited, how Jupiter could one day be found to the east of all the aforementioned stars when the day before it had been west of two of them.” Presumably this observation was puzzling because it was not the expected one based on his fixed-star hypothesis.
Galileo continues, “… forthwith I became afraid lest the planet might have moved differently from the calculation of astronomers, and so had passed those stars by its own proper motion.” This statement suggests that Galileo has not yet rejected the fixed-star hypothesis. Instead, he has generated an ad hoc hypothesis that the astronomers made a mistake, i.e., perhaps their records were wrong about how Jupiter moves relative to the fixed stars in the area. This hypothesis could subsequently be tested as follows:
If … the astronomers made a mistake,
and … I observe the next night,
then … Jupiter should continue to move east relative to the stars, and the objects should look like this:
Of course, we cannot know whether this is what Galileo was thinking, but if he were thinking along these lines, he would have had a very clear prediction to compare with the observations he hoped to make the next night.
January 9 and 10
Galileo continues: “I therefore waited for the next night with the most intense longing, but I was disappointed of my hope, for the sky was covered with clouds in every direction. But on January 10th the stars appeared in the following position with regard to Jupiter, the third, as I thought, being hidden by the planet.”
What conclusion can be drawn from this observation in terms of the astronomers-made-a-mistake hypothesis? Consider the following reasoning:
If … the astronomers made a mistake,
and … I observe the next night,
then … Jupiter should continue to move east relative to the “stars,” and the objects should look like this:
But … the objects did not look like this, instead they looked like this:
Therefore … the astronomers-made-a-mistake hypothesis is not supported.
Interestingly, Galileo states:
When I had seen these phenomena, as I knew that corresponding changes of position could not by any means belong to Jupiter, and as, moreover, I perceived that the stars which I saw had always been the same, for there were no others either in front or behind, within the great distance, along the Zodiac—at length, changing from doubt into surprise, I discovered that the interchange of position which I saw belonged not to Jupiter, but to the stars to which my attention had been drawn.
(p. 60)
So, Galileo concluded that the astronomers had not made a mistake, i.e., the changes of position were not the result of Jupiter's motion. Instead, they were due to motions of the “stars.”
January 11 and Later
Galileo is now left with the task of formulating and testing another hypothesis. The following observation and remarks make it clear that he did not take long to do so:
Accordingly, on January 11 I saw an arrangement of the following kind:
namely, only two stars to the east of Jupiter, the nearer of which was distant from Jupiter three times as far as from the star to the east; and the star furthest to the east was nearly twice as large as the other one; whereas on the previous night they had appeared nearly of equal magnitude. I, therefore, concluded, and decided unhesitatingly, that there are three stars in the heavens moving about Jupiter, as Venus and Mercury round the sun.
(p. 60)
Galileo's remarks make it is clear that he has “conceptualized” a situation in which these objects are traveling around Jupiter in a way analogous to the way our moon travels around the Earth. Thus, he has rejected the fixed star hypothesis and accepted an alternative hypothesis in which the objects are traveling around Jupiter—they are moons of Jupiter. How could Galileo have arrived at such a conclusion? Consider the following reasoning:
If … the objects are orbiting Jupiter,
and … I observe the objects over several nights,
then … some nights they should appear to the east of Jupiter and some nights they should appear to the west. Further, they should always appear along a straight line on either side of Jupiter.
And … this is precisely how they appeared.
Therefore … the moons-of-Jupiter hypothesis is supported.
Galileo's previous statement continues as follows:
… which at length was established as clear as daylight by numerous other subsequent observations. These observations also established that there are not only three, but four, erratic sidereal bodies performing their revolutions round Jupiter … These are my observations upon the four Medicean planets, recently discovered for the first time by me.
(pp. 60–61)
MODELING GALILEO’S REASONING
Kosslyn and Koenig's description of brain subsystem functioning is about recognizing objects present in the visual field during a very brief time period—not distant spots of light seen through a telescope. Nevertheless, the hypothetico-deductive nature of this system functioning is clear. And all one need do to apply the same principles to Galileo's case is to extend the time frame over which observations are made—observations that either match or mismatch expectations. For example, Figure 4 shows how the brain subsystems may have been involved in Galileo's reasoning as he tests his moons hypothesis.

Figure 4. What might have been in Galileo's working memory when he tested the moons hypothesis?
The figure highlights the contents of Galileo's working memory, which is seated in the lateral prefrontal cortex, in terms of one cycle of If/then/Therefore reasoning. As shown, to use If/then/Therefore reasoning to generate and test his moon hypothesis, Galileo must not only allocate attention to it and its predicted consequences, he must also inhibit his previously generated fixed-stars and astronomers-made-a-mistake hypotheses. Thus, working memory can be thought of as a temporary network to sustain information while it is processed. During reasoning, one must pay attention to task-relevant information and inhibit task-irrelevant information. Consequently, working memory involves more than simply allocating attention and temporarily keeping track of it. Rather, during the reasoning process, working memory actively selects information relevant to one's goals and actively inhibits irrelevant information.
INSTRUCTIONAL IMPLICATIONS
How then do people learn? The answer seems to be through encountering puzzling observations and trying to explain them through cycles of If/then/Therefore hypothetico-deductive reasoning. Presumably, this is because this is the way that the brain spontaneously processes information. The more skilled people are at reasoning in this manner, the better they are at learning, at constructing new knowledge (Lawson et al., 2000). The key point in terms of instruction is that for meaningful and lasting learning to occur, students must personally and repeatedly engage in the generation and test of their own self-generated ideas. This means that laboratory and field-based activities become the main instructional vehicles. But such activities cannot be “cookbook” in nature. Instead, they should allow students the freedom to openly inquire and raise puzzling observations. The puzzling observations should then prompt students to generate and test their own alternative explanations with the following sorts of questions becoming the central focus of instruction:
What did you observe?
What is puzzling about what you observed?
What questions are raised?
What are some possible answers/explanations?
How could these possibilities (alternative hypotheses) be tested?
What does each hypothesis and planned test lead you to expect to find?
What are your results?
How do your results compare with your predictions?
What conclusions, if any, can be drawn?
ACKNOWLEDGMENTS
This material is supported in part by the National Science Foundation under award EHR 0412537.
FOOTNOTES
1 Any opinions, findings, and conclusions or recommendations expressed in this publication are those of the author and do not necessarily reflect the views of the National Science Foundation.