Metacognition and Self-Efficacy in Action: How First-Year Students Monitor and Use Self-Coaching to Move Past Metacognitive Discomfort During Problem Solving
Abstract
Stronger metacognitive regulation skills and higher self-efficacy are linked to increased academic achievement. Metacognition and self-efficacy have primarily been studied using retrospective methods, but these methods limit access to students’ in-the-moment metacognition and self-efficacy. We investigated first-year life science students’ metacognition and self-efficacy while they solved challenging problems, and asked: 1) What metacognitive regulation skills are evident when first-year life science students solve problems on their own? and 2) What aspects of learning self-efficacy do first-year life science students reveal when they solve problems on their own? Think-aloud interviews were conducted with 52 first-year life science students across three institutions and analyzed using content analysis. Our results reveal that while first-year life science students plan, monitor, and evaluate when solving challenging problems, they monitor in a myriad of ways. One aspect of self-efficacy, which we call self-coaching, helped students move past the discomfort of monitoring a lack of understanding so they could take action. These verbalizations suggest ways we can encourage students to couple their metacognitive skills and self-efficacy to persist when faced with challenging problems. Based on our findings, we offer recommendations for helping first-year life science students develop and strengthen their metacognition to achieve improved problem-solving performance.
INTRODUCTION
Have you ever asked a student to solve a problem, seen their solution, and then wondered what they were thinking while they were problem solving? As college instructors, we often ask students in our classes to solve problems. Sometimes we gain access to our students’ thought process or cognition through strategic question design and direct prompting. Far less often we gain access to how our students regulate and control their own thinking (metacognition) or their beliefs about their capability to solve the problem (self-efficacy). Retrospective methods can and have been used to access this information from students, but students often cannot remember what they were thinking a week or two later. We lack deep insight into students’ in-the-moment metacognition and self-efficacy because it is challenging to obtain their in-the-moment thoughts.
Educators and students alike are interested in metacognition because of its malleable nature and demonstrated potential to improve academic performance. Not having access to students’ metacognition in-the-moment presents a barrier towards developing effective metacognitive interventions to improve learning. Thus, there is a need to characterize how life science undergraduates use their metacognition during individual problem-solving and to offer evidence-based suggestions to instructors for supporting students’ metacognition. In particular, understanding the metacognitive skills first-year life science students bring to their introductory courses will position us to better support their learning earlier on in their college careers and set them up for future academic success.
Metacognition and Problem Solving
Metacognition, or one’s awareness and control of their own thinking for the purpose of learning (Cross and Paris, 1988), is linked to improved problem-solving performance and academic achievement. In one meta-analysis of studies that spanned developmental stages from elementary school to adulthood, metacognition predicted academic performance when controlling for intelligence (Ohtani and Hisasaka, 2018). In another meta-analysis specific to mathematics, researchers found a significant positive correlation between metacognition and math performance in adolescences, indicating individuals who demonstrated stronger metacognition also performed better on math tasks (Muncer et al., 2022). The strong connection between metacognition and problem-solving performance and academic achievement represents a potential leverage point for enhancing student learning and success in the life sciences. If we explicitly teach life science undergraduates how to develop and use their metacognition, we can expect to increase the effectiveness of their learning and subsequent academic success. However, in order to provide appropriate guidance, we must first know how students in the target population are employing their metacognition.
Based on one theoretical framework of metacognition, metacognition is comprised of two components: metacognitive knowledge and metacognitive regulation (Schraw and Moshman, 1995). Metacognitive knowledge includes one’s awareness of learning strategies and of themselves as a learner. Metacognitive regulation encompasses how students act on their metacognitive knowledge or the actions they take to learn (Sandi-Urena et al., 2011). Metacognitive regulation is broken up into three skills: 1) planning how to approach a learning task or goal, 2) monitoring progress towards achieving that learning task or goal, and 3) evaluating achievement of said learning task or goal (Stanton et al., 2021). These regulation skills can be thought of temporally: planning occurs before learning starts, monitoring occurs during learning, and evaluating takes place after learning has occurred. As biology education researchers, we are particularly interested in life science undergraduates’ metacognitive regulation skills or the actions they take to learn because regulation skills have been shown to have a more dramatic impact on learning than awareness alone (Dye and Stanton, 2017).
Importantly, metacognition is context-dependent, meaning metacognition use may vary depending on factors such as the subject matter or learning task (Kelemen et al., 2000; Kuhn, 2000; Veenman and Spaans, 2005). For example, the metacognitive regulation skills a student may use to evaluate their learning after reading a text in their literature course may differ from those skills the same student uses to evaluate their learning on a genetics exam. This is why it is imperative to study metacognition in a particular context, like problem solving in the life sciences.
Metacognition helps a problem solver identify and work with the givens or initial problem state, reach the goal or final problem state, and overcome any obstacles presented in the problem (Davidson and Sternberg, 1998). Specifically, metacognitive regulation skills help a solver select strategies, identify obstacles, and revise their strategies to accomplish a goal. Metacognition and problem solving are often thought of as domain-general skills because of their broad applicability across different disciplines. However, metacognitive skills are first developed in a domain-specific way and then those metacognitive skills can become more generalized over time as they are further developed and honed (Kuhn, 2000; Veenman and Spaans, 2005). This is in alignment with research from the problem-solving literature that suggests stronger problem-solving skills are a result of deep knowledge within a domain (Pressley et al., 1987; Frey et al., 2022). For example, experts are known to classify problems based on deep conceptual features because of their well-developed knowledge base whereas novices tend to classify problems based on superficial features (Chi et al., 1981). Research on problem solving in chemistry indicates that metacognition and self-efficacy are two key components of successful problem solving (Rickey and Stacy, 2000; Taasoobshirazi and Glynn, 2009). College students who achieve greater problem-solving success are those who: 1) use their metacognition to conceptualize problems well, select appropriate strategies, and continually monitor and check their work, and 2) tend to have higher self-efficacy (Taasoobshirazi and Glynn, 2009; Cartrette and Bodner, 2010).
Metacognition and Self-efficacy
Self-efficacy, or one’s belief in their capability to carry out a task (Bandura, 1977, 1997), is another construct that impacts problem solving performance and academic achievement. Research on self-efficacy has revealed its predictive power in regards to performance, academic achievement, and selection of a college major (Pajares, 1996). The large body of research on self-efficacy suggests that students who believe they are capable academically, engage more metacognitive strategies and persist to obtain academic achievement compared with those who do not (e.g., Pintrich and De Groot, 1990; Pajares, 2002; Huang et al., 2022). In STEM in particular, studies tend to reveal gender differences in self-efficacy with undergraduate men indicating higher self-efficacy in STEM disciplines compared with women (Stewart et al., 2020). In one study of first-year biology students, women were significantly less confident than men and students’ biology self-efficacy increased over the course of a single semester when measured at the beginning and end of the course (Ainscough et al., 2016). However, self-efficacy is known to be a dynamic construct, meaning one’s perception of their capability to carry out a task can vary widely across different task types and over time as struggles are encountered and expertise builds for certain tasks (Yeo and Neal, 2006).
Both metacognition and self-efficacy are strong predictors of academic achievement and performance. For example, one study found that students with stronger metacognitive regulation skills and greater self-efficacy beliefs (as measured by self-reported survey responses) perform better and attain greater academic success (as measured by GPA; Coutinho and Neuman, 2008). Additionally, self-efficacy beliefs were strong predictors of metacognition, suggesting students with higher self-efficacy used more metacognition. Together, the results from this quantitative study using structural equation modeling of self-reported survey responses suggests that metacognition may act as a mediator in the relationship between self-efficacy and academic achievement (Coutinho and Neuman, 2008).
Most of the research on self-efficacy has been quantitative in nature. In one qualitative study of self-efficacy, interviews were conducted with middle school students to explore the sources of their mathematics self-efficacy beliefs (Usher, 2009). In this study, evidence of self-modeling was found. Self-modeling or visualizing one’s own self-coping during difficult tasks can strengthen one’s belief in their capabilities and can be an even stronger source of self-efficacy than observing a less similar peer succeed (Bandura, 1997). Usher (2009) described self-modeling as students’ internal dialogues or what they say to themselves while doing mathematics. For example, students would tell themselves they can do it and that they would do okay as a way of keeping their confidence up or coaching themselves while doing mathematics. Other researchers have called this efficacy self-talk, or “thoughts or subvocal statements aimed at influencing their efficacy for an ongoing academic task” (Wolters, 2003, p. 199). For example, one study found that college students reported saying things to themselves like “You can do it, just keep working” in response to an open-ended questionnaire about how they would maintain effort on a given task (Wolters, 1998; Wolters, 2003). As qualitative researchers, we were curious to uncover how both metacognition (planning, monitoring, and evaluating) and self-efficacy (such as self-coaching) might emerge out of more qualitative, in-the-moment data streams.
Methods for Studying Metacognition
Researchers use two main methods to study metacognition: retrospective and in-the-moment methods. Retrospective methods ask learners to reflect on learning they’ve done in the past. In contrast, in-the-moment methods ask learners to reflect on learning they’re currently undertaking (Veenman et al., 2006). Retrospective methods include self-report data from surveys like the Metacognitive Awareness Inventory (Schraw and Dennison, 1994) or exam “wrappers” or self-evaluations (Hodges et al., 2020). Whereas in-the-moment methods include think-aloud interviews, which ask students to verbalize all of their thoughts while they solve problems (Bannert and Mengelkamp, 2008; Ku and Ho, 2010; Blackford et al., 2023), or online computer chat log-files as groups of students work together to solve problems (Hurme et al., 2006; Zheng et al., 2019).
Most metacognition research on life science undergraduates, including our own work, has utilized retrospective methods (Stanton et al., 2015, 2019; Dye and Stanton, 2017). Important information about first-year life science students’ metacognition has been gleaned using retrospective methods, particularly in regard to planning and evaluating. For example, first-year life science students tend to use strategies that worked for them in high school, even if they do not work for them in college, suggesting first-year life science students may have trouble evaluating their study plans (Stanton et al., 2015). Additionally, first-year life science students abandon strategies they deem ineffective rather than modifying them for improvement (Stanton et al., 2019). Lastly, first-year life science students are willing to change their approach to learning, but they may lack knowledge about which approaches are effective or evidence-based (Tomanek and Montplaisir, 2004; Stanton et al., 2015).
In both of the meta-analyses described at the start of this Introduction, the effect sizes were larger for studies that used in-the-moment methods (Ohtani and Hisasaka, 2018; Muncer et al., 2022). This means the predictive power of metacognition for academic performance was more profound for studies that used in-the-moment methods to measure metacognition compared with studies that used retrospective methods. One implication of this finding is that studies using retrospective methods might be failing to capture metacognition’s profound effects on learning and performance. Less research has been done using in-the-moment methods to study metacognition in life science undergraduates likely because of the time-intensive nature of collecting and analyzing data using these methods. One study that used think-aloud methods to investigate biochemistry students’ metacognition when solving open-ended buffer problems found that monitoring was the most commonly used metacognitive regulation skill (Heidbrink and Weinrich, 2021). Another study that used think-aloud methods to explore Dutch third-year medical school students’ metacognition when solving physiology problems about blood flow also revealed a focus on monitoring, with students also planning and evaluating but to a lesser extent (Versteeg et al., 2021). We hypothesize that in-the-moment methods like think-aloud interviews are likely to reveal greater insight into students monitoring skills because this metacognitive regulation skill occurs during learning tasks. Further investigation into the nature of the metacognition first-year life science students use when solving problems is needed in order to provide guidance to this population and their instructors on how to effectively use and develop their metacognitive regulation skills.
Research Questions
To pinpoint first-year life science students’ metacognition in-the-moment and to describe the relationship between their metacognition and self-efficacy during problem solving, we conducted think-aloud interviews with 52 students from three different institutions to answer the following research questions:
What metacognitive regulation skills are evident when first-year life science students solve problems on their own?
What aspects of learning self-efficacy do first-year life science students reveal when they solve problems on their own?
METHODS
Research Participants & Context
This study is a part of a larger longitudinal research project investigating the development of metacognition in life science undergraduates which was classified by the Institutional Review Board at the University of Georgia (STUDY00006457) and University of North Georgia (2021-003) as exempt. For that project, 52 first-year students at three different institutions in the southeastern United States were recruited from their introductory biology or environmental science courses in the 2021–2022 academic year. Data was collected at three institutions to represent different academic environments because it is known that context can affect metacognition (Table 1). Georgia Gwinnett College is classified as a baccalaureate college predominantly serving undergraduate students, University of Georgia is classified as doctoral R1 institution, and University of North Georgia is classified as a master’s university. Additionally, in our past work we found that first-year students from different institutions differed in their metacognitive skills (Stanton et al., 2015, 2019). Our goal in collecting data from three different institution types was to ensure our qualitative study could be more generalizable than if we had only collected data from one institution.
Georgia Gwinnett College | University of Georgia | University of North Georgia | |
---|---|---|---|
Institution type | Baccalaureate College | Doctoral R1 | Master’s University |
Setting | Suburban | City | Suburban |
Number of undergraduates | 10,949 | 30,166 | 18,155 |
Students from racially minoritized groups | 57.8% | 14.4% | 19.3% |
Students who identify as women | 58.7% | 58.9% | 57.8% |
Students who identify as first-generation | 37% | 9% | 20.6% |
Average high school GPA | 3.0 | 4.1 | 3.5 |
Average SAT score | 1065 | 1355 | 1135 |
Students at each institution were invited to complete a survey to provide their contact information, answer the revised 19-item Metacognitive Awareness Inventory (Harrison and Vallin, 2018), 32-item Epistemic Beliefs Inventory (Schraw et al., 1995), and 8-item Self-efficacy for Learning and Performance subscale from the Motivated Strategies for Learning Questionnaire (MSLQ; Pintrich et al., 1993). They were also asked to self-report their demographic information including their age, gender, race/ethnicity, college experience, intended major, and first-generation status. First-year students who were 18 years or older and majoring in the life sciences were invited to participate in the larger study. We used purposeful sampling to select a sample that matched the demographics of the student body at each institution and also represented a range in metacognitive ability based on students’ responses to the revised Metacognitive Awareness Inventory (Harrison and Vallin, 2018). In total, eight students from Georgia Gwinnett College, 23 students from the University of Georgia, and 21 students from the University of North Georgia participated in the present study (Table 2). Participants received $40 (either in the form of a mailed check, or an electronic Starbucks or Amazon gift card) for their participation in Year 1 of the larger longitudinal study. Their participation in Year 1 included completing the survey, three inventories, and a 2-hour interview, of which the think aloud interview was one quarter of the total interview.
Georgia Gwinnett College | University of Georgia | University of North Georgia | |
---|---|---|---|
Number of participants | 8 | 23 | 21 |
Participants from underrepresented racially minoritized groups | 4 | 5 | 5 |
Participants who identify as women | 8 | 13 | 15 |
Participants who identify as first-generation | 5 | 3 | 6 |
Average High School GPA | 3.3 | 4.0 | 3.6 |
Average College GPA | 3.4 | 3.7 | 2.9 |
Data Collection
All interviews were conducted over Zoom during the 2021–2022 academic year when participants had returned to the classroom. Participants (n = 52) were asked to think aloud as they solved two challenging biochemistry problems (Figure 1) that have been previously published (Halmo et al., 2018, 2020; Bhatia et al., 2022). We selected two challenging biochemistry problems for first-year students to solve because we know that students do not use metacognition unless they find a learning task challenging (Carr and Taasoobshirazi, 2008). If the problems were easy, they may have solved them quickly without needing to use their metacognition or by employing metacognition that is so automatic they may have a hard time verbalizing it (Samuels et al., 2005). By having students solve problems we knew would be challenging, we hoped this would trigger them to use and verbalize their metacognition during their problem-solving process. This would enable us to study how they used their metacognition and what they did in response to their metacognition. The problems we selected met this criterion because participants had not yet taken biochemistry.
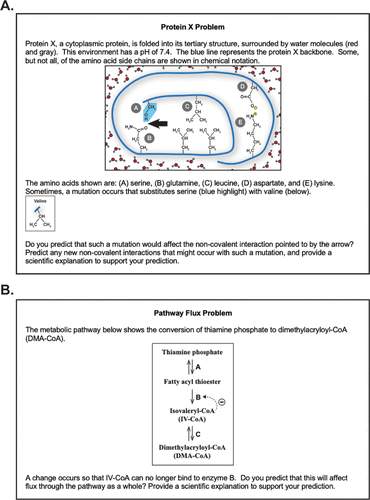
FIGURE 1. Think-Aloud Problems. Students were asked to think aloud as they solved two challenging biochemistry problems. Panel A depicts the Protein X Problem previously published in Halmo et al., 2018 and 2020. Panel B depicts the Pathway Flux problem previously published in Bhatia et al., 2022. Both problems are open-ended and ask students to make predictions and provide scientific explanations for their predictions.
The problems were open-ended and asked students to make predictions and provide scientific explanations for their predictions about: 1) noncovalent interactions in a folded protein for the Protein X Problem (Halmo et al., 2018, 2020) and 2) negative feedback regulation in a metabolic pathway for the Pathway Flux Problem (Bhatia et al., 2022). Even though the problems were challenging, we made it clear to students before they began that we were not interested in the correctness of their solutions but rather we were genuinely interested in their thought process. To elicit student thinking after participants fell silent for more than 5 seconds, interviewers used the following two prompts: “What are you thinking (now)?” and “Can you tell me more about that?” (Ericsson and Simon, 1980; Charters, 2003). After participants solved the problems, they shared their written solutions with the interviewer using the chat feature in Zoom. Participants were then asked to describe their problem-solving process out loud and respond to up to four reflection questions (see Supplemental Material for full interview protocol). The think-aloud interviews were audio and video recorded and transcribed using a professional, machine-generated transcription service (Temi.com). All transcripts were checked for accuracy by members of the research team before analysis began.
Data Analysis
The resulting transcripts were analyzed by a team of three researchers in three cycles. In the first cycle of data analysis, half of the transcripts were open coded by members of the research team (S.M.H., J.D.S., and K.A.Y.). S.M.H. entered this analysis as a postdoctoral researcher in biology education research with experience in qualitative methods and deep knowledge about student difficulties with the two problems students were asked to solve in this study. J.D.S., an associate professor of cell biology and a biology education researcher, entered this analysis as an educator and metacognition researcher with extensive experience in qualitative methods. K.A.Y. entered this analysis as an undergraduate student double majoring in biology and psychology and as an undergraduate researcher relatively new to qualitative research. During this open coding process, we individually reflected on the contents of the data, remained open to possible directions suggested by our interpretation of the data, and recorded our initial observations using analytic memos (Saldaña, 2021). The research team (S.M.H., J.D.S., and K.A.Y.) then met to discuss our observations from the open coding process and suggest possible codes that were aligned with our observations, knowledge of metacognition and self-efficacy, and our guiding research questions. This discussion led to the development of an initial codebook consisting of inductive codes discerned from the data and deductive codes derived from theory on metacognition and self-efficacy. In the second cycle of data analysis, the codebook was applied to the dataset iteratively by two researchers (S.M.H. and K.A.Y.) using MaxQDA2020 software (VERBI Software; Berlin, Germany) until the codebook stabilized or no new codes or modifications to existing codes were needed. Coding disagreements between the two coders were discussed by all three researchers until consensus was reached. All transcripts were coded to consensus to identify aspects of metacognition and learning self-efficacy that were verbalized by participants. Coding to consensus allowed the team to consider and discuss their diverse interpretations of the data and ensure trustworthiness of the analytic process (Tracy, 2010; Pfeifer and Dolan, 2023). In the third and final cycle of analysis, thematic analysis was used to uncover central themes in our dataset. As a part of thematic analysis, two researchers (S.M.H. and K.A.Y.) synthesized one-sentence summaries of each participant’s think aloud interview. Student quotes presented in the Results & Discussion have been lightly edited for clarity, and all names are pseudonyms.
Problem-Solving Performance as One Context for Studying Metacognition
To compare the potential effect of institution and gender on problem solving performance, we scored the final problem solutions, and then interrogated them using R Statistical Software (R Core Team, 2021). A one-way ANOVA was performed to compare the effect of institution on problem-solving performance. This analysis revealed that there was not a statistically significant difference in problem-solving performance between the three institutions (F[2, 49] = 0.085, p = 0.92). This indicates students performed similarly on the problems regardless of which institution they attended (Supplemental Data, Table 1). Another one-way ANOVA was performed to compare the effect of gender on problem-solving performance which revealed no statistically significant differences in problem-solving performance based on gender (F[1, 50] = 0.956, p = 0.33). Students performed similarly on the problems regardless of their gender (Supplemental Data, Table 2). Taken together, this analysis suggests a homogeneous sample in regard to problem-solving performance.
Participants’ final problem solutions were individually scored by two researchers (S.M.H. and K.A.Y.) using an established rubric and scores were discussed until complete consensus was reached. The rubric used to score the problems is available from the corresponding author upon request. The median problem-solving performance of students in our sample was two points on a 10-point rubric. Students in our sample scored low on the rubric because they either failed to answer part of the problem or struggled to provide accurate explanations or evidence to support their predictions. Despite the phrase “provide a scientific explanation to support your prediction” included in the prompt, most students’ solutions contained a prediction, but lacked an explanation. For example, the majority of the solutions for the Protein X problem predicted the noncovalent interaction would be affected by the substitution, but lacked categorization of the relevant amino acids or identification of the noncovalent interactions involved, which are critical problem-solving steps for this problem (Halmo et al., 2018, 2020). The majority of the Pathway Flux solutions also predicted that flux would be affected, but lacked an accurate description of negative feedback inhibition or regulation release of the pathway, which are critical features of this problem (Bhatia et al., 2022). This lack of accurate explanations is not unexpected. Previous work shows that both introductory biology and biochemistry students struggle to provide accurate explanations to these problems without pedagogical support, and introductory biology students generally struggle more than biochemistry students (Bhatia et al., 2022; Lemons, personal communication).
RESULTS AND DISCUSSION
What metacognitive regulation skills are evident when first-year life science students solve problems on their own?
To address our first research question, we looked for statements and questions related to the three skills of planning, monitoring, and evaluating in our participants’ think aloud data. Because metacognitive regulation skills encompass how students act on their metacognitive awareness, participants’ explicit awareness was a required aspect when analyzing our data for these skills. For example, the statement “this is a hydrogen bond” does not display awareness of one’s knowledge but rather the knowledge itself (cognition). In contrast, the statement “I know this is a hydrogen bond” does display awareness of one’s knowledge and is therefore considered evidence of metacognition. We found evidence of all three metacognitive regulation skills in our data. First-year life science students plan, monitor, and evaluate when solving challenging problems. However, our data collection method revealed more varied ways in which students monitor. We present our findings for each metacognitive regulation skill (Table 3). For further demonstration of how students use these skills in concert when problem solving, we offer problem-solving vignettes of a student from each institution in Supplemental Data.
Metacognitive regulation skill | Category | Description | Example Data | Implications for instruction |
---|---|---|---|---|
Planning | Assessing the task | Student identifies what the problem is asking them to do either successfully or unsuccessfully. | So, I know that not only do I have to give my answer, but I also have to provide information on how I got my answer.I cannot predict anything. Not sure what [the question] wants. | Model planning for students by verbalizing how to assess the task and what strategies to use and why before walking through a worked example.Provide students with immediate feedback on the accuracy of their assessment of the task. |
Monitoring | Relevance | Student describes what parts of the prompt or pieces of their own knowledge are relevant or irrelevant to solving the problem. | So now I’m looking back up top and I’m like, “is the pH irrelevant or relevant to the question?” | Explicitly teach students relevant strategies that can help resolve confusion, a lack of understanding, or uncertainty. See Stanton et al., 2021 for an evidence-based teaching guide on metacognition. |
Confusion | Student expresses a general lack of understanding or knowledge about the problem. | Well, I first look at the image and I’m already kind of confused with it. | ||
Familiarity | Student describes what is familiar or not familiar to them or something they remember or forget from class. | I’m seeing some stuff that I understand or learned about in my bio class, like tertiary structure, pH, and amino acid side chains.I’m not familiar with the specific amino acids and how they’re different. | Encourage students to assess the effectiveness of their strategy use in response to their monitoring. For example, was acknowledging and using an assumption helpful in moving forward when you were uncertain? | |
Understanding | Student describes specific pieces of knowledge they know or don’t know. | I know that enzymes speed up processes from my previous knowledge.I don’t know what a flux is either. | Provide guidance on how to keep track of the information gleaned from these types of monitoring during problem solving. For example, by writing down what they do and do not know. | |
Questions | Student asks themselves a question. | Covalent bonds are sharing a bond, but what does noncovalent mean? | ||
Correctness | Student corrects themselves while talking out loud | Sorry. I just noticed that that’s not even a carboxyl group. That’s a carbonyl group and that’s a hydroxyl group. | ||
Evaluating | Solution | Student assesses the accuracy of their solution, double checks their answer, or rethinks their solution. | I think I got the answer right.Now I’m kind of double guessing my own answer… | Provide students with immediate feedback about the accuracy of their solution(s) to help them evaluate and develop well-calibrated self-evaluation skills. For example, provide answer keys on formative assessments.Encourage students to self-coach during problem-solving to overcome potentially negative emotions or feelings of discomfort that may occur when they are metacognitive. |
Experience | Student assesses the problem difficulty or the feelings associated with their thought process. | It’s a very hard question.At first, I was kind of happy because I knew what was going on.I went into this kind of worried, because a lot of this does not look really that familiar. |
Planning: Students did not plan before solving but did assess the task in the moment
Planning how to approach the task of solving problems individually involves selecting strategies to use and when to use them before starting the task (Stanton et al., 2021). Planning did not appear in our data in a classical sense. This finding is unsurprising because the task was: 1) well-defined, meaning there were a few potentially accurate solutions rather than an abundant number of accurate solutions, 2) straightforward meaning the goal of solving the problem was clearly stated, and 3) relatively short meaning students were not entering and exiting from the task multiple times like they might when studying for an exam. Additionally, the stakes were comparatively low meaning task completion and performance carried little to no weight in participants’ college careers. In other data from this same sample, we know that these participants make plans for high-stakes assessments like exams but often admit to not planning for lower stakes assessments like homework (Stanton, personal communication). Related to the skill of planning, we observed students assessing the task after reading the problem (Table 3). We describe how students assessed the task and provide descriptions of what happened after students planned in this way.
Assessing the task
While we did not observe students explicitly planning their approach to problem solving before beginning the task, we did observe students assessing the task or what other researchers have called “orientation” after reading the problems (Meijer et al., 2006; Schellings et al., 2013). Students in our study either assessed the task successfully or unsuccessfully. For example, when Gerald states, “So I know that not only doI have to give my answer, but I also have to provide information on how I got my answer…” he successfully identified what the problem was asking him to do by providing a scientific explanation. In contrast, Simone admits her struggle with figuring out what the problem is asking when she states, “I’m still trying to figure out what the question’s asking. I don’t want to give up on this question just yet, but yeah, it’s just kinda hard becauseI can’t figure out what the question is asking meif I don’t know the terminology behind it.” In Simone’s case, the terminology she struggled to understand is what was meant by a scientific explanation. Assessing the task unsuccessfully also involved misinterpreting what the problem asked. This was a frequent issue for students in our sample during the Pathway Flux problem because students inaccurately interpreted the negative feedback loop, which is a known problematic visual representation in biochemistry (Bhatia et al., 2022). For example, students like Paulina and Kathleen misinterpreted the negative feedback loop as enzyme B no longer functioning when they stated, respectively, “So if enzyme B is taken out of the graph…”, or “…if B cannot catalyze…” Additionally some students misinterpreted the negative feedback loop as a visual cue of the change described in the problem prompt (IV-CoA can no longer bind to enzyme B). This can be seen in the following example quote from Mila: “So I was looking at it and I see what they’re talking about with the IV-CoA no longer binding to enzyme B and I think that’s what that arrow with the circle and the line through it is representing. It’s just telling me that it’s not binding to enzyme B.”
What happened after assessing the task?
Misinterpretations of what the problem was asking like those shared above from Simone, Paulina, Kathleen, and Mila led to inaccurate answers for the Pathway Flux problem. In contrast, when students like Gerald could correctly interpret what the problem asked them to do, this led to more full and accurate answers for both problems. Accurately interpreting what a problem is asking you to do is critical for problem-solving success. A related procedural error identified in other research on written think-aloud protocols from students solving multiple-choice biology problems was categorized as misreading (Prevost and Lemons, 2016).
Implications for Instruction & Research about Planning
In our study, we did not detect evidence of explicit planning beyond assessing the task. This suggests that first-year students’ approaches were either unplanned or automatic (Samuels et al., 2005). As metacognition researchers and instructors, we find first-year life science students’ absence of planning before solving and presence of assessing the task during solving illuminating. This means planning is likely one area in which we can help first-year life science students grow their metacognitive skills through practice. While we do not anticipate that undergraduate students will be able to plan how to solve a problem that is unfamiliar to them before reading a problem, we do think we can help students develop their planning skills through modeling when solving life science problems.
When modeling problem solving for students, we could make our planning explicit for students by verbalizing how we assess the task and what strategies we plan to use and why. From the problem-solving literature, it is known that experts assess a task by recognizing the deep structure or problem type and what is being asked of them (Chi et al., 1981; Smith et al., 2013). This likely happens rapidly and automatically for experts through the identification of visual and key word cues. Forcing ourselves to think about what these cues might be and alerting students to them through modeling may help students more rapidly develop expert-level schema, approaches, and planning skills. Providing students with feedback on their assessment of a task and whether or not they misunderstood the problem also seems to be critical for problem-solving success (Prevost and Lemons, 2016). Helping students realize they can plan for smaller tasks like solving a problem by listing the pros and cons of relevant strategies and what order they plan to use selected strategies before they begin could help students narrow the problem solving space, approach the task with focus, and achieve efficiency to become “good strategy users” (Pressley et al., 1987).
Monitoring: Students monitored in the moment in a myriad of ways
Monitoring progress towards problem-solving involves assessing conceptual understanding during the task (Stanton et al., 2021). First-year life science students in our study monitored their conceptual understanding during individual problem solving in a myriad of ways. In our analysis, we captured the specific aspects of conceptual understanding students monitored. Students in our sample monitored: 1) relevance, 2) confusion, 3) familiarity, 4) understanding, 5) questions, and 6) correctness (Table 3). We describe each aspect of conceptual understanding that students monitored and we provide descriptions of what happened after students monitored in this way (Figure 2).

FIGURE 2. How monitoring can impact the problem-solving process. The various ways first-year students in this study monitored are depicted as ovals. See Table 3 for detailed descriptions of the ways students monitored. How students in this study acted on their monitoring are shown as rectangles. In most cases, what happened after students monitored determined whether or not problem solving moved forward. Encouraging oneself using positive self-talk, or self-coaching, helped students move past the discomfort associated with monitoring a lack of conceptual understanding (confusion, lack of familiarity, or lack of understanding) and enabled them to use problem-solving strategies, which moved problem solving forward.
Monitoring Relevance
When students monitored relevance, they described what pieces of their own knowledge or aspects of the problem prompts were relevant or irrelevant to their thought process (Table 3). For the Protein X problem, many students monitored the relevance of the provided information about pH. First-year life science students may have focused on this aspect of the problem prompt because pH is a topic often covered in introductory biology classes, which participants were enrolled in at the time of the study. However, students differentially decided whether this information was relevant or irrelevant. Quinn decided this piece of information was relevant: “The pH of the water surrounding it.I think it’s importantbecause otherwise it wouldn’t really be mentioned.” In contrast, Ignacio decided the same piece of information was irrelevant: “Sothe pH has nothing to do with it. The water molecules had nothing to do with it as well. So basically, everything in that first half, everything in that first thing, right there is basically useless.So,I’m just going to exclude that information out of my thought processcause the pH has nothing to do with what’s going on right now…” From an instructional perspective, knowing the pH in the Protein X problem is relevant information for determining the ionization state of acidic and basic amino acids, like amino acids D and E shown in the figure, could be helpful. However, this specific problem asked students to consider amino acids A and B, so Ignacio’s decision that the pH was irrelevant may have helped him focus on more central parts of the problem. In addition to monitoring the relevance of the provided information, sometimes students would monitor the relevance of their own knowledge that they brought to bear on the problem. For example, consider the following quote from Regan: “I just think that it might be a hydrogen bond, whichhas nothing to do with the question.” Regan made this statement during her think aloud for the Protein X problem, which is intriguing because the Protein X problem deals solely with noncovalent interactions like hydrogen bonding.
What happened after monitoring relevance?
Overall, monitoring relevance helped students narrow their focus during problem solving, but could be misleading if done inaccurately like in Regan’s case (Figure 2).
Monitoring Confusion
When students monitored confusion when solving, they expressed a general lack of understanding or knowledge about the problem (Table 3). As Sara put it, “I have no cluewhat I’m looking at.” Sometimes monitoring confusion came as an acknowledgement of lack of prior knowledge students felt they needed to solve the problem. Take for instance when Ismail states, “I’ve never really had any prior knowledge on pathway fluxes and like how they work and it obviouslydoesn’t make much sense to me.” Students also expressed confusion about how to approach the problem, which is related to monitoring one’s procedural knowledge. For example, when Harper stated, “I’m not sure how to approach the question,” she was monitoring a lack of knowledge about how to begin. Similarly, after reading the problem Tiffani shared, “I am not sure how to solve thisonebecause I’ve actually never done it before…”
What happened after monitoring confusion?
When students monitored their confusion, one of two things happened (Figure 2). Rarely, students would give up on solving altogether. In fact, only one individual (Roland) submitted a final solution that read, “I have no idea.” More often students persisted despite their confusion. Rereading the problem was a common strategy students in our sample used after identifying general confusion. As Jeffery stated after reading the problem, “I didn’t really understand that, so I’m gonna read that again.” After rereading the problem a few times, Jeffery stated, “Oh, and we have valine here. I didn’t see that before.” Some students like Valentina revealed their rereading strategy rationale after solving, “First I just read it a couple of times because I wasn’t really understanding what it was saying.” After rereading the problem a few times Valentina was able to accurately assess the task by stating “amino acid (A) turns into valine.” When solving, some students linked their general confusion with an inability solve. As Harper shared, “I don’t think that I have enough like basis or learning to where I’m able to answer that question.” Despite making this claim of self-doubt in their ability to solve, Harper monitored in other ways and ultimately came up with a solution beyond a simple, “I don’t know.” In sum, when students acknowledged their confusion in this study, they usually did not stop there. They used their confusion as an indicator to use a strategy, like rereading, to resolve their confusion or as a jumping off point to further monitor by identifying more specifically what they did not understand. Persisting despite confusion is likely dependent on other factors, like self-efficacy.
Monitoring Familiarity
When students monitored familiarity, they described knowledge or aspects of the problem prompt that were familiar or not familiar to them (Table 3). This category also captured when students would describe remembering or forgetting something from class. For example, when Simone states, “I rememberlearning covalent bonds in chemistry, butI don’t rememberright now what that meant” she is acknowledging her familiarity with the term covalent from her chemistry course. Similarly, Oliver acknowledges his familiarity with tertiary structure from his class when solving the Protein X problem. He first shared, “This reminds me of somethingthat we’ve looked at in class of a tertiary structure. It was shown differently butI do remember something similar to this.” Then later, he acknowledges his lack of familiarity with the term flux when solving the Pathway Fux problem, “That word flux.I’ve never heard that word before.” Quinn aptly pointed out that being familiar with a term or recognizing a word in the problem did not equate to her understanding, “I mean, I know amino acids, but that doesn’t… likeI recognize the word, but it doesn’t really mean anything to me. And then non-covalent, I recognize the conjunction of words, but again, it's like somewhere deep in there…”
What happened after monitoring familiarity?
When students recognized what was familiar to them in the problem, it sometimes helped them connect to related prior knowledge (Figure 2). In some cases, though, students connected words in the problem that were familiar to them to unrelated prior knowledge. Erika, for example, revealed in her problem reflection that she was familiar with the term mutation in the Protein X problem and formulated her solution based on her knowledge of the different types of DNA mutations, not noncovalent interactions. In this case, Erika’s familiarity with the term mutation and failure to monitor the relevance of this knowledge when problem solving impeded her development of an accurate solution to the problem. This is why Quinn’s recognition that her familiarity with terms does not equate to understanding is critical. This recognition can help students like Erika avoid false feelings of knowing that might come from the rapid and fluent recall of unrelated knowledge (Reber and Greifeneder, 2017). When students recognized parts of the problem they were unfamiliar with, they often searched for familiar terms to use as footholds (Figure 2). For example, Lucy revealed the following in her problem reflection: “So first I tried to look at the beginning introduction to see if I knew anything about the topic. Unfortunately, I did not know anything about it. So, I just tried to look for any trigger words that I did recognize.” After stating this, Lucy said she recognized the words protein and tertiary structure and was able to access some prior knowledge about hydrogen bonds for her solution.
Monitoring Understanding
When students monitored understanding, they described specific pieces of knowledge they either knew or did not know, beyond what was provided in the problem prompt (Table 3). Monitoring understanding is distinct from monitoring confusion. When students displayed awareness of a specific piece of knowledge they did not know (e.g., “I don’t know what these arrows really mean.”) this was considered monitoring (a lack of) understanding. In contrast, monitoring confusion was a more general awareness of their overall lack of understanding (e.g., “Well, I first look at the image andI’m already kind of confused with it[laughs].”). For example, Kathleen demonstrated an awareness of her understanding about amino acid properties when she said, “I knowthat like the different amino acids all have different properties like some are, what’s it called? Like hydrophobic, hydrophilic, and then some are much more reactive.” Willibald monitored his understanding using the mnemonic “when in doubt, van der Waals it out” by sharing, “So, causeI knowbasically everything has, well not basically everything, but a lot of things have van der Waal forces in them. So that’s why I say that a lot of times. But it’s a temporary dipole, I think.” In contrast, Jeffery monitored his lack of understanding of a specific part of the Pathway Flux figure when he stated, “I guessI don’tunderstandwhat this dotted arrow is meaning.” Ignoring or misinterpreting the negative feedback loop was a common issue as students solved this problem, so it’s notable that Jeffery acknowledged his lack of understanding about this symbol. When students identified what they knew, the incomplete knowledge they revealed sometimes had the potential to lead to a misunderstanding. Take for example Lucy’s quote: “I knowa hydrogen bond has to have a hydrogen. I know that much. And it looks like they both have hydrogen.” This statement suggests Lucy might be displaying a known misconception about hydrogen bonding – that all hydrogens participate in hydrogen bonding (Villafañe et al., 2011).
What happened after monitoring understanding?
When students could identify what they knew, they used this information to formulate a solution (Figure 2). When students could identify what they did not know, they either did not know what to do next or they used strategies to move beyond their lack of understanding (Figure 2). Two strategies students used after identifying a lack of understanding included disregarding information and writing what they knew. Kyle disregarded information when he didn’t understand the negative feedback loop in the Pathway Flux problem: “…there is another arrow on the side I see with a little minus sign. I’m not sure what that means… it’s not the same as [the arrows by] A and C. So, I’m just going to disregard it sort of for now. It’s not the same. Just like note that in my mind that it’s not the same.” In this example, Kyle disregards a critical part of the problem, the negative feedback loop, and does not revisit the disregarded information which ultimately led him to an incorrect prediction for this problem. We also saw one example of a student, Elaine, use the strategy of writing what she knew when she was struggling to provide an explanation for her answer: “I should know this more, but I don’t know, like a specific scientific explanation answer, but I’m just going to write what I do know so I can try to organize my thoughts.” Elaine’s focus on writing what she knew allowed her to organize the knowledge she did have into a plausible solution that specified which amino acids would participate in new noncovalent interactions (“I predict there will be a bond between A and B and possibly A and C.”) despite not knowing “what would be required in order for it to create a new noncovalent interaction with another amino acid.” The strategies that Kyle and Elaine used in response to monitoring a lack of understanding shared the common goal of helping them get unstuck in their problem-solving process.
Monitoring Questions
When students monitored through questions, they asked themselves a question out loud (Table 3). These questions were either about the problem itself or their own knowledge. An example of monitoring through a question about the problem itself comes from Elaine who asked herself after reading the problem and sharing her initial thoughts, “What is this asking me?” Elaine’s question helped reorient her to the problem and put herself back on track with answering the question asked. After Edith came to a tentative solution, she asked herself, “But what about the other information?How does that pertain to this?” which helped her initiate monitoring the relevance of the information provided in the prompt. Students also posed questions to themselves about their own content knowledge. Take for instance Phillip when he asked himself, “So, would noncovalent be ionic bonds or would it be something else? Covalent bonds are sharing a bond, butwhat doesnoncovalentmean?” After Phillip asked himself this question, he reread the problem but ultimately acknowledged he was “not too sure what noncovalent would mean.”
What happened after monitoring questions?
After students posed questions to themselves while solving, they either answered their question or they didn’t (Figure 2). Students who answered their self-posed questions relied on other forms of monitoring and rereading the prompt to do so. For example, after questioning themselves about their conceptual knowledge, some students acknowledged they did not know the answer to their question by monitoring their understanding. Students who did not answer their self-posed questions moved on without answering their question directly out loud.
Monitoring Correctness
When students monitored correctness, they corrected their thinking out loud (Table 3). A prime example of this comes from Kyle’s think aloud, where he corrects his interpretation of the problem not once but twice: “It said the blue one highlighted is actually a valine, which substituted the serine, so that’s valine right there. And then I’m reading the question.No, no, no. It’s the other way around.So, serine would substitute the valine and the valine is below…Oh waitwait, I had it right the first time.So, the blue highlighted is this serine and that’s supposed to be there, but a mutation occurs where the valine gets substituted.” Kyle first corrects his interpretation of the problem in the wrong direction but corrects himself again to put him on the right track. Icarus also caught himself reading the problem incorrectly by replacing the word noncovalent with the word covalent, which was a common error students made: “Oh, wait, I think I read that wrong.I think I read it wrong. Well, yeah. Then that will affect it. I didn’t read the noncovalent part. I just read covalent.” Students also corrected their language use during the think aloud interviews, like Edith: “becauseenzyme B is no longer functioning… No, not enzyme B…becauseIV-CoA is no longer functionaland able to bind to enzyme B, the metabolic pathway is halted.” Edith’s correction of her own wording, while minor, is worth noting because students in this study often misinterpreted the Pathway Flux problem to read as “enzyme B no longer works”. There were also instances when students corrected their own knowledge that they brought to bear on the problem. This can be seen in the following quote from Tiffani when she says, “And tertiary structure. It has multiple…No, no, no. That’s primary structure.Tertiary structure’s when like the proteins are folded in on each other.”
What happened after monitoring correctness?
When students corrected themselves, this resulted in more accurate interpretations of the problem and thus more accurate solutions (Figure 2). Specifically, monitoring correctness helped students avoid common mistakes when assessing the task which was the case for Kyle, Icarus, and Edith described above. When students do not monitor correctness, incorrect ideas can go unchecked throughout their problem-solving process, leading to more inaccurate solutions. In other research, contradicting and misunderstanding content were two procedural errors students experienced when solving multiple-choice biology problems (Prevost and Lemons, 2016), which could be alleviated through monitoring correctness.
Implications for Instruction & Research about Monitoring
Monitoring is the last metacognitive regulation skill to develop, and it develops slowly and well into adulthood (Schraw, 1998). Based on our data, first-year life science students are monitoring in the moment in a myriad of ways. This may suggest that college-aged students have already developed monitoring skills by the time they enter college. This finding has implications for both instruction and research. For instruction, we may need to help our students keep track of and learn what do with the information and insight they glean from their in-situ monitoring when solving life science problems. For example, students in our study could readily identify what they did and did not know, but they sometimes struggled to identify ways in which they could potentially resolve their lack of understanding, confusion, or uncertainty or use this insight in expert-like ways when formulating a solution.
As instructors who teach students about metacognition, we can normalize the temporary discomfort monitoring may bring as an integral part of the learning process and model for students what to do after they monitor. For example, when students glean insight from monitoring familiarity, we could help them learn how to properly use this information so that they do not equate familiarity with understanding when practicing problem solving on their own. This could help students avoid the fluency fallacy or the false sense that they understand something simply because they recognize it or remember learning about it (Reber and Greifeneder, 2017).
The majority of the research on metacognition, including our own, has been conducted using retrospective methods. However, retrospective methods may provide little insight into true monitoring skills since these skills are used during learning rather than after learning has occurred (Schraw and Moshman, 1995; Stanton et al., 2021). More research using in-the-moment methods, which are used widely in the problem-solving literature, are needed to fully understand the rich monitoring skills of life science students and how they may develop over time. The monitoring skills of life science students in both individual and small group settings, and the relationship of monitoring skills across these two settings, warrants further exploration. This seems particularly salient given that questioning and responding to questions seems to be an important aspect of both individual metacognition in the present study and social metacognition in our prior study, which also used in-the-moment methods (Halmo et al., 2022).
Evaluating: Students evaluated their solution and experience problem solving
Evaluating achievement of individual problem solving involves appraising an implemented plan and how it could be improved for future learning after completing the task (Stanton et al., 2021). Students in our sample revealed some of the ways they evaluate when solving problems on their own (Table 3). They evaluated both their solution and their experience of problem solving.
Evaluating A Solution
Evaluating a solution occurred when students assessed the accuracy of their solution, double-checked their answer, or rethought their solution (Table 3). While some students evaluated their accuracy in the affirmative (that their solution is right), most students evaluated the accuracy of their solution in the negative (that their solution is wrong). For example, when Kyle states, “I don’t think hydrogen bonding is correct.” Kyle clarified in his problem reflection, “I noticed [valine] did have hydrogens and the only noncovalent interaction I know of is probably hydrogen bonding. So, I just sort of stuck with that and just said more hydrogen bonding would happen with the same oxygen over there [in glutamine].” Through this quote, we see that Kyle went with hydrogen bonding as his prediction because it’s the only noncovalent interaction he could recall. However, Kyle accurately evaluated the accuracy of his solution by noting that hydrogen bonding was not the correct answer. Evaluating accuracy in the negative often seemed like hedging or self-doubt. Take for instance Regan’s quote that she shared right after submitting her final solution: “The chances of being wrongare100%, just like, you know [laughs].”
Students also evaluated their solution by double-checking their work. Kyle used a very clearly-defined approach for double checking his work by solving the problem twice: “So that’s just my initial answer I would put, and then what I do next wasI’d just like reread the question and sort of see if I come up with the same answer after rereading and redoing the problem.So, I’m just going to do that real quick.” Checking one’s work is a well-established problem-solving step that most successful problem solvers undertake (Cartrette and Bodner, 2010; Prevost and Lemons, 2016).
Students also evaluated by rethinking their initial solution. In the following case, Mila’s evaluation of her solution did not improve her final answer. Mila initially predicted that the change described in the Pathway Flux problem would affect flux, which is correct. However, she evaluates her solution when she states, “Oh, wait a minute, now that I’m saying this out loud, I don’t think it’ll affect it because I think IV-CoA will be binding to enzyme B or C. Sorry, hold on.Now I’m like rethinking my whole answer.” After this evaluation, Mila changes her prediction to “it won’t affect flux”, which is incorrect. In contrast, some students’ evaluations of their solutions resulted in improved final answers. For example, after submitting his solution and during his problem reflection, Willibald states, “Oh, I just noticed. I said there’ll be no effect on the interaction, but then I said van der Waals forces which is an interaction. So,I just contradicted myself in there.” After this recognition, Willibald decides to amend his first solution, ultimately improving his prediction. We also observed one student, Jeffery, evaluating whether or not his solution answered the problem asked, which is notable because we also observed students evaluating in this way when solving problems in small groups (Halmo et al., 2022): “I guess I can’t say for sure, but I’ll say this new amino acid form[s] a bond with the neighboring amino acids and results in a new protein shape.The only issue with that answer is I feel like I’m not really answering the question: Predict any new noncovalent interactions that might occur with such a mutation.” While the above examples of evaluating solution occurred spontaneously without prompting, having students describe their thinking process after solving the problems may have been sufficient to prompt them to evaluate their solution.
What happened after evaluating a solution?
When students evaluated the accuracy of their solution, double-checked their answer, or rethought their solution it helped them recognize potential flaws or mistakes in their answers. After evaluating their solution, they either decided to stick with their original answer or amend their solution. Evaluating a solution often resulted in students adding to or refining their final answer. However, these solution amendments were not always beneficial or in the correct direction because of limited content knowledge. In other work on the metacognition involved in changing answers, answer-changing neither reduced or significantly boosted performance (Stylianou-Georgiou and Papanastasiou, 2017). The fact that Mila’s evaluation of her solution led to a less correct answer, whereas Willibald’s evaluation of his solutions led to a more correct answer further contributes to the variable success of answer-changing on performance.
Evaluating Experience
Evaluating experience occurred when students assessed the difficulty level of the problem or the feelings associated with their thought process (Table 3). This type of evaluation occurred after solving in their problem reflection or in response to the closing questions of the think aloud interview. Students evaluated the problems as difficult based on the confusion, lack of understanding, or low self-efficacy they experienced when solving. For example, Ivy stated, “I just didn’t really have any background knowledge on them, which kind of made it difficult.” In one instance, Willibald’s evaluation of difficulty while amending his solution was followed up with a statement about self-efficacy: “This one was a difficult one. I told you I’m bad with proteins [laughs].” Students also compared the difficulty of the two problems we asked them to solve. For example, Elena determined that the Pathway Flux problem was easier for her compared with the Protein X problem in her problem reflection: “I didn’t find this question as hardas the last question just cause it was a little bit more simple.” In contrast, Elaine revealed that she found the Protein X problem challenging because of the open-ended nature of the question: “I just thought that was a little more difficultbecause it’s just asking me to predict what possibly could happen instead of like something that’s like, definite, like I know the answer to. So, I just tried to think about what I know…” Importantly, Elaine indicated her strategy of thinking about what she knew in the problem in response to her evaluation of difficulty.
Evaluating experience also occurred when students assessed how their feelings were associated with their thought process. The feelings they described were directly tied to aspects of their monitoring. We found that students associated negative emotions (nervousness, worry, and panic) with a lack of understanding or a lack of familiarity. For example, in Renee’s problem reflection, she connected feelings of panic to when she monitored a lack of understanding: “I kind ofpanickedfor a second, not really panicked cause I know this isn’t like graded or anything, but I do not know what a metabolic pathway is.” In contrast, students associated more positive feelings when they reflected on moments of monitoring understanding or familiarity. For example, Renee also stated, “At first I was kind ofhappybecause I knew what was going on.” Additionally, some students revealed their use of a strategy explicitly to engender positive emotions or to avoid negative emotions, like Tabitha: “I looked at the first box, I tried to break it up into certain sections,so I did not get overwhelmedby looking at it.”
What happened after evaluating experience?
When students evaluated their experience problem solving in this study, they usually evaluated the problems as difficult and not easy. Their evaluations of experience were directly connected to aspects of their monitoring while solving. They associated positive emotions and ease with understanding and negative emotions and difficulty with confusion, a lack of familiarity, or a lack of understanding. Additionally, they identified the purpose of some strategy use was to avoid negative experiences. Because their evaluations of experience occurred after solving the problems, most students did not act on this evaluation in the context of this study. We speculate that students may act on evaluations of experience by making plans for future problem solving, but our study design did not necessarily provide students with this opportunity. Exploring how students respond to this kind of evaluation in other study designs would be illuminating.
Implications for Instruction & Research about Evaluating
Our data indicate that some first-year life science students are evaluating their solution and experience after individual problem solving. As instructors, we can encourage students to further evaluate their solutions by prompting them to: 1) rethink or redo a problem to see whether they come up with the same answer or wish to amend their initial solution, and 2) predict whether they think their solution is right or wrong. Encouraging students to evaluate by predicting whether their solution is right or wrong is limited by content knowledge. Therefore, it is imperative to help students develop their self-evaluation accuracy by following up their predictions with immediate feedback to help them become well-calibrated (Osterhage, 2021). Additionally, encouraging students to reflect on their experience solving problems might help students identify and verbalize perceived problem-solving barriers to themselves and their instructors. There is likely a highly individualized level of desirable difficulty for each student where a problem is difficult enough to engage their curiosity and motivation to solve something unknown but also does not generate negative emotions associated with failure that could prevent problem solving from moving forward (Zepeda et al., 2020; de Bruin et al., 2023). The link between feelings and metacognition in the present study is paralleled in other studies that used retrospective methods and found links between feelings of (dis)comfort and metacognition (Dye and Stanton, 2017). This suggests that the feelings students associate with their metacognition is an important consideration when designing future research studies and interventions. For example, helping students coach themselves through the negative emotions associated with not knowing and pivoting to what they do know might increase the self-efficacy needed for problem-solving persistence.
What aspects of learning self-efficacy do first-year life science students reveal when they solve problems on their own?
To address our second research question, we looked for statements related to self-efficacy in our participants’ think aloud data. Self-efficacy is defined as one’s belief in their capability to carry out a specific task (Bandura, 1997). Alternatively, self-efficacy is sometimes operationalized as one’s confidence in performing specific tasks (Ainscough et al., 2016). While we saw instances of students making high-self efficacy statements (“I’m confident that I was going in somewhat of the right direction”) and low self-efficacy statements (“I’m not gonna understand it anyways”) during their think aloud interviews, we were particularly intrigued by a distinct form of self-efficacy that appeared in our data that we call “self-coaching” (Table 4). We posit that self-coaching is similar to the ideas of self-modeling or efficacy self-talk that other researchers have described in the past (Wolters, 2003; Usher, 2009). In our data, these self-encouraging statements either: 1) reassured themselves about a lack of understanding, 2) reassured themselves that it’s okay to be wrong, 3) encouraged themselves to keep going despite not knowing, or 4) reminded themselves of their prior experience. To highlight the role that self-coaching played in problem solving in our dataset, we first present examples where self-coaching was absent and could have been beneficial for the students in our study. Then we present examples where self-coaching was used.
Category | Description | Example data |
---|---|---|
High self-efficacy | Student expresses confidence in their knowledge or ability to do something. | I knew about all the pH stuff I would say pretty confidently. |
The thought processes that apply to every science class … made me feel more confident, probably than I should’ve. | ||
That’s actually a pretty good guess if I do say so myself. | ||
Low self-efficacy | Student expresses a lack of confidence in their knowledge or ability to do something. | I’m not very good with noncovalent [bonds] at all. |
No, I cannot [answer the question to the best of my ability]. | ||
I probably sound stupid. | ||
Self-coaching | Student makes a self-encouraging statement about their lack of understanding. | I don’t know what flux is. That’s okay. |
Student makes a self-encouraging statement about being wrong. | So, my strategy for this one is it’s okay to get it wrong. | |
Student makes a self-encouraging statement to keep going despite not knowing. | I’m not too sure what flux means, but I’m going to keep on going. | |
Student makes a self-encouraging statement about their prior experience. | I haven’t had chemistry in such a long time [pause], but at the same time, this is bio. So, I should still know it. |
When students monitored without self-coaching, they had hard time moving forward in their problem-solving
When solving the challenging biochemistry problems in this study, first-year life science students often came across pieces of information or parts of the figures that they were unfamiliar with or did not understand. In the Monitoring section, we described how students monitored their understanding and familiarity, but perhaps what is more interesting is how students responded to not knowing and their lack of familiarity (Figure 2). In a handful of cases, we witnessed students get stuck or hung up on what they did not know. We posit that the feeling of not knowing could increase anxiety, cause concern, and increase self-doubt, all of which can negatively impact a student’s self-efficacy and cause them to stop problem solving. One example of this in our data comes from Tiffani. Tiffani stated her lack of knowledge about how to proceed and followed this up with a statement on her lack of ability to solve the problem, “I am actually not sure how to solve this. I do not think I can solve this one.” A few lines later, Tiffani clarified where her lack of understanding rested, but again stated she cannot solve the problem, “I’m not really sure how these type of amino acids pair up, so I can’t really solve it.” In this instance, Tiffani’s lack of understanding is linked to a perceived inability to solve the problem.
Some students also linked not knowing with perceived deficits. For example, in the following quote Chandra linked not knowing how to answer the second part of the Protein X problem with the idea that she is “not very good” with noncovalent interactions: “I’m not really sure about the second part. I do not know what to say at all for that, to predict any new noncovalent, I’m not very good with noncovalent at all.” When asked where she got stuck during problem solving, Chandra stated, “The “predict any new noncovalent” cause [I’m] not good with bonds. So, I cannot predict anything really.” In Chandra’s case, her lack of understanding was linked to a perceived deficit and inability to solve the problem. As instructors, it is moments like these where we would hope to intervene and help our students persist in problem solving. However, targeted coaching for all students each time they solve a problem can seem like an impossible feat to accomplish in large, lecture-style college classrooms. Therefore, from our data we suggest that encouraging students to self-coach themselves through these situations is one approach we could use to achieve this goal.
When students monitored and self-coached, they persisted in their problem-solving
In contrast to the cases of Tiffani and Chandra shared above, we found instances of students self-coaching after acknowledging their lack of understanding about parts of the problem by immediately reassuring themselves that it was okay to not know (Table 4). For example, when exploring the arrows in the Pathway Flux problem figure Ivy states, “I don’t really know what that little negative means,but that’s okay.” After making this self-coaching statement Ivy moves on to thinking about the other arrows in the figure and what they mean to formulate an answer. In a similar vein, when some students were faced with their lack of understanding, one strategy they deployed was not dwelling on their lack of knowledge and pivoting to look for a foothold of something they do know. For example, in the following quote we see Viola acknowledge her initial lack of understanding and familiarity with the Pathway Flux problem and then find a foothold with the term enzymes which she knows she has learned about in the past, “I’m thinking there’s very little here that I recognize or understand. Just… okay. So, talking about enzymes, I know we learned a little bit about that.”
Some students acknowledged this strategy of pivoting to what they do know in their problem reflections. In their problem reflections, Quinn and Gerald expanded that they will rely on what they do know, even if it is not accurate. As Quinn put it, “taking what I think I know, even if it’s wrong, like I kind of have to, you have to go off of something.” Similarly, Gerald acknowledged his strategy of “it’s okay to get it wrong” when he doesn’t know and connects this strategy to his experience solving problems on high-stakes exams.
I try to use information that I knew and I didn’t know a lot. So, I had to kind of use my strategy where I’m like, if this was on a test, this is one of the questions that I would either skip and come back to or write down a really quick answer and then come back to. So,my strategy for thisoneis it’s okay to get it wrong. You need to move on and make estimated guess.Like if I wasn’t sure what the arrows meant, so I was like, "okay, make an estimated guess on what you think the arrows mean. And then using the information that you kind of came up with try to get a right answer using that and like, explain your answer so maybe they’ll give you half points…" – Gerald
We also observed students encouraging themselves to persist despite not knowing (Table 4). In the following quote we see Kyle acknowledge a term he doesn’t know at the start of his think aloud and verbally choose to keep going, “So the title is pathway flux problem. I’m not too sure what flux means,but I’m going to keep on going.” Sometimes this took the form of persisting to write an answer to the problem despite not knowing. For example, Viola stated, “I’m not even really sure what pathway flux is. So, I’m also not really sure what the little negative sign is and it pointing to B.But I’m going to try to type an answer.” Rather than getting stuck on not knowing what the negative feedback loop symbol depicted, she moved past it to come to a solution.
We also saw students use self-coaching to remind themselves of their prior experience (Table 4). In the following example, we see Mila talk herself through the substitution of serine with valine in the Protein X problem: “So, there’s not going to be a hydroxyl anymore, but I don’t know if that even matters, but there, valine, has more to it. I don’t know if that means there would be an effect on the covalent interaction. I haven’t had chemistry in such a long time [pause], but at the same time,this is bio. So, I should still know it.[laughs]” Mila’s tone as she made this statement was very matter-of-fact. Her laugh at the end suggests she did not take what she said too seriously. After making this self-coaching statement, Mila rereads the question a few times and ultimately decides that the noncovalent interaction is affected because of the structural difference in valine and serine. Prior experiences, sometimes called mastery experiences, are one established source of self-efficacy that Mila might have been drawing on when she made this self-coaching statement (Bandura, 1977; Pajares, 1996).
Implications for Instruction about Self-Coaching
Students can be encouraged to self-coach by using some of the phrases we identified in our data as prompts (Table 4). However, we would encourage instructors to rephrase some of self-coaching statements in our data by removing the word “should” because this term might make students feel inadequate if they think they are expected to know things they don’t yet know. Instead, we could encourage students to remind themselves of when they’ve successfully solved challenging biology problems in the past by saying things like, “I’ve solved challenging problems like this before, so I can solve this one.” Taken together, we posit that self-coaching could be used by students to decrease anxiety and increase confidence when faced with the feeling of not knowing that can result from monitoring, which could potentially positively impact a student’s self-efficacy and metacognitive regulation. Our results reveal first-year students are monitoring in a myriad of ways. Sometimes when students monitor, they may not act further on the resulting information because it makes them feel bad or uncomfortable. Self-coaching could support students to act on their metacognition or not actively avoid being metacognitive.
LIMITATIONS
Even with the use of in-the-moment methods like think aloud interviews, we are limited to the metacognition that students verbalized. For example, students may have been employing metacognition while solving that they simply did not verbalize. However, using a think aloud approach in this study ensured we were accessing students’ metacognition in use, rather than their remembrance of metacognition they used in the past which is subject to recall bias (Schellings et al., 2013). Our study, like most education research, may suffer from selection bias where the students who volunteer to participate represent a biased sample (Collins, 2017). To address this potential pitfall, we attempted to ensure our sample represented the student body at each institution by using purposeful sampling based on self-reported demographics and varied responses to the revised Metacognitive Awareness Inventory (Harrison and Vallin, 2018). Lastly, while our sample size is large (N = 52) for qualitative analyses and includes students from three different institutional types, the data are not necessarily generalizable to contexts beyond the scope of the study.
CONCLUSION
The goal of this study was to investigate first-year life science students’ metacognition and self-efficacy in-the-moment while they solved challenging problems. Think aloud interviews with 52 students across three institutions revealed that while first-year life science students plan, monitor, and evaluate while solving challenging problems, they predominantly monitor. First-year life science students associated monitoring a lack of conceptual understanding with negative feelings whereas they associated positive feelings with monitoring conceptual understanding. We found that what students chose to do after they monitored a lack of conceptual understanding impacted whether their monitoring moved problem solving forward or not. For example, after monitoring a lack of conceptual understanding, students could either not use a strategy and remain stuck or they could use a strategy to move their problem solving forward. One critical finding revealed in this study was that self-coaching helped students use their metacognition to take action and persist in problem solving. This type of self-efficacy related encouragement helped some students move past the discomfort associated with monitoring a lack of conceptual understanding and enabled them to select and use a strategy. Together these findings about in-the-moment metacognition and self-efficacy offer a positive outlook on ways we can encourage students to couple their developing metacognitive regulation skills and self-efficacy to persist when faced with challenging life science problems.
ACKNOWLEDGMENTS
We would like to thank Dr. Paula Lemons for allowing us to use problems developed in her research program for this study and for her helpful feedback during the writing process, the College Learning Study participants for their willingness to participate in this study, Dr. Mariel Pfeifer for her assistance conducting interviews and continued discussion of the data during the writing of this manuscript, C.J. Zajic for his contribution to preliminary data analysis, and Emily K. Bremers, Rayna Carter, and the UGA BERG community for their thoughtful feedback on earlier versions of this manuscript. We are also grateful for the feedback from the monitoring editor and reviewers at LSE, which strengthened the manuscript. This material is based on work supported by the National Science Foundation under Grant Number 1942318. Any opinions, findings, and conclusions or recommendations expressed in this material are those of the authors and do not necessarily reflect the views of the National Science Foundation.