Developing Student Expertise in Evolution: Cognitive Construals Complement Key Concepts in Student Representations
Abstract
Genetic variation is historically challenging for undergraduate students to master, potentially due to its grounding in both evolution and genetics. Traditionally, student expertise in genetic variation has been evaluated using Key Concepts. However, Cognitive Construals may add to a more nuanced picture of students’ developing expertise. Here, we analyze the occurrence of Key Concepts and Cognitive Construals among three types of student representations: interviews, drawn models, and constructed responses (CRs). Our mixed-methods analysis indicates that differential survival and differential reproduction occur more often in interviews than in CRs. In our interviews, presence of Cognitive Construals indicate varying levels of understanding of genetic variation, but we were not able to detect Cognitive Construals in students’ models or CRs. Finally, our analyses of both Key Concepts and Cognitive Construals in student representations indicate that Cognitive Construals can co-occur with any number of Key Concepts, and that the presence of Construal-based language alone does not seem to correlate to the expert nature of a response. Taken together, our results highlight the need for instructors to avoid treating Construal-based language as implicit disconnects in student understanding, and to use multiple methods to gain a holistic picture of student expertise.
INTRODUCTION
Genetic variation is a fundamental concept in biology education that spans Vision and Change’s Core Concepts (Brewer and Smith, 2011) of both evolution, and information flow, exchange, and storage. Extensive research has been conducted on student knowledge of normative or expert-like concepts and misconceptions around genetics (Nehm and Haertig, 2011). In the current work, we use the term “expert” in keeping with Nehm and Ridgway’s (2011) definition as follows:
Experts and novices refer to socially established categories emblematic of the degree of formal educational training and experience in the subject domain irrespective of measured knowledge or skills in the domain; expert- and novice-like refer to descriptions of behaviors, performances, or knowledge considered typical of the social categories “expert” and “novice”… (p. 671)
More recently, informal nonnormative patterns in student, or novice, biological thinking have been characterized as cognitive construals (CCs; Coley and Tanner, 2012). Here, we probe the occurrence of expert-like concepts and CCs around the core concept of, information storage and transfer, using a question about the origin and spread of genetic variation, among three types of student-constructed representations: oral interviews, written constructed responses (CRs), and drawn models.
THEORETICAL BACKGROUND
Genetic Variation in Genetics and Evolution Education
Extensive research has been conducted to identify and address student ideas of genetic variation in both the realms of genetics and evolution education. In genetics Smith et al., (2008) developed the Genetics Concept Assessment (GCA) to improve student understanding of learning goals such as mutations, heredity, phenotypic prevalence, and frequency. The GCA was able to capture significant differences in genetics knowledge between majors and nonmajors (Knight and Smith, 2010) and after instruction (Smith and Knight, 2012).
Research into students’ understanding of evolution concepts revealed several Key Concepts (KCs) and misconceptions (Nehm and Schonfeld, 2008; Nehm and Haertig, 2011). In their pioneering work, Nehm and Schonfeld (2008) summarized seven KCs and thirty-one alternative conceptions, before characterizing student use of these ideas in open ended interviews, an Open Response Instrument, and a multiple-choice instrument. Later, Nehm and Haertig (2011) summarized seven KCs from extensive previous work: 1) the existence of variation among organisms and the cause of that variation, 2) heritability of traits, 3) differential reproductive (DR) success and differential survival (DS), 4) extensive production of progeny; 5) restriction of resources; 6) competition for resources; and 7) generational changes in phenotype and genotype distribution. The first of these, the presence and cause of genetic variation, has also been captured in items in the GCA. Price and colleagues developed the Genetic Drift Inventory (GeDI; Price et al., 2014) to measure what upper-division undergraduates mastered at the intersection of evolution and genetics. To our knowledge, this is the only metric that evaluates concepts from both evolution and information flow, exchange, and storage core concepts.
Intuitive Thinking and Misconceptions in Biology Education
In addition to extensive research on evolution misconceptions (Nehm and Reilly, 2007; Nehm and Haertig, 2011), links between cognitive psychology and disciplinary knowledge have been professed in the form of three CCs (Coley and Tanner, 2012). Rather than having specific difficulty with concepts, Coley and Tanner (2012) hypothesized that learner confusion may result from long-held “cognitive shortcuts” inappropriately applied to biological concepts. The authors characterized Teleology as students’ purpose- or goal-oriented explanations for biological phenomena, such as when they state, “populations want to evolve to get better.” Essentialist thinking occurs when students overgeneralize single properties or characteristics to an entire group, such as when they claim that individuals of the same species are identical to one another. Anthropic thinking encompasses two distinct subtypes: anthropomorphic and anthropocentric (Betz et al., 2019). Anthropomorphic thinking refers to the inappropriate application of human characteristics to nonhuman entities, which can range from bacterial cells to plants and animals. Anthropocentric thinking refers to placing undue emphasis on humans during analogies or explanations. Coley, Tanner, and colleagues have conducted extensive research into the relationship between CCs and misconceptions (Coley and Tanner, 2015; Richard et al., 2017). Both studies found significant associations between intuitive thinking based on a specific CC and a misconception arising from that Construal. Adding further nuance, recent work has indicated that the distinctions between CCs are not as defined as first indicated. For example, Richard et al., (2017) found significant overlap of anthropocentric and teleological language in student writing about antibiotic resistance.
CCs themselves may have varied persistence among individuals, as research is attempting to clarify. Coley and Tanner’s (2015) work appeared to demonstrate that Construal-based thought patterns appear to be persistent across various levels of adolescence and adulthood. Coley et al. (2017) found little difference in CC preference in a written survey among eighth grade students and biology majors and nonmajors. Additionally, the authors found both misconceptions and intuitive thinking in all undergraduate levels in biology majors, but not among biology faculty. An alternative proposition (Gouvea and Simon, 2018) argued that students’ informal thinking was not as stable as originally believed. These authors found that students agreed with corrected construal-based statements and disagreed with explicit construal-based statements in ways that disproved the stability of incorrect mental frameworks. CCs may therefore, provide a nuanced fluid picture of student understanding of biological phenomena, while providing room for conclusions about the stability of incorrect or informal thought structures.
Representations as a Reflection of Student Thinking
Along with the fluidity of KCs and CCs themselves, how we ask students to represent their understanding can affect how well they are able to communicate their understanding, and how accurately we interpret their representations. External representations are essential in sciences, and potentially valuable to researchers and instructors. However, many workers have noted the differences in student products generated using different representations: forced choice versus CRs (Urban-Lurain et al., 2009; Haudek et al., 2012); written versus oral responses (Huxham et al., 2012); and more recently, drawings and models (Dauer et al., 2013; Harle and Towns, 2013; Speth et al., 2014; Dauer and Long, 2015; Hester et al., 2018). We sought to characterize the utility of each type of response for subjects individually, and aggregated as a population, by asking subjects to generate three different representations responding to the same question, about how a biologist would explain the origin and spread of genetic variation in a population.
Although scientists, mathematicians, and engineers use multiple representations in their disciplines, little work has focused on comparing how learners use different representations. Waldrip and colleagues (2006) compared how middle-school-aged learners used verbal and graphical representations of the nature of matter and the concept of force. Their findings supported the importance of the purpose and function of each kind of representation for learners: for example, to use graphical representation, people must be familiar with what axes, scales, points, and curves represent. Gobert and Clement (1999) compared primary students’ written verbal and drawn representations of plate tectonics in a pre-/postinstruction design, concluding that instruction enriched drawn representations much more than written verbal representations. In their summary of eight evolution education instruments that purported to measure knowledge of evolutionary processes Tornabene and colleagues (2018) also noted whether the response types were forced choice (multiple choice, True and False) or open (verbal) response; three of the eight instruments collected open response data. We were unable to locate any other published research about how written-text, oral, and/or drawn products represent college students’ conceptual knowledge of biological principles, nor about how college instructors use different representations to elicit student conceptual knowledge. We, therefore, sought to characterize the utility of student-constructed, written verbal, oral verbal, and drawn modalities in elucidating student understanding of the origin and spread of genetic information.
RESEARCH QUESTIONS AND HYPOTHESES
We were interested in whether and how subjects incorporate the five KCs of evolution (Nehm and Schonfeld, 2008) into their multimodal representations of genetic variation and its origins. In this work, we will not focus on misconceptions or solely naïve ideas, because they have been documented extensively in the literature (e.g., Nehm and Schonfeld, 2008; Moharreri et al., 2014). Instead, we were interested in probing the more subtle CCs. Based on our teaching experiences and established research literature, we hypothesized that there would be variation in the frequency of the KCs both within subjects (among representations), and among subjects. In exploring that research question, we posed two additional research questions. First, to what extent were CCs present in how subjects represented genetic variation and its origin; and if present, did CCs relate to the quality of the student’s explanation or model? We hypothesized that the presence of CCs would vary inversely with the number of KCs that subjects invoked. Second, how did each type of representation compare to the other representations in eliciting student understanding of these core concepts? Because comparisons among multiple representations of biological concepts are unusual, we posed the null hypothesis: that each type of representation was equally utilitarian at revealing student thinking. We understood this null hypothesis was unlikely a priori.
Incorporating our hypotheses and thoughts about KCs and CCs resulted in the following concise Research Questions:
RQ1: How do subjects incorporate the five KCs of evolution into their representations of genetic origin and variation?
RQ2: Are CCs present in how subjects represent genetic origin and variation? If CCs are present, how do they relate to the normative quality of the representations? (explanation, model)
RQ3: Subjects represented their knowledge in three different ways. How did their thinking emerge in each?
MATERIALS AND METHODS
We used multiple methods to investigate each of our research questions. Briefly, RQ1 required a mixed methods approach to compare student usage of KCs (Figure 1). RQ2 and RQ3 involved qualitative analysis of CC language and evaluation of student models. Using these methods and constructs allowed us to gain a multifaceted picture of student usage of KCs and CCs in the description of genetic variation. We used a Concurrent Nested Mixed Methods Design (Warfa, 2016) to investigate RQ1 (Figure 1). Briefly, this design begins with a qualitative data collection and analysis phase, followed by a quantitative data collection and analysis phase, which allows for extraction of conclusions from the data. We collected three types of qualitative data: 1) written verbal CRs; 2) oral verbal interviews, during which we also collected 3) drawn models. Our qualitative coding scheme allowed us to generate quantitative scores for CR item responses and interviews, which allowed us to quantitatively analyze any relationships.
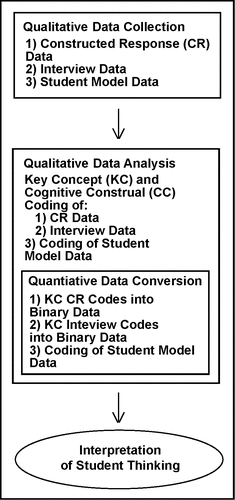
Figure 1. Our investigation of student understanding of genetic variation followed a concurrent, nested mixed-methods design.
Qualitative Data Collection
We investigated our research questions by posing our CR question, “How would a biologist describe the origin and spread of new genetic variation in a population?” (hereafter, referred to as the OA question) to students (n = 14) enrolled in an introductory college biology course at a small public Midwest land-grant university. We adapted this item from the Genetics Concepts Assessment (Smith et al., 2008), which lends our item content validity as assessing important topics in biology. We have addressed face validity because one of the authors [removed for review] has participated in many iterations of this item.
Our concurrent nested design (Warfa, 2016) asked students to represent their knowledge and understanding in three ways: CR, collecting student-generated English text; oral responses in individual interviews with one of the authors; and a model, or an original, student-generated pictorial or diagrammatic representation drawn on paper. We entered the OA question into the course management system (CMS) as an optional, ungraded, long-response question using the Quiz feature. We invited all students to participate in an oral interview with one of the authors and selected the first 14 respondents to the solicitation. Slightly more than two-third of our interview population (71%) self-identified as female (Table 1). Most of our participants were first-year students (79%). One to three weeks after students completed their online, written CR, we conducted private face-to-face semistructured interviews, recorded with written student consent (“interviews”; protocol provided in Appendix A), to explore student conceptualization of the KCs in the OA question.
Breakdown of year in college | Breakdown of gender | ||
---|---|---|---|
First-year | 79% (n = 11) | Female | 71% (n = 10) |
Sophomore | 7% (n = 1) | Male | 29% (n = 4) |
Junior | 7% (n = 1) | ||
Senior | 7% (n = 1) |
During these interviews, we also asked subjects to draw a model in response to the OA question (“models”) on a sheet of blank paper, which we collected. In the course from which we invited subjects, all subjects received regular modeling instruction in representing concepts as a part of the course curriculum. During the interview period, we explained to all subjects the purpose of the research and obtained their written informed consent. We asked subjects to talk about their models while creating them, but ultimately decided that the models were reasonably complete without needing their narration. We compensated subjects financially for their time. We completed all human subjects investigation under [removed for review] University’s IRB authorization 1809004-EXP.
We transcribed recordings of the interviews. One of the researchers (K.S.) split interview transcripts into segments which we defined thus: “interviewer question” + “respondent answer” (+ “follow-up question” + “follow-up answer” if the initial “respondent answer” was one word). Each interview contained an average of six segments, with a range of three to ten segments.
Because of the wealth of research on both KCs and CCs to date, we decided to use a structural coding (Saldaña, 2021) approach to our interview segments. We, therefore, developed a blended codebook that allowed us to simultaneously apply KCs and CCs to each interview segment. Our definitions of KCs (Table 2) were based on Nehm and Schoenfeld (2008) and included these five KCs: presence of variation (PV), cause of variation (CV), heritability (H), DR success, and DS. We expanded on Nehm and Schoenfeld’s (2008) “variation” to differentiate between the presence and CV. We further refined “DS” so that DS and reproductive success were distinct concepts. To this codebook, we also added codes for the three CCs (Table 2) from Coley and Tanner (2012): anthropocentrism, essentialism, and teleology.
Code | Abbreviation | Definition |
---|---|---|
Key Conceptsa | KCs | |
presence of variation | PV | Verbal or written responses identify that genetic variation in a population, typically by comparing two alleles or phenotypes |
cause of variation | CV | Responses identify that variation is caused by mutation |
heritability | H | Responses identify that genes are particles passed from one or both parents to offspring |
differential survival | DS | Responses identify that different genes or alleles allow the organisms that possess them to survive longer in the current habitat |
differential reproductive success | DR | Responses identify that different genes or alleles allow the organisms that possess them to produce more offspring in the current habitat |
Cognitive Construalsb | CCs | |
Teleology | T | Responses attribute a goal or purpose to a non-human entity (e.g., an animal, a gene, or natural selection itself) |
Essentialism | E | Responses indicate a single feature or property of an entity determines its overall function or behavior. |
QUALITATIVE DATA ANALYSIS
Coding Interviews
One author (K.S.) randomized interview segments within each individual’s interview. Using subsets of the segments within one to two interviews at a time, both researchers independently coded each segment for the presence and identity of each of the five KCs and CCs. The PIs independently used this codebook to code interview segments, individually in rounds of one to two interviews, where presence of a code in a segment was coded with a “1” and absence was coded with a “0” in a Microsoft Excel workbook. We then met to compare codes and discuss disagreements until we could agree on a unified set of codes for each segment. This resulted in a consensus set of KC and CC codes for each segment in each interview, on which we based our subsequent analyses. The code descriptions for each KC and each CC were nonoverlapping. We repeated this iterative cycle of coding and refinement eight times, until all segments were coded to consensus or near-consensus. During this process, we updated the KC “reproductive success” to “DR success,” as we realized this distinction was important to understanding the limits of student concept representation. We also found during the coding process that the CC anthropocentrism was never represented in any of our data sets. We applied the same codebook to each subject’s written CR (finalized codebook in Table 2).
Student Model Analysis
Because our codebook was difficult to apply to drawn models, we used an approach like previous (unpublished data) work we have completed, using a three-level scheme for the evaluation (Kanchupati et al., 2018). We rated a model “normative” if it included ≥ 3 of the five KCs; “incomplete” if the model contained two KCs, or lacked clarity, or completeness; and “nonnormative” if it included ≤ 1 KC. As before, we randomized the order in which we coded models, and each author coded individually before comparing our determinations.
Quantitative Data Analysis
Once we reached consensus in our qualitative coding of CR and Interview samples, we transformed these data into quantitative information. Each CR item response, each complete interview, and each complete model were considered “samples” in our experimental design. CR items and interviews could each potentially contain KCs and CCs. When present, each KC or CC was designated with a “1”; absence corresponded to “0.” We sought to conduct a similar quantification with CCs, but we did not detect CCs in CRs or models. Using these quantitative data, we performed Wilcoxon Signed Rank Tests to explore within-subject differences in how subjects represented the OA question, whether by CR or by interview. This mixed-methods analysis allowed us to deeply investigate student usage of KCs in multiple representations of genetic variation.
RESULTS
RQ1: How do subjects incorporate the five KCs into their representations of genetic origin and variation?
Because of extensive existing research into student understanding of evolution, we conducted parallel mixed-methods analyses on the occurrence of KCs (Table 2) described by Nehm and Schonfeld (2008). We focused on three means of representation: 1) oral semistructured interviews; 2) CR items, which the subjects completed before being interviewed; and 3) modeling or asking subjects to draw their models of the OA question during the interview. We began by identifying expert representations in interviews, models and CRs independently (Table 4, green cells). Expert representations in interviews were indicated by both presence of all five KCs and no CCs. Interviews from subjects 3, 13, and 14 met this criterion (Table 4, green cells). We found the most-used KCs were “PV” (Table 2; 14 out of 14 interviews; Figure 1, blue bars), “H” (Table 2) and DS (Table 2; 13 out of 14 interviews each). “DR success” (DR, Table 2) was the least-used KC (nine out of 14 interviews).
Four subjects (2, 3, 10, and 14) drew normative models that included three or more KCs (Table 4, green cells). Three of those four subjects’ models included all five KCs. Six subjects (4, 5, 8, 11, 12, and 13) drew incomplete models, with two KCs. Four subjects (1, 6, 7, and 9) drew nonnormative models, including 0 or 1 KCs.
Finally, we analyzed each subject’s CR, collected before interviews, for presence of KCs. Compared to the interviews, these showed a sparser occurrence of KCs, with only one CR (that of student 2) including all five KCs (Table 4). As in interviews, “PV” occurred in almost all CRs (13 out of 14; Figure 2, orange bars), while “DR success” occurred infrequently (three out of 14 CRs). In contrast to interviews, “CV” (Table 2) was also frequently invoked in CRs (14 out of 14), while DS was an additional infrequent KC (two out of 14 CRs). We attempted to qualitatively evaluate whether model correctness had any relationship to prevalence of KCs in interviews but found no relationship.
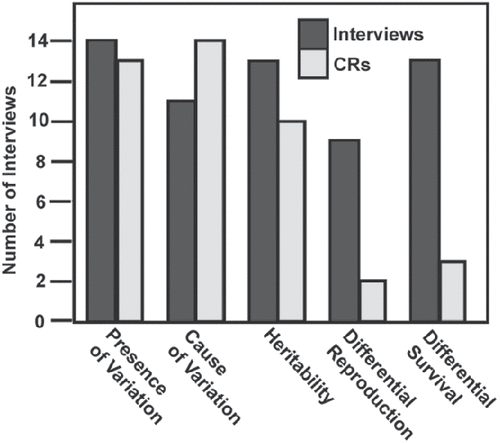
Figure 2. Population frequency of individual KCs in interviews (blue bars) and CRs (orange bars).
In our interviews, we found that PV was represented in all 14 interviews, with H and DS occurring in 13 out of 14 interviews each (Figure 2). In contrast, DR was represented in just over half the interviews (nine out of 14). For CRs, we found that “PV” (Table 2) and “CV” (Table 2) occurred in all or almost all CRs, while DS and DR were rare in CRs, appearing two and three times, respectively. Frequency of student mentions of DR varied substantially between oral interviews and written CRs (nine and two, respectively), as did frequency of mentions of DS (13 and three, respectively; Table 5).
The fact that we had two linguistic representations (CR and interviews) of student thinking at distinct time points for each student allowed us to investigate whether the two representations had any congruence between one another. We used paired Wilcoxon signed-rank tests to compare KC usage in interviews and CRs (Table 5). DS and DR showed significant (p ≤ 0.02 and p ≤ 0.002, respectively) ability to predict model quality and KC presence in interviews (DS = 9 and DR = 13 interviews, respectively) compared with CRs (DS = 2 and DS = 3 CRs out of 14, respectively).
Our mixed-methods analysis of our interview data allowed us to discover differences in how subjects used qualitative KCs in spoken, written, and drawn representations of genetic variation. Our follow-up quantitative analysis showed that KCs differ significantly in their usage in subjects with normative and nonnormative representations.
RQ2: Are CCs present in how subjects represent genetic origin and variation? If CCs are present, how do they relate to the normative quality of the representations?
We next investigated whether and how CCs (Table 2) occurred in student oral and written responses regarding genetic variation. We only found occurrences of CCs in interviews, not in subjects’ models or CRs. This trend may reveal the power of interview settings to reveal subtle distinctions in student thinking that are less easily accessed using written responses or drawn representations. In total, seven of 14 subjects’ interviews contained CC language. We found teleological (T) language in five out of 14 interviews, and essentialist (E) language in four out of 14 interviews. Two interviews contained both T and E CCs.
We found both unambiguous and more nuanced uses of CCs language in student interviews, indicating that students can hold CCs alongside their developing normative KCs in their minds. A predominant trend we observed was that subjects’ verb choices indicated that concepts in evolution had inherent agency. For example, a common teleology was natural selection “choosing” or “favoring” certain traits, such as in the segment from Subject 4 below. All quotes have been lightly edited for readability, with author-added emphasis (bold and underlined).
Interviewer: So first if you could take a look at this question that may be related to some content that you’ve seen either this semester or last semester. And if you could read over it, you don’t have to read it out loud if you don’t want to. If you could talk through your answer to the two parts.
Subject 4: So, um, the origin of new traits, I think it’s mutations. So, like, um, they just happen randomly in the genes. And if there’s a mutation that codes for something that, uh, gives you a trait that’s something, like, beneficial, and um, so, like, natural selection will choose that trait because that lets you survive more and reproduce more. So, yeah, that’s how it spreads. And where it comes from.
We observed this type of language in three subjects (4, 10, and 12; Table 4). Of these three, two subjects were able to explain the normative scientific principles behind the informal language. A similar use of language occurs when subject 12 described genetic variation:
Interviewer: Great, thank you. Can you describe what happens to the other form of the gene? You know, like, the predators that have the less camouflaged um, [overlapping speech] color. Yeah.
Subject 12: Um, well, I think that is- uh, I think that is probably, in my opinion, the basis of evolution. You know, those species, I think, in my opinion, are the ones that- that are at a- at a disadvantage compared with the new species that are, you know, the- this evolved species in my opinion. I think uh, some of these variations in the alleles can definitely pave way for evolution, um, because that’s how, you know, you know, a species is just trying to evolve, just trying to get better and these, uh, these specific organisms who do not have this variation might be at a disadvantage because they can be seen more by other- their prey, so [clears throat] I feel like they can be left behind and eventually you know, die out and [overlapping speech] then, you know. Yeah.
Similarly, two subjects (2 and 6; Table 4) used Essentialist language to describe the mechanism of natural selection. Subject 6 states a similar Essentialist heuristic about evolution in a population:
Interviewer: Do you see the trait being passed down in animals with a similar mechanism, or would it be slightly different, or completely different?
Subject 6: Yeah, with animals it would be a similar concept of one generation to the next, and then, obviously, the strong survive and the weak die out.
When probed, the student responds with another essentialist heuristic rather than applying normative scientific principles. This indicates that this student probably has an incomplete understanding of natural selection:
Interviewer: Then that’s [strong survive and weak die out] because um, because that trait kind of helps them deal with their environment better than the individuals that don’t have that trait?
Subject 6: Right. It’s kind of like how you never see askinny polar bear.
Subject 2 also states the common axiom “survival of the fittest” when discussing natural selection, with a slight variation from subjects 4 and 6:
Interviewer: Can you talk a little bit about how natural selection is related to the origin and spread of new alleles if at all?
Subject 2: I would say like we were discussing earlier, natural selection is nature’s way of survival of the fittest, just the species that are the strongest and have the best genetics and traits and are more likely to survive because they’re more likely to get resources that competing against amongst animals in their same species group and in the ecosystem, …so all animals are competing for those things [resources], even with plants … [resources are] all highly competitive, so only the best are able to survive, and the weak, or the ones that are unable to get these resources, die. So natural selection is kind of nature’s way of over time, um, as um, kind of selecting for, or you know, the way that just the stronger ones over time, kind of become more prevalent because they stand the test of time, while the weaker ones do not, and part of the way, um, you know, I guess, over time, natural selection, the evolution – or they evolve, is when there are these new random mutations. If it is beneficial, then those, um, ones are kind of the ones that do get the resources that are in high competition. therefore, survive, are naturally selected for, and kind of then, just the randomness of the mutation, if it is, um, proving to be advantageous for the animal, or the animal or the species, they will have a competitive advantage over the others in their ecosystem, and are there going to survive over time and naturally selected for.
However, the last few sentences of Subject 2’s quote (“If it is beneficial, then those…are…the ones that do get the resources…” and onward) indicate a normative understanding of natural selection. The fact that our subjects are sometimes able to explain the CC shorthand they use indicates that they have reconciled the simplified Essentialism with normative scientific concepts. Thus, students may be able to explain such shorthand with varying success.
We also observed more subtle examples of CC language in our interviews. These examples were interesting because the language may be interpreted as scientific explanations by those without an expert-like understanding of the origin and spread of genetic variation. The following example from Subject 6 exhibits this trend, using Teleological language:
Interviewer: All right, so, um, first what we’ll do is just have you, uh, look over this question that should be related to some concepts that you’ve seen, maybe last semester and this semester in your biology classes. You don’t have to read the question out loud, but if you could, once you’ve read it, talk through how you would answer each part of the question.
Subject 6: New traits within a population can either be due to adaptation, like how some leaves develop little hairs on them to prevent water loss, or to affect how the wind blows across it, to manipulate water loss and photosynthesis. Um, they can also be …we talked about antibiotic resistance and adapting to something it’s been exposed to for so long, to try and overcome that. Similar with the superweeds that everyone is afraid of, with GMOs and developing the genes that are in one population could crossover to another through pollination or other preproductive [sic] aspects.
This segment exposes two distinct nonnormative ideas in the subject’s understanding. First, the student describes genetic variation as adaptation, a common novice understanding. Second, the student oversimplifies how antibiotic resistance occurs (“…antibiotic resistance and so just, adapting to something it’s been exposed to for so long…”).
In summary, the in-depth nature of our interviews revealed different types of CC usage that were absent from both our models and CRs. Sometimes common Essentialist language was accompanied by a more scientific explanation, indicating that perhaps the student did possess a more expert-like understanding of the phenomenon. In other, more subtle cases, subjects used Construal-based language in ways that indicated a deep understanding but could not offer a more sophisticated explanation upon further probing. Our results therefore suggest that CC language itself is not indicative of nonexpert understanding, and that other aspects of student representations should be considered to gain a full understand of the student’s understanding.
RQ3: Subjects represented their knowledge in three different ways. How did their thinking emerge in each?
Having investigated both subjects’ normative and informal ideas of genetic variation, we next turned to probing the trends we observed among our three representations of student thinking. How do KC and CC language co-occur in subjects’ representations, and what might the cooccurrences tell us about students’ developing expertise in this topic? We began by investigating how KCs and CCs were distributed across subjects’ interviews and CRs, and how these metrics aligned with normative models (Table 3). We focused on the five subjects that exhibited all five KCs in their interviews (3, 4, 10, 13, and 14; Table 4). We observed that these subjects did not necessarily display all KCs in CRs or have normative models. Three subjects (3, 10, and 14) drew normative models. For example, Subject 3’s flow chart model (Table 6, left column) invokes all five KCs by showing how an organism has a random mutation that generates a new trait (CV) which leads to offspring production with that new trait (H). The offspring with the new trait is then selected for by the environment (DS), which will lead to a higher incidence of the new trait in the population (PV). Those offspring that do not have the new trait will result in a lower proportion of the population, eventually dying off (DS). Two other subjects who invoked all five KCs, four and 13, drew incomplete models (Table 3). For example, although Subject 4 did depict a mutation in DNA (CV), they incorrectly indicated that a beneficial mutation would be passed down (H) while a nonbeneficial mutation would not be passed down. This student did not mention DS or DR of organisms with the beneficial trait, or the PV within the population. All five of the five-KC subjects generated CRs with three KCs. Four out of these five subjects were missing DR and DS in their CR’s (3, 4, 13, and 14), while the fifth subject missed H and DS (Subject 10).
Code | Description |
---|---|
Normative | Model contains ≥ 3 KCs |
Incomplete | Model contains 2 KCs |
Non-normative | Model contains ≤ 1 KC |
![]() |
Interestingly, there was no apparent correlation between the occurrence of CC language and the occurrence of KCs. Six out of the seven interviews containing CC language also contained a high number of KCs (Table 4: four KCs = subjects 1, 2, 6, 9, and 12; five KCs = Subject 10). Two Teleological interviews contained all five KCs, with the remaining CC interviews containing at least three KCs. Similarly, there seemed to be no pattern regarding the presence of KCs in CRs for interviews containing CC language. Only one Essentialist interview (Subject 2) contained all five KCs in their CR. One T interview (Subject 12) contained four of five possible KCs in their CR. The rest of the interviews containing CC language contained three KCs in their CRs (1, 4, 6, and 10) except for Subject 9, whose CR contained only two KCs. Finally, there seemed to be no relationship between presence of CC language in interviews, and model normativeness. Subjects 2 and 10 produced Normative models despite having E and T language in their interviews, respectively (Table 3). Subjects 4 and 12 exhibited T language and drew Incomplete models, while the rest of the interviews containing CC language produced Incorrect models despite exhibiting a mix of CC language types in their interviews (E language in Interview 9; E and T language in Interviews 1 and 6).
A few examples (Table 7) illustrate the complex interplay between CCs and model representational quality. For example, Subject 2’s segment (in Table 7 and above) exhibits the Essentialism heuristic of survival of the fittest when discussing natural selection. However, their model exhibits all five KCs in a very complete manner. Additionally, their CR contains all five KCs. This may indicate either that Subject 2 believes that the mechanism of survival of the fittest involves the interplay of all five KCs in the origin and spread of new alleles; or that the subject was just using shorthand during the interview. In contrast, subject 12 exhibits Teleological language (example in Table 7 and above) when describing a species “…trying to evolve”. Their interview contains only three KCs, and they created an Incomplete model during the interview. However, their CR does include all five KCs. This different pattern may imply that the student did at one point carry all five KCs in their mind and forgot a few during the interview, filling in the gaps with convenient shorthand. An alternative explanation may be that more targeted prompts during the interview would have resulted in the student’s adding in the missing KCs.
Our results indicate that KCs and CC language frequently co-occurred in our student population, and do not appear to be directly related to the quality of other representations. Additionally, our results show that CC language can (and does) coexist with sophisticated explanations and normative models for students whose expert understanding of the OA question is still under construction. Furthermore, subjects who demonstrated knowledge of any or all the five KCs did not uniformly use them across the three representations of their knowledge.
Discussion
After instruction about the origin and spread of genetic variation, 14 beginning undergraduate biology students represented their understanding of the core concept to a common prompt, each in three different ways. None of the 14 Subjects produced three completely normative representations (Table 4). This is unsurprising given that these subjects were enrolled in their second semester of introductory college biology. Still, just four subjects (29%) drew fully normative models, with ≥3 KCs (KCs) present. Just one student (3%) wrote a CR invoking all five KCs; another student wrote a CR invoking four of five KCs – together, amounting to two out of 14, or just 7% of written responses. However, under semistructured interviews, 13 out of 14 subjects were able to invoke at least four of five KCs. Taken together, we conclude from these observations that written or drawn responses were incomplete representations of student knowledge at the introductory college-biology level. Semistructured interviews, closely related in their purpose to the discourse that happens within active biology classrooms, were highly successful at evoking student understanding, evidenced by the high prevalence of KCs among subjects, by the presence and frequency of KCs within subjects, and because they were the only representation to evoke CCs.
RQ1: How do subjects incorporate the five KCs into their representations of genetic origin and variation?
There are five KCs in Nehm and Schoenfeld’s (2008) model, but the frequency of student mentions of KCs varied among written verbal (CR), drawn (model), and oral verbal (interview) representations (RQ1). Oral interviews were most successful at evoking the highest frequency of KCs within all but one subject (Table 3: subject 12). Many subjects invoked many of the five KCs: prevalence of the KCs was all ≥64%, or ≥9 out of 14 subjects. Subjects mentioned two KCs, PV and CV, with high frequency in both interviews and CRs – regardless of whether the subject’s representations were normative overall (Table 3). In other words, novice students with nascent expertise are as likely to mention PV and CV as students whose mental models are more complete and more normative. Hence, the presence of these two KCs has little value in discriminating between novice and advanced understanding for instructors or researchers.
Subjects mentioned one KC, H, with relatively high frequency in interviews, and less frequently in CRs (Table 5). This is likely because of our small sample but could indicate that some students invoking this KC will have normative understanding of the origin and spread of genetic variation, and some will not yet have that normative understanding. Instructors may conclude that students who mention H have normative conceptual understanding but should probe further.
Key Concept | Percent Usage in: | P value (*signif) | |
---|---|---|---|
interview | CR | ||
presence of variation (PV) | 100% (n = 14) | 93% (n = 13) | 1 |
cause of variation (CV) | 79%(n = 11) | 100%(n = 14) | 0.15 |
heritability (H) | 93%(n = 13) | 71%(n = 10) | 0.15 |
differential reproductive success (DR) | 64%(n = 9) | 14%(n = 2) | 0.02* |
differential survival (DS) | 93%(n = 13) | 21%(n = 3) | 0.002* |
Subject Model | Subject CR (KCs underlined by authors) |
---|---|
Subject 3: Normative Model
![]() | Subject 3 CR: Three KCsOrigin: A new trait comes from a random mutation in the DNA of an organism. For the mutation to arise and benefit the organism, the mutation must happen in the part of the DNA that codes for essential proteins.Spread: To have the mutation spread in a population, the mutation must benefit the species. If it does, the organisms with the best fit due to the mutation will live to pass on the mutation to their offspring. As long as the [sic] mutation benefits the species, the species will see an increase in the allele frequency of the mutation. |
Subject 10: Normative Model
![]() | Subject 10 CR: Three KCsOrigin: New traits originate in a population either from a random mutation or by being brought in [sic] from an individual that immigrated from an outside population.Spread: Traits spread when they offer some sort of advantage to the individual that allows it to reproduce more and spread the trait. |
Subject 14: Normative Model
![]() | Subject 14 CR: Three KCsOrigin: New traits originate from mutations that occur randomlySpread:Reproduction, especially selective mating, spreads new traits |
Subject 4: Incomplete Model
![]() | Subject 4 CR: Three KCsOrigin: The origin would be from a mutation that would cause a new trait.Spread: The mutant gene will pass down to future generations if it is beneficial. |
Subject 13: Incomplete Model
![]() | Subject 13 CR: Three KCsOrigin: The origin of a new trait in a population could be in the form of a mutation on a gene, such as a point mutation. This mutation causes a change in that individual’s [sic] DNA that through the process described below can be introduced into a population.Spread: This would spread through the process of reproduction; it could get carried on from generation to generation. This occurs because the DNA from both parents comes together to form an offspring and if that specific trait is expressed it could continue to carry on if they continue to keep reproducing. This could eventually cause an evolutionary change in the population. |
CC Interview Excerpt | Model | CR |
---|---|---|
Subject 2: Four KCs, Essentialism CCInterviewer: Can you talk a little bit about how natural selection is related to the origin and spread of new alleles if at all? Subject 2: I would say like we were discussing earlier, natural selection is nature’s way of survival of the fittest, just the species that are the strongest and have the best genetics and traits and are more likely to survive because they’re more likely to get resources that competing against amongst animals in their same species group and in the ecosystem, food is limited, space is limited, water is limited, so all animals are competing for those things, even with plants, sunlight, to get to the sunlight, the water, the nutrients in the roots is all highly competitive, so only the best are able to survive, and the weak, or the ones that are unable to get these resources, die. So natural selection is kind of nature’s way of over time, um, as um, kind of selecting for, or you know, the way that just the stronger ones over time, kind of become more prevalent because they stand the test of time, while the weaker ones do not, and part of the way, um, you know, I guess, over time, natural selection, the evolution – or they evolve, is when there are these new random mutations. If it is beneficial, then those, um, ones are kind of the ones that do get the resources that are in high competition. therefore, survive, are naturally selected for, and kind of then, just the randomness of the mutation, if it is, um, proving to be advantageous for the animal, or the animal or the species, they will have a competitive advantage over the others in their ecosystem, and are there going to survive over time and naturally selected for. | Subject 2 drew a normative model (all five KCs; highlighting is the subject’s):
![]() | Subject 2 CR: Five KCsOrigin: Origin of new traits come into the population from random individual mutations. From my understanding and what we have learned in class so far, new traits are a result of mutations. Mutations happen when DNA is replicated and/or translated incorrectly. This can be the result of insertion or deletion, misreading a three-nucleotide base set, or most likely substitution of a nucleotide. Just by adjusting one nucleotide can change an entire protein and thus lead to a mutation, as seen in the case of sickle cell. This is how a new trait can gain its origin.Spread: A new trait is spread into the population when the individual who had the mutation happen to them (origin) reproduces and genetically passes on the new trait/mutation to their offspring. If the mutation is advantageous, or in other words yields some benefit to the organism, individuals with the new trait/mutation will be more likely to survive, thus more likely to reproduce, and thus will cause the new trait to continue to be passed on from parent to offspring. If the new trait is harmful to the organism, individuals with the mutation are more likely not to survive and thus the mutation will eventually come to an end. |
Subject 12: Three KCs, Teleology CCInterviewer: Great! Thank you. Can you describe what happens to the other form of the gene? You know, like, the predators that have the less camouflaged um, [overlapping speech] color. Yeah. Subject 12: Um, well, I think that is- uh, I think that is probably, in my opinion, the basis of evolution. You know, those species, I think, in my opinion, are the ones that- that are at a- at a disadvantage compared with the new species that are, you know, the- this evolved species in my opinion. I think uh, some of these variations in the alleles can definitely pave way for evolution, um, because that’s how, you know, you know, a species is just trying to evolve, just trying to get better and these, uh, these specific organisms who do not have this variation might be at a disadvantage because they can be seen more by other- their prey, so [clears throat] I feel like they can be left behind and eventually you know, die out and [overlapping speech] then, you know. Yeah. | Subject 12 drew an incomplete model (Two KCs: H, DS)
![]() | Subject 12 CR: Four KCs (PV, CV, H, and DS)Origin: gene mutation, natural selection, genetic drift, and gene flow.Spread: In terms of natural selection – the traits that have helped in survival, advantageous traits are passed on successfully through generations and cause a change and spreading of a new trait in the population. This is the basis of adaptation in species. Traits that are new can occur when genes are transferred between populations like migration. this can also happen between species as in the case of horizontal gene transfer. |
In their CRs, subjects mentioned three KCs significantly less frequently than with interviewer probing in interviews: DR, and DS. These KCs have significant discriminatory value (p ≤ 0.08; Table 5) for instructors and researchers interested in students’ mastery of the origin and spread of genetic variation. Because of the nature of drawn models, it was difficult for us to code these models at the grain of individual KCs. We estimated the presence or absence of KCs in each model and tallied (counted) their frequency for each student.
RQ2: Are CCs present in how subjects represent genetic origin and variation? If CCs are present, how do they relate to the normative quality of the representations?
CCs (Table 2), those simplifying but nonnormative constructs that novices invoke as they learn scientific principles, were present, but with lower prevalence, and no discernable association with KCs. Students make opaque simplifying assumptions of particular types – opaque to both students and, sometimes, instructors – about their conceptual understanding, whether they explain or draw in response to questions about concepts. CCs showed no relationship to the frequency with which subjects invoked KCs in oral or written verbal representations, nor the evaluation of their model representations. Hence, two subjects who drew normative models invoked ≥ 3 KCs in their CRs, and four to five KCs in interviews – yet still expressed one or more CCs (2 and 10). In contrast, subjects who drew nonnormative or incomplete models composed the majority of those who did not express Teleologies or Essentialisms during the oral interviews. Both examples illustrate how subtle disconnects in student understanding may occur even when a student is well along their way to normative understanding of this concept. It also illustrates the importance of oral expression and discourse for revealing CCs, which can sometimes be “mental snags” in the fabric of student understanding of fundamental core concepts. Sometimes compounding the issue is that experts can invoke cognitive-construal-like language when explaining concepts, perhaps in attempts to simplify or provide access to complex ideas (Betz et al., 2019). Such language may inadvertently reinforce incorrect beliefs about agency and purpose related to genetic variation and its spread.
CCs appear to provide an additional, subtler dimension to thinking about student understanding rather than just the extremes of present and missing KCs versus incorrect conceptions. The results from our interviews indicate that students incorporate language that is expert-like or expert-adjacent when discussing evolutionary and genetics topics. Further probing is needed to know if students understand the words they use, or if they are merely parroting back a mixture of instructors’ speech and language from everyday life to “sound correct” (e.g., “procedural display”; Bloome et al., 1989).
RQ3: Subjects represented their knowledge in three different ways. How did their thinking emerge in each?
Finally, we compared three different forms of representation in eliciting student understanding of the origin and spread of genetic variation. This comparison revealed the relative strengths of the representation types. Similar to prior work (e.g., Nehm and Schonfeld, 2008; Beggrow et al., 2014), we noted that our interviews gave us a richer picture of student thinking than did our other two representations for several reasons. First, as Beggrow and colleagues (2014) also described, our ability to ask follow-up questions in our interviews revealed student concurrent use of KCs and CCs. This deeper probing revealed that, while some students use Construal-based language because they have misconceptions, others sometimes use this type of language even while knowing the underlying scientific principles. Second, and more notably, the interviews were our only representation to reveal Construal-based thinking at all (Table 4). Nehm and Schonfeld (2008) similarly found that their interviews revealed, while not necessarily a greater number, more nuanced portrayals of alternative concepts. While CRs allowed us to evaluate KCs similar to interviews, we did not observe Construal-based language in this representation. This may be because an interview setting sometimes encourages students to speak more informally than their written voice, but would be an interesting area for further study. Lastly, the drawn models afforded the least detailed instances of KCs; indeed we counted models with only three KCs (or more) as normative models.
We were somewhat surprised that we did not find many examples of comparing students’ understanding between or among different representations. What few comparisons between or among representations existed in mathematics education research reinforced what we observed: after instruction, students are successful first with oral verbal, then written verbal explanations, with models and diagrammatic representations least successful – even when students received long-term and sustained instruction on representing concepts with models. Our work builds upon previous research on students’ representations of genetic variation. For example, Bray Speth and colleagues (2014) found that, with instruction, students’ representations of mutations improved during a semester, but that students typically still had trouble mastering the concept. Our study, although focused on a single snapshot rather than an entire semester, shows similar struggles and heterogeneity in student thinking, as seen in differences in KC count and the presence of CCs in our three-representation sets.
Our work additionally provides deeper insight into progression of expertise in evolution. Work from Tanner, Coley and colleagues (e.g., Coley and Tanner, 2015: Richard et al., 2017) has revealed some association between CCs and misconceptions in students, indicating that the former may cause the latter in certain situations. The authors’ recent work, however, has also indicated that Construal-based language is even present in instructors (Betz et al., 2019), and thus may be a feature excluded by expertise. To provide broader context, Beggrow and Nehm (2012) presented a theoretical framework where experts were defined as using only KCs in their explanations, emerging experts were defined as using some but not all KCs and no naïve ideas, and novices were defined as using naïve ideas with or without KCs. Our work may begin to hint at a more complex layer of such an expert-novice continuum. For example, Subject 6 in the essentialist examples of quotes (p. 17) above did not exhibit any naïve ideas or misconceptions (although the latter appeared in the same student’s quote using teleological language on page 19). Therefore, based on the student’s essentialist quotes, they may be classified as an emerging expert using Beggrow and Nehm’s (2014) theoretical framework. However, when probed, the student was not able to provide a deeper underlying explanation, and almost appeared to be using essentialist language to cover up their lack of more nuanced understanding. In contrast, Subject 2 (also quoted above) used similar essentialist language, but was able to more fully explain the underlying concepts. Subject 2 also did not use naïve ideas or misconceptions in this segment, and may also be categorized as an emerging expert like Subject 6. Both subjects may have acquired the phrasing in their vocabulary in the same context, for example from instructors in class (e.g., Betz et al., 2019), or from popular culture. However, our interviews clearly showed that the Subject 2 gave more expert-like explanations, indicating perhaps that presence of KCs and indeed, even of Construal-based language, should not be the only criteria for determining expertise. Such subtleties in expertise were also observed by Betz and colleagues (2019), who identified some Construal-based language in scientifically accurate statements of instructors.
Caveats
There are several caveats for our study. Our small sample size was small, indicating that not all our conclusions may be generalized. A study with a larger sample size might clarify types and usages of Construal-based language. For example, how common is it for students to use Construal-based language in conjunctions with misconceptions versus KCs? Are these trends consistent across lower- and upper-division undergraduates? Across multiple modes of higher education? An additional limitation is the grain size of our metrics. Almost all students used four or more KCs in interviews, and more than half of students used three or more KCs in CRs. A finer-grained rubric may reveal greater subtlety in student use of KCs, an important area for future study. Due to the nature of drawn models, our model evaluation rubric for the models is even coarser-grained. Future investigations of model construction could include think-aloud interviews focused specifically on students’ thought processes during model construction to capture these finer-grained processes.
Some alternative explanations also exist for our results. Similar to previous trends (e.g., Beggrow et al., 2014), we may have elicited, on average, more KCs in interviews than in CRs due to the increased active time that students spent in interviews versus completing CRs or models. Additionally, participants may have experienced a priming effect during interviews, which may explain some variations in occurrence of KCs among the representation types.
Implications for teaching and research
Our work expands on instructor knowledge about student challenges learning about the origin and spread of genetic variation in two new ways. First, we demonstrate that not all KCs are equally challenging for students to learn. PV and CV were present in both verbal representations (CRs and interviews) in high frequency, regardless of other KCs and CCs. This indicates that students incorporate these concepts more easily, at least in this population. H showed up in most subjects’ verbal responses, but relatively less frequently in CRs. This may indicate that either the KC is either not yet fully incorporated or recognized as a KC by some students and requires explicit elucidation from instructors. DS and DR success (DS) showed the greatest difference in prevalence between CR and interview. Even with prompting, only approximately two out of three subjects invoked DR in their explanations. Instructors would be wise to focus the balance of their instruction on these two KCs.
Next, we show that the type of representation we ask students to make influences their success – and hence, the conclusions that instructors and researchers may draw. Not surprisingly, an approach that asks students to produce multiple representations gives the most detailed picture of student learning. For instructors of medium- and large-sized courses, it is not possible to evaluate every response and provide individualized feedback. However, asking students to respond to similar prompts in pairs or small groups provides students the opportunity to practice more expert-like explanations, and instructors can use these results to target feedback to CCs or missing KCs. For example, instructors could pose the questions: “If you heard yourself saying ‘a population evolves because it needs to adapt,’ what is a more scientific way to say that?” (addressing the CC) or “What did you and your neighbor decide was the role of reproductive success or survival in natural selection?” (addressing the KC). By having targeted, probing questions ready, instructors can still give students the ability to evaluate their explanations. Using similar questions from the (AACR) ACORNS question pool. Moharreri and colleagues’ work (2014) also affords instructors the opportunity to evaluate their course population’s invoking of KCs.
CCs are particularly “sticky,” both in terms of our success evoking them, and in terms of their persistence. They did not emerge in either CRs or models, only interviews. They also did not seem to follow any pattern in terms of whether they were more prevalent with novice students or students closer to mastery. Researchers and instructors should be aware that they exist, across the student expertise spectrum. While they may sometimes simplify conversations with other colleagues who are already experts, we should be particularly mindful of listening for them and not invoking them in our explanations in discourse with students. One interesting question for further research is how we could distinguish between the expert use of what sounds like a CC as a shortcut, and a nonexpert’s use of a CC that indicates incomplete knowledge. Is there some diagnostic threshold of KCs that can assure a listener that the respondent’s knowledge is expert-like?
At the introductory college level, especially in large to very large enrollment courses where individual attention may be impossible, instructors may wrestle with the best ways to communicate the facts or ideas of a core concept, perhaps based on the implicit assumption that all KCs are equivalently “easy” or “hard” for students to grasp and assimilate. Our work shows that students do not typically struggle with the origin and heritability of variation, but they do struggle with assimilating DS and differential reproduction. This suggests that a teaching approach targeted to DR and DS, along with what incorrect but convenient CCs sound like, will most benefit the greatest number of students. It is also a reminder to instructors that the allegories we use matter, and that accuracy both in concept and processes invoked is a worthy intellectual challenge for us.
ACKNOWLEDGMENTS
We thank R.-A. Dibbs, K. Haudek, M. Orth, and M. Urban-Lurain for their careful reviews that significantly improved this manuscript. We also thank two anonymous reviewers for helpful comments that strengthened the manuscript. Any errors of fact or omission are our own.