How Is Science Being Taught? Measuring Evidence-Based Teaching Practices across Undergraduate Science Departments
Abstract
While there is a wealth of research evidencing the benefits of active-learning approaches, the extent to which these teaching practices are adopted in the sciences is not well known. The aim of this study is to establish an evidential baseline of teaching practices across a bachelor of science degree program at a large research-intensive Australian university. Our purpose is to contribute to knowledge on the adoption levels of evidence-based teaching practices by faculty within a science degree program and inform our science curriculum review in practical terms. We used the Teaching Practices Inventory (TPI) to measure the use of evidence-based teaching approaches in 129 courses (units of study) across 13 departments. We compared the results with those from a Canadian institution to identify areas in need of improvement at our institution. We applied a regression analysis to the data and found that the adoption of evidence-based teaching practices differs by discipline and is higher in first-year classes at our institution. The study demonstrates that the TPI can be used in different institutional contexts and provides data that can inform practice and policy.
INTRODUCTION
There is a large pool of evidence for the benefits of active-learning techniques (Freeman et al., 2014). This evidence, in conjunction with a strong call for change in the classroom (American Association for the Advancement of Science, 2009), highlights the need for instructors to drastically alter their teaching methods. However, there is also significant difficulty in getting teaching faculty to fully adopt and properly implement active-learning techniques (Gess-Newsome et al., 2003; Wilson, 2010). The evidence supporting active learning is not enough for instructors to implement it (Andrews and Lemons, 2015). Instead, there are a number of interrelated issues that contribute to the lack of active-learning implementation. These include instructors seeing teaching as conflicting with their “professional identity” (Brownell and Tanner, 2012) or as a “necessary evil” (Anderson et al., 2011) and a lack of support (Henderson et al., 2011, 2012). Furthermore, many instructors find themselves pressured to place a lower priority on teaching due to the lack of incentives when compared with research (Wilson, 2010).
There is a lack of alignment between education research and teaching practice (Dolan, 2015) that the issues described above only partly explain. Moving forward, Wieman (2015) argues strongly that a necessary condition for change is to have reliable and objective measures of teaching quality. This focus on teaching quality is essential given the evidence about effective teaching approaches (National Research Council, 2012). To this end, one of the main challenges in measuring the implementation of active-learning techniques is finding a suitable method to gauge such practices. Our knowledge of the adoption levels of these teaching approaches at the broad institutional level is limited (Wieman and Gilbert, 2014), but there is a growing body of literature that we now describe.
Some early studies of active-learning uptake involved directly interviewing teachers regarding their teaching (Trigwell et al., 1994). These approaches often used qualitative methods, focusing on the intentions and ideals of the teachers rather than actual practice. A popular approach employed in 97% of departments (Berk, 2005) in gauging teaching quality is student course evaluations, but many concerns have been raised about their reliability. The major concern is that the evaluations are biased by factors unrelated to the teaching, such as class size, difficulty or workload, interest in the subject, and personality of the teacher (Wachtel, 1998; Marsh, 2007). Both Wachtel and Marsh conclude that student evaluations are generally reliable, but some studies find a clear bias in terms of personality (e.g., Shevlin et al., 2000). It is for these reasons, among others explained by Wieman (2015), that student course evaluations may not be an appropriate tool for the objective measure of active-learning implementation.
The most direct approach is for independent observers to attend classes and record the use of different active-learning methods by instructors. Several different protocols have been proposed for this purpose. The Reformed Teaching Observation Protocol (RTOP; Sawada et al., 2002) measures 25 qualities of the instruction with a five-point Likert scale: there was a strong correlation between the RTOP score and learning gains for the classes where pre/post testing was available. The RTOP was also used by Ebert-May et al. (2011). The Classroom Observation Protocol for Undergraduate STEM (COPUS; Smith et al., 2013) takes a quantitative approach, measuring how much time instructors (and optionally students) spend on different categories of activity. Analysis of the COPUS results for 50 science, technology, engineering, and mathematics (STEM) courses showed that there was a wide, continuous range of teaching practices that did not cluster into traditional and active approaches (Smith et al., 2014). Lund et al. (2015) used a clustering analysis combining both RTOP and COPUS data for 73 instructors and identified 10 broad teaching styles (ranging from lecturing through to group work). Hora and Ferrare (2013) combined interviews with observations (using the Teaching Dimensions Observation Protocol) in a study of 57 instructors, revealing qualitative differences in teaching methods between different disciplines. The physics, chemistry, and biology instructors in their study used a wider range of teaching approaches in class than the mathematics and geology instructors.
An alternative approach is to ask instructors to self-report on their teaching practices. This approach has the advantage that all aspects of a course can be measured and there is no need to fund and train observers. The Higher Education Research Institute Faculty Survey (Eagan et al., 2014) has collected a very wide range of data—including teaching practices—since 1989. It demonstrates a slow decrease in lecture-dominated teaching in favor of more active approaches. Marbach-Ad et al. (2014) asked teaching assistants and faculty members how often they implement active-learning techniques in the classroom: there was still a high level of “extensive lecturing” reported. While this approach focused more directly on teaching, many of the questions had qualitative-response options (such as “most class sessions”). Teaching practice surveys have also been applied to specific disciplines: geoscience (Macdonald et al., 2005), engineering (Borrego et al., 2010), and physics (Henderson et al., 2012).
The Teaching Practices Inventory (TPI; Wieman and Gilbert, 2014) is a new survey instrument specifically designed for the rapid measurement of research-based teaching practices. It attempts to be more objective than some previous work by asking yes/no questions and requiring definite number ranges for activities. Most importantly, it uses a scoring rubric that weights activities according to the research evidence linking them to learning gains. For instance, the use of a personal response system (e.g., “clickers”) contributes to the score only if it is “followed by student–student discussion.” The TPI also offers a more extensive range of questions than some previous surveys. There has been some discussion about the accuracy of self-reporting approaches such as the TPI, but Smith et al. (2014) compared TPI results with independent COPUS observations and found that instructors were reporting reliably on their approaches.
PURPOSE
Our broad aim is twofold, focused on both research and practice. First, we want to contribute new knowledge by using the TPI to measure the use of evidence-based teaching in the bachelor of science (BSc) program at a large research-intensive university. Our work extends the Wieman and Gilbert (2014) study to a new context. Such a study would begin to address the limited literature on the uptake of evidence-based teaching practices at the discipline and degree-program levels, which is needed in the sciences as we shift from individual practices to whole-program approaches to enhance the student learning experience (Fairweather, 2008). Second, we seek to capture quantitative data on teaching practices within our institution to inform a BSc curriculum review (McManus and Matthews, 2015). These data will allow us to establish an evidential baseline and prioritize areas offering the highest return on our resourcing of teaching development (see Knight, 2001; Wieman et al., 2010; Mercer-Mapstone and Matthews, 2017).
We address the following questions in this paper:
To what extent are evidence-based teaching approaches used in the BSc courses at our institution?
How do these compare with the corresponding data from a Canadian university?
How does the use of evidence-based teaching approaches differ, within our institution, by class size, year level, and discipline?
Throughout this paper we use the term “course” to refer to a single unit of study or subject.
METHODS
Context
The study was situated within an Australian research-intensive university ranked in the top 100 universities worldwide (in the Times Higher Education World University Rankings and the Quacquarelli Symonds World University Rankings, among others). The university is a large public university with a student body just under 49,000 (74% undergraduates, 25% postgraduate course work, and 1% research higher-degree students). It has a research budget of AU$380 million per annum. The faculty of science is one of six faculties within the university and includes eight multidisciplinary departments. The BSc degree program is administered by the faculty of science with all eight departments (plus five departments from other faculties) teaching courses in the degree program.
The BSc degree program comprises 3 years of undergraduate study with an optional fourth year for honors, and consistently attracts applicants straight from high school. The BSc is marketed as a flexible, generalist degree that prepares students for a range of postgraduate opportunities. Students have a wide range of elective choices, although they must complete a first-year statistics course and meet requirements of a major in a specific discipline to graduate. BSc courses typically involve 3 hours of lectures in a week plus a 2- or 3-hour laboratory (either every week or biweekly) or tutorial sessions (more common in mathematics/statistics).
Survey Instrument
We used the TPI (Wieman and Gilbert, 2014) to measure the use of evidence-based teaching approaches in our institution. The TPI is a 72-item questionnaire that asks an instructor specific questions about the use of selected evidence-based practices within a designated course. Typical questions are “Students asked to read/view material on upcoming class session” (yes/no) or (what is the) “Average number of times per class: show demonstrations, simulations, or video clips.” The survey groups the questions into eight different categories of teaching activity (see Table 1, which also gives example questions) and gives a score in each category as well as the total score for a course. The scores are calculated by weighting the activities according to their learning impact (see Wieman and Gilbert, 2014).
Category | Maximum score | Sample question |
---|---|---|
I. Course information | 6 | List of topic-specific competencies |
II. Supporting information | 7 | Lecture notes or course PowerPoint presentations |
III. In-class activities | 15 | Reflective activity at end of class, e.g., “one-minute paper” or similar |
IV. Assignments | 6 | Encouragement and facilitation for students to work collaboratively on their assignments |
V. Feedback | 13 | Students see midsemester exam(s) answer key(s) |
VI. Diagnostics | 10 | Use of pre–post survey of student interest and/or perceptions about the subject |
VII. Tutor training | 4 | Tutors receive one-half day or more of training in teaching |
VIII. Collaboration | 6 | Sit in on colleague’s lectures (any lecture) to get/share ideas for teaching |
We made minor modifications to the TPI to clarify certain terms for use in our local context, then tested the survey twice. First, staff from the teaching and learning institute of our university confirmed the language was appropriate for an Australian context. We then tested the survey on members of our science teaching and learning committee as a key stakeholder group. They gave useful advice about the survey implementation, notably to offer prizes to incentivize participation (10 gift vouchers were offered). They also suggested we clarify the type of teaching involved (lectures, not laboratory classes) with the explanatory statement we added to the preamble, to keep in line with the intended scope of the TPI (Wieman and Gilbert, 2014). The list of all the changes we made is given in Table 2. We present our modified survey in the Supplemental Material for this paper.
Section | Original text | Modifed text |
---|---|---|
Preamble | Added: “This inventory is specifically focused on the ‘lecture’ contact of your course, which can include a range of activities. For example, lecturing, ‘lectorial’, workshops, discussions, student led activities, problem solving, and the ‘flipped classroom’ model. This survey is not asking about tutorials or practical class contact.” | |
Preamble | Current term | Current semester |
Preamble | Course number | Course code |
Preamble | Section number(s) or instructor name | Course coordinator name |
II.i,ii | Contribution from you | Contribution from you, tutors, or other academics |
III.A,B | Class | Lecture |
III.B.i | Clickers | Removed so as to include other electronic devices in current use |
V.A | To instructor | To academics |
VII | Teaching assistant (TA) | Tutor |
VII | Instructor | Course coordinator |
Participants
We asked the staff member coordinating each course to complete the TPI, following the practice of Wieman and Gilbert (2014). We selected courses satisfying all the following criteria:
Courses contributing to BSc degree in semester 1, 2015.
Courses with more than 15 BSc students enrolled. This cutoff is arbitrary, but removes very small courses while including the core third-year courses.
Courses that are delivered in class rooms (i.e., excluding fieldwork, laboratory-based, and online courses).
There were 178 courses contributing to the BSc, with 136 remaining after the other criteria were applied, drawn from 13 departments (multidisciplinary organizational units; see Table 3). These courses had student enrollments ranging from 15 (the minimum criterion) to 1425. Ethics approval was given by the local ethics committee (approval number 2015000345).
Department | Courses | Completion rate (%) | Mean (SD)a |
---|---|---|---|
1 | 18 | 83 | 32.5 (8.9) |
2 | 12 | 100 | 34.3 (6.4) |
3 | 11 | 91 | 34.5 (8.1) |
4 | 18 | 100 | 26.6 (5.9) |
5 | 10 | 90 | 30.2 (4.8) |
6 | 13 | 92 | 27.5 (8.0) |
7 | 29 | 97 | 30.3 (7.9) |
8 | 17 | 100 | 30.2 (5.9) |
9–13b | 8 | 100 | 26.9 (7.5) |
Total | 136 | 95 | 30.3 (7.5) |
Data Collection
We opened the survey at the start of week 5 of the 13-week semester 1 teaching period in 2015 via an email inviting instructors coordinating courses in the BSc to complete the online survey. An incentive was offered (a drawing to win a $100 gift voucher) and two reminder emails were sent to encourage participation in the study. We closed the survey at the start of week 9 of the semester, at which point we had 129 responses, giving an overall completion rate of 95%. The average reported completion time was 11.4 minutes and only one instructor raised concerns about the time taken. We calculated TPI scores using the spreadsheet provided by Wieman and Gilbert (2014) and present the individual scores for all courses in Supplemental Table S1.
Analysis Methods
To explore our first research question—the use evidence-based practice at our institution—we calculated descriptive statistics (e.g., frequencies, means, standard deviations) of the results for our institution, including overall scores (Table 3 and Figure 1), the adoption rates of selected practices (Table 4), and scores by category (Table 5, Supplemental Table S2, and Figure 2).
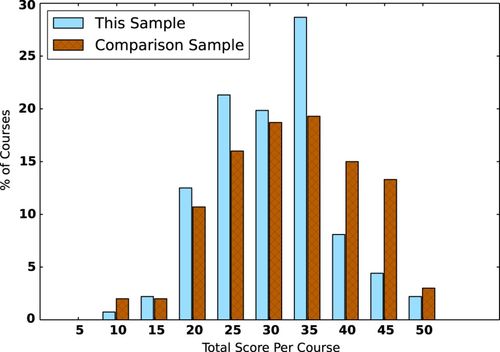
FIGURE 1. Comparison of total TPI scores for our institution (129 courses) and the comparison sample (93 courses; Wieman and Gilbert, 2014). The graph shows the percentage of courses falling into the score ranges displayed on the horizontal axis. Note that the maximum score is 67 but no courses scored above 50.
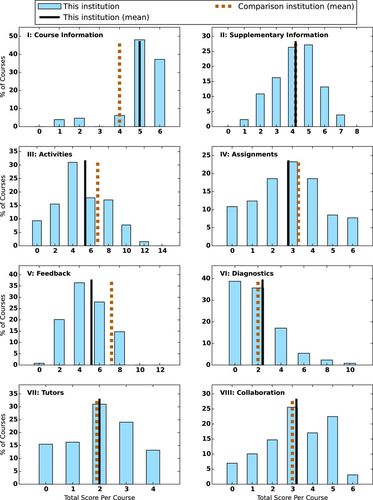
FIGURE 2. For each of the eight teaching categories, we compare the TPI scores for our institution (distributions and means) with the mean scores of the comparison institution (Wieman and Gilbert, 2014). The horizontal axis for each category shows the maximum score possible in that category.
Category | Robust practice | Percent |
---|---|---|
I. Course information | List of topic-specific competencies | 91 |
II. Supporting Information | No practices were scored as robust | — |
III. In-class activities | Average number of times per class: have small-group discussions or problem solving >1 | 29 |
Average number of discussions per term on why material useful and/or interesting from students’ perspective >5 | 33 | |
Students read/view material on upcoming class session and complete assignments or quizzes on it… | 32 | |
Fraction of typical class period you spend lecturing <60% | 18 | |
Questions posed followed by student–student discussion | 22 | |
At least one category III robust item | 74 | |
IV. Assignments | Problem sets/homework assigned and contributed to course grade at intervals of 2 weeks or fewer | 45 |
Encouragement and facilitation for students to work collaboratively on their assignments | 49 | |
At least one category IV robust item | 74 | |
V. Feedback | Assignments with feedback before grading | 20 |
Number of midterm exams >1 | 13 | |
At least one category V robust item | 32 | |
VI. Diagnostics | Use of instructor-independent pre/posttest (e.g., concept inventory) to measure learning | 8 |
Use of pre/posttest that is repeated in multiple offerings of the course to measure and compare learning | 21 | |
New teaching methods or materials were tried along with measurements to determine impact on student learning | 33 | |
At least one category VI robust item | 45 | |
VII. Tutor training | There are instructor–TA meetings every 2 weeks or more frequently in which student learning and difficulties and the teaching of upcoming material are discussed | 46 |
VIII. Collaboration | Read literature about teaching and learning relevant to this course (>2 on scale of never to very frequently) | 56 |
Sat in on colleague’s class (any class) to get/share ideas for teaching (>2 on scale of never to very frequently) | 28 | |
At least one category VIII robust item | 62 |
Category | N | Total | I. Course information | II. Supporting information | III. In-class activities | IV. Assignments | V. Feedback | VI. Diagnostics | VII. Tutor Training | VIII. Collaboration |
---|---|---|---|---|---|---|---|---|---|---|
This institution: mean (SD) | 129 | 30.3 (7.5) | 5.0 (1.2) | 4.2 (1.4) | 5.4 (2.8) | 2.8 (1.7) | 5.2 (1.9) | 2.4 (2.1) | 2.0 (1.2) | 3.2 (1.6) |
Comparison institution: mean (SD) | 93 | 32.4 (8.9) | 4.0 (1.7) | 4.2 (1.5) | 6.8 (3.2) | 3.3 (1.6) | 7.1 (2.1) | 2.0 (2.0) | 1.9 (1.4) | 3.0 (1.7) |
p Value | 0.066 | <0.001 | 1.000 | 0.001 | 0.026 | <0.001 | 0.151 | 0.578 | 0.377 | |
Adjusted p value | 0.119 | <0.001** | 1.000 | 0.003** | 0.059 | <0.001** | 0.227 | 0.651 | 0.484 |
We compared our institution with the Wieman and Gilbert (2014) data from a Canadian institution—our second research question—by testing for different mean scores in the TPI categories. This involved multiple t tests, which increases the probability of false detections based on the individual p values. We controlled for the false discovery rate over multiple tests by applying the Benjamini and Hochberg (1995) correction to the p values (using the p.adjust function in the R programming language). We considered two results to be significantly different in a set of tests when the adjusted p value was p´ < 0.05. The Benjamini and Hochberg (1995) correction means that the probability of making a false detection with this condition across a set of tests is less than 0.05. We use these adjusted p values in all of our analyses given here.
Wieman and Gilbert (2014) noted a possible bias in their data if the responding instructors were not representative of their whole departments. We minimized any bias by limiting any comparisons to the three departments in the Wieman and Gilbert data with completion rates greater than 70% (n = 93 courses). Such bias in our data is likely to be small given our high overall completion rate: all our departments were more than 80% complete (Table 3).
We used a multiple linear regression analysis to address our third research question: how the TPI scores within our institution vary by class size, year level, and discipline. The advantage of a regression approach is that it determines the relative contributions of the different parameters simultaneously (see Theobald and Freeman, 2014).
We defined discipline by classifying all the courses into the following four broad, cognate discipline clusters (a common classification of disciplines for government reporting in Australia):
Biological (biology and life sciences)
Physical (chemistry, physics, and earth sciences)
Mathematical (mathematics, statistics, and computer science)
Psychology
Our research question defines three predictor variables: discipline and year level (both categorical variables) and class size (a continuous variable). We report the TPI results for subsamples split by these variables in Supplemental Table S2. We tested the independence of these variables using a chi-square test between the categorical variables (discipline and year level) and an analysis of variance test between each of the categorical variables and the continuous class size variable. We used the chisq.test and aov functions in the R programming language, respectively, for these tests and present the results in Supplemental Table S3. There is evidence of correlations between all the variables. There is one extremely significant correlation (adjusted p value < 10−9) between class size and year level. We therefore discounted class size as an independent variable, leaving discipline and year level as the two predictor variables for our analysis.
The possible outcome variables are the eight individual TPI category scores, but as we discuss below, our institution has a mandatory policy that ensures a high score in the first TPI category, so we discount this category from our model. Our model for the linear regression is therefore seven relations that predict the TPI scores for the categories course information (II) through to collaboration (VIII). Each relation has the form
Category | II. Supporting information | III. In-class activities | IV. Assignments | V. Feedback | VI. Other (Diagnostics) | VII. Tutor Training | VIII. Collaboration |
---|---|---|---|---|---|---|---|
Coefficient | β ± SE (p´) | β ± SE (p´) | β ± SE (p´) | β ± SE (p´) | β ± SE (p´) | β ± SE (p´) | β ± SE (p´) |
Intercept β0i | 3.52 ± 0.26 | 4.75 ± 0.52 | 2.90 ± 0.31 | 4.52 ± 0.35 | 1.85 ± 0.37 | 1.70 ± 0.23 | 2.29 ± 0.29 |
Biological β1i | 0.52 ± 0.30 (0.214) | 1.06 ± 0.62 (0.214) | 0.63 ± 0.37 (0.214) | 0.31 ± 0.41 (0.639) | 0.95 ± 0.45 (0.171) | 0.40 ± 0.27 (0.271) | 0.94 ± 0.34 (0.047)** |
Mathematical β2i | 0.47 ± 0.37 (0.361) | −0.25 ± 0.77 (0.785) | 0.14 ± 0.45 (0.785) | 0.95 ± 0.51 (0.214) | −0.70 ± 0.55 (0.361) | 0.07 ± 0.33 (0.828) | 0.67 ± 0.42 (0.230) |
Psychology β3i | 0.74 ± 0.39 (0.214) | −0.42 ± 0.80 (0.707) | −0.95 ± 0.47 (0.212) | 0.36 ± 0.53 (0.668) | 0.56 ± 0.58 (0.489) | 1.10 ± 0.35 (0.035)** | 0.76 ± 0.44 (0.214) |
First-year β4i | 0.86 ± 0.33 (0.059) | 1.16 ± 0.67 (0.214) | −0.18 ± 0.40 (0.743) | 1.26 ± 0.45 (0.047)** | 1.33 ± 0.48 (0.047)** | 0.17 ± 0.29 (0.696) | 1.28 ± 0.37 (0.026)** |
Second-year β5i | 0.29 ± 0.30 (0.489) | 0.99 ± 0.61 (0.230) | −0.38 ± 0.36 (0.465) | 0.45 ± 0.41 (0.445) | 0.16 ± 0.44 (0.785) | −0.16 ± 0.27 (0.696) | 0.17 ± 0.34 (0.707) |
RESULTS
Extent of Use of Evidence-Based Teaching Practices in BSc at a Research-Intensive Australian University
We show the extent of uptake of the most robust evidence-based teaching practices at our institution in Table 4. We define robust as the “practices for which there is evidence suggesting they provide particularly large and robust benefits” (Wieman and Gilbert, 2014, p. 556). These practices are indicated by a weight of 2 or more in the TPI scoring rubric. We did not include category II (supporting information), as all the activities in this category had weights less than 2. We also give the percentage of courses that used at least one of the robust activities in each category. The most notable observations are as follows:
Category I (course information): The adoption of a “list of topic-specific competencies” was very high (91%). This was the only category in which the Australian institution scored significantly higher than the Canadian institution.
Category III (in-class activities): 74% of courses used at least one robust practice in this category, but many were not using these practices effectively. For example, 82% used demonstrations, simulations, or video, but only 14% used the better approach, in which “students first record predicted behavior and then afterwards explicitly compare observations with predictions.” The data revealed several such examples of staff adopting practices but not including the scaffolding that made the practices most effective.
Category V (feedback): This category had the lowest adoption of high-impact activities (32%). This is consistent with the low overall low scores for the category at the Australian institution.
Category VI (other, diagnostics): This category was notable for the very low (8%) use of independent pre–post tests. This may be due to a perceived lack of such instruments, since many are available, as reviewed by Libarkin (2008).
Category VII (tutors): The only robust practice (regular meetings with tutors) was adopted by less than half the courses. This result is inconsistent with the tutor training at our institution, which implies staff will meet regularly with tutors.
Category VIII (collaboration): A considerable number of staff (56%) consult the literature about their teaching, indicating many instructors adopt a scientific approach to teaching (Handelsman et al., 2004). However, only 28% were actively visiting a colleague’s classes.
Comparison of Results across Two Research-Intensive Universities
We compare the total TPI scores for our courses with the Wieman and Gilbert (2014) sample in Figure 1. We used the mean total scores and standard deviations published by Wieman and Gilbert (2014) and only used their departments with completion rates greater than 70%. Our mean score of 30.3 (SD = 7.5)1 is lower than that of the comparison data, with a mean of 32.4 (SD = 8.9). This difference in mean scores is not statistically significant (see Table 5), even though the distribution in Figure 1 shows that our institution has fewer high-scoring courses than the comparison institution.
We compare the scores in each category for our institution with the comparison sample in Figure 2. The scores in most categories have broad distributions at our institution, but Figure 2 shows that the course information category (I) is skewed to high values and the diagnostics category (VI) is skewed to low values. We compare the numerical values with the comparison data in Table 5. The mean scores demonstrated a statistically significant difference in three categories. Our institution scored higher than the comparison institution in the course information category (I) and lower for the in-class activities (III) and feedback (V) categories (see Table 5).
Variation within an Australian Institution by Year Level, Class Size, and Discipline
We split the data from our institution into separate subsamples based on year level, class size, and discipline. We present the mean TPI results for these different subsamples in Supplemental Table S2.
As discussed earlier, we used a multiple regression model to separate the effects of the different predictor variables on the TPI scores after removing class size from the model, as it is extremely highly correlated with year level. We present the results of the regression model in Table 6. Our null hypothesis is that none of the predictor variables (discipline and year level) contribute to any of the TPI category scores II–VIII (supporting information through to collaboration). Table 6 shows that five of the regression coefficients have values significantly different from zero (based on the adjusted p values), so our null hypothesis is rejected.
The predictor variables with significant (positive) contributions according to the model are as follows. First, courses in the biological discipline have higher scores for collaboration. This includes activities such as attending lectures by colleagues. Second, psychology courses have a higher score for tutor (teaching assistant) training (e.g., tutors have at least half a day of training). Third, independent of discipline, first-year courses have higher scores for feedback (V), diagnostics (VII), and collaboration (VIII). Feedback activities include the release of midsemester exam answers and diagnostic activities include the use of pre and post student surveys.
DISCUSSION
Our aim was to contribute to both research and practice on evidence-based teaching practices in the sciences by adopting the TPI for use in our local context. Having measured practice across our whole degree program, we can now determine which institutional policies and practices have contributed positively to the TPI scores at our institution. We have also used the data to identify priorities for future development.
Central Policies That Contribute to Stronger TPI Results
We have identified three central policies at our institution that have contributed to improved TPI results across our BSc program.
The courses at our institution had consistently high TPI scores for category I (course information), the only category that was significantly higher than the comparison institution (Table 5). More than 90% of our courses provide a “list of topic-specific competencies,” so students are given a clear sense of the course aims, objectives, learning activities, and assessments. The importance of this information is grounded in educational literature on constructive alignment and student-centered learning (Biggs, 1996). We can explain the high result in this TPI category by a strong central policy at our institution. The policy requires that all courses have an electronic “course profile” document that is publicly accessible and updated each semester. The policy is strongly supported by an institutional system that captures examination details from these profiles such that examinations will not be scheduled for a course unless its profile has been published. Staff create the profiles by completing a fixed-format electronic form that requires the inclusion of aims and objectives. Implemented in 2006, the system is a common source of complaints among academics but is used across all courses, demanded by students, and now accepted by staff.
The regression analysis of our results (Table 6) revealed that our first-year courses have significantly higher TPI scores for feedback, diagnostics, and collaboration. This is contrary to our expectation that teaching practices become more personalized and aligned with evidence-based approaches in smaller, upper-level courses. The first-year courses in our institution have large enrollments and are typically characterized by hundreds of students sitting in a lecture theater with one or two instructors. Given the high levels of students leaving science within the first year, tremendous energy and resources have been devoted to effective teaching practices in our first-year courses. Following the previous large-scale review of the BSc program (Foster et al., 2008), the faculty of science made first-year course development the top priority for internal teaching and learning grants. There was also an institution-wide focus on “first-year experience” projects. The TPI data suggest that these central policies have had a measurable effect on improving our first-year courses.
The institution being studied also has a central policy on tutor training. The faculty of science runs 5 hours of training on topics such as the expectations of being a tutor, strategies for tutoring effectively, and how to provide assessment. This training is mandatory for all new tutors. Many departments (multidisciplinary organizational units) also require tutors to attend further training sessions specific to their classes (e.g., laboratory training). This method of having individual departments manage further tutor training is a common theme among Australian universities (Chan et al., 2007; Mocerino et al., 2009). This policy should have contributed to stronger scores for category VII (tutor training), but 55% of respondents responded “no” when asked if tutors “receive ½ day or more of training in teaching.” This result from the TPI survey revealed a communication problem at our institution, since effective policies and practice for tutor training are in place and have been for 5 years.
Contribution of Discipline Practice to Stronger TPI Results
Given the body of research highlighting the role of discipline on teaching (Becher and Trowler, 2001; Mårtensson et al., 2012) and the broad nature of our BSc, which includes majors in psychology, mathematics, and biosciences, we expected to find disciplinary differences. We have identified local practices that are associated with higher TPI scores for individual disciplines.
The regression analysis (Table 6) showed that courses in the biology and life sciences discipline scored higher for collaboration (category VIII), even after controlling for year level. Courses in this discipline are taught by two departments at our institution. While neither department has a specific policy regarding collaboration, both departments have regular staff meetings (one to two times per semester) to discuss assessment and curriculum. There may also be a greater need for collaboration in this discipline, because a small number of large first-year biology courses provide foundation material for a large number of different specialty areas across the life sciences. The staff teaching the higher-level courses need to coordinate with those teaching the foundation courses to ensure the appropriate prerequisite material is taught.
The regression analysis (Table 6) also showed that, after controlling for other variables, courses in the psychology discipline had higher scores for the tutor-training category. This follows from a long tradition of supporting and training tutors (teaching assistants) in this discipline at our institution. The tutor-training program used by the institution was originally developed in this discipline.
Future Policy and Practice Resulting from TPI Data
We can also identify areas to prioritize for future development from our TPI results, especially in comparison with other institutions.
Wieman and Gilbert (2014) piloted the TPI at a university known for its effort in enhancing science teaching and learning. Both their institution and ours are large, public, research-intensive universities. While changing science teaching practices is notoriously challenging in research-intensive universities (Wieman et al., 2010), the comparative nature of this study, drawing on the TPI, indicates that improvement is possible. Furthermore, comparing results helps to make sense of the findings and provides evidence to have focused conversations among instructors, which Cooper et al. (2015) proposed as the key to transforming tertiary science education.
The comparison between institutions (Table 5) shows that scores at our institution were significantly lower than the comparison institution in the categories of in-class activity, assignments, and feedback. Our institution has adopted these as priority areas for our BSc program, by setting “high impact learning activities such as assessment and feedback” as the top priority for internal teaching and learning grants.
Our central policy has had a strong effect in some areas (see Central Policies That Contribute to Stronger TPI Results above), but it may be limited in its further effect on personal teaching practice given the importance of the local, department-level culture. It is well established that academics are most strongly influenced in their teaching practices by their immediate peers (Becher and Trowler, 2001; Handelsman et al., 2004; da Silva et al., 2009; Mårtensson et al., 2012). This is consistent with the department-specific practices we have identified at our institution. The TPI results also reveal exemplary courses already using a range of active-learning activities that could be shared within and across departments. Focusing professional development at the department and discipline levels, drawing on informal social learning and evidence of effective practice, is known to be effective in changing teaching practices (Handelsman et al., 2004; Mårtensson et al., 2012). A local approach can also help address the tendency of science academics not to engage with generic teaching development (Matthews et al., 2014). As a result, our faculty of science has funded locally based teams to upgrade a few courses in each department: this funding is specifically tied to the adoption of high-impact practices listed in the TPI (see Table 4).
While strong local peer networks drive teaching practice, there is a danger they can become isolated, limiting opportunities to introduce new ideas, innovation, or change (e.g., Roxå and Mårtensson, 2009). Matthews et al. (2015) argue that it is therefore vital to have links beyond the immediate local networks to spread innovation. For this reason, it will not be sufficient to work within the existing departmental networks, but it will be necessary to expose them to external connections. Matthews et al. (2015) discuss the role of academic developers who can join local networks to act as “weak ties” to bring in ideas from other networks. This connection is something that the central faculty body could support at a policy level, while still effecting change in the local environment.
Limitations of the TPI Data
Several possible limitations should be considered when interpreting our study. First, there might be some bias in how instructors self-report on their courses. Wieman and Gilbert (2014) conducted several tests of the TPI measurements. They did not find any significant differences when the data about the course structure were independently measured. They also tested the in-class activity data by direct observation in a number of courses using the COPUS (Smith et al., 2013) and found no systematic difference in the results. Smith et al. (2014) conducted a more extensive comparison of TPI and COPUS results in 51 courses and also found they were consistent. Second, two instructors contacted us (after receiving a summary of results for their course) to report errors they had made when completing the TPI. As busy instructors rushed to complete the TPI, some misread questions and thus entered the wrong responses. Such errors in survey-type instruments are to be expected. Third, some terminology in the TPI initially confused instructors, particularly those not active in scholarly teaching approaches. We addressed this by piloting the instrument with colleagues to identify such terminology. It is particularly important to test the terminology when using the TPI in a different cultural/country context.
Conclusion and Recommendations
The complexities of changing teaching practices in undergraduate STEM courses are well articulated by Henderson et al. (2011). They remind us that change has to align with the institutional and disciplinary norms and personal beliefs. The TPI was developed with an appreciation for the belief systems of scientists and provides data on teaching practices at the individual, departmental, and degree-program levels. As a research tool, it offers much-needed insight into how science courses are being taught. Furthermore, it provides data that can be used to inform and guide undergraduate curricular reviews in the sciences focused on “how teaching happens.” The data are actionable. If we shift our view from teaching to learning, from academic to student (Barr and Tagg, 1995), then future research and practice has to view science curricula from the perspective of students who experience many courses over several years. As a result of this study, we make the following recommendations to engage instructors across multiple departments in a more integrative approach to promoting effective teaching practice:
Focus the “unit of analysis” on a whole degree program, extending beyond departments, to characterize how students are being taught at the program level.
Before administering the TPI, consult with faculty on the purpose of collecting such data.
Analyze the TPI data to measure the effect of central policy and local department practice, as well as the use of teaching practices in individual courses.
When planning staff development based on the TPI results, consider both central and local policy approaches that allow for the way staff form their beliefs about teaching practices.
Instruments like the TPI that can measure teaching practices across a whole degree program, as opposed to individual teaching champions, are what is needed to move undergraduate science education forward into the 21st century. This study has demonstrated that the TPI can be used in different institutional contexts and that it provides data that can inform both policy and practice.
FOOTNOTES
1 We quote standard deviations of all values unless stated otherwise.
ACKNOWLEDGMENTS
We thank Carl Wieman and Sarah Gilbert for helpful advice about this study and for providing additional TPI data. We thank our colleagues in our institution’s central teaching and learning unit and our science teaching and learning committee for piloting the instrument and offering helpful feedback. We thank Gwen Lawrie (University of Queensland) for helpful comments on the draft paper. We particularly thank our anonymous referees and the editor for very insightful suggestions that have greatly enhanced this article. Finally, we are very grateful to those instructors who completed the TPI and engaged in workshops on the TPI results. This work was funded by a teaching fellowship to M.J.D. from the Institute for Teaching and Learning Innovation and the Faculty of Science, University of Queensland.