University Students’ Conceptual Knowledge of Randomness and Probability in the Contexts of Evolution and Mathematics
Abstract
Students of all ages face severe conceptual difficulties regarding key aspects of evolution—the central, unifying, and overarching theme in biology. Aspects strongly related to abstract “threshold” concepts like randomness and probability appear to pose particular difficulties. A further problem is the lack of an appropriate instrument for assessing students’ conceptual knowledge of randomness and probability in the context of evolution. To address this problem, we have developed two instruments, Randomness and Probability Test in the Context of Evolution (RaProEvo) and Randomness and Probability Test in the Context of Mathematics (RaProMath), that include both multiple-choice and free-response items. The instruments were administered to 140 university students in Germany, then the Rasch partial-credit model was applied to assess them. The results indicate that the instruments generate reliable and valid inferences about students’ conceptual knowledge of randomness and probability in the two contexts (which are separable competencies). Furthermore, RaProEvo detected significant differences in knowledge of randomness and probability, as well as evolutionary theory, between biology majors and preservice biology teachers.
INTRODUCTION
Evolution through natural selection is a central, unifying, and overarching theme in biology. Evolutionary theory is the integrative framework of modern biology and provides explanations for similarities among organisms, biological diversity, and many features and processes of our world. For example, the evolution of oxygenic photosynthesis massively affected geochemistry, and the evolution of organisms with calcareous shells led to the formation of limestone (e.g., Castanier et al., 1999; Kopp et al., 2005). Evolutionary theory is also applied in many other fields, both biological (e.g., agriculture and medicine) and nonbiological (e.g., economics and computer science). Therefore, the essential tenets of evolutionary theory have long been regarded as key parts of the foundations of science education (e.g., Bishop and Anderson, 1990; Beardsley, 2004; Nehm and Reilly, 2007; Pugh et al. 2010; Speth et al., 2014). Accordingly, the American Association for the Advancement of Science (AAAS, 2006), the Next Generation Science Standards (NGSS Lead States, 2013), the National Education Standards of Germany (Secretariat of the Standing Conference of the Ministers of Education and Cultural Affairs of the Länder in the Federal Republic of Germany [KMK], 2005a), and official documents of many other countries all describe evolution as an organizing principle for biological science and include the topic as a learning goal.
Although evolutionary processes may occur in many kinds of systems, unless specified otherwise, evolution generally refers to changes over time (also referred to as “between generations”) in populations or taxa of organisms due to the generation of variation and natural selection (Gregory, 2009). There is a massive empirical body of work on evolution, myriads of processes involved have been elucidated (e.g., genetic drift, genetic linkage, endosymbiosis, adaptive radiation, and speciation), and an extensive terminology has been developed (e.g., Rector et al., 2013; Reinagel and Speth, 2016). However, biologists generally agree that three principles are necessary and sufficient for explaining evolutionary change by means of natural selection: 1) the generation of variation, 2) heritability of variation, and 3) differential reproductive success of individuals with differing heritable traits (Endler, 1986; Gregory, 2009). This framework is deceptively simple, because myriad interactions are involved in phenomena such as adaptive radiation (the diversification of taxa leading to the filling of vacant ecological niches; Schluter, 2000). Furthermore, key processes such as speciation may occur gradually over long periods of time and many generations or in a single generation, if a massive chromosomal change or polyploidization is involved. Similarly, some important processes involve atomic-level phenomena, while others involve large-scale spatiotemporal variations in environmental variables and populations’ genetic structures. Moreover, natural selection acts on phenotypes (organisms’ observable traits), but adaptive changes are mediated by genetic changes that generally either enhance organisms’ reproductive success (thereby allowing the alleles they carry to spread in their respective populations) or enable colonization of new niches (Schluter, 2000). Hence, evolutionary change is far from simple, and it is still poorly understood by students throughout the educational hierarchy (Shtulman, 2006; Nehm and Reilly, 2007; Spindler and Doherty, 2009), science teachers (Osif, 1997; Nehm et al., 2009), and the general public (Evans et al., 2010). This poor understanding has been attributed to diverse cognitive, epistemological, religious, and emotional factors (for an overview, see Rosengren et al., 2012).
L. Tibell and U. Harms (unpublished data) concluded that complete understanding of evolutionary theory might require the understanding of more general abstract concepts like randomness, probability, and different scales in space and time. These general abstract concepts coincide with a set of recently proposed “threshold concepts” in genetics and evolution (Taylor, 2006; Ross et al., 2010). According to emerging theory initiated by Meyer and Land (2006), such concepts are portals that provide access to new ways of thinking; acquisition of understanding of these concepts is said to alter students’ perspectives and lead them to see things through a different lens. Threshold concepts are distinguished from “key” or “core” concepts, as they are more than mere building blocks toward understanding within a discipline and are tentatively proposed to have five characteristics: they are transformative (occasioning a shift in perception and practice), probably irreversible (unlikely to be forgotten or unlearned), integrative (emerging patterns and connections), often disciplinarily bounded, and troublesome (Meyer and Land, 2006). Threshold concepts in diverse disciplines have been examined, including economics (Davies and Mangan, 2007), chemistry (Park and Light, 2009), biology (Taylor and Cope, 2007), biochemistry (Loertscher et al., 2014), and computer science (Zander et al., 2008).
In the context of evolution, Tibell and Harms (unpublished data) developed a two-dimensional framework connecting principles and key concepts of evolutionary theory with the abovementioned general abstract concepts like randomness and probability. They propose that complete understanding of evolutionary theory requires the development of knowledge concerning not only the principles of evolution but also general abstract concepts like randomness and probability, and the ability to freely navigate through this two-dimensional framework.
Randomness, Stochasticity, and Probability
Random and probabilistic processes are key elements of evolutionary theory, and several studies report educational problems associated with the underlying abstract concepts (Ross et al., 2010; Robson and Burns, 2011). When considering random processes in evolution, students are reportedly challenged by both the terminology (Mead and Scott, 2010) and the conceptual complexity (Garvin-Doxas and Klymkowsky, 2008).
The term “random,” as used in everyday life and scientific contexts (e.g., mathematics and biology), is connected to various conceptions and interpretations. In everyday life, an event is often called random if it is very rare, strange, or unusual, and hence unpredictable or uncertain (Bennett, 1998). This common perception of randomness or “chance occurrences” does not change with increasing age (Falk and Konold, 1997; Kattmann, 2015), which hinders understanding of the concept of randomness (and the closely related concept stochasticity) in scientific disciplines, including mathematics (Kaplan et al., 2014) and biology (Mead and Scott, 2010). Random and stochastic are widely treated as synonymous terms, and definitions vary, but most mathematical texts and dictionaries note a distinction. Here, the term “random” is used when referring to phenomena (such as rolling dice) “where the outcome is probabilistic rather than deterministic in nature; that is, where there is uncertainty as to the result” (Smith, 2012, p. 1). In accordance with Oxford Dictionaries (www.oxforddictionaries.com), “stochastic” is used to describe processes for which outcomes have “a random probability distribution or pattern that may be analysed statistically but may not be predicted precisely.” It should be noted that random and stochastic can often be used in the same contexts, because a process may be random in the sense that it is influenced by random variables and stochastic in the sense that it has probabilistic outcomes. More formally, “a stochastic process is a family of random variables {Xθ}, indexed by a parameter θ, where θ belongs to some index set Θ” (Breuer, 2006, p. 1).
Randomness and stochasticity are fundamental elements of biological theories related to phenomena at all scales and levels, including the evolutionary gene-, individual-, population-, and environment-level processes involved in both the generation of variation and natural selection (Heams, 2014; Tibell and Harms, unpublished data). For example, the individual-level processes of mutation and recombination are regarded as random. Mutations may occur (at low frequencies) either in coding regions (thereby potentially affecting the structure and function of encoded proteins) or noncoding regions (thereby potentially affecting expression patterns). Hence, mutations of either kind may profoundly change organisms’ phenotypes. Clearly, the reactions involved must follow physicochemical laws, but they are regarded as random, because the individual-level outcomes are far beyond our ability to model predictively at this level (Heams, 2014), although we can determine population-level (stochastic) frequencies of mutations at given sites or sequences of DNA. Further, at population or environmental levels, random processes may involve, for example, the death of single organisms through causes that cannot be directly linked to selective (dis)advantages (Tibell and Harms, unpublished data) so even organisms close to an adaptive peak may die as juveniles. Thus, randomness and stochasticity are major elements of biological processes generally, and evolution specifically. However, a desire to ascribe causes to all events appears to be an intrinsic element of human nature (Falk, 1991), which may lead to a denial of chance in general and explain why students have difficulties perceiving evolutionary events as aimless random occurrences (Kattmann, 2015). Furthermore, students tend to perceive biological processes as efficient and random processes as inefficient (Garvin-Doxas and Klymkowsky, 2008).
To summarize, randomness and stochasticity (as defined here) are closely related, but randomness refers to processes or variables that are uncertain rather determinate, while stochasticity refers to probabilities of outcomes of processes in or affecting populations. Probability is the likelihood of a particular outcome and is assigned a numerical value between 0 and 1 (Feller, 1968). The closer a probability value is to 1, the more likely the outcome. Crucially, an outcome that is extremely rare at the individual level, such as a given beneficial mutation, is extremely likely to occur at least once in a population that is sufficiently large or over a sufficiently long time frame (in terms of number of generations). In the context of evolution, probability plays a role in all three of the principles mentioned earlier, but particularly selection and inheritance (Tibell and Harms, unpublished data). For example, fertilization in sexual reproduction involves probabilistic events like the choice of mate. The best-adapted individuals are most likely to survive to reproductive maturity, mate, and thus to reproduce. Hence, the frequencies of organisms with given traits in a given environment depend on many random events, and the process of selection can also be defined as the probabilities of individuals with differing traits in a given population surviving and reproducing in a specific environment. Although reproduction depends upon survival and many other different factors (as mentioned earlier), it is still the process of reproduction encompassing fitness that is evolutionarily relevant. However, it should also be remembered that selection acts on random processes involved in generation of variation (Mayr, 2001), but the importance of these processes seems to be a learning obstacle for students (Lynch, 2007; Garvin-Doxas and Klymkowsky, 2008).
Moreover, biology students not only struggle to grasp the importance and roles of randomness, probability, and stochasticity in evolutionary theory (Gregory, 2009), but also often have a weak understanding of mathematics (Jungck, 1997; Hester et al., 2014). This clearly hinders the teaching and learning of evolution, as mathematical descriptions of randomness and probability are key elements of the explanations of random and stochastic evolutionary (and other) biological processes (Wagner, 2012; Buiatti and Longo, 2013). To date, there is no empirical evidence about students’ conceptual structures regarding randomness and probability in biological contexts, and their connections (if any) to conceptual structures in mathematics contexts. However, some studies indicate that mathematical modeling can generally lead to improvements in problem solving and qualitative conceptual knowledge, that is, students’ ability to predict likely outcomes of processes (Chiel et al., 2010; Schuchardt and Schunn, 2016). Thus, there is a need to explore the possible connections between understanding of evolutionary theory and conceptual knowledge of randomness and probability in both evolutionary and mathematical contexts.
Development of Content-Related Knowledge in Higher Education
In Germany, higher education in biology is divided into two stages, generally consisting of a 3- to 4-year course leading to a bachelor’s degree followed by a 1- to 2-year course leading to a master’s degree (KMK, 2010). Bachelor’s courses are intended to equip students with a broad qualification by providing academic subject–specific foundations, methodological skills, and competences related to the professional field, while master’s courses provide further subject and academic specialization (KMK, 2010).
Higher education leading to a degree in teaching includes at least two subjects, and students can take—depending on the Land (federal state) or higher education institution—either a basic foundation course (concluding with the first state exam) or a graded course (with bachelor’s and master’s degrees) (KMK, 2010). In all programs, subject areas, subject didactics, and educational science components are coupled and supplemented with practical components in the form of school internships. The relative amounts of time allocated to subject areas and educational science depend on the Land and type of school in which the students aspire to teach. Typically, the contents of preservice biology teachers’ education in their chosen subjects (e.g., biology) account for 30–40% of the total for bachelor’s and basic foundation courses and 20–25% for master’s courses (Verband Deutscher Biologen und biowissenschaftlicher Fachgesellschaften e.V [VBIO], 2006).
At the beginning of bachelor’s programs, most universities offer a compulsory module on the topic of general biology. This module should enable students to gain sound knowledge about the structure and function of cells, acquire insights into the diversity and evolution of plants and animals, and learn the basic techniques of biological investigations. Students subsequently take various compulsory or elective modules, such as genetics, ecology, evolution, cell biology, and/or molecular biology, depending on the university and whether they are biology majors or preservice teachers (VBIO, 2006). Regarding evolution (or evolutionary theory), both sets of students are normally exposed to the topics of mechanisms of evolution, micro- and macroevolution, evolutionary theories, and abiotic and biotic factors (see Supplemental Table S1). Nevertheless, there is a substantial difference in development of biological knowledge between biology majors and preservice biology teachers. Although some seminars are attended by both, preservice biology teachers have fewer opportunities to learn the subject. Therefore, preservice biology teachers may tend to have less deep and detailed knowledge about specific biological processes. As evolution is described as an organizing principle for biological science and an explicitly stated learning goal in diverse standards (e.g., KMK, 2005a; AAAS, 2006; NGSS, 2013), both biology majors and preservice teacher students should ideally have a shared core of knowledge regarding evolutionary changes through natural selection. Further, this general knowledge is important, because evolutionary theory is the integrative framework of modern biology, and its essential tenets are key parts of the foundations of and for science education.
Research Objective
Diverse instruments have been developed for measuring evolutionary knowledge (e.g., Anderson et al., 2002; Nadelson and Southerland, 2009; Nehm et al., 2012; Price et al., 2014). However, we are not aware of any tool for measuring understanding of randomness and probability, although they play major roles in evolutionary processes (Tibell and Harms, unpublished data). Thus, a robust test instrument for measuring understanding of these two abstract concepts, and their roles in evolution, is required to advance evolution education research and assess both biological courses and students. In efforts to meet this need, we have developed an instrument called the Randomness and Probability Test in the Context of Evolution (RaProEvo) and a sister instrument called the Randomness and Probability Test in the Context of Mathematics (RaProMath) to explore the empirical structure of biology students’ conceptual knowledge of randomness and probability and the relationship of this knowledge to their conceptual knowledge of evolutionary theory. During development of these instruments, we applied previous findings on students’ common difficulties when trying to learn evolutionary concepts (e.g., Gregory, 2009; Mead and Scott, 2010). Here, we describe their development, provide indications of their validity measures (expert ratings and criterion-related validity measures), and present results of field tests of the instruments on biology majors and preservice biology teachers.
METHODS
Participants
During the 2015–2016 academic year, we recruited 140 biology students (26.4% male; 72 biology majors [30.6% male] and 68 preservice biology teachers [22.1% male]) enrolled at 23 German universities to complete an online survey. The participants’ average age was 22.9 years (SD = 3.7); 22.2 years (SD = 2.9) for biology majors and 23.7 years (SD = 4.3) for preservice biology teachers. On average, they had matriculated for 5.3 semesters (SD = 3.6) in tertiary education, with a mean of 4.7 semesters (SD = 3.9) for biology majors and 5.8 semesters (SD = 3.2) for preservice biology teachers. A total of 79 students (56.4% of all participants; 41 biology majors and 38 preservice biology teachers) had taken compulsory modules on evolution or evolutionary biology and had been introduced to the topic of evolution (e.g., mechanisms of evolution, micro- and macroevolution, evolutionary theories, and abiotic and biotic factors). Furthermore, 48 of these students (34.3% of all participants) had also taken compulsory modules in genetics, ecology, and cell or molecular biology, while 10 students (7.1% of all participants) had only taken the evolutionary module. Students were also asked to provide Likert-type responses ranging from 1 (not at all) to 4 (intensively) to the items regarding their learning opportunities in the contexts of evolution, genetics, and ecology. Their self-reported statements indicate that considerable attention was paid to evolution (M = 9.51, SD = 1.8) genetics (M = 8.43, SD = 2.68), and ecology (M = 8.67, SD = 2.27) during their higher education.
Procedure
Participants responded to a basic demographic questionnaire (including items probing their academic self-concept) and completed tests on conceptual knowledge of randomness and probability in both evolutionary and mathematical contexts. The structure of the online survey was the same for all participants and had no time limit. On average, the students took 58 minutes, 56 seconds (SD = 15 minutes, 14 seconds; range: 20 minutes, 4 seconds, to 94 minutes) to complete the survey. All respondents were given the opportunity to participate in a lottery for 10 vouchers, each worth 50 euros (approximately US$54 at the time of the survey).
Measures
Randomness and Probability Knowledge Test
Development.
The first step in developing or considering an instrument to measure students’ conceptual knowledge of randomness and probability in the context of evolution is to clarify the types of knowledge they should acquire during their education. To do so, we first designated two focal topics (contexts): evolution and mathematics. For the evolution context, we identified the following five aspects in which randomness and probability play important roles that biology graduates and teachers should understand: 1) origin of variation, 2) accidental death (single events, such as the death of one individual rather than another that is not linked to differences in adaptation to the environment; e.g., an individual could be struck by lightning, while less well-adapted individuals escape injury and produce more offspring), 3) random phenomena, 4) process of natural selection, and 5) probability of events. For the mathematics context, we selected the following five topics: 1) single events, 2) random phenomena, 3) probability as ratio, 4) sample reasoning, and 5) probability of events. To explore knowledge of these topics (explained in Table 1), we reviewed previously published instruments for testing evolutionary knowledge (e.g., Anderson et al., 2002; Bowling et al., 2008; Robson and Burns, 2011; Fenner, 2013) and knowledge of randomness and/or probability in various fields (e.g., Green, 1982; Falk and Konold, 1997; Garfield, 2003; Eichler and Vogel, 2012). Items deemed suitable were included in a pool of questions (N = 65 items; Table 2). Most items were translated from English into German, and almost all were modified more than once to fit the specific purpose of the instrument. Additionally, a number of questions were created by three researchers of the EvoVis project group (EvoVis: Challenging Threshold Concepts in Life Science—enhancing understanding of evolution by visualization). Distractors for these items were mainly based on students’ alternative conceptions reported in previous studies (e.g., Gregory, 2009). A coding scheme was provided for each item.
Topic | Learning objective: Students should be able to: | Question numbers |
---|---|---|
Evolution | ||
Origin of variation | Explain the causes of genetic variability (e.g., mutation, recombination), their impact on survival, and their importance for evolutionary processes. | E01, E02, E03, E07, E11, E12, E17 |
Accidental death (single event) | Recognize that the sudden death of an individual in a population is not per se due to natural selection and is therefore a random process. | E04, E09 |
Random phenomena | Identify and explain common processes in evolution that are considered random (e.g., mutations). | E05, E13, E14, E15 |
Process of natural selection | Determine that natural selection acts on phenotypes of populations with different organisms producing different numbers of offspring, which can result in specialization for particular ecological niches over time. | E06, E10, E16, E18 |
Probability of events | Apply mathematical modeling to biological processes and provide reasonable explanations. | E08, E19 |
Mathematics | ||
Single event | Determine the definitions of random processes as 1) unpredictability of single outcomes but 2) predictable in the long term, and provide reasonable explanations. | M02, M03, M06, M10, M14, M17, M25, M26, M29, M33 |
Random phenomena | Interpret results as outcomes of random phenomena. | M23, M27 |
Probability as ratio | Distinguish between equally likely and not equally likely experiments and thus predict the probability of simple experiments. | M01, M04, M08, M12, M15, M16, M18, M20, M21, M22, M28 |
Probability of events | Applying appropriate methods to predict the probability of multistage experiments (e.g., probability tree diagram or combinatorics). | M05, M07, M09, M11, M13, M24, M32 |
Sample reasoning | Explain how samples are linked to populations and what conclusions can be made from samples to populations. | M19, M30, M31 |
Context | Topic | Item number | Source of the idea/item (item code)a |
---|---|---|---|
Evolution | Origin of variation | E01 | Fenner, 2013 (item 24 pretest) |
E02 | Fenner, 2013 (item 26 pretest) | ||
E03 | Robson and Burns, 2011 (item 5 pretest) | ||
E07 | Campbell and Reece, 2011 (item 3, chap. 23) | ||
E11 | Author | ||
E12 | Bowling et al., 2008 (item 9) | ||
E15 | Author | ||
E17 | Campbell et al., 2006 (item 8) | ||
Accidental death (single event) | E04 | Author | |
E09 | Author | ||
Random phenomena | E05 | Campbell et al., 2006 (item 16) | |
E13 | Author | ||
E14 | Klymkowsky et al., 2010 (item 4) | ||
Process of natural selection | E06 | Author | |
E10 | Fenner, 2013 (item 20, pretest) | ||
E16 | Author | ||
E20 | Author | ||
Probability of events | E08a | Author | |
E08b | Author | ||
E19a | Green, 1982 (item 7) | ||
E19b | Author | ||
Mathematics | Single event | M02 | Green, 1982 (item 8) |
M03 | Green, 1982 (item 1) | ||
M06 | Green, 1982 (item 21a) | ||
M10 | Green, 1982 (item 21d) | ||
M14 | Jones et al., 1997 (item CP1) | ||
M17 | Author | ||
M25 | Eichler and Vogel, 2012 | ||
M26 | Author | ||
M29 | Author | ||
M33 | Green, 1982 (item 25) | ||
Random phenomena | M23 | Author | |
M27 | Falk and Konold, 1997 | ||
Probability as ratio | M01 | Garfield, 2003 (item 8) | |
M04 | Green, 1982 (item 3) | ||
M08 | Green, 1982 (item 2) | ||
M12 | Jones et al., 1997 (item CP2) | ||
M15 | Green, 1982 (item 17) | ||
M16 | Author | ||
M18 | Green, 1982 (item 6d) | ||
M20 | Herget et al., 2009 (item 1a, test part 3) | ||
M21 | Weber and Mathea, 2008 (item 5, test form 2) | ||
M22 | Jones et al., 1997 (item CP2) | ||
M28 | Herget et al., 2009 (item 1b, test part 3) | ||
Probability of events | M05 | Author | |
M07 | Garfield, 2003 (item 18) | ||
M09 | Green, 1982 (item 22) | ||
M11 | Garfield, 2003 (item 13) | ||
M13 | Garfield, 2003 (item 19) | ||
M24 | Garfield, 2003 (item 9) | ||
M32 | Weber and Mathea, 2008 (item 6, test form 1) | ||
Sample reasoning | M19 | Garfield, 2003 (item 14) | |
M30 | Green, 1982 (item 21d) | ||
M31 | Green, 1982 (item 23) |
Two preliminary versions of tests were developed to capture biology students’ conceptual knowledge of randomness and probability in the contexts of evolution and mathematics, designated RaProEvo and RaProMath, respectively. The RaProEvo test included a mixture of dichotomously scored (0 = no credit, 1 = full credit) and partial-credit (0 = no credit, 1 = partial credit, 2 = full credit) items, while items of the RaProMath test were all dichotomously scored (0 = no credit, 1 = full credit). To assess interrater reliability of the open-ended items, two raters (including D.F.) independently coded the responses using scoring rubrics. Cohen’s kappa interrater reliability statistics (Cohen, 1960) for these RaProEvo and RaProMath versions were 0.93 and 0.91, respectively. Discrepancies were resolved via deliberation between the raters. Items with a negative or low discrimination index (rit < 0.10) were excluded from further analysis (n = 3).
Faculty Review.
We examined content validity measures of the developed test instruments by soliciting faculty input to help validate the items. For this purpose, we administered an online version of RaProEvo to evolutionary biology faculty members (hereafter, biology experts) and an online version of RaProMath to faculty members with expertise in stochastics and/or probability (hereafter, mathematics experts) of different institutions. Biology experts were asked to select the correct response for each item, and were asked whether the item 1) tests the intended learning objective (Table 1) and 2) is scientifically accurate. A summary of their alignment is presented in Table 3. Experts could also add comments regarding each item and provide feedback. Mathematics experts were asked to follow the same procedure but to evaluate the mathematical accuracy of the items (Table 3). A total of 13 biology experts (10 faculty members and three PhD students) and 10 mathematics experts (eight faculty members and two PhD students) provided feedback on the instruments. In all cases, items with an agreement <80% had been flagged by the experts as potentially problematic and thus were deleted or critically revised. The experts’ suggestions on the intended learning objective were primarily to reword questions to increase precision and eliminate possible ambiguities. Altogether, two RaProEvo and six RaProMath items were finally deleted. At the end of this process, we were left with a 21-item RaProEvo test (16 multiple-choice, three free-response, and two matching items; see Supplemental Material 1) and a 33-item RaProMath test (30 multiple-choice and three free-response items; see Supplemental Material 2).
Items with given faculty agreement | |||
---|---|---|---|
>90% | >80% | <80% | |
RaProEvo | |||
The item tests the intended learning objective. | 18 | 4 | 1 |
The information given in the item is scientifically accurate. | 15 | 5 | 3 |
RaProMath | |||
The item tests the intended learning objective. | 32 | 0 | 7 |
The information given in the item is mathematically accurate. | 32 | 0 | 7 |
Test of Evolutionary Knowledge.
Students’ conceptual knowledge of evolutionary theory was assessed using the Open Response Instrument published by Nehm and Reilly (2007). This instrument was designed to determine how successfully biology majors can answer questions about natural selection at different levels of complexity and to identify both student knowledge and alternative conceptions. We used the following three, of five, items from this instrument:
Explain why some bacteria have evolved resistance to antibiotics (that is, the antibiotics no longer kill the bacteria).
Cheetahs (large African cats) can run faster than 60 miles (97 kilometers) per hour when chasing prey. How would a biologist explain how the ability to run fast evolved in cheetahs, assuming their ancestors could run at only 20 miles (32 kilometers) per hour?
Cave salamanders (amphibious animals) are blind (they have eyes that are not functional). How would a biologist explain how blind cave salamanders evolved from ancestors that could see?
To score students’ evolutionary explanations, we established and refined two scoring rubrics in a pilot study with a set of 39 biology students. The first scoring rubric—“key concepts”—covered eight key concepts: 1) origin of variation (e.g., mutation and recombination), 2) individual variation, 3) differential survival potential linked to specific traits, 4) inheritance of traits, 5) reproductive success, 6) selection pressure, including limitations of resources, 7) limited survival, and 8) changes in populations or distributions of individuals with certain traits (explained in Table 4). Two raters (including D.F.) independently coded their responses in these terms to compute interrater reliability, and Cohen’s kappa interrater reliability was found to be 0.76. In cases of disagreement, all coding discrepancies were resolved via deliberation. This scoring rubric was used to quantify the presence or absence of the eight key concepts in each of the students’ responses. The mean numbers of key concepts each student referred to in responses to all three items (hereafter, key concept score) and in responses to each of the three items (hereafter, key concept diversity [KCD]) were found to be 8.01 (SD = 4.89, range: 0–19, out of a maximum possible score of 24) and 4.61 (SD = 2.42, range: 0–8, out of a maximum possible score of 8), respectively. The second scoring rubric, “alternative conceptions concerning natural selection” (hereafter, alternative conceptions), was developed using seven common, well-known alternative conceptions that have been extensively documented in research literature (Bishop and Anderson, 1990; Gregory, 2009; Nehm and Reilly, 2007; Nehm et al., 2012): 1) need, 2) use and disuse, 3) anthropomorphism, 4) essentialism, 5) soft inheritance, 6) events versus processes, and 7) source versus sorting of variation (explained in Table 4). Two raters (including D.F.) independently coded their responses in these terms to compute interrater reliability, and Cohen’s kappa interrater reliability was found to be 0.73. In cases of disagreement, all coding discrepancies were resolved via deliberation. This scoring rubric was used to quantify the presence or absence of the seven common alternative conceptions in each of the students’ responses. The mean numbers of alternative concepts each student referred to in responses to all three items (hereafter, alternative concept score) and in responses to each of the three items (hereafter, alternative concept diversity [ACD]) were found to be 0.55 (SD = 0.71, range: 0–3, out of a maximum possible score of 21) and 0.35 (SD = 0.56, range: 0–3, out of a maximum possible score of 7), respectively.
Topic | The response refers to the following aspects: |
---|---|
Key concepts | |
Origin of variation | Changes are caused by mutation or recombination. |
Individual variation | Differences in the traits of individuals are addressed (e.g., the fastest). |
Differential survival potential | Individuals have different survival potentials due to specific traits (e.g., higher survival potential, evolutionary advantage). |
Inheritance of traits | Traits are passed on from the individuals to their offspring (or next generation). |
Reproductive success | Some individuals have higher reproductive success than others. |
Selection pressure | Designation of selection factors, selection pressure, or limited resources (e.g., light, prey). |
Limited survival | Imagine that some individuals will survive, while others die. |
Changes in populations | [Beneficial] traits become more frequent. |
Alternative conceptions | |
Need | Individuals develop the new trait or behavior because they need it to survive (or the trait disappears because they do not need it). |
Use and disuse | New traits or physical changes result from use or nonuse and are passed on directly to the offspring. |
Anthropomorphism | The individual knows about the benefit/lack of benefit of a characteristic and therefore the characteristic appears or disappears. Natural selection (nature) is understood as a sorting-out force. |
Essentialism | The individuals of a population change at the same time and develop the new feature. |
Soft inheritance | Characteristics acquired by an individual during its lifetime are passed on to the offspring. |
Events vs. processes | Natural selection is an event with a beginning and an end (and is not understood as continuous). |
Source vs. sorting of variation | Mutations appear because of a changed environment and are therefore advantageous. |
To quantify students’ evolutionary knowledge in terms of key concept and alternative conception measures more fully, we used the Natural Selection Performance Quotient (NSPQ) of Nehm and Reilly (2007). The NSPQ is derived by multiplying KCD/(KCD + ACD) and KCD/maximum possible key concept score, and expresses the product on a scale of 0 to 100. The “first term expresses the proportion of students’ answers that were correct, and the second expresses how the correct proportion compared with the most complete possible answer” (Nehm and Reilly, 2007, p. 266). Further, the NSPQ distinguishes between students who have significant knowledge of natural selection, but conceptual problems, and those with no alternative conceptions but differing levels of knowledge (Nehm and Reilly, 2007). The mean NSPQ of our sample was 0.55 (SD = 0.31).
High School Grade Point Average (GPA).
The high school GPA is one of the most important criteria for selecting candidates for higher education in Germany (Heine et al., 2006) and is widely used as a proxy for cognitive ability (Anderson and Lebière, 1998). Thus, we used self-reported GPA to assess the convergent validity measures of the RaProEvo and RaProMath tests. GPA was captured by a single item, with scores ranging from 1 (good performance) to 4 (poor performance). The results indicate that our students’ GPAs, and hence cognitive ability, covered a sufficiently wide range for robustly testing our instruments (M = 2.17, SD = 0.53, minimum [min] = 1.00, maximum [max] = 3.50).
Students’ Academic Self-Concept.
To investigate the criterion-related validity measures of the RaProEvo and RaProMath tests, we assessed students’ academic self-concept. This is reportedly a highly important and influential predictor of cognitive and behavioral outcomes such as performance and self-worth, and it also seems to be strongly related to academic achievement (Marsch and Martin, 2011). Further, Paulick et al. (2016) showed that preservice biology teachers’ academic self-concept is positively related to their biological knowledge. Hence, RaProEvo and RaProMath scores should be positively correlated with academic self-concept in evolutionary theory and stochastics, respectively.
To assess our participants’ academic self-concept, we used the Knowledge Processing subscale of the Berlin Evaluation Instrument for Self-Evaluated Student Competencies (BEvaKomp; Braun et al., 2008). This instrument operationalizes knowledge processing as students’ self-reported competency (based on self-knowledge and evaluation of value or worth of one’s own capabilities) regarding a specific subject. We adapted the (five) selected BEvaKomp items to the topics of evolutionary theory and stochastics, then asked our students to provide Likert-type responses ranging from 1 (does not apply at all) to 4 (fully applies) to the items regarding both contexts (see Supplemental Material 3 for items). The results indicate that our students had medium self-reported competency in evolutionary theory (M = 3.04, SD = 0.72, min = 1.00, max = 4.00; Cronbach’s alpha = 0.93) and somewhat lower self-reported competency in stochastics (M = 2.42, SD = 0.82, min = 1.00, max = 4.00; Cronbach’s alpha = 0.94). All rating levels were chosen by at least five students.
Statistical Analysis
Test Instrument Dimensionality.
To tackle the question of whether students’ conceptual knowledge of randomness and probability in the context of evolution and mathematics follows a single dimension or is better modeled as two separate dimensions, we first conducted a principal components analysis on Rasch scores in IBM SPSS Statistics (version 23). It has been suggested that unidimensionality be assumed when the first component explains at least 20% of the total variance (Reckase, 1979). Further, a single dimension is supported with one large eigenvalue and a large ratio of the first and second eigenvalue (Hutten, 1980; Lord, 1980).
Rasch analysis was applied in ACER ConQuest (version 1; Wu et al., 2007) to analyze the psychometric distinction of students’ conceptual knowledge of randomness and probability in the contexts of evolution and mathematics. Because the two tests were designed to capture students’ conceptual knowledge of randomness and probability in two contexts, a two-dimensional model was fitted to the data, based on the assumption that students have separable competencies for evolution and mathematics, which can be captured as the latent traits “competency in RaProEvo” (measured by the 21 evolutionary items) and “competency in RaProMath” (measured by the 33 mathematical items), respectively. This model was compared with a one-dimensional model presuming a single competency, that is, that items represent one latent trait (“competency in randomness and probability,” measured by 21 evolutionary combined with 33 mathematical items).
To determine which model provides the best fit to the acquired data, we calculated final deviance values, which are negatively correlated with how well the model fits the data (and thus indicate degrees of support for underlying assumptions). To test whether the two-dimensional model fits the data significantly better than the one-dimensional model, we applied a χ2 test (Bentler, 1990). In addition, we applied two information-based criteria, Akaike’s (1981) information criterion (AIC) and Bayes’s information criterion (BIC), to compare the two models. These criteria do not enable tests of the significance of differences between models, but generally the values are negatively correlated to the strength of how well the model fits the data (Wilson et al., 2008).
Test Instrument Evaluation by Rasch Modeling.
Assuming that evolution and mathematics competencies differ, the reliability measures and internal structure of the RaProEvo and RaProMath instruments were evaluated by analyzing the participants’ responses using the Rasch partial-credit model (PCM) and Wright maps. The PCM is rooted in item response theory and provides a means for dealing with ordinal data (Wright and Mok, 2000; Bond and Fox, 2015) by converting them into interval measures, thus allowing the calculation of parametric descriptive and inferential statistics (Smith, 2000; Wright and Mok, 2000; Bond and Fox, 2015). The discrepancy between a considered PCM and the data is expressed by so-called fit statistics (Bond and Fox, 2015). Because person and item measures are used for further analyses, only items fitting the model should be included; otherwise, values of these measures could be skewed and lead to wrong conclusions in further analyses. To calculate fit statistics for the RaProEvo and RaProMath instruments, we used ACER ConQuest item response modeling software (version 1; Wu et al., 2007). ConQuest provides outfit and infit mean square statistics (hereafter outfit and infit, respectively) to measure discrepancies between observed and expected responses. The infit statistic is mainly used for assessing item quality, as it is highly sensitive to variation in discrepancies between models and response patterns, while outfit is more sensitive to outliers (Bond and Fox, 2015). Furthermore, aberrant infit statistics usually raise more concern than aberrant outfit statistics (Bond and Fox, 2015). Therefore, we used the weighted mean square (WMNSQ): a residual-based fit index with an expected value of 1 (if the underlying assumptions are not violated), ranging from 0 to infinity. We deemed WMNSQ values acceptable if they were within the range 0.5–1.5 (Wright and Linacre, 1994) and had t values that did not significantly deviate from 1.0 (being within the range −2.0–2.0).
To test whether the developed test instruments fit the Rasch model, we calculated model fit indices regarding the items and participants’ abilities (“person ability”). Person ability and item difficulty were estimated using Masters’s (1982) partial-credit model, as RaProEvo includes a mixture of dichotomously scored and partial-credit items. The partial-credit model allows analysis of items scored in more than two ordered categories, with different measurement scales for different items, and estimates a distinct threshold parameter for each item (Wright and Mok, 2000). Four reliability indices—person reliability, person separation, item reliability, and item separation—were calculated (Bond and Fox, 2015). For further analysis, person parameters were estimated by calculating weighted maximum likelihood estimation (WLE) values.
Validity Measures Check.
Spearman’s rho correlation coefficients were used to assess criterion-related (convergent/discriminate) validity measures of the applied instruments and the relationship between students’ knowledge of evolutionary theory and their conceptual understanding of randomness and probability. The instruments’ convergent validity measures were assessed by testing the association between the participants’ person ability scores and GPAs (assumed to be negatively correlated), while their discriminant validity measures were assessed by testing the association between their person ability scores and academic self-concepts (assumed to be stronger for corresponding than for noncorresponding self-concepts).
Furthermore, we applied one-way analyses of covariance (ANCOVAs) to explore differences between biology majors’ and preservice biology teachers’ knowledge in terms of 1) person RaProEvo ability, 2) person RaProMath ability, and 3) students’ evolutionary knowledge (KCD, ACD, and NSPQ). In all cases, participant GPA was a covariate.
RESULTS
Test Instrument Dimensionality
Rasch score principal components analysis was conducted to tackle the issue of dimensionality. The first component obtained in this analysis explained 11.25% of the total variance. In order, the eigenvalues of the first five components were 6.08, 3.71, 2.88, 2.39, and 2.13. Correspondingly, the ratio of the first and second eigenvalues was 1.64, indicating the lack of a dominant single dimension.
To determine whether students’ conceptual knowledge of randomness and probability in the context of evolution is psychometrically distinct from their mathematical knowledge of randomness and probability, we compared two-dimensional and one-dimensional partial-credit models fitted to data obtained from coding 140 biology students’ responses to the two instruments. Rasch analysis results and AIC values indicate that the two-dimensional model provides a better fit to the data, although values of the other information-based criterion applied (BIC) indicates that the one-dimensional model provides a better fit (Table 5). Nevertheless, results of a χ2 test show that the two-dimensional model significantly outperformed the one-dimensional model: χ2 (2, N = 140) = 6.23, p = 0.044. Thus, students’ conceptual knowledge of randomness and probability in evolutionary and mathematical contexts appear to be empirically separable competencies. Accordingly, the Spearman’s correlation coefficients between their knowledge in the two contexts were rlatent = 0.86 and rmanifest = 0.59 (p < 0.001), indicating that the two competencies are closely related but distinct.
Context of conceptual knowledge | One-dimensional model | Two-dimensional model | |
---|---|---|---|
Allocation to dimension | Evolution | A | A |
Mathematics | A | B | |
Deviance (no. of free parameters) | 6178.15 (57) | 6171.92 (59) | |
AIC | 6292.15 | 6289.92 | |
BIC | 6459.83 | 6463.48 |
Test Instrument Analysis
As the two-dimensional model represents students’ conceptual knowledge of randomness and probability slightly better than the one-dimensional model, the results regarding the reliability measures and internal structure of RaProEvo (N = 140, 21 items) and RaProMath (N = 140, 33 items) are presented separately (see Supplemental Tables S2 and S3 for item parameter estimates).
RaProEvo.
The Wright map acquired from analysis of the RaProEvo test results (Figure 1) was used to analyze the internal structure of the instrument (Boone and Rogan, 2005). In such a map, the distributions of persons and items of the instrument (or more strictly person ability and item difficulty estimates) are plotted along the same dimension (conventionally to the left and right, respectively) and can be directly compared. Items of equivalent difficulty are located at the same position on the scale (e.g., rpE14 and nsE18; Figure 1), and persons at the same position or height on the scale as a particular item have a 50% chance of answering that item correctly, while those located above and below an item have a 50% higher and lower chance of answering it correctly, respectively. The RaProEvo Wright map suggests that a typical respondent would answer most questions correctly, as 38.1% and 61.9% of the items were, respectively, above and below the position of the mean person (dotted line). Nevertheless, fits for items forming the test for conceptual knowledge of randomness and probability in evolution were acceptable, with WMNSQ values ranging from 0.81 to 1.07 and t values from −1.9 to 0.7. For the 21 items of the RaProEvo test, an item separation reliability of 0.98, a WLE person separation reliability of 0.58, and a Cronbach’s alpha (internal consistency) value of 0.66 were computed. Mean person ability (person parameters) was found to be 0.01 (SD = 0.08), and the mean score (item parameters) was 17.27 points (SD = 2.93, range: 6–22 points, maximum possible score = 23 points).
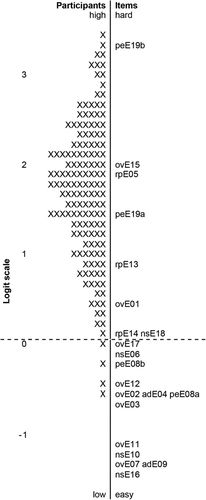
FIGURE 1. Wright map of responses to items of the RaProEvo test (N = 140; 21 items). Abilities of persons who took the test are displayed on the left and difficulty of the (coded) items on the right. Each “X” indicates 0.9 individuals in the sample. The first two letters represent: ov, origin of variation; ad, accidental death (single event); rp, random phenomena; ns, process of natural selection; and pe, probability of events. “E” represents the content of evolutionary theory; the numbers 01–19 indicate the item number in the RaProEvo test; and the last letter represents item 1 (“a”) or item 2 (“b”) within a similar item task.
RaProMath.
The Wright map acquired from analysis of the RaProMath test results (Figure 2) was also used to assess the internal structure of the instrument (Boone and Rogan, 2005). Like the RaProEvo map, it suggests that a typical respondent would answer most questions correctly, as 42.4% and 57.6% of the items were above and below the position of the mean person, respectively (dotted line). Like those in the RaProEvo test, the items forming the test for conceptual knowledge of randomness and probability in mathematics had acceptable fit, with WMNSQ values ranging from 0.80 to 1.12 and t values from −1.8 to 1.8. For the 33 items of the RaProMath test, an item separation reliability of 0.99, a WLE person separation reliability of 0.68, and a Cronbach’s alpha (internal consistency) value of 0.69 were computed. Mean person ability (person parameters) was found to be 0.02 (SD = 0.90), and the mean score (item parameters) was 24.01 points (SD = 3.66, range: 10–31 points, maximum possible score = 33 points). Additionally, the joint Wright map generated from the two-dimensional model (Figure 3), which enables comparison of patterns of knowledge of randomness and probability in evolution and mathematics contexts, shows that the two instruments detected similar spread in our students’ abilities.

FIGURE 2. Wright map of responses to items of the RaProMath test (N = 140; 33 items). Abilities of persons who took the test are displayed on the left and difficulty of the (coded) items on the right. Each “X” indicates 1.1 individuals in the sample. The first two letters represent: se, single event; rp, random phenomena; pr, probability as ratio; pe, probability of events; and sr, sample reasoning. “M” represents the content of mathematics; the numbers 01–33 indicate the item number in the RaProMath test.

FIGURE 3. Wright map of responses to items linked to the two dimensions of the RaProEvo test (bold; N = 140; 21 items) and RaProMath test (N = 140; 33 items). Abilities of persons who took the test are displayed on the left and the difficulty of the (coded) items on the right. Each “X” indicates 1.0 individuals in the sample. The first two letters represent: ov, origin of variation; ad, accidental death (single event); rp, random phenomena; se, single event; ns, process of natural selection; pe, probability of events; pr, probability as ratio; and sr, sample reasoning. E01 to E19 indicate the item number in the RaProEvo test; M01 to M33 represent the item number in the RaProMath test; and the last letter represents item 1 (“a”) or item 2 (“b”) within a similar item task.
Validity Measure Check
To test the instruments’ validity measures, we first analyzed the relationships between the participants’ GPAs and person ability in the two knowledge dimensions of randomness and probability in evolutionary and mathematical contexts to assess their convergent validity measures. The results confirmed our hypotheses that GPA values would be negatively correlated with both RaProEvo and RaProMath person abilities (rs = −0.25, p = 0.004, and rs = −0.33, p < 0.001, respectively; n = 129 in both cases).
Next, we analyzed the relationship between the two dimensions of person ability (knowledge of randomness and probability in evolutionary and mathematical contexts) and the participants’ academic self-concepts to assess the tests’ discriminant validity measures. The results confirmed our hypothesis that participants’ academic self-concepts in the contexts of evolutionary theory and mathematics would be more strongly connected to their RaProEvo and RaProMath composite scores, respectively (Table 6).
Academic self-concept | ||
---|---|---|
Evolutionary theory | Stochastics | |
RaProEvo | 0.40** | 0.13 |
RaProMath | 0.19* | 0.23** |
The results also showed that KCD in students’ responses was significantly positively related to person ability as measured by both RaProEvo (rs = 0.45) and RaProMath (rs = 0.35), while ACD was significantly negatively related to person ability (rs = −0.32 and −0.17, respectively). Furthermore, the NSPQ was significantly positively related to person ability measured by RaProEvo (rs = 0.47) and RaProMath (rs = 0.36). These findings (p < 0.001, N = 140 in all cases) confirm the hypothesis that their conceptual knowledge of evolutionary theory would be positively correlated with their conceptual knowledge of randomness and probability.
Biology Majors versus Preservice Biology Teachers
To assess effects of study program on the participants’ performance, we applied one-way ANCOVAs to compare RaProEvo- and RaProMath-measured abilities of biology majors and preservice biology teachers and their KCD scores, ACD scores, and NSPQs while controlling for cognitive ability as indicated by GPA.
We detected a significant effect of study program on RaProEvo scores: biology majors obtained significantly higher RaProEvo person ability scores (adjusted M = 0.33, SEM = 0.11, n = 69) than preservice biology teachers (adjusted M = −0.33, SEM = 0.12, n = 60): F(1, 126) = 15.97, p < 0.001. In contrast, the study program had no significant effect on RaProMath scores: F(1, 126) = 1.54, p = 0.217. Regarding KCD and ACD scores, biology majors (adjusted M = 5.02, SEM = 0.29, n = 69) used significantly more key concepts in their answers than preservice biology teachers (adjusted M = 4.00, SEM = 0.31, n = 60): F(1, 126) = 5.78, p = 0.018, while study program had no significant effect on numbers of alternative conceptions identified in their responses: F(1, 126) = 1.40, p = 0.239. Nevertheless, biology majors obtained significantly higher NSPQs (adjusted M = 0.60, SEM = 0.04, n = 69) than preservice biology teachers (adjusted M = 0.46, SEM = 0.04, n = 60): F(1, 126) = 6.27, p < 0.001.
DISCUSSION
We have attempted to address the need for instruments capable of measuring understanding of two important abstract concepts underlying the biological concepts in evolutionary theory (randomness and probability) and advance evolutionary education research. Using the presented instruments, we explored the psychometric distinction of biology students’ conceptual knowledge of randomness and probability in the context of both evolution (RaProEvo) and mathematics (RaProMath). We then assessed the reliability and validity measures of the RaProEvo and RaProMath instruments. Finally, we investigated the relationships of RaProEvo and RaProMath scores with evolutionary knowledge (KCD, ACD, and NSPQ) and the difference in this knowledge between biology majors and preservice biology teachers.
Several of the empirical findings are of potential interest, particularly given the importance of understanding randomness and probability, both in science generally, as highlighted in national and international education standards (KMK, 2005a,b; NGSS, 2013), and specifically in teaching and learning evolution (Mead and Scott, 2010; Tibell and Harms, unpublished data). First, the percentage of the total variance explained by the first components of a Rasch score principal components analysis and the ratio of the first and second eigenvalues of this principal components analysis reveals a lack of unidimensionality. Second, Rasch analysis also indicated that a two-dimensional model fits the participants’ responses slightly but significantly better than a one-dimensional model, supporting the assumption that RaProEvo and RaProMath measure separate competencies. We obtained promising indications of the instruments’ reliability measures, albeit preliminary due to the small sample size, and their validity measures were confirmed by experts and criterion-related indications. Furthermore, biology students’ RaProEvo scores, KCDs, and NSPQs (but not ACDs) were all higher than those of the preservice teachers, indicating that they had more evolutionary knowledge. In contrast, RaProMath scores did not differ between biology students and preservice teachers.
Randomness and Probability Knowledge
There was a good fit between the data set and the Rasch model, indicating that the tests had strong internal validity measures. Detailed analysis indicated that the RaProEvo instrument’s difficulty was not optimal for our sample of biology students: many items clustered at the low end of the scale, and there was a lack of sufficiently difficult items to distinguish high performers. Nevertheless, the Wright maps obtained from our analysis of responses to items of the tests provided indications of informative patterns regarding students’ thinking (which require further verification), as outlined in Test Instrument Analysis.
The RaProEvo Wright map indicates that most students could satisfactorily answer items regarding the process of natural selection (Figure 1), which mainly concerned broad, probabilistic aspects of the process, rather than specific contributory processes or key associated concepts. Illustrative phenomena used in these questions might be mostly familiar, such as changes in color of foxes’ fur in adaptive responses to environmental changes, a frequently used example of natural selection–mediated change that many students may learn from textbooks. In contrast, only high-performing students correctly answered questions with complex probabilistic backgrounds (psE19; probability of events).
Similar patterns were discerned in responses to the RaProMath instrument. Questions concerning probability as a ratio seemed quite easy for the participants. This may seem unsurprising, as pupils learn to calculate ratios in primary school (KMK, 2005b). However, only high performers correctly answered items concerning probability of events, although students also should have learned this topic in school (KMK, 2004, 2015). This finding corroborates indications presented by various authors (e.g., Chi et al., 1981) that students tend to ignore connections to underlying concepts (e.g., probability) that would allow them to transfer their understanding to other problems. This is a concern, as students have to calculate and apply ratios explicitly in biology to topics such as Mendelian inheritance and Hardy-Weinberg equilibrium (e.g., Campbell et al., 2006) and (more often) implicitly in diverse contexts (e.g., the influence of alleles’ selective strength on the probability of fixation as a function of the strength of genetic drift), which increases the sophistication of the required conceptualization (Tibell and Harms, unpublished data).
In the mathematical context, students found some of the single-event items challenging (some were apparently easy, but responses to more than half were distributed across the scale). Even when asked about the (un)predictability of single events, students seemed to think about predictability in aggregate terms. Similarly, in the evolutionary context, items regarding origin of variation, either generally (e.g., ovE03, ovE17) or linked to specific sources of variation like recombination (e.g., ovE07) and mutation (e.g., ovE12) were also distributed across the entire scale. Finally, random phenomena seemed quite challenging for our students in evolutionary contexts. When they had to explain why evolutionary change through natural selection is a nonrandom process, they often forgot that natural selection acts upon randomly generated variation. Indeed, as noted by Mayr (2001): “Without variation, there would be no selection.” Even Darwin (1859) suggested that variation is a fundamental requirement for evolutionary change in On the Origin of Species by Means of Natural Selection (for more information, see Gregory, 2009), although he could not explain where the variation comes from. Nevertheless, only 17% of the participants stated this in their answers.
A particularly important source of new variation in the focal contexts is mutation, which is regarded as a random process, partly because the probability of mutations occurring is not affected by the selective consequences and partly because their occurrence in a given individual at a given time is far beyond our modeling capacities (Gregory, 2009; Heams, 2014). Nevertheless, several studies have indicated that students tend to struggle with both the importance of random processes such as the origin of variation in evolutionary processes and understanding why mutations are called random (e.g., Garvin-Doxas and Klymkowsky, 2008; Smith et al., 2008; Speth et al., 2014). Our results corroborate these findings that random processes pose learning difficulties.
Differences between Biology Majors and Preservice Biology Teachers
Most studies of evolutionary knowledge focus on differences between novice and advanced students attending similar study programs (e.g., Frasier and Roderick, 2011; Nehm and Ridgway, 2011). However, possible differences between biology majors and preservice biology teachers are also potentially important, particularly as the latter will form the next generation to teach evolutionary theory. So, it might be acceptable for preservice biology teachers to lack detailed knowledge of specific associated processes, and thus obtain lower scores in tests such as RaProEvo, but they should have similar general understanding (as measured, e.g., by KCD and NSPQ) of evolutionary change through natural selection. Alarmingly, we found significant deficits (relative to the biology majors) in both their conceptual knowledge of randomness and probability in evolutionary contexts and their evolutionary knowledge. These findings cannot be explained by differences in cognitive abilities, because we accounted for variations in participants’ GPAs, and Klusmann (2013) found no differences in cognitive characteristics between students attending to become a teacher and other university education courses. However, we cannot exclude the possibility that these findings are simply a manifestation of differences that existed between the groups before their higher educational training.
Regardless of the reasons for the preservice teachers’ lower RaProEvo scores, there will clearly be potential problems in teaching evolution if the next generation of teachers has only modest knowledge of evolution(or harbors misconceptions about it). Thus, when considering strategies to improve biology students’ understanding, it is important not only to foster development of accurate evolutionary knowledge but also to ensure that the next generations of teachers develop an adequate knowledge base. As proposed by Tibell and Harms (unpublished data), a step toward appropriate solutions could be to deepen students’ knowledge of abstract concepts underlying evolutionary processes.
Limitations and Future Research
Mathematics is a compulsory subject in school, and mathematical concepts, particularly randomness and probability, are fundamental elements of descriptions of myriad biological interactions, relationships, and processes (Jungck, 1997; Chiel et al., 2010). However, most previous studies on evolutionary knowledge have solely considered biological aspects (Tibell and Harms, unpublished data). A major implication of our study is that conceptual knowledge of randomness and probability is important for biology students’ understanding of evolutionary theory. In contrast to other studies on students’ misunderstanding of random processes (Garvin-Doxas and Klymkowsky, 2008; Robson and Burns, 2011), we also detected clear differences in students’ conceptual knowledge of randomness and probability in evolutionary and mathematical contexts. In developing the instruments, we also tried to extend extant research by showing that threshold concepts are important factors for a deeper conceptual knowledge of evolutionary theory, and thus important in students’ education.
Nevertheless, instruments such as RaProEvo and RaProMath have intrinsic limitations, partly because they need to be reasonably short and not require much time to complete or mark. Thus, they must include only a few items targeting each concept. Hence, the instruments should be used mainly for formative purposes, that is, for instructors to identify obstacles their students are currently facing. The instruments were not intended to be summative evaluation tools. The utility of RaProEvo and RaProMath lies in their proposed ability to assess students’ general conceptual knowledge about randomness and probability in two contexts (evolution and mathematics), rather than exhaustively assess their knowledge of specific constructs (e.g., genetic drift).
We also note the obvious limitation of the small sample size in our study. We obtained promising preliminary results, but the reliability measures of the instruments must be confirmed with a larger group of students. Further, the participants were all German students from a single cohort. To assess the generality of the findings and identify causes of possible variations in findings, tests of the instruments internationally and with other cohorts are required.
Finally, we hope that our instruments will facilitate efforts to design more tools to assess students’ conceptual knowledge of randomness and probability. In addition, having developed an instrument for measuring conceptual knowledge of randomness and probability in the context of evolution (RaProEvo), we found that instruction about randomness and probability connected to evolutionary concepts warrants attention. Therefore, an objective of ongoing research is to investigate whether visualizations and/or instructional support can help students to develop better conceptual knowledge of the roles of randomness and probability in evolution and, hence, better evolutionary knowledge.
ACKNOWLEDGMENTS
This study is part of the Swedish–German cooperation project “EvoVis: Challenging Threshold Concepts in Life Science—enhancing understanding of evolution by visualization,” funded by the Swedish Research Council (VR 2012:5344, LT). We thank the whole EvoVis group and Dr. Jörg Großschedl and Charlotte Neubrand for helpful discussions that contributed to this study. Special thanks to Dr John Blackwell for the language review and valuable and insightful comments on evolution aspects of the paper. We are also very thankful to the students who participated in this study and the experts for their comments on the instruments.