Development of a Biological Science Quantitative Reasoning Exam (BioSQuaRE)
Abstract
Multiple reports highlight the increasingly quantitative nature of biological research and the need to innovate means to ensure that students acquire quantitative skills. We present a tool to support such innovation. The Biological Science Quantitative Reasoning Exam (BioSQuaRE) is an assessment instrument designed to measure the quantitative skills of undergraduate students within a biological context. The instrument was developed by an interdisciplinary team of educators and aligns with skills included in national reports such as BIO2010, Scientific Foundations for Future Physicians, and Vision and Change. Undergraduate biology educators also confirmed the importance of items included in the instrument. The current version of the BioSQuaRE was developed through an iterative process using data from students at 12 postsecondary institutions. A psychometric analysis of these data provides multiple lines of evidence for the validity of inferences made using the instrument. Our results suggest that the BioSQuaRE will prove useful to faculty and departments interested in helping students acquire the quantitative competencies they need to successfully pursue biology, and useful to biology students by communicating the importance of quantitative skills. We invite educators to use the BioSQuaRE at their own institutions.
INTRODUCTION
Multiple national reports—BIO2010, Scientific Foundations for Future Physicians, and Vision and Change—have called for reform in biology education (National Research Council [NRC], 2003; Association of American Medical Colleges–Howard Hughes Medical Institute Joint Committee [AAMC-HHMI], 2009; American Association for the Advancement of Science [AAAS], 2011). Each report emphasizes the quantitative nature of biology and the need for students to be able to apply mathematical concepts and models to formally describe complex biological phenomena (Bialek and Botstein, 2004). Inadequate mathematics preparation has been suggested as one reason that students fail to obtain degrees in the various disciplines of science, technology, engineering, and mathematics (STEM). Fewer than 50% of high school students who took the ACT, for example, meet or exceed math or science benchmarks that indicate they will do well in college algebra I or introductory biology (ACT, 2015).
Whether referring to it as a “STEM crisis,” a “quantitative reasoning crisis” (Gaze, 2014), or the “mathematics-preparation gap” (President’s Council of Advisors on Science and Technology [PCAST], 2012), many suggest that weak quantitative preparation is in part to blame for the low percentage of college degrees awarded in STEM (Business Higher Education Forum, 2011). In 2005 the percentage of incoming students reporting a need for remedial math in college was 24% (Pryor et al., 2007). Studies indicate that, for students attending community colleges, mathematics is a barrier to graduation for approximately two-thirds of those who arrive underprepared in mathematics (Bryk and Treisman, 2010). These observations and others led the PCAST (2012) to recommend “a national experiment in postsecondary mathematics education to address the mathematics-preparation gap” (p. 27).
Because not all entering college students complete the ACT or SAT and an increasing number of schools have adopted test-optional admissions policies, a key challenge for instructors and departments of biology comes from the need to identify an instrument that provides feedback about the quantitative skills of arriving students interested in biology. Such data allow instructors, departments, or programs to consider the skills needed by students and the point(s) in the curriculum at which students can hone or develop these competencies.
Existing instruments aimed at describing the quantitative acumen of students assess quantitative reasoning in the broad sense (e.g., not in a specific context, such as biology), serve as tools to examine specific pedagogical interventions, or focus on precalculus or calculus skills (Carlson et al., 2010, 2015). For example, the Quantitative Literacy and Reasoning Assessment (QLRA; Gaze et al., 2014a,b), the Test of Scientific Literacy Skills (TOSLS; Gormally et al., 2012), and the Quantitative Reasoning Test (Sundre, 2008) measure general quantitative skills. Individually, these instruments examine only a subset of the quantitative skills indicated by national reports as necessary for success as a biology major and pose questions in multiple contexts. Alternatively, several instruments assess the pedagogical impacts of specific interventions. For example, assessment tools have been developed for evaluating the impact of online resources such as MathBench (Thompson et al., 2010) or course-based interventions such as Data Nuggets (Schultheis and Kjelvik, 2015) or curricular change (Speth et al., 2010). Finally, the Pre-calculus Concept Assessment (Carlson et al., 2010) and the Calculus Concept Readiness (Carlson et al., 2015) probe understanding and reasoning abilities required for beginning calculus.
Missing from this repertoire of tools is an instrument derived from the recommendations found in reports such as BIO2010, Scientific Foundations for Future Physicians, and Vision and Change (NRC, 2003; AAMC-HHMI, 2009; AAAS, 2011). Such an instrument would enable instructors, departments, and divisions to describe the baseline quantitative skills of incoming biology students and serve as a prompt to recognize the point(s) at which students develop such skills during an introductory curriculum that might include supporting courses from other departments (e.g., chemistry, mathematics, statistics, physics). Such empirical data about the skills students arrive with and the skills that instructors think are important for success could contribute to creating or adapting strategies to provide opportunities for learning. Aikens and Dolan (2014, p. 3479) highlighted the acute need for such targeted assessment instruments with this call:
More tools are needed to document students’ progress toward quantitative biology-related outcomes, especially beyond introductory or nonmajors biology. To this end, we encourage teams of biologists, quantitative scientists, and education specialists to collaborate in developing and testing a broader suite of assessment tools related to quantitative biology.
In response, we (a consortium of faculty from nine liberal arts colleges and educational psychologists from two universities) have developed a 29-item instrument, the Biology Science Quantitative Reasoning Exam (BioSQuaRE). The BioSQuaRE assesses the quantitative skills (as outlined in national reports) that students should possess after they have completed an introductory biology sequence. This paper documents the development and psychometric strength of the BioSQuaRE as a tool to measure quantitative skills within the context of biology. Efforts to align the assessment to national reports, gather expert feedback, and validate the inferences made from the assessment by collecting a developmental data set and applying the Rasch model are detailed. We invite readers to use the BioSQuaRE at their own institutions to communicate the importance of quantitative skills to life science students and to provide data to faculty on the quantitative acumen of their students and the efficacy of curricular reforms.
BioSQuaRE DEVELOPMENT
Throughout the development of BioSQuaRE, we employed multiple methods to examine the measurement quality of our assessment as recommended in the Standards for Educational and Psychological Testing (American Educational Research Association, American Psychological Association, National Council on Measurement in Education, and Joint Committee on Standards for Educational and Psychological Testing [AERA-APA-NCME], 2014). On the basis of this framework, we observed an appropriate degree of validity evidence for the scores and inferences we made about biology students’ quantitative skills.
We began by reviewing five national-level reports that outline core competencies and quantitative skills essential for students enrolled in introductory biology courses: 1) BIO2010 (NRC, 2003), 2) Vision and Change in Undergraduate Biology Education (AAAS, 2011), 3) Scientific Foundations for Future Physicians (AAMC-HHMI, 2009), 4) AP Biology Quantitative Skills: A Guide for Teachers (College Board, 2012), and 5) Next Generation Science Standards Science & Engineering Practices (Next Generation Science Standards [NGSS] Lead States, 2013). Table 1 shows the test blueprint for the BioSQuaRE and the mapping of content recommended by the five reports.
Content | Students should be able to: | BIO2010 | Vision and Change | SFFP | AP Bio | NGSS S&E |
---|---|---|---|---|---|---|
Algebra, functions, and modeling | Carry out basic mathematical computations. (e.g. proportional reasoning, unit conversion, center, and variation) | X | X | X | X | X |
Recognize and use logarithmic or exponential relationships | X | X | ||||
Fit a model such as population growth | X | X | ||||
Use a representation or a model to make predictions | X | X | X | X | X | |
Describe/infer relationships between variables (scatter plots, regression, network diagrams, maps) | X | X | ||||
Perform logical/algorithmic reasoning | X | X | X | X | ||
Statistics and probability | Calculate or use the concept of the likelihood of an event | X | X | X | ||
Calculate or use conditional probability | X | X | X | |||
Recognize and interpret what summary statistics represent | X | X | X | |||
Identify different types of error | X | |||||
Recognize that biological systems are inherently variable (e.g., stochastic vs. deterministic) | X | X | ||||
Formulate hypothesis statements | X | X | X | X | ||
Understand what a p value is | X | X | X | X | ||
Understand when causal claims can be made (e.g., correlation vs. causation) | X | X | X | X | ||
Visualization | Choose the appropriate type of graph | X | X | X | X | X |
Interpret a graph (e.g., functional relationships, logarithmic relationships) | X | X | X | X | X | |
Be able to use a table | X | X | X | |||
Use spatial reasoning to interpret multidimensional numerical and visual data (geographic information) | X |
Thirty-eight faculty members from five liberal arts colleges reviewed the initial blueprint to examine coverage and provide feedback on the importance of the skills and competencies to be assessed in the content areas (Supplement A, Table A1, in the Supplemental Material). The responses indicated that, while the initial test blueprint included content faculty considered important for students, it was also missing content. For example, in response to feedback, we added content related to students’ understanding of logarithmic and exponential relationships. After finalizing the test blueprint (Table 1), 65 faculty members attending the 2016 National Academies Special Topics Summer Institute on Quantitative Biology verified the importance and coverage of blueprint content (Supplement A, Table A2, in the Supplemental Material).
We then used the test blueprint to guide our review of existing assessment instruments. With author permission, we adapted seven selected-response items from two existing instruments (delMas et al., 2007; Sikorskii et al., 2011). The stems of these items were modified to provide biological context and, when necessary, to align the items more closely with our blueprint. Additional items were piloted as free-response questions to explore variation in student responses and converted to selected-response items by using common incorrect responses as distractors, as recommended by Haladyna et al. (2002) and Thorndike and Thorndike-Christ (2010). All item writing was guided by the recommendations of the AERA-APA-NCME (2014) and Haladyna et al. (2002).
The items from the initial version of the BioSQuaRE were iteratively refined based on the analysis of response data from six administrations (see Supplement B in the Supplemental Material for development details). A few items were rewritten extensively or, in some cases, removed. Whenever items were dropped, additional items were written to ensure the content of the BioSQuaRE matched the test blueprint. In general, items have only undergone minor revision. For example, Figure 1 shows the evolution of an item from its origin as a free-response item. After the item was converted into a selected-response item, the stem was also modified for clarity. Additionally, the color palette for the plot was also changed from red–green to blue–yellow, a color combination accessible to students with red–green color blindness. After the second administration, the item’s stem was further clarified, and the number of response options was reduced from four to three based on psychometric analysis.
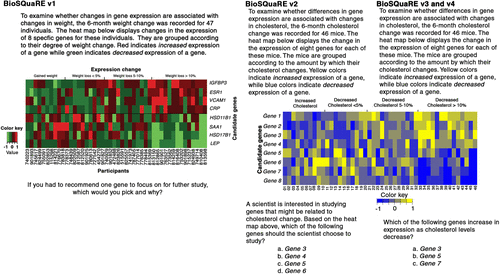
FIGURE 1. Example of changes in a BioSQuaRE item through different administrations. The free-response question in the first administration (version 1) led to the change in coloring and the creation of the selected-response question used in the second administration (version 2). The item stem and number of response choices were further modified in the third and fourth (versions 3 and 4) administrations. This item showed similar psychometric properties in the third, fourth, and fifth administrations.
During the third and fourth administrations of BioSQuaRE, we continued to evaluate item performance. Data were collected from 1140 students from seven different postsecondary institutions across the United States. Participants came from a variety of institutional types (i.e., private liberal arts schools, M1 master’s universities, and R1 and R3 doctoral universities). On the basis of student response information, we refined items as needed. Many of the items performed well and needed no modification. In fact, 19 of the 29 items on the most current form of BioSQuaRE remained the same for the third and fourth administrations. During this time, we also piloted new items that included content covering students’ ability to reason and interpret visual representations of data (see Supplement B in the Supplemental Material for development details).
METHODS
Psychometric analysis was used throughout the development process. Here, we focus on the most recent (fifth) administration of BioSQuaRE, unless otherwise noted. Student responses were analyzed using the framework of item response theory (IRT), a general statistical theory that links students’ item responses and test performance to the underlying trait or ability measured by test content. By capitalizing on this relationship, IRT models can be used not only to compute item statistics (e.g., difficulty, discrimination), but also to estimate student ability (i.e., estimates on the latent trait). Another advantage of IRT is that item statistics are reported on the same scale as ability. The latter allowed us to examine the relationship between students’ ability and their performance on any particular item. Finally, the IRT models also allowed us to estimate the standard errors of scores conditional on student ability.
Although IRT models allow for continuous or mixed-format item responses, we focus here on a set of models that use dichotomously scored responses (correct/incorrect). The simplest of these—the Rasch model—describes students’ observed responses as a function of ability and a single parameter for each item, namely the item’s difficulty. Before presenting the results of the Rasch analysis, we first describe the sample of students included in the analysis.
The most recent BioSQuaRE administration comprised 555 students from five different postsecondary institutions across the United States, including two Hispanic-serving institutions, an M1 master’s university, an R1 doctoral university, and a private liberal arts college. Of the sample, 64% reported being female, and 35% reported being first-generation college students. In addition, 42% of students indicated that they identify as white, 17% as Asian, 23% as Hispanic, 3% as Black, and 10% as some other race or as multiracial. These numbers mirror those obtained through the National Center for Education Statistics (2015, Table 322.30). The students in our sample reported completing a range of biology courses at the postsecondary level; 21% reported that they had completed one or fewer biology courses, 32% reported having completed two or three, and 43% reported having completed four or more.
RESULTS
To begin the analysis of BioSQuaRE, we examined the degree to which items were internally consistent, reflected in the reliability of scores. Several methods have been used to compute the reliability of scores (e.g., coefficient alpha, Gutmann’s lambda), the most common of which is coefficient alpha. Coefficient alpha for the BioSQuaRE scores was 0.81, 95% CI = [0.78, 0.83]. This meets the recommended value of 0.8 for “very good” reliability (Kline, 2011). We also computed the average interitem correlation, which was 0.13. Although not high, the value is not surprising, given the broad content covered on BioSQuaRE. It is also worth noting that score reliability remained fairly constant between the third and fifth administrations.
Under the IRT framework, reliability is considered somewhat differently than under a classical test theory framework; however, under each framework, reliability provides insights about measurement precision. Supplement C in the Supplemental Material describes and discusses additional person and item reliability analysis of the BioSQuaRE instrument.
Rasch Analysis
Using the 29 items from the most current (fifth) administration, the Rasch model was fitted to the 555 respondents’ data using the ‘ltm’ package (Rizopoulos, 2006) in the statistical language R. This model, which expresses the probability of responding correctly to an item, can be expressed mathematically as
where P(Xi = 1) is the probability of responding correctly to item i, θj is the jth student’s ability on the latent trait (i.e., ability level), and θi is the ith item’s difficulty parameter. The item difficulty estimates based on this analysis are provided in Table 2.
B | SE | Content | Item |
---|---|---|---|
Algebra, functions, and modeling | |||
−1.73 | 0.118 | Compute probability from a two-way table | 1 |
−0.59 | 0.096 | Predicting from a genetic model | 24 |
−0.47 | 0.095 | Understanding variation in log-transformed measurements | 3 |
0.48 | 0.095 | Translating content to tabular summaries | 10 |
0.80 | 0.098 | Translating between two graphs of data | 13 |
0.84 | 0.098 | Interpreting plots of logarithms | 14 |
0.92 | 0.100 | Predicting from a recursive model of population growth | 16 |
1.30 | 0.106 | Interpreting plots of logarithms | 15 |
1.69 | 0.116 | Graphing a nonlinear function | 25 |
Statistics and probability | |||
−1.77 | 0.120 | Understanding variation in measurements | 2 |
−1.38 | 0.109 | Translating summary statistics to a distribution | 5 |
−1.35 | 0.108 | Relating sample size to uncertainty | 4 |
−0.62 | 0.096 | Understanding p value | 8 |
−0.47 | 0.095 | Relationship between summary statistics and statistical significance | 23 |
−0.15 | 0.094 | Translating content to a statistical hypothesis | 6 |
−0.04 | 0.093 | Understanding relationship between p value and effect | 9 |
1.10 | 0.102 | Understanding p value | 7 |
Visualization | |||
−1.77 | 0.120 | Interpreting relationships between variables from a line plot | 20 |
−1.05 | 0.102 | Interpreting variation in a heat map | 11 |
−0.86 | 0.099 | Interpreting relationships between variables from a line plot | 19 |
−0.61 | 0.096 | Interpreting interaction effects from a plot | 18 |
−0.55 | 0.096 | Interpreting trend in a heat map | 12 |
−0.47 | 0.095 | Understanding relationship between data, RQ, and plot | 28 |
−0.20 | 0.094 | Interpreting variation in a choropleth map | 22 |
−0.16 | 0.094 | Interpreting interaction effects from a plot | 17 |
0.06 | 0.093 | Interpreting trend in a choropleth map | 21 |
0.52 | 0.095 | Understanding relationship between data, RQ, and plot | 26 |
0.55 | 0.096 | Understanding relationship between data, RQ, and plot | 27 |
1.32 | 0.107 | Understanding relationship between data, RQ, and plot | 29 |
The difficulty estimates presented in Table 2 indicate the ability level at which the probability of responding to the item correctly is 0.5. Thus, students with an ability level higher than the difficulty estimate are more likely to respond correctly to the item than they are to respond incorrectly. (Note that the ability levels are standardized so that an ability level of 0 indicates average ability.) The items show a range of difficulty; some are easier (negative values) and some are more difficult (positive values).
Model–Data Fit
Using the Rasch paradigm, one can examine how well the student response data fit the underlying Rasch model. While there have been many proposed methods for evaluating different aspects of the IRT model fit, there is no consensus on which approach is best (van der Linden and Hambleton, 1997). To evaluate how well our data align with the Rasch model, we opted to examine both model-level and item-level fit.
Model-Level Fit.
To examine the fit at the model level, we first used Monte Carlo simulation to generate 200 data sets from the Rasch model, and compute Pearson’s r2 for each of the data sets. The observed value of Pearson’s r2 from the development data set does not suggest a misfit to the Rasch model (p = 0.350). We also examined several other model-level fit indices. Table 3 shows that the root-mean-square error of approximation (RMSEA) and two standardized root-mean-square residual approaches (SRMR and SRMSR) indicate good to reasonable fit to the model.
Fit measure | Value | Criteria for “good” model fit | ||||||||||||||||||||||||
---|---|---|---|---|---|---|---|---|---|---|---|---|---|---|---|---|---|---|---|---|---|---|---|---|---|---|
RMSEA [95% CI] | 0.041 [0.034, 0.047] |
| ||||||||||||||||||||||||
SRMR | 0.058 |
| ||||||||||||||||||||||||
SRMSR | 0.075 |
|
Item-Level Fit.
To further explore the fit of the Rasch model, we examined measures of item-level fit. The mean squared infit and outfit statistics represent two common measures of item fit. These measures have an expected value of 1 under the Rasch model. Items that have infit and outfit values that deviate too far from 1 do not fit the model and are viewed as not productive to the measurement process. Linacre (2002) suggests that values between 0.5 and 1.5 indicate reasonable fit to the Rasch model. Items with values below 0.5 or above 1.5 are misfit to the model and are generally not productive to the measurement process; and items having values above 2.0 can even hinder the measurement process. Table 4 shows the mean squared infit and outfit statistics for the BioSQuaRE items. None of the items suggests misfit to the model.
Algebra, functions, and modeling | Statistics and probability | Visualization | ||||||||||||
---|---|---|---|---|---|---|---|---|---|---|---|---|---|---|
Yen (1981) | Yen (1981) | Yen (1981) | ||||||||||||
Item | Infit | Outfit | r2 | p | Item | Infit | Outfit | r2 | p | Item | Infit | Outfit | r2 | p |
1 | 0.94 | 0.89 | 8.76 | 0.582 | 2 | 0.92 | 0.86 | 14.29 | 0.149 | 11 | 0.94 | 0.91 | 16.59 | 0.095 |
3 | 0.98 | 0.97 | 10.23 | 0.567 | 4 | 0.98 | 0.93 | 8.18 | 0.647 | 12 | 0.94 | 0.92 | 18.20 | 0.065 |
10 | 0.91 | 0.87 | 30.70 | 0.005 | 5 | 0.96 | 0.92 | 9.79 | 0.478 | 17 | 1.08 | 1.10 | 2.51 | 0.990 |
13 | 1.08 | 1.11 | 14.06 | 0.214 | 6 | 0.94 | 0.92 | 13.49 | 0.224 | 18 | 1.03 | 1.08 | 14.47 | 0.194 |
14 | 0.95 | 0.93 | 17.64 | 0.060 | 7 | 0.94 | 0.96 | 15.53 | 0.124 | 19 | 0.97 | 0.93 | 10.44 | 0.453 |
15 | 1.00 | 1.00 | 7.34 | 0.741 | 8 | 1.08 | 1.15 | 12.85 | 0.239 | 20 | 1.02 | 1.27 | 5.92 | 0.846 |
16 | 0.96 | 0.94 | 16.33 | 0.105 | 9 | 1.08 | 1.09 | 10.58 | 0.448 | 21 | 1.00 | 0.98 | 12.02 | 0.403 |
24 | 1.06 | 1.08 | 16.70 | 0.100 | 23 | 0.92 | 0.89 | 17.04 | 0.080 | 22 | 0.93 | 0.90 | 14.59 | 0.204 |
25 | 1.04 | 1.19 | 10.27 | 0.408 | 26 | 1.06 | 1.07 | 14.26 | 0.149 | |||||
27 | 1.15 | 1.23 | 8.64 | 0.697 | ||||||||||
28 | 0.97 | 0.95 | 6.41 | 0.836 | ||||||||||
29 | 1.11 | 1.25 | 11.04 | 0.428 |
Item fit was also examined by using a simulation method suggested by Yen (1981) that categorizes students based on their ability estimates. The proportion of students responding correctly to a particular item is then evaluated against the expected proportion using an r2 goodness of fit test. A significant r2 value indicates potential item misfit. Table 4 also shows the results of fitting this analysis to the BioSQuaRE data using 200 Monte Carlo replications. Item 10 is the only item that suggests potential misfit to the model. The decision was made to keep item 10 despite the significance of Yen’s method, because the infit and outfit measures for item 10 were both reasonable, and fit evidence from significance tests tends to flag items as misfitting more than they should (i.e., type I error).
Wright Map
Using the Rasch model, we computed ability estimates from the student response data from the most current (fifth) administration of the BioSQuaRE. These estimates are a function of the item parameters and the students’ response patterns. A Wright map (Wright and Masters, 1982; Figure 2) provides a visual representation of the BioSQuaRE by plotting the item difficulty values on the same measurement scale as the ability of the respondents. This allows a comparison of both respondents and items, which helps us to better understand the measurement properties of the BioSQuaRE instrument.
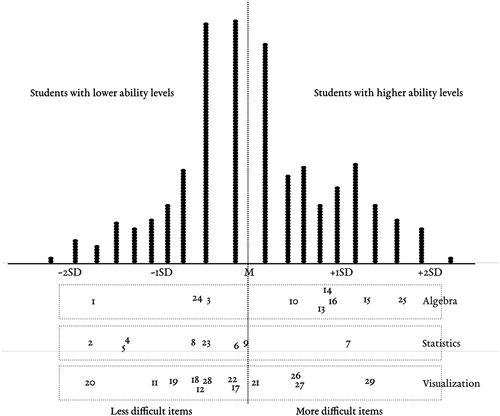
FIGURE 2. Wright map of the 555 respondents’ estimated ability levels (top half) and the estimated difficulty parameters for the 29 BioSQuaRE items sorted by primary content area (bottom half). A vertical line is displayed at the mean (M) ability level.
The Wright map is organized vertically in two parts. The top half of the map shows the distribution of the 555 respondents’ ability estimates. These ability estimates provide a measurement of the respondents’ quantitative reasoning in a biological context. A vertical line is drawn at the average ability level. A respondent with an ability estimate that falls to the left of this vertical line has lower than average skills in quantitative reasoning in a biological context, while a respondent with an ability estimate to the right of this vertical line has higher than average skills in quantitative reasoning in a biological context. The distribution is relatively symmetric with 72% of the students’ ability estimates within 1 SD of the mean.
The bottom half of the Wright map displays the distribution of item difficulties from least difficult (item 2) to most difficult (item 25). The item difficulty scale is the same scale used for the respondent ability levels. This makes it easier to make statements about students and items. For example, a student of average ability (at the M level in Figure 2) is likely to respond correctly to all the items that are to the left of the vertical line in Figure 2.
An examination of the distribution of difficulty values of items shows that the BioSQuaRE provides measurement across a range of student abilities, although 18 of the 29 items are of less than average difficulty. Parsing this for each of the three primary content areas represented on the BioSQuaRE, we see that there are items in the categories related to algebra or visualization that measure at levels that span the range of student abilities. However, items with statistics and probability content tend to do a better job of measuring students’ reasoning at lower ability levels and may not provide as much information about students of higher ability.
The conversations about how or where in the undergraduate curriculum students develop the skills revealed to be lacking by data accumulated using BioSQuaRE should also consider the following observations. A student of average ability who completes the BioSQuaRE would be expected to respond correctly to items that include content such as computing conditional probabilities from a table (item1), identifying variability given a table of data (item 2), or interpreting relationships between variables from a line plot (item 20; Figure 2). This may reflect the inclusion of this content in recent K–12 science and mathematics standards (e.g., National Council of Teachers of Mathematics, 2000; National Governors Association Center for Best Practices and Council of Chief State School Officers, 2010; NGSS Lead States, 2013). In contrast, only students at higher ability levels would be able to correctly answer questions related to probabilistic interpretation of a p value (item 7), interpreting plots in which the response variable has been transformed using a base-2 logarithm (item 15), plotting a nonlinear function (item 25), and selecting appropriate graphs to answer a research question given the description of a study (item 29; Figure 2). Some of these difficulties have been previously documented in the STEM literature. For example, students’ difficulty with logarithms, primarily in the context of pH, has been described (DePierro et al., 2008; Watters and Watters, 2006), and misconceptions about hypothesis testing are known to be a challenge in statistics education (e.g., Castro Soto et al., 2009).
DISCUSSION
The BioSQuaRE is able to assess quantitative skills in a biological context for students with a wide range of abilities. The instrument was developed with national reports and expert knowledge to inform content and item writing standards to reduce measurement error, and it was refined using data collected across a diverse range of institutions and students. As such, the BioSQuaRE should prove useful to educators and researchers who aim to answer the ongoing calls to improve the quantitative skills of undergraduate biology students.
The process by which we developed the BioSQuaRE provides a model for others hoping to develop similar types of assessment instruments. As a team of faculty and graduate students from departments of biology, chemistry, educational psychology, and mathematics, we brought diverse and multidisciplinary perspectives to bear on the instrument’s design. The inclusion of educational psychologists on the development team in particular provided the expertise needed to frame, analyze, and revise the instrument. Repeated administrations of the BioSQuaRE at multiple liberal arts institutions, interspersed with small semiannual workshops, allowed for efficient evaluation and revision, while late-stage piloting of the BioSQuaRE by three large, graduate degree–granting institutions grew the data set in terms of both size and the diversity of participants, lending more statistical power to the analyses.
The inclusion of items with content related to data visualization stands as a distinguishing feature of the BioSQuaRE. Among the desired competencies listed in the five national reports that served as content guides for the instrument, only four are listed by all five reports: “basic computations,” “using a model to make predictions,” “choosing an appropriate type of graph,” and “interpreting a graph” (Table 1). The fact that two of these four competencies concern data visualization urged us to develop an instrument in which a majority of the items contain content related to data visualization (e.g., the item featured in Figure 1). In the fifth administration, 79% (23/29) of the items contain a graph or a table in either the stem or response choices. In addition to the tool’s broad biological context, this emphasis on data visualization distinguishes the BioSQuaRE from other quantitative skills assessment tools such as the QLRA (Gaze et al., 2014a,b) and TOSLS (Gormally et al., 2012).
In contrast to instruments designed to assess the impact of interventions in a specific course or lab, our hope is that the BioSQuaRE will stimulate curricular conversations at the departmental or interdepartmental level. The focus on quantitative skills that students should possess after they have completed an introductory biology sequence provides flexibility in how the instrument can be used. Administered at the beginning of an introductory sequence, it can help delineate the skills that students already possess. Administered at the end of an introductory sequence, the BioSQuaRE can instead be used to assess learning gains. Given even later in the curriculum, the instrument can be used to assess retention and/or reinforcement of acquired skills. In that the instrument was developed to assess a wide range of quantitative topics and still be completed by a student within 30–40 minutes, feedback of only limited granularity can be provided to individual students. In contrast, because our analysis indicates that the instrument measures a single latent trait—quantitative reasoning in a biological context—the aggregate score report (see Supplement C in the Supplemental Material for an example) provides useful and potentially actionable information. At a departmental level, if BioSQuaRE results identify prospective biology students who arrive at an institution with weak quantitative preparation, conversations can focus on where in the curriculum these students can be expected to strengthen those skills. BioSQuaRE results can also be used for programmatic assessment or to compare the efficacy of different curricula. Oberlin College, for example, is currently using the BioSQuaRE to assess programming offered through their Quantitative Skills Center.
Individuals interested in examining or using BioSQuaRE should complete the Instructor Survey for BioSQuaRE, which can be found at www.macalester.edu/hhmi/biosquare. This survey gathers basic contact information (institutional type, departmental listing, class size, range of students, etc.). Once the survey is completed, directions will be sent, along with a link that will allow instructors to examine the instrument and students to complete the instrument online. We will then provide instructors who use the BioSQuaRE with a report that summarizes the responses of their students (see Supplement D in the Supplemental Material). Please note that these summaries will be aggregated to the course or institution level and will be provided only if the number of students completing the BioSQuaRE is large enough to protect the anonymity of individual students.
FUTURE WORK
Our hope is that BioSQuaRE will continue to be refined and improved. For example, several BioSQuaRE items are relatively easy and measure nearly the same ability level. Removing some items or making other items more difficult may improve the utility of the instrument.
Establishing instrument validity and reliability remains a time-intensive endeavor, and a single study rarely provides all forms of evidence needed to support such claims (Messick, 1995; Reeves and Marbach-Ad, 2016; Campbell and Nehm, 2013). Based on the sources of validity evidence articulated by Campbell and Nehm (2013), Table 5 highlights the methods that have been used, are in progress, or could be used to strengthen the inferences made using the BioSQuaRE instrument. We note that our current effort provides solid evidence of content and internal structure validity. The use of open-ended student responses to develop response choices represents a start toward substantive evidence, but additional evidence through think-alouds or interviews about how students are solving problems would address limitation of instrument-irrelevant responses. Examples of such irrelevant responses could include student guesses or variation in test-taking skills. Similarly, the development and preliminary data gathering at multiple postsecondary institutions serves as a starting point for generalization validity. Gathering responses from students at a greater number and variety of schools (community colleges, comprehensive institutions, etc.) would provide additional insights into the utility and potential limitations of this instrument. Furthermore, a larger and more comprehensive set of data would enable a robust differential item functioning (DIF) analysis. DIF analysis has the potential to enhance instrument fairness and avoid items that measure more than one latent trait (Martinkova et al., 2017). Finally, additional work remains to establish evidence for external structure or consequences validity.
Source of validity evidence | Question addressed | Methods used, in progress, or proposed | ||||||||||||
---|---|---|---|---|---|---|---|---|---|---|---|---|---|---|
Content | Does the assessment appropriately represent the specified knowledge domain, biological science quantitative reasoning? | Used: Alignment of content of the BioSQuaRE to national reports (Table 1); modification of BioSQuaRE test blueprint using expert feedback from 38 faculty members (Supplement A, Tables A1 and A2, in the Supplemental Material) | ||||||||||||
Substantive | Are the thinking processes intended to be used to answer the items the ones that were actually used? |
| ||||||||||||
Internal structure | Do the items capture one latent trait, biological science quantitative reasoning? | Used: Coefficient alpha; Rasch analysis | ||||||||||||
External structure | Does the construct represented in the BioSQuaRE align with expected external patterns of association? | In progress: Longitudinal study examining correlation of the BioSQuaRE scores to strength of biology course work and SAT and/or ACT scores | ||||||||||||
Generalization | Are the scores derived from the BioSQuaRE meaningful across populations and learning contexts? |
| ||||||||||||
Consequences | In what ways might the scores derived from the BioSQuaRE lead to positive or negative consequences? | Proposed: Stimulate curricular conversations, assist in department and/or program evaluation |
Future administration of BioSQuaRE at a diverse set of institutions should assist in furthering the sources of validity evidence and help to establish a robust baseline of student performance, allowing individual biology programs to better gauge the quantitative preparation of their own students. In an effort to understand more about what additional insights BioSQuaRE may provide, we are currently undertaking, with support from the HHMI, a multi-institution longitudinal study seeking to understand the relationship between performance on BioSQuaRE, success in biology course work, and performance on standardized exams such as the SAT and ACT.
ACKNOWLEDGMENTS
This work has been supported in part by a collaborative science education PILOT grant from the HHMI. We thank Stephen Adolph (Stuart Mudd Professor of Biology, Harvey Mudd College), Robert Drewell (associate professor of biology, Clark University), David Hansen (chair of the Department of Chemistry, Amherst College), and Catherine MacFadden (Vivian and D. Kenneth Baker Professor in the Life Sciences, Harvey Mudd College) for their contributions to the early stages of developing the BioSQuaRE. G.K.D. is supported by the National Science Foundation under grant no. IOS-1557678. We also acknowledge the improvements to this article that were made possible by feedback provided by two anonymous external reviewers and the monitoring editor, Ross Nehm.
REFERENCES
ACT (2015). The condition of college & career readiness, Retrieved November 11, 2017, from www.act.org/content/dam/act/unsecured/documents/Condition-of-College-and-Career-Readiness-Report-2015-United -States.pdf. Google Scholar- 2014). Teaching quantitative biology: Goals, assessments, and resources. Molecular Biology of the Cell 25, (22), 3478-3481. Medline, Google Scholar (
American Association for the Advancement of Science (2011). Vision and change in undergraduate biology education: A call to action, Washington, DC. Google ScholarAmerican Educational Research Association, American Psychological Association, National Council on Measurement in Education, and Joint Committee on Standards for Educational and Psychological Testing (2014). Standards for educational and psychological testing, Washington, DC: AERA,. Google ScholarAssociation of American Medical Colleges–Howard Hughes Medical Institute Joint Committee (2009). Scientific foundations for future physicians, Washington, DC: AAMC,. Google Scholar- 2004). Introductory science and mathematics education for 21st-century biologists. Science 303, (5659), 788-790. Medline, Google Scholar (
- 2010). Make math a gateway, not a gatekeeper. Chronicle of Higher Education Retrieved November 11, 2017, from www .chronicle.com/article/Make-Math-a-Gateway-Not-a/65056. Google Scholar (
Business Higher Education Forum (2011). Creating the workforce of the future: The STEM interest and proficiency challenge, Retrieved November 11, 2017, from www.bhef.com/sites/default/files/BHEF_2011_stem_inerest _proficiency.pdf. Google Scholar- 2013). A critical analysis of assessment quality in genomics and bioinformatics education research. CBE—Life Sciences Education 12, 530-541. Link, Google Scholar (
- 2015). A study of student’ readiness to learn calculus. International Journal of Research in Undergraduate Math Education 1, 209-233. Google Scholar (
- 2010). The Precalculus Concept Assessment: A tool for assessing students’ reasoning abilities and understandings. Cognition and Instruction 28, (2), 113-145. Google Scholar (
- 2009). How confident are students in their misconceptions about hypothesis tests?. Journal of Statistics Education 17, (2), Retrieved November 11, 2017, from ww2.amstat.org/publications/jse/v17n2/castrosotos.html. Medline, Google Scholar (
College Board (2012). AP biology quantitative skills: A guide for teachers, New York. Google Scholar- 2007). Assessing student’ conceptual understanding after a first course in statistics. Statistics Education Research Journal 6, (2), 28-58. Google Scholar (
- 2008). Helping students make sense of logarithms and logarithmic relationships. Journal of Chemical Education 85, (9), 1226-1228. Google Scholar (
- 2014). Teaching quantitative reasoning: A better context for algebra. Numeracy 7, (1), Article 1. Google Scholar (
- 2014a). Quantitative Literacy and Reasoning Assessment [Measurement instrument], Retrieved November 11, 2017, from https://serc.carleton .edu/qlra/index.html. Google Scholar (
- 2014b). Towards developing a quantitative literacy/reasoning assessment instrument. Numeracy 7, (2), Article 4. Google Scholar (
- 2012). Developing a Test of Scientific Literacy Skills (TOSLS): Measuring undergraduates’ evaluation of scientific information and arguments. CBE—Life Science Education 11, (4), 364-377. Link, Google Scholar (
- 2002). A review of multiple-choice item-writing guidelines for classroom assessment. Applied Measurement in Education 15, (3), 309-333. Google Scholar (
- 1999). Cut off criteria for fit indexes in covariance structure analysis: Conventional criteria versus new alternatives. Structural Equation Modeling: A Multidisciplinary Journal 6, (1), 1-55. Google Scholar (
- 2011). Principles and practice of structural equation modeling, New York: Guilford,. Google Scholar (
- 2002). What do infit and outfit, mean-square and standardized mean?. Rasch Measurement Transactions 16, (2), 878. Google Scholar (
- 1996). Power analysis and determination of sample size for covariance structure modeling. Psychological Methods 1, 130-149. Google Scholar (
- 2017). Checking equity: Why differential item functioning analysis should be a routine part of developing conceptual assessments. CBE—Life Sciences Education 16, (2), rm2. Link, Google Scholar (
- 2013). Goodness-of-fit assessment of item response theory models. Measurement: Interdisciplinary Research and Perspectives 11, (3), 71-101. Google Scholar (
- 1995). Validity of psychological assessment: Validation of inferences from persons’ responses and performances as scientific inquiry into score meaning. American Psychologist 50, 741-749. Google Scholar (
National Center for Education Statistics (2015). Bachelor’s degrees conferred by postsecondary institutions, by race/ethnicity and field of study: 2012–13 and 2013–14 [Table]. Digest of Education Statistics Retrieved November 11, 2017, from https://nces.ed.gov/programs/digest/d15/ tables/dt15_322.30.asp. Google ScholarNational Council of Teachers of Mathematics (2000). Principles and standards for school mathematics, Reston, VA. Google ScholarNational Governors Association Center for Best Practices & Council of Chief State School Officers (2010). Common core state standards, Washington, DC. Google ScholarNational Research Council (2003). BIO2010: Transforming undergraduate education for future research biologists, Washington, DC: National Academies Press,. Google ScholarNext Generation Science Standards Lead States (2013). Next generation science standards: For states, by states, Washington, DC: National Academies Press,. Google ScholarPresident’s Council of Advisors on Science and Technology (2012). Engage to excel: Producing one million additional college graduates with degrees in science, technology, engineering, and mathematics, Retrieved November 11, 2017, from www.whitehouse.gov/sites/default/files/microsites/ostp/pcast-engage-to-excel-final_2-25-12.pdf. Google Scholar- 2007). The American freshman: Forty year trends, Los Angeles: Higher Education Research Institute, University of California, Los Angeles,. Google Scholar (
- 2016). Contemporary test validity in theory and practice: A primer for discipline-based educational researchers. CBE—Life Sciences Education 15, (1), rm1. Link, Google Scholar (
- 2006). ltm: An R package for latent variable modeling and item response theory analyses. Journal of Statistical Software 17, (5), 1-25. Google Scholar (
- 2015). Data nuggets: Bringing real data into the classroom to unearth students’ quantitative and inquiry skills. American Biology Teacher 77, (1), 19-29. Google Scholar (
- 2011). Quantitative literacy at Michigan State University, 1: Development and initial evaluation of the assessment. Numeracy 4, (2), Article 5. Google Scholar (
- 2010). 1, 2, 3, 4: Infusing quantitative literacy into introductory biology. CBE—Life Sciences Education 9, (3), 323-332. Link, Google Scholar (
Sundre (2008). The quantitative reasoning test, Version 9: Test manual, Harrisonburg, VA: Center for Assessment and Research Studies,. Google Scholar- 2010). Online interactive teaching modules enhance quantitative proficiency of introductory biology students. CBE—Life Science Education 9, (3), 277-283. Link, Google Scholar (
- 2010). Measurement and evaluation in psychology and education, Boston: Prentice Hall,. Google Scholar (
- 1997). Handbook of modern item response theory, New York: Springer,. Google Scholar (
- 2006). Student understanding of pH: “I don’t know what the log actually is, I only know where the button is on my calculator.”. Biochemistry and Molecular Biology Education 34, (4), 278-284. Medline, Google Scholar (
- 1982). Rating scale analysis, Chicago, IL: MESA Press,. Google Scholar (
- 1981). Using simulation results to choose a latent trait model. Applied Psychological Measurement 5, (2), 245-262. Google Scholar (