A Biology Core Concept Instrument (BCCI) to Teach and Assess Student Conceptual Understanding
Abstract
Instruments for teaching and assessing student understanding of the five core concepts in biology from Visionand Change are needed. We developed four Biology Core Concept Instruments (BCCIs) that teach and assess students’ ability to describe a concept in their own words, identify concepts represented in biological phenomena, and make connections between concepts. The BCCI includes a narrative, followed by a series of 10 true-false/identify (TF/I) and three open-ended questions. The TF/I questions are aligned with Cary and Branchaw’s Conceptual Elements Framework and were iteratively developed with feedback from biology experts and student performance and feedback obtained during think-aloud interviews. A component scoring system was developed to discriminate between a student’s ability to apply and identify each core concept from his or her ability to make connections between concepts. We field-tested the BCCIs (n = 152–191) with students in a first-year course focused on learning the five core concepts in biology and collected evidence of interrater reliability (α = 0.70) and item validity. With component scoring, we identified examples in which students were able to identify concepts singularly, but not make connections between concepts, or were better able to apply concepts to one biological phenomenon than another. Identifying these nuanced differences in learning can guide instruction to improve students’ conceptual understanding.
INTRODUCTION
The development of a framework for learning biology has been proposed by life science educators, who recommend that undergraduate biology education be centered around core concepts to better guide the transition of novice to expert thinking (American Association for the Advancement of Science [AAAS], 2011). These overarching core concepts—evolution (E); information flow, exchange, and storage (IFES); structure and function (S&F); pathways and transformations of energy and matter (PTEM); and systems (S)—span subdisciplines and biological scales. Currently, few instructional and assessment tools exist that engage students in identifying and applying the core concepts in the context of complex biological phenomena (Smith et al., 2013; Summers et al., 2018; Couch et al., 2019). One tool invites participants to sort cards printed with biological questions into categories of the participant’s choosing, and the categories are scored for conceptual understanding (Smith et al., 2013). This card-sorting activity is effective in discriminating novice from expert-level understanding of biological concepts at different subscales and across biological scales, but it cannot easily be administered to an entire class. A second set of tools, the Ecology and Evolution and General Biology Measuring Achievement and Progression in Science (Eco-Evo MAPS, GenBio-MAPS) tools, measure student thinking on foundational concepts in ecology and evolution and general biology, respectively, and are designed to assess student thinking at various time points in the curriculum (Summers et al., 2018; Couch et al., 2019). These tools can be administered broadly and provide inferences on how students gain knowledge about each of the core concepts in biology over the course of their undergraduate degree, but do not assess a student’s ability to make connections within complex biological phenomena. Finally, concept inventories are designed to assess student learning of disciplinary-specific biological concepts (e.g., Anderson et al., 2002; Klymkowsky and Garvin-Doxas, 2008; Smith et al., 2008; Couch et al., 2015), but they do not assess whether students are able to apply concepts to novel biological phenomena or to make connections between concepts. We sought to develop an instrument that could be used in large classes and that would challenge students to identify, apply, and connect their knowledge of multiple core concepts in complex biological phenomena.
At our institution, a first-year seminar course, Exploring Biology, uses the Vision and Change core concepts to introduce biology to incoming students interested in the life sciences (Wienhold and Branchaw, 2018). Exploring Biology is offered before students take an introductory biology course and is designed to help them develop a cognitive framework around the five core concepts into which they can situate prior knowledge and incorporate new knowledge. Developing an understanding of the five core concepts is the primary learning goal of the course, but the specific topics and biological phenomena used to teach the concepts vary from semester to semester. Because the focus is on learning about core concepts that can be applied across different phenomena, we sought to develop an instrument to teach and assess students’ core concept knowledge and ability to apply it, regardless of the specific topics and biological phenomena taught each semester.
With this goal in mind, we created an instrument template that could serve as the backbone for generating a variety of topic-specific instruments (Figure 1). The template includes a short narrative to introduce a complex biological phenomenon followed by open-ended questions and a series of true-false/identify (TF/I) questions. The variety of question types challenge students to apply, identify, and connect core concepts within the context of the narrative. True-false questions are generated using the Conceptual Elements (CE) Framework (Cary and Branchaw, 2017), a list of key subconcepts for each core concept, as a guide (Table 1). During development of the CE Framework, we collected evidence of content validity through a national review by more than 60 experts.
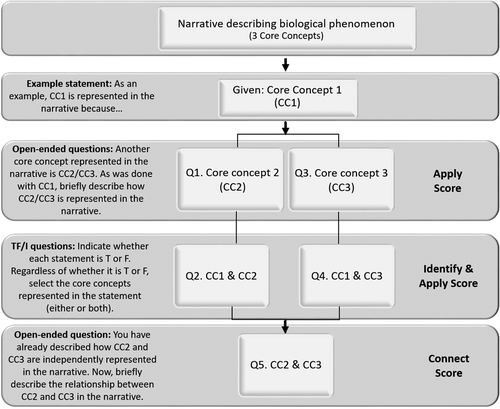
FIGURE 1. Template design, including question type and scoring component(s) (identify, apply, and/or connect score) attributed to each question. Students begin the assessment by reading a narrative that describes a biological phenomenon and is crafted to address three core concepts (CC1, CC2, and CC3). Students then answer a series of questions (1–5) designed to assess their ability to identify, apply, and connect core concepts.
![]() |
The template provides the necessary components and structure to measure student understanding of each core concept with the expressed goal of assessing differential understanding (i.e., do students grasp certain concepts over others?) and whether students can make connections between concepts. The advantage of this template is the ability to create unlimited, customized narratives and associated questions that target specific core concepts/subconcepts and connections of interest to the instructor and/or researcher. Because all Biology Core Concept Instruments (BCCIs) are built using the CE Framework, one could assess student understanding of a particular concept or conceptual element by comparing student performance on different narratives that address the same concepts/subconcepts. In this way, the template provides a structure to develop and assess students’ ability to transfer their core concept knowledge to new biological phenomena as they progress through a single course or through multiple courses in a degree program. Furthermore, with this template, one can parse out different ways of knowing and compute a component score that discriminates between a student’s ability to apply and identify each core concept from his or her ability to make connections between concepts.
In this article, we describe the development and use of the template and four separate BCCIs, each centered around a different biological phenomenon, or narrative (antibiotic resistance [AR]; evolution of beak shape in Galápagos finches [GF]; recombinant humulin [RH]; and sloth, moth, and algae symbiosis [SMAS]). We present evidence of validity and reliability for the BCCIs through iterative expert review, student think-aloud interviews, and field-testing with students in Exploring Biology. The BCCIs are versatile and can be used as formative learning tools and summative assessments within a classroom. However, at this stage in BCCI development, greater evidence of reliability should be gathered before the BCCIs are used as research tools. We invite those interested in collaborating on this data collection to contact the authors.
PHASE 1: BCCI TEMPLATE DEVELOPMENT AND COMPONENT SCORING
Our primary goal in developing the BCCIs was to assess student ability to identify, apply, and make connections between concepts in authentic, biological phenomena. Because the Vision and Change core concepts are broad, we needed to define a specific biological scenario that included the concepts of interest to focus student thinking. We developed criteria to guide narrative development to avoid inadvertently assessing reading proficiency and/or disciplinary mastery of content, including disciplinary-specific jargon. Narratives must:
describe a biological phenomenon that includes biological details representing multiple concepts and Conceptual Elements (Cary and Branchaw, 2017),
be easily interpretable at an introductory biology level (i.e., be a topic likely covered in introductory biology courses),
minimize use of scientific jargon, and
be short in length (∼350 words).
Also, because we did not want to assess student ability to define the Vision and Change core concepts as titled, a general definition for each core concept was provided to students for reference while completing the instrument (see Supplemental Material for definitions).
The first iteration of the template gave students a narrative about a biological phenomenon and an example of how the first core concept (CC1) was represented in the narrative and asked them to identify and describe two additional core concepts (CC2 and CC3) de novo. To make the instrument useful in large classes, we balanced the burden of scoring an instrument consisting of all open-ended questions by adding constrained-choice follow-up questions that could be graded efficiently. Initially, we used one multiple-choice (MC) question asking students to identify two of four statements that correctly described how their chosen second core concept (CC2) interacted with the first core concept (CC1). With this question type, we were trying to assess a student’s content knowledge and, simultaneously, his or her ability to make connections between concepts. We pilot tested this version with students in the Exploring Biology first-year seminar course (Wienhold and Branchaw, 2018) to gather student feedback that would guide template revision (n = 148). Students completed the instrument and provided feedback as an out-of-class, extra-credit assignment using an online platform (Qualtrics Survey software). If students did not answer all of the questions, took less than 10 minutes to complete the instrument, or submitted nonsensical responses to the open-ended questions, we removed their answers from the data analysis.
The pilot test revealed that, when given definitions, 98% of students identified at least one of the two most prominent core concepts in the narrative, and the majority of students (56%) were able to identify both concepts. Students who chose less obvious concepts typically struggled to formulate an appropriate response, such that we could not properly assess their ability to describe how a concept related to the phenomenon. We realized that directing students to apply specific concepts to the narratives (i.e., “How is concept X represented in the narrative?”) would improve our ability to assess their thinking as they made connections between concepts in follow-up questions. Also, constraining the concepts addressed in each open-ended question minimized variation in student responses, allowing us to develop a standard rubric for scoring (see Scoring Open-Ended Questions) and eliminating the need to develop probable MC questions for core concepts that were not well represented in the narrative (a task that proved challenging). Additionally, scores from the MC question format did not discriminate accurately between levels of student understanding (i.e., student scores were not measurably different from one another) and led us to abandon this question type.
A variety of other constrained-choice question types (e.g., two-part MC, true-false) with both students and experts were tested. Individual true-false (TF) statements coupled with a concept-identification (I) question format proved most useful. This construction allowed us to assess student ability to identify single or multiple concepts referred to in the TF/I statement, as well as students’ ability to apply their knowledge of the concept(s). Each TF/I statement could be linked to one or two core concepts and therefore defined as a “single-concept” question or a “dual-concept” question, respectively. Furthermore, the TF/I statements could be generated using the CE Framework (Cary and Branchaw, 2017) and mapped to individual CE.
Finally, we asked students to reflect on the instrument language and the utility of the narrative when answering questions. The majority of students found the narrative (87%) and the question prompts (96%) easy or fairly easy to follow, and 74% of the students stated that the narrative was very helpful. When asked to disagree or agree with the following statement: “I feel the questions about [narrative topic] and the 5 core concepts accurately tested my understanding of how to apply the 5 core concepts to biological phenomena,” 96% agreed or strongly agreed.
The final template is presented in Figure 1. In summary, students are asked to read a narrative describing a biological phenomenon and are given a brief description of how one core concept (CC1) is represented in the narrative. Following this example, they are prompted to generate their own description for a second concept (CC2) in an open-ended question. Five subsequent TF statements probe student knowledge and application of CC1 and CC2 in the narrative and require students to identify (I) which of these concepts (CC1, CC2, or both) are represented in each statement. This block of questioning (one open-ended question followed by five TF/I statements) is repeated with a third concept (CC3). The final open-ended question asks students to make a connection between the two concepts they have already described (CC2 and CC3). In this way, students advance through a series of questions challenging them to first describe a single concept as it relates to the narrative, then identify how more than one concept can be described jointly in the narrative, and finally generate their own connection between two concepts.
BCCIs
We have developed four BCCIs, each describing a unique biological phenomenon: antibiotic resistance (AR); evolution of beak shape in Galápagos finches (GF); recombinant humulin (RH); and a symbiotic relationship between a sloth, moth, and algae (SMAS). Each BCCI narrative addresses three core concepts and includes three associated open-ended and 10 TF/I questions. A comprehensive table of specifications (Table 1) identifies the core concepts and subconcepts addressed in each narrative, including the number of questions that address each concept and subconcept. Please see the Supplemental Material for instructor-ready packets for each BCCI, which include the student version of the final BCCI narrative, associated questions, scoring key, and a narrative-specific table of specifications.
Component Scoring
With this template, we can assess a student’s ability to identify and apply each individual concept separately from his or her ability to connect two concepts, resulting in three component scores for each narrative: an apply score, an identify score, and a connect score. The apply and identify scores are summed to determine a total Concept score that can be used to compare student performance on each core concept across different biological scenarios. Referring to Figure 1, a student’s component scores are determined as follows:
Identify Score: Each block of five TF/I questions (Figure 1, Q1/Q3), consists of three questions written so that both concepts are identifiable in the question (i.e., “dual” questions for which students should identify both concepts; e.g., CC1 and CC2) and two questions that address only one of the two concepts (e.g., either CC1 or CC2). A student’s ability to correctly identify whether a concept was represented or not in the TF statement determines his or her identify score.
Apply Score: The first two open-ended questions (Figure 1, Q2/Q4) ask students to describe how a single core concept is represented in the narrative and are scored as “apply” questions. A student’s ability to correctly apply his or her knowledge to answer the TF/I statements as “true” or “false” is then added to his or her performance on the single-concept open-ended questions to determine the apply score.
Connect Score: The final open-ended question (Figure 1, Q5) asks the student to connect two core concepts and therefore infers his or her ability to connect two concepts and constitutes the connect score.
We used the CE to guide the generation of questions that target specific subconcepts within the standard TF/I block format (i.e., three dual-concept questions, two single-concept questions).
Scoring Open-Ended Questions
We developed a rubric using the CE to score the open-ended questions (see scoring keys in the Supplemental Material), which minimized scoring subjectivity and increased scoring efficiency. Recall that the first two open-ended questions ask students, “How is concept X represented in the narrative?” For example, following the narrative about antibiotic resistance, students were asked to describe how SF is represented in the narrative. Our rubric for this question contains five elements of SF based on the CE Framework (e.g., SF1: structure is determined by chemical and physical properties, which enables and constrains function). If a student’s response described this element—or any other single element—correctly in the context of the narrative, the student was awarded 2 points. More sophisticated answers often described up to three elements and were awarded an additional 1 point for each additional element (two elements = 3 points, three elements = 4 points). We chose to give the first element more weight and to “cap” a student’s score after three elements had been described, because the question prompt did not ask students to exhaustively describe how a concept was represented in the narrative. Although it is likely that some students felt they answered the question sufficiently after addressing one element, our pilot testing indicated that an average biology student would include approximately two elements when describing the core concepts. Importantly, not all core concepts are characterized with the same number of elements in the CE Framework, nor are all elements appropriate for describing all biological phenomena; defining a sophisticated answer as including three elements satisfies these concerns while providing a mechanism to award additional points for responses beyond a baseline acceptable answer.
The final open-ended question asks students to describe how CC2 and CC3 are connected in the narrative. Because this question was included to assess student ability to make connections between concepts, the rubric reflects this and weights the connection portion of the answer more heavily (2 points) than the description of each individual concept (1 point each).
All open-ended questions were evaluated for quality of response. Students were awarded an additional 1 point if their responses were clear, cohesive, and included appropriate language. Student responses needed to address how the core concept was represented in the specific narrative using language that was appropriate to the concept and demonstrating their understanding of the concept. Student responses were not evaluated for grammar unless egregious errors made it impossible to understand a statement. Responses were not awarded quality points if students simply rephrased the core concept definition without applying it to the biological scenario in the specific narrative or if responses highlighted a misconception about the concept. The sample student responses below demonstrate how quality points were awarded:
▪ Sample 1: Antibiotic Resistance (SF)
“The alanine residue is changed to a lactate residue in the cell walls of the antibiotic resistant bacteria. This allows the cell walls of the bacteria to stay cross-linked retaining the structure of the cell walls. The bacteria is then able to grow without being inhibited by the antibiotic.”—awarded quality points
“The structure of the cell wall changed, so the antibiotic could not bind to it, causing the bacterial cell wall to form normal crosslinks.”—awarded quality points
“Structure and Function is represented in antibiotic resistance because it describes the relationship of how biological entities influence each other by physical and chemical means.”—not awarded quality points
▪ Sample 2: Galápagos Finches (E)
“Evolution is represented in the Galapagos finch in the way the finch with the large beaks, which were essential for survival, lived through the shortage of soft seeds and were able to reproduce and pass along their traits to future generations. Meanwhile the finch with small beaks died off as a result of not getting the proper nutrition. In the end the population shifted to having more large beak finches because they were the ones who were able to reproduce.”—awarded quality points
“Evolution is represented in the Galapagos finch narrative because as environmental conditions changed the food supply altered between large hard seeds or smaller softer seeds. These external conditions caused the birds to adapt and therefore evolve in order to survive.”—not awarded quality points
PHASE 2: TF/I QUESTION DEVELOPMENT AND EVIDENCE OF VALIDITY
Methods
Development and Revision.
We employed an iterative process of development and revision to create the TF/I questions, which included ongoing revisions of the narratives (Cresswell and Cresswell, 2018; Figure 2). We initially referenced concept inventories (e.g., Anderson et al., 2002; Klymkowsky and Garvin-Doxas, 2008; Smith et al., 2008; Couch et al., 2015), common student misconceptions (e.g., Michael, 1998; Tanner and Allen, 2005; Wilson et al., 2006; Nehm and Reilly, 2007; D’Avanzo 2008; Heitz et al., 2010), and textbooks (Freeman et al., 2002; Reece et al., 2011; Brooker et al., 2014) to draft the TF/I questions. These references guided the content knowledge probed by each question and are reflected in the CE (Cary and Branchaw, 2017). Because development of the BCCI was ongoing, early draft TF/I questions for each narrative did not follow the five TF/I block format as described in the Component Scoring section. The AR and GF narratives had six, rather than five, TF/I questions per block during testing.

FIGURE 2. The iterative revision process illustrating the timeline and how the TF/I questions were developed and validated.
Evidence of Validity.
We collected evidence of construct validity for the TF/I statements using multiple approaches (Messick 1995; American Educational Research Association, 1999; Campbell and Nehm, 2013; Reeves and Marbach-Ad, 2016). We examined content validity, or the extent to which an instrument represents the breadth of a given construct, in two ways: 1) by using the expert-reviewed CE Framework (Cary and Branchaw, 2017) to guide the generation of TF/I statements; and 2) by having experts review each question for biological accuracy. The CE Framework was nationally reviewed by biology experts and > 92% of reviewers agreed that the framework was ready for use by the scientific community, as determined by its scientific accuracy and completeness (Cary and Branchaw, 2017). We collected evidence of substantive validity, or to what extent the instrument reflects the cognitive processes used to answer the items, through think-aloud interviews in which students completed the BCCIs and a series of other tasks related to their conceptual knowledge of biology (Messick, 1995). This allowed us to determine whether students were interpreting the questions as intended and whether their answers (T or F, core concept identification) aligned with their understanding of biology. We examined internal structure validity, or to what extent individual items align with one another, using linear regression analyses and employed an external measure to collect evidence of external structure validity. External structure validity looks at how well an instrument aligns with another measurement based on the expected relationship between the intended construct and the measures (Messick, 1995). We compared student performance on the BCCI with performance on a card-sorting activity also hypothesized to measure a student’s ability to apply conceptual thinking to biological scenarios (modified from Smith et al., 2013). For both internal and external structure validity, a stronger correlation suggests that the items or measures being compared are measuring the same thing.
Once the TF/I statements were developed, their accuracy was reviewed by local experts for content validity, and statements were revised based on feedback. Further evidence of content validity was examined by having experts (n = 5) complete the questions (both TF/I and concept identification) individually, playing the role of student, and then discussing commonalities and differences among answers. This iterative process resulted in revisions of all of the TF/I questions before engaging students in cognitive interviews and field-testing of the instruments. When collecting evidence of content validity, it is important to demonstrate that the instrument comprehensively covers the intended breadth of knowledge (Messick, 1995). To demonstrate the breadth of biology content addressed by the BCCI questions, we report a comprehensive table of specifications (Table 1) that characterizes each question by core concepts and CE.
Students were invited to complete think-aloud interviews to validate the inferences we could make from student responses, as a measure of substantive validity (Collins, 2003; Willis 1999, 2005). Sophomore students who had completed or were near completing their second semester of introductory biology engaged in a 60–75-minute think-aloud interview, which encouraged students to “think aloud,” or verbalize, their thought processes as they answered each question (Redline et al., 2001). The interviews allowed us to determine whether students engaged in critical analysis of the concepts themselves to identify and apply their knowledge or used test-taking strategies to answer the questions. Students self-reported demographic information. The interview population (n = 21) was 62% female (38% male), 86% majority white (14% underrepresented minority), 76% continuing-generation (24% first-generation) students with reported grades in introductory biology ranging from “B” to “A” letter grades. Each interview was video-recorded with student consent, and students were told that they could choose to end the interview at any time. Participants were incentivized with Amazon gift cards ($10/hour; University of Wisconsin–Madison IRB protocol #2014-4034).
During the interview, students answered the TF/I questions in writing, then the interviewer (T.L.C.) used an “active intervention” approach (Dumas and Redish, 1999) to prompt students to verbalize their thoughts, using phrases like “Why do you think that?” or “Can you explain your answer to this question?” but did not provide instruction or lead students to specific answers. Each narrative and associated questions were completed by 5–10 students during individual think-aloud interviews (AR = 6 students; GF = 5 students; RH and SMAS = 10 students; the same students completed both narratives; however, students were randomly chosen to receive RH or SMAS first). Student interviews were recorded and played back for analysis. Student responses to TF/I statements were scored for accuracy of their written answers on the instrument and how well their verbal explanations aligned with their written answers, including how they identified and applied the core concepts. The highest alignment score (3 points) was awarded to responses that were both correct and aligned, while the lowest alignment score (0 points) was awarded to aligned incorrect responses (both written and verbal understanding was incorrect). Misaligned responses (either correct written answer with incorrect verbal understanding or incorrect written answer with correct verbal understanding) were awarded an intermediate score (1.5 points). We chose not to differentiate between the types of misaligned responses, as both equally reflected an unclear question for students and could necessitate question revision. Scoring student TF/I statements in this way allowed us to investigate substantive validity by comparing the alignment score against the student’s written TF/I performance. Additionally, misalignment of student written and verbal responses identified TF/I statements that were not written clearly enough to capture student understanding and informed question revision.
In addition, the think-aloud interviews were designed to provide evidence of external structure validity by incorporating two sets of card-sorting activities. Students were given 20 individual cards containing one question related to a biological concept; all questions in card sort 2 were unique from card sort 1 (modified from Smith et al., 2013). Rather than answer the questions, students were instructed to organize the cards in any manner that seemed appropriate to them. They were told that there was no correct or incorrect way to organize the cards, and they were given as much time as needed to complete the activity. Each card could be sorted in either a “surface” or “conceptual” manner. For example, four of the cards could be grouped together because they all asked questions about insects (surface sort) or because they all asked questions about energy processing in living organisms (conceptual sort); see Smith et al. (2013) for further description of the card-sort construction. Students conducted one card sort before taking the BCCI (card sort 1) and one after completion of the BCCI (card sort 2). Before and immediately after completing the BCCI, students completed a distraction task, an image-sorting activity, to discourage them from thoughtlessly applying BCCI language to the second card sort. If we were properly assessing student ability to think conceptually with the BCCI, we would expect that students who sorted the cards in a conceptual manner in card sort 1 would also perform better on the BCCI. Therefore, a positive correlation in student performance between the two measures would provide evidence of external structure validity.
All statistical analyses were performed in R v. 3.4.2, and statistical significance was determined for alpha values ≤0.05. Adjusted R2 values are reported for linear regressions associated with think-aloud data due to the small sample sizes. A paired t test was conducted to compare the percentage of conceptual card sorts between a naïve sort and the second card sort.
Results and Discussion
Student responses during the think-aloud interviews allowed us to examine substantive validity of the BCCI and determine whether any questions should be revised or removed from future BCCI iterations. The alignment score measured a student’s ability to understand the questions being asked, answer accurately in writing, and provide a verbal explanation that aligned with his or her understanding. There was a significant positive correlation between the written answers on the TF/I questions and a student’s alignment score for all narratives, except the GF narrative (AR: R2 = 0.965; F(1, 4) = 140.2, p < 0.001); GF: R2 = 0.602; F(1, 3) = 7.1, p = 0.078); RH: R2 = 0.633; F(1, 8) = 16.5, p = 0.004; SMAS: R2 = 0.795; F(1, 8) = 140.2, p < 0.001). This indicated that, for the AR, RH, and SMAS narratives, a student’s written answer reflected his or her understanding of what the question was asking and ability to accurately answer the question (Figure 3), as opposed to using test-taking strategies that circumvent the analytical processing of the concepts in question (e.g., guessing or using key words out of context). In other words, higher performance on the BCCI was positively correlated with increased student understanding as assessed by alignment of their written and verbal answers.
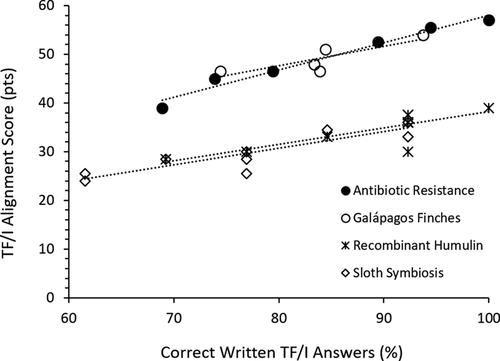
FIGURE 3. Students were asked to explain their reasoning out loud while answering the TF/I questions of each BCCI. Verbal answers were compared with written answers, and an alignment score was assigned for each question. The total summed alignment score for all TF/I questions was correlated with the actual percentage of correct written answers to the TF/I questions. A positive correlation existed for the AR, RH, and SMA narratives (p ≤ 0.004).
Comparing student written answers to verbal responses on individual questions gave us information about how to clarify or remove items that yielded misaligned answers. Also, for all think-aloud exercises, we tested more TF/I questions than necessary in order to reject poorly constructed questions. As a result, one AR question with poor alignment was removed from future iterations, while all other AR questions resulted in ≥83% congruency. All GF questions resulted in ≥80% congruency, but one question yielded no correct responses and was removed from future iterations. The RH narrative resulted in all but one question with ≥90% congruency. We reworded the question with the lowest congruency (70%) to improve clarity, and removed one question to conform to the TF/I block format. All SMAS narrative questions had ≥80% congruency; however, to improve clarity, we revised the wording in three questions and removed one question to conform to the TF/I block format. The high level of congruency in student written and verbal responses led us to conclude that these questions were interpreted appropriately by students.
During the think-aloud interviews, we administered a card-sorting activity before the BCCI (card sort 1). We predicted that, if a student naïvely sorted the cards in a conceptual manner, they would also perform better on the BCCI. This was true for the AR, RH, and SMAS narratives, in which, student performance on the BCCI was positively correlated with the percent of conceptual card sorts. However, the regression analysis was only significant for the SMAS narrative (Figure 4d; adjusted R2 = 0.332; F(1, 8) = 5.46, p = 0.048), not for the AR and RH narratives, in which only a small percentage of the variation in student performance was explained by ability to naïvely sort the cards in a conceptual manner (Figure 4, a and c; AR: adjusted R2 = −0.070; F(1, 4) = 0.675, p = 0.458; RH: adjusted R2 = 0.047; F(1, 8) = 1.44, p = 0.265).
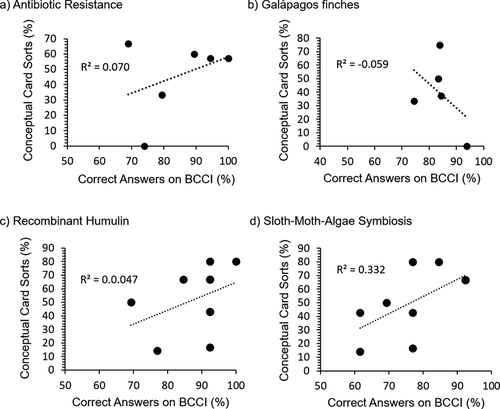
FIGURE 4. The percent card sorts during card sort 1 determined to be conceptually based correlated to the percent BCCI written correct answers for each student (based on TF/I statements). Data are presented for four different iterations of the BCCI: (a) antibiotic resistance (AR), (b) Galápagos finches (GF), (c) recombinant humulin (RH), and (d) sloth, moth, and algae symbiosis (SMA). A positive correlation existed for the students who completed the AR, RH, and SMA iterations, but not for the GF iteration; and regression analysis only indicated a significant relationship for the SMA narrative (p = 0.048). Adjusted R2 values are presented on each graph, along with the linear trend line.
For the fourth narrative (GF), we found a negative correlation between BCCI performance and the conceptual card sort, but the regression analysis determined that only a small percentage of the variation in student performance was explained by the percentage of conceptual card sorts (Figure 4b; adjusted R2 = −0.059; F(1, 3) = 0.776, p = 0.443). Notably, one student who completed the GF narrative performed well on the BCCI, but did not sort any cards in a conceptual manner. This had an impact on the statistical analysis, given the relatively small number of students interviewed. Upon further investigation, we learned that the introductory biology curriculum, which all of our interview students had completed, introduced students to Galápagos finches, but not the biological phenomena in the other three narratives This suggested that the student’s understanding of the GF narrative was enhanced by prior knowledge and that his/her ability to answer the GF questions from memory, rather than from a conceptual understanding of the narrative, likely influenced the results.
Overall, these findings provide evidence of external structure validity, but larger sample sizes and additional think-aloud interviews with students who have not yet been exposed to the narrative topics are needed. Further evidence of validity is presented in Phase 3: Field-Testing of the BCCI and Component Scoring, in which student performance on the BCCI is compared with their performances on a standard exam and their course grades.
To explore use of the BCCIs as learning tools, we compared the percentage of student card sorts that were conceptual before and after administration of the BCCI (naïve card sort 1 compared with card sort 2). On average, students increased their percent of conceptual card sorts after completing any of the BCCI versions (t(19) = −6.91, p < 0.001; Figure 5), indicating they were better able to think conceptually after being primed with the BCCI. But, we did not control for a test–retest effect (i.e., test student card sort performance without the BCCI intervention), so we are unable to conclude with certainty that this finding is due to student exposure to the BCCI rather than increased student familiarity with the activity. However, students did not use the terminology of Vision and Change in card sort 2 (except evolution); rather, they used their own words to convey a concept (e.g., metabolism, homeostasis, relationships between species, transfer of genetic information, environment–organism interactions), suggesting they were not simply mimicking what they had been shown in the BCCI. The increase in the number of conceptual sorts combined with the generation of novel language by students to describe the concepts after completing the BCCI provides evidence that the instrument has value as a learning tool.
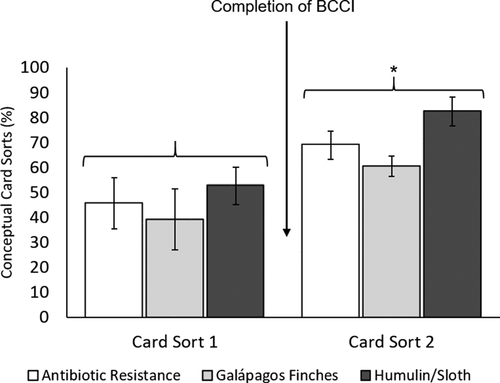
FIGURE 5. Evidence that BCCI completion increases “conceptual” card sorting. The percent of card sorts that were categorized as “conceptual” versus “surface” for both card sort 1 and card sort 2. Card sort 2 was completed following the completion of the BCCI. After completing the BCCI, students significantly increased their use of conceptually based sorts to organize biological information (p < 0.001). Trials 1–3 represent three different groups of students completing at least one of the four narratives (trial 1 = AR, trial 2 = GF, trial 3 = RH and SMA).
PHASE 3: FIELD-TESTING OF THE BCCI AND COMPONENT SCORING
Methods
We field-tested the BCCIs in the first-year seminar Exploring Biology course to measure item difficulty, discrimination, test the utility of component scoring, and collect further evidence of validity and reliability. We administered the AR and GF BCCIs electronically through Moodle, a learning management system. To encourage maximal effort from students, we did not assign a grade for the BCCIs, but students (n = 152) were given course points for thoughtful completion (i.e., providing written answers with reasonable responses and completing all TF statements). This resulted in thoughtful completion by 97% of the students who participated, which was 80% of students enrolled in the course. Scoring of TF/I questions was done automatically in the Moodle software; the open-ended responses were scored by the author (T.L.C.) and four trained raters.
The RH and SMAS narratives were administered in hard copy as part of the course final exam during class time (n = 191; 100% student completion). The completed BCCIs were distributed to multiple graders for scoring efficiency. Scoring of the open-ended responses was done by the authors (T.L.C., C.J.W., J.B.) and two additional, trained graders (n = 5). For consistency, all six open-ended responses for each student were scored by a single grader, with consultation among graders as needed.
Statistical Analyses.
To determine whether a student’s ability to identify a core concept was influenced by whether or not the TF statement was true or false, we compared student’s “identify” performance on true versus false statements using Student’s t tests. We further analyzed the TF/I statements for item statistics. Both item difficulty and item discrimination were calculated to identify questions that should be re-evaluated for efficacy in differentiating student learning. The item difficulty index (P) represents the percentage of students who correctly answered each question, with a lower P value corresponding to more difficult questions (Wood, 1960; Doran, 1980; Crocker and Algina, 1986). The item discrimination index (D) represents how well a specific question differentiates between the top-performing students (top 33% of total scores on BCCI) and the bottom-performing students (bottom 33% of total scores on BCCI). We used the following formula to calculate D for individual TF/I questions: D = (NH – NL)/(N/3), where NH is the number of correct responses by the top 33% of students, NL is the number of correct responses by the bottom 33% of students, and N is the total number of student responses (Doran, 1980).
We employed psychometric measures to further examine validity and reliability of the BCCIs. To collect evidence of internal structure validity, we performed linear regression analyses to compare student performance on portions of the BCCI that we hypothesize are measuring similar constructs of student conceptual understanding. We calculated Pearson’s correlations coefficients to determine the strength of the relationship between: 1) CC1 identification in TF/I question blocks 1 and 2; 2) student ability to apply CC2 in TF format with their performance on the CC2 open-ended question (and repeated this for CC3); and 3) the combined student performance on both CC2 and CC3 open-ended questions with their performance on the final open-ended question that asks students to connect CC2 and CC3 in their own words. To ensure open-ended question scoring was consistent, we calculated interrater reliability using one-way random, intraclass correlation (ICC) for the AR and GF BCCIs (Shrout and Fleiss, 1979; Landers, 2015). Because only one rater graded each open-ended question for the in-class final exam, we were unable to collect evidence of interrater reliability for the RH and SMAS BCCIs. All statistical analyses were conducted using SPSS v. 24, and statistical significance was determined for alpha values ≤0.05.
Field-Testing of Component Scoring.
To test the robustness of the component scoring, we applied it to student performance on the RH and SMAS narratives. For an example of how component scoring is applied to the TF/I questions, see Figure 6. The first, third, and fifth TF/I questions address PTEM (CC1) and systems (S; CC3) and are therefore considered “dual-concept” questions. These questions were scored 1 point for accuracy (i.e., Did the student choose the correct answer, T or F?) and whether the student identified both concepts represented in the TF/I statement (1 point for each concept = 2 points). Overall, each TF/I question was worth 3 points. Performance on these questions was included in the apply and identify scores for both PTEM and systems (S), because the student should recognize that both PTEM and systems (S) are represented in the statement. Additionally, student performance on the TF/I statement and whether they correctly identified PTEM would be analyzed and grouped with student performance on other questions addressing PTEM to formulate a concept score for PTEM; the same would be true for systems (S) to formulate a systems (S) concept score. In contrast, a question addressing only PTEM or systems (S) would be classified as a “single-concept” question, and student performance on the TF/I statement would be split between PTEM and systems (S) appropriately. Student performance on any concept from both single- and dual-concept questions can be compared with performance on all other concepts in the instrument. In this way, the component scoring provides a detailed diagnosis of the degree to which students understand individual concepts and whether students are able to make connections between concepts.
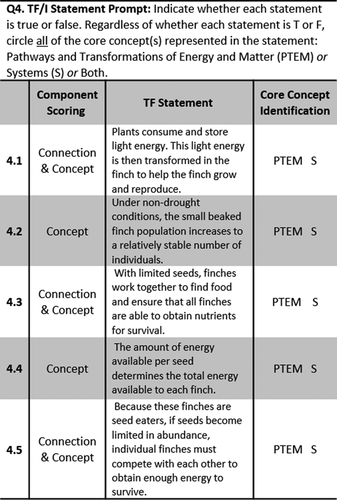
FIGURE 6. Component scoring example. In the Galápagos finches narrative, CC1 is pathways and transformations of energy and matter (PTEM), CC2 is evolution (E), and CC3 is systems (S). The CC2 and CC3 questions are presented to illustrate how each question is profiled to contribute to the concept and/or the connect score.
Results and Discussion
The ability of students to identify core concepts in the TF/I questions was not influenced by whether or not the TF statement was true or false (for all BCCIs: p values ranged from 0.12 to 0.75). Therefore, students were able to independently apply their knowledge and identify core concepts in the TF/I questions. This finding supports our TF/I question format as a plausible way to independently measure a student’s ability to apply knowledge to a TF statement and to identify core concepts.
Difficulty and Discrimination of TF/I Questions.
We calculated TF/I question difficulty and discrimination for all four BCCIs. The item difficulty index (P) and the item discrimination index (D) helped us determine which questions challenged students and/or appropriately discriminated top-performing students from bottom-performing students and, ultimately, directed our decisions regarding question revision.
Together, the AR and GF BCCIs comprehensively address all five core concepts. Because the TF/I questions include a standard TF statement plus an associated concept-identification question, we could analyze the student responses in three ways: 1) the entire question (all-or-none, TF/I), 2) only TF statement, or 3) only concept identification. The item difficulty for both narrative TF/I questions had large ranges when questions were analyzed as all-or-none (AR: 0.07–0.72; GF: 0.03–0.62; Figure 7, a and b). When questions were analyzed for student performance on either the TF statement alone or the concept identification alone, either the TF or I part of the question challenged students more. For example, in AR question 2, TF statement difficulty index was 0.90, indicating that the statement was easy for most students to answer; however, concept-identification difficulty was only 0.35, indicating that most students struggled to identify the correct concepts in that question. An ideal median range of item difficulty is between 0.5 and 0.6 with a distribution of items ranging from easy to difficult in a normal distribution pattern (Backhoff and Tirado, 1992). An item discrimination index between 0.25 and 0.39 is considered good at discriminating between top- and bottom-performing students, while an index >0.40 is considered excellent for discrimination (Ebel and Frisbie, 1986; Doran, 1980). When analyzed as all-or-none, the AR narrative TF/I questions had 33% excellent and 25% good discrimination (Figure 7c). The TF-only analysis had a similar outcome, while the concept identification alone resulted in 33% good discrimination and no questions with excellent discrimination. The opposite trend was found with the GF narrative TF/I questions. The all-or-none and concept identification–only analyses revealed ≥50% of the questions were either good or excellent discriminators, while the TF-only analysis resulted in fewer good discriminators (25%; Figure 7d). Interestingly, for both BCCI narratives, the questions that probed understanding of evolution (E) had the highest ability to discriminate between strong and weak students (AR: Q6 and Q12; GF: Q3 and Q5).
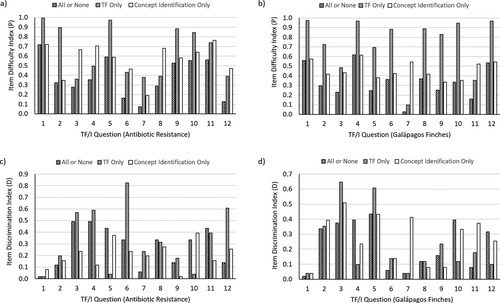
FIGURE 7. Item difficulty index (P) and item discrimination index (D) values for all AR (a,c) and GF (b,d) narrative TF/I questions completed by Exploring Biology students (n = 152) as a preassessment. The p values represent the proportion of correct answers; therefore, lower values indicate more difficult questions. D values represent how well the question discriminates between higher- and lower-performing students; higher values indicate a greater ability to discriminate. Note: These versions of the questions included six, rather than five TF/I questions.
Together, the RH and SMAS BCCIs also comprehensively address all five core concepts. The item difficulty index for the RH narrative TF/I questions ranged from 0.25 to 0.64, while item difficulty of the SMAS narrative TF/I questions was greater (ranging from 0.29 to 0.99) meaning that the SMAS questions were generally easier for students to answer correctly (Figure 8, a and b). As seen with the other BCCIs, typically one part of the TF/I question challenged students more, and in both the RH and SMAS narratives, the concept-identification portion was more difficult to answer correctly than the TF statement in 8/10 questions. When analyzed as all-or-none, the RH narrative TF/I questions had 60% good discrimination (Figure 8c). The TF-only analysis resulted in 30% good and 20% excellent discrimination, while the concept identification alone resulted in 60% good discrimination and one question (10%) with excellent discrimination. A similar trend was found with the SMAS narrative TF/I questions; however, the TF-only and concept-identification portions resulted in lower good discrimination (30% discrimination), and the concept identification had one question with excellent discrimination (10%; Figure 8d).

FIGURE 8. Item difficulty index (P) and item discrimination index (D) values for both RH (a,c) and SMA (b,d) narrative TF/I questions completed by Exploring Biology students (n = 191) as a postassessment. The p values represent the proportion of correct answers; therefore, lower values indicate more difficult questions. D values represent how well the question discriminates between higher- and lower-performing students; higher values indicate a greater ability to discriminate.
Breaking down the different portions of the questions in this way increased our ability to decide which questions should be retained, removed, or revised. For both the AR and GF BCCIs, two questions were chosen for removal, because the final template uses two blocks of five questions each (rather than the two blocks of six questions used in this iteration). We further reworded questions for clarity (three in AR, four in GF) and replaced one question in each narrative to fit the final TF/I five question block format. For the RH narrative, one question was revised to increase difficulty of the TF statement, while one question was removed and replaced. For the SMAS narrative, one question was reworded for clarity. See the Supplemental Material for all final BCCI narratives and associated questions.
Evidence of Validity and Reliability.
The BCCI template requires students to identify CC1 in both blocks of TF/I questions and apply CC2 and CC3 in two question formats: TF statements and open-ended questions. With this item structure, we calculated correlation coefficients for all narratives and collected the following evidence of internal structure validity. Student performance on CC1 identification on TF/I block 1 and TF/I block 2 was significantly correlated for all BCCI narratives (p < 0.05). A student’s ability to apply CC2 in TF format and in an open-ended response was positively correlated for AR and GF (p < 0.01); the same was found for CC3 in RH (p < 0.05) and AR (p < 0.01). Finally, for all BCCI narratives, a student’s ability to apply CC2 and CC3 alone in an open-ended question was positively correlated to his or her ability to connect the two concepts in the final open-ended question (p < 0.01).
In Exploring Biology, students explicitly learned to apply the core concepts in biology to various biological phenomena. Therefore, we expected a relationship to exist between student performance on traditional exam questions and their performance on the BCCI. We analyzed this to collect evidence of external structure validity of the BCCI by comparing student performance on part 1 and part 2 of the course final exam. Part 1 included traditional-style questions (e.g., MC, TF, short answer) designed to assess student understanding of the content explored during the semester, which, as noted earlier, focused on applying the core concepts to various biological phenomena. Part 1 questions included a mix of content-specific questions along with questions asking students to identify and apply their knowledge of the core concepts. Part 2 was the RH and SMAS BCCI narratives. The percent correct for each part of the exam was calculated for each student. Student performance on the traditional portion of the exam was positively correlated with their combined performance on the BCCIs (R2 = 0.293; F(1, 189) = 79.59, p < 0.001; Figure 9). Only 30% of the variance in BCCI scores can be explained by student performance on the traditional portion of the exam, indicating that there are other important factors driving student BCCI performance. We recognize that the traditional-style questions focused on content knowledge more so than the concepts per se; however, because the five core concepts were the content of the course, we concluded that the positive correlation provides further evidence of external structure validity. Additionally, when student course grades in Exploring Biology were compared with their performance on each of the BCCIs, we found a positive correlation with AR and GF performance (Spearman’s rho, p < 0.05), but not with RH and SMAS (p > 0.05).
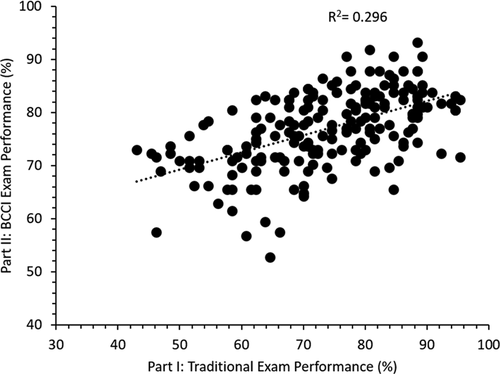
FIGURE 9. Comparison of Exploring Biology student performance on the first and second part of the postassessment. Performance on the BCCI questions was positively correlated with performance on traditional, content-based questions (p < 0.001). The R2 value is presented, along with the linear trend line.
We achieved an acceptable level of interrater reliability for the AR narrative (ICC(1) = 0.70), in that 70% of the variability in rater scoring represented student ability to apply concepts to the biological phenomena (Landis and Koch, 1977; Landers, 2015). However, we failed to obtain evidence of interrater reliability for the GF narrative (ICC(1) = 0.43). A codebook for training raters was used, but it was in development during field-testing and is being revised using student responses from this implementation. We anticipate future implementations and subsequent ratings to achieve higher interrater reliability, because the revised codebook will improve rater calibration.
Component Scoring.
The implementation in Exploring Biology allowed us to test our component scoring method. Because the TF/I questions and template were still in development at the time of field-testing, the component scoring results reflect a nonstandardized TF/I question profile. However, we were still able to determine nuanced differences in conceptual understanding of concepts and discriminate this from student ability to make connections between concepts.
The associated individual concepts and concept connections for each narrative are given in Table 2, along with the average percentage of students who answered each component of the BCCI correctly. Student performance overall (not broken down by components) was 13% higher on the SMAS narrative (86%) compared with the RH narrative (73%).
Apply score | Overall scores | ||||||
---|---|---|---|---|---|---|---|
Core concepts | TF % (possible points) | Open-ended % (possible points) | Apply score % (possible points) | Identify score % (possible points) | Connect score % (possible points) | TF/I score % (possible points) | Total concept score % (possible points) |
RH: 18.3/25 points = 73% | |||||||
CC1: S&F | 76.6 (6) | n/a | 76.6 (6) | 71.6 (10) | 68.0 (18) | 73.5 (16) | |
CC2: IFES | 73.0 (4) | 93.5 (2) | 79.8 (6) | 61.8 (5) | 70.0 (12) | 71.6 (11) | |
CC3: PTEM | 78.8 (4) | 66.3 (2) | 74.8 (6) | 64.2 (5) | 76.2 (12) | 70.0 (11) | |
IFES/PTEM | n/a* | 67.9 (3) | n/a | 67.9 (3) | n/a | ||
SMA: 24.1/28 points = 86% | |||||||
CC1: PTEM | 82.2 (7) | n/a | 82.2 (7) | 83.7 (10) | 80.9 (21) | 83.1 (17) | |
CC2: E | 71.1 (3) | 91.5 (2) | 79.3 (5) | 83.8 (5) | 77.8 (9) | 81.5 (10) | |
CC3: S | 96.6 (4) | 96.9 (2) | 96.7 (6) | 76.2 (5) | 83.0 (12) | 87.4 (11) | |
E/S | n/a | 86.6 (3) | n/a | 86.6 (3) | n/a |
Average performance among individual concepts varied within and between narratives. In the RH narrative, IFES was the most difficult concept for students to identify in TF/I questions (61.8%), but students performed much better describing IFES in the open-ended questions (93.5%), which resulted in a higher apply score for IFES compared with S&F or PTEM. The total concept score for S&F (68.0%), was lower than for either IFES (73.3%) or PTEM (74.8%) in the RH narrative. In the SMAS narrative, students performed very well on individual concepts (>80% concept scores); however, student performance was lowest when asked to apply knowledge of evolution (E) in TF statements, indicating that these questions were most challenging (71.1%). By implementing both the RH and SMA BCCIs, we could identify whether students were better able to describe how a concept was represented in one biological scenario compared with another. In this case, both narratives asked students to consider PTEM. Overall, students earned a higher PTEM concept score with questions related to SMAS than to RH (80.9% compared with 74.8%). In particular, students identified PTEM more readily and better described how PTEM related to the SMAS scenario with their open-ended responses.
A large difference was also found between student ability to apply knowledge to connect two core concepts together in their own words. The average connect score for connecting IFES and PTEM in the RH narrative was 18.7% lower than when students were asked to connect evolution (E) and systems (S) in the SMAS narrative. This finding aligns with the apply and identify scores, in that IFES and PTEM seem to be more challenging concepts for students in general.
In summary, the field-testing provided information regarding item statistics, evidence of validity and reliability, and the utility of component scoring to identify nuanced differences in student thinking. Calculating item difficulty and discrimination for individual questions allowed us to identify TF/I questions that were either unclear, too easy, or did not discriminate well between bottom- and top-performing students. We collected evidence of item validity by calculating correlation coefficients between items purported to measure the same construct; in particular, we found that questions in which students were asked to identify CC1 held together for all BCCI narratives. A positive correlation was found when we compared student performance on both parts of the Exploring Biology final exam. Because both part 1, traditional-style exam questions, and part 2, the RH and SMAS narratives, probed student ability to identify and apply core concepts within the context of biological phenomena, this relationship provides further evidence of external structure validity. Finally, the component scoring system was evaluated to determine whether student thinking surrounding the core concepts could be meaningfully dissected into “apply,” “identify,” and “connect.” We determined that these skill categories are meaningful, and the component scoring allowed us to decipher differential student performance on different core concepts within and across narratives.
LIMITATIONS
We acknowledge that field-testing of the BCCI has been limited to one large, midwestern university, and therefore, we lack evidence of generalizability across different populations of students. This limited administration might result in spurious conclusions due to biased population sampling.
Situational features will likely impact a student’s ability to transfer knowledge across biological phenomena, which generates threats to validity inferences, specifically construct-irrelevant variance, and could possibly lead to inaccurate interpretations of student thinking (Campbell and Nehm, 2013; Reeves and Marbach-Ad, 2016). Though the BCCI template will allow for assessment of a single concept across multiple narratives, our limited sample size did not allow us to investigate the impact that situational features may have on student performance across narratives. Situational features such as the biological scale represented in the narrative and the nature of the living organisms in the narrative (e.g., plant, animal, or human) have been shown to influence student performance (Freidenreich et al., 2011; Schmiemann et al., 2017). Differences in the concepts and CE covered, including the order with which the concepts are introduced, are situational features that need to be considered when interpreting data about a particular concept from different narratives. In the future, studies with larger and more diverse student populations will allow us to investigate the impact of situational features within student groups that are homogeneous as well as across heterogeneous groups with students who have different academic backgrounds, cultural backgrounds, genders, race/ethnicity, and so on.
The think-aloud interview results suggested that BCCI assessments may be most effective at assessing conceptual thinking when they describe a biological phenomenon with which students are not familiar. In particular, one student who had prior knowledge of Galápagos finches showed misalignment of written and card-sorting activity performances, suggesting that prior knowledge may have allowed the student to do well on the assessment without needing to think conceptually. This result occurred with only one student, so it is premature to draw conclusions. However, moving forward, we plan to modify the think-aloud protocol to collect information about students’ prior knowledge. This will allow us to test the impact of prior knowledge on the effectiveness of the BCCIs to measure student thinking.
We were unable to collect evidence of internal structure reliability due to the complexity of the BCCI questions. Complex instruments assessing a variety of conceptual areas often fail to produce high internal reliabilities (Smith et al., 2008). Although we performed exploratory factor analyses for all BCCIs, specifically investigating the TF/I questions, the complexity of the questions resulted in nonsensical groupings. We found that when we eliminated dual-concept questions and further teased out the single-concept questions by the components of “apply” and “identify,” we could achieve convergence on three factors that represented the three core concepts of that BCCI. However, we felt this fragmentation required an artificial stripping down of the questions and did not inform the reliability of the instrument. We performed Kuder-Richardson 20 analyses for questions that we hypothesized were testing the same constructs, but this yielded low α values, which might be a reflection of our small data set and/or the dichotomous nature of the TF/I questions. We considered using item response theory (IRT) models to shed light on the complexity of the TF/I questions. However, because our instrument is not unidimensional, meaning it is designed to measure student thinking on multiple core concepts, a unidimensional IRT model is not appropriate (Ackerman, 1994). A more appropriate model would be a multidimensional IRT. Regardless, our data set is not large enough to run unidimensional or multidimensional IRT analyses (Ackerman, 1994; de Ayala, 1994, 2009). de Ayala (1994) suggested that a 5:1 ratio of individuals to parameters is a common ratio for running IRT. For the BCCI TF/I questions, this would require a sample size greater than 300 individuals (30-item test × 2 response options for each = 60 parameters), while multidimensional IRT would require at least 2000 examinees to obtain satisfactory two-dimensional item parameter estimates (de Ayala, 2009, pp. 42–43). We argue that with more student data and/or with more BCCIs targeting the same constructs (i.e., core concepts and CE), we will better be able to collect evidence of instrument reliability.
VALUE AND VERSATILITY OF THE BCCIs
Developing Students’ Conceptual Frameworks
The BCCIs can be used to support student development of a conceptual framework for organizing and learning biological information (Ausubel, 1960). We know students are able to grasp specific ideas in biology generally, but often struggle to make connections across major concepts (Ambrose et al., 2010) and across biological subdisciplines. Helping students build a framework or mental structure that enhances their ability to transfer knowledge about concepts learned in one course to the same concept in another course can increase their retention of biological knowledge (Larkin et al., 1980; Dufresne et al., 1992; National Research Council, 2003; AAAS, 2011) and their interest and engagement in the topic (Seymour and Hewitt, 1997). After engaging with the BCCIs during the think-aloud interviews, students constructed more-conceptual card sorts and used their own words to convey a concept, suggesting they were not simply mimicking what they had been shown in the BCCI. When asked whether they had ever thought about their understanding of biology in this way before, most students replied negatively, but added that they had enjoyed the “new” way of thinking about biology (T.L.C., personal observation). The BCCI narratives provide concrete examples that give students context for the broad overarching concepts in biology, while the associated questions scaffold student development of a framework for biology information though practicing how to identify, apply, and connect the core concepts.
Diagnosing Conceptual Learning
The BCCI component scoring can be used to diagnose student thinking by dissecting student performance on the different core concepts and skills (apply, identify, connect). Thus, component scoring allows an instructor to determine whether a student has specific areas of strength or weakness for a specific concept or subconcept. For example, a student might be able to identify evolution (E), but not be able to apply his or her knowledge to answer TF statements or connect evolution (E) to another concept in the narrative. Comparing conceptual understanding in various biological scenarios at different biological scales (i.e., narratives written at different scales) can identify whether students are better able to apply concepts at one biological scale compared with another. With this information, educators can pinpoint concepts that are less clear to students and modify instruction to facilitate student learning of that concept. For example, given low student performance on applying and identifying PTEM in the RH narrative versus the SMAS narrative (Table 2), an instructor might develop instructional materials with molecular examples of PTEM to facilitate student understanding of PTEM at the molecular scale.
Guiding Instructional Design
The BCCI template can be used to guide the development of instructional materials and formative assessments that advance a student’s ability to identify, apply, and connect core concepts. The BBCI asks students to use the lens of a core concept to analyze a biological phenomenon or determine how two concepts relate to one another within that phenomenon. Because all BCCIs are built from the same template, this design allows for the creation of unlimited, customized narratives and associated questions that target the specific needs of the instructor. We envision instructors using the template to design class activities in which the narrative could be one of the instructor’s own generation or perhaps drawn from a popular press article. Students would then complete the same open-ended questions prompting them to apply and connect whichever combination of core concepts and CE the instructor chooses to target. TF/I questions could also be generated by the instructor or students could be given the CE Framework and tasked with writing a TF/I question to further advance their learning. Additionally, students can use their performance on various BCCIs to self-assess gaps in their own understanding of biology.
Assessing Learning across Courses and Subdisciplines
The BCCIs are versatile in application and can be used in both small and large classes to serve the needs of instructors and, with further development, researchers. Thoughtfully administering two BCCIs at the same time can support and assess student learning of all five core concepts (e.g., AR and GF together cover all five core concepts). We have described BCCI administration to a freshman seminar course of ∼200 students via both an online software system and in paper format during one class period. On average, first-year students were able to complete two BCCIs in under 45 minutes, regardless of how it was administered. Importantly, online administration allows for automatic scoring of the TF/I questions, which is key for large-enrollment classes. Scoring the open-ended responses is time intensive with a large course enrollment, but the constrained question format and rubric make scoring feasible. Once a scorer was trained, the average time spent scoring a student’s open-ended responses electronically (all six questions per BCCI) was 2.5 minutes. We have scored student responses in paper format as well, and found the time spent per question was slightly longer than that of electronic responses due to interpretation of student handwriting.
The BCCI template serves as a blueprint for developing a suite of narratives across biological subdisciplines. BCCIs created with the template can be developed to track the progression of student thinking from start to finish of an introductory biology sequence and at the academic program or department level. We propose a portfolio of assessment instruments with narratives that span biological subdisciplines (i.e., biochemistry to ecology) could be developed and administered throughout an undergraduate biology curriculum. Student learning of specific concepts (and CE) could be tracked across courses via the component scoring system, allowing an academic program to determine how successfully, and in what courses, particular concepts are learned. Coupling use of BCCI assessments with the BioMAPS instruments (e.g., Summers et al., 2018) and concept inventories would be a particularly powerful strategy to track development of student thinking. Triangulation of learning assessment data from multiple sources like these would provide information about the impact of specific courses or other types of learning experiences (e.g., undergraduate research) on student learning of particular concepts. However, we have yet to collect evidence of generalizability of the BCCIs for students at different time points throughout their biology curriculum, and using the BCCIs for this purpose would require gathering validity evidence to support this application.
FUTURE DIRECTIONS
We are interested in ongoing data collection to gather evidence of validity and reliability, specifically with diverse populations of students at different institutions. Validation is an ongoing process, and although we argue that the BCCIs can be used to make inferences about how students identify, apply, and connect core concepts in biology, we have not gathered evidence of generalizability (e.g., freshmen to seniors, racial/ethnic populations, different types of institutions), nor have we repeatedly tested students with the same version of the BCCI to gather evidence of stability. A collection of BCCIs addressing the same concepts and CE would allow us greater statistical power to examine evidence of reliability. Due to the complex nature of the BCCI, using the test–retest method, in which the BCCI would be administered to the same students over repeat administrations, could also provide a more informative measure of instrument reliability (Couch et al., 2015).
We plan to use the template to develop new BCCIs that assess student thinking across biological scales and subdisciplines. Because the TF/I questions and rubric for grading open-ended questions are grounded in the CE Framework, assessment results from these instruments can be compared and compiled. Specifically, the TF/I block format of “dual-concept” versus “single-concept” questions resulted in TF/I statements that can be easily adapted for use in other narratives that assess the same concepts and connections.
In the long term, we aspire to build an online resource library of BCCIs for which evidence of validity and reliability has been gathered for broad use by the biology education community.
CONCLUSIONS
Though there is evidence that the biological sciences education community has increased use of active learning and conceptual approaches to teaching biology (Freeman et al., 2014; Brancaccio-Taras et al., 2016) and is developing and curating quality resources to support active-learning pedagogies (CourseSource: www.coursesource.org), there has been much less reported on the development and validation of assessment instruments to measure student conceptual learning gains (e.g., Smith et al., 2008; Couch et al., 2015, 2019; Summers et al., 2018). Consequently, our ability to assess the impact of active-learning pedagogies on students’ conceptual learning is limited and remains a challenge for advancing research in biology education. The BCCIs and template presented in this article contribute to our efforts in addressing this challenge.
ACKNOWLEDGMENTS
We thank Rosemary Russ for her guidance developing the think-aloud interview protocol and questions. We thank our expert reviewers for their expertise and feedback, which greatly improved the quality of the TF/I statements. We thank Amanda Butz for her guidance with statistical analyses and interpretation. We offer special thanks to those who offered substantial input and review of the article: Claudia Alvarez-Baron, Amanda Butz, Kyriaki Chatzikyriakidou, Michelle Harris, Jessica Maher, Beth Meyerand, Chris Pfund, Kevin Strang, Jessica TeSlaa, Cara Theisen, and Chris Trimby. This work was funded by an Undergraduate Science Education grant from the Howard Hughes Medical Institute to the University of Wisconsin–Madison (52006959) and by the Wisconsin Institute for Science Education and Community Engagement (WISCIENCE).