Advancing the Guidance Debate: Lessons from Educational Psychology and Implications for Biochemistry Learning
Abstract
Research in science, technology, engineering, and mathematics education supports a shift from traditional lecturing to evidence-based instruction in college courses, yet it is unknown whether particular evidence-based pedagogies are more effective than others for learning outcomes like problem solving. Research supports three distinct pedagogies: worked examples plus practice, productive failure, and guided inquiry. These approaches vary in the nature and timing of guidance, all while engaging the learner in problem solving. Educational psychologists debate their relative effectiveness, but the approaches have not been directly compared. In this study, we investigated the impact of worked examples plus practice, productive failure, and two forms of guided inquiry (unscaffolded and scaffolded guidance) on student learning of a foundational concept in biochemistry. We compared all four pedagogies for basic knowledge performance and near-transfer problem solving, and productive failure and scaffolded guidance for far-transfer problem solving. We showed that 1) the four pedagogies did not differentially impact basic knowledge performance; 2) worked examples plus practice, productive failure, and scaffolded guidance led to greater near-transfer performance compared with unscaffolded guidance; and 3) productive failure and scaffolded guidance did not differentially impact far-transfer performance. These findings offer insights for researchers and college instructors.
INTRODUCTION
Trailblazing work over the last 20 years supports a shift from traditional lecturing to evidence-based pedagogies in college science, technology, engineering, and mathematics (STEM) courses (Knight and Wood, 2005; Haak et al., 2011; Freeman et al., 2014; Deslauriers et al., 2019). For example, discipline-based education research (DBER) has shown that active learning improves performance and reduces the achievement gap for STEM students compared with lecture (Freeman et al., 2011, 2014; Haak et al., 2011). Since prominent studies like these, DBER has focused increasingly on second-generation instructional research, using findings from educational psychology to inform instructional design and testing these designs for certain topics and student populations (Eddy and Hogan, 2014; Freeman et al., 2014). As more STEM instructors join the movement toward evidence-based pedagogy, one enduring question remains: What type of instruction is optimal for student learning?
Instruction should be aligned to desired learning outcomes to optimize student learning in biology. Biology lessons almost always teach basic knowledge, including key terminology, the use of terms in context, and interpretation of common visual representations. Many instructors also aim for students to build procedural and conceptual knowledge, which enables them to explain how facts and terms connect and facilitates principle-based reasoning (Rittle-Johnson and Schneider, 2015; Loibl et al., 2017). This type of learning can be assessed using problems that resemble those used during instruction, referred to as “near-transfer problems” (McDaniel et al., 2018). Finally, some ambitious instructors aim for students to adapt learned concepts to new situations or different types of problems (Loibl et al., 2017). This type of learning can be assessed using problems that appear foreign or different from all previous practice, referred to as “far-transfer problems” (Loibl et al., 2017). Indeed, since Vision and Change, the ability to solve both near- and far-transfer problems has been viewed as a key learning outcome for biology education and, thus, the focus of instructional design (American Association for the Advancement of Science, 2011).
While biology educators agree that instruction should focus on transfer, determining the most effective type of instruction for enhancing transfer is a topic of ongoing debate, particularly in educational psychology (Kirschner et al., 2006; Hmelo-Silver et al., 2007; Sweller et al., 2007; Kapur, 2016). At the heart of this debate is the nature and timing of the guidance provided during instruction (Schwartz and Bransford, 1998; Mayer, 2004; Lazonder and Harmsen, 2016). We define guidance broadly as any form of assistance offered during the learning process that aims to either provoke or provide information concerning the process or content involved (adapted from Lazonder and Harmsen, 2016). Regarding the nature of guidance, researchers debate whether guidance should be highly explicit, such as providing explanations, or less explicit, such as providing prompts (Lazonder and Harmsen, 2016). The timing of guidance is also debated. Some argue that novice learners should be explicitly told concepts and procedures before solving problems independently (Sweller et al., 1998; Sweller, 2016; Paas et al., 2003; Renkl, 2014; Glogger-Frey et al., 2015; Hsu et al., 2015). Others argue that learners should explore problems on their own before being given explicit instructions (Schwartz and Martin, 2004; Schwartz et al., 2011; Kapur, 2008, 2011; Kapur and Bielaczyc, 2012; Kapur and Rummel, 2012; Weaver et al., 2018). Furthermore, some argue that guidance that fades away as knowledge and skills are built should be provided throughout the learning event (Hmelo-Silver et al., 2007). From this debate, three evidence-based pedagogies emerge: worked examples plus practice, productive failure, and guided inquiry. All three pedagogies engage learners in problem solving and share the ultimate goal of enhancing student learning. Notably, none of these pedagogies involve unguided problem-solving practice (Mayer, 2004). Yet the nature and timing of guidance recommended by each approach varies based on the theories in which they are situated (Figure 1). Likewise, each pedagogy is hypothesized to target different levels of transfer (Kapur, 2016).

FIGURE 1. Worked examples plus practice, productive failure, and guided inquiry are three evidence-based pedagogies that vary in the nature of guidance, the timing of guidance, and the targeted learning outcome.
Distinct Pedagogies and Their Theoretical Underpinnings
Worked Examples plus Practice.
In worked examples plus practice (Figure 1), students receive explicit step-by-step explanations on how to solve a problem, usually through an expert solution, and then practice implementing these solution procedures through independent problem solving. According to cognitive load theory, worked examples reduce the amount of cognitive load or mental effort invested in working memory during learning (Sweller et al., 1998; Paas et al., 2003). Cognitive load theory suggests that, when students study worked examples, they can focus their limited working memory on constructing the knowledge needed to solve the problem rather than using cognitive resources to search the problem space for a solution (Kirschner et al., 2006; Sweller, 2016). Studies in support of cognitive load theory demonstrate that students who learn using worked examples plus practice perform better on subsequent problem-solving tests than students who only solve practice problems on their own without guidance (Sweller and Cooper, 1985; Renkl, 2014). Some cognitive load theorists use these findings to argue that less-guided pedagogies, such as guided inquiry, are not ideal for learners, particularly learners with limited prior knowledge, because of their high demands on working memory (Kirschner et al., 2006). Yet others have argued that research on worked examples plus practice relies on weak controls (i.e., minimal guidance) and results in narrow learning outcomes (e.g., near transfer; Kapur, 2016), and that high levels of cognitive load directed toward exploring problems can benefit the development of deeper levels of conceptual understanding and transfer (Schwartz et al., 2011; Kapur, 2016).
Productive Failure.
In productive failure (Figure 1), students explore problems and generate possible solutions on their own before receiving explicit guidance (e.g., explanations; Kapur, 2008). The productive failure approach stems from research on desirable difficulties. Learning tasks that contain desirable difficulties require more effort and make learning more challenging in the short term but more durable in the long term (Schmidt and Bjork, 1992; Bjork, 1994). The demands on cognitive load are useful, because presenting students with challenging problems first, before guidance, prepares them for future learning (Schwartz and Martin, 2004; Schwartz et al., 2011). Studies show that students who learn from productive failure outperform students who receive instruction in the form of lecture followed by problem-solving practice (Kapur, 2011; Kapur and Bielaczyc, 2012; Weaver et al., 2018; Steenhof et al., 2019). The benefits of productive failure include gains in conceptual knowledge and far transfer (Schwartz et al., 2011; Kapur, 2016; Loibl et al., 2017). Proponents of productive failure hypothesize that it is advantageous, especially for far transfer, because it helps learners activate their prior knowledge, recognize their own knowledge gaps, and focus on the underlying structure of problems before explicit instruction (Kapur, 2016; Loibl et al., 2017). In contrast, the worked examples plus practice approach risks that students will merely learn to apply provided procedures to practice problems without the deep conceptual understanding needed for transfer (Schwartz et al., 2011). However, some have argued that research on productive failure also suffers from inappropriate control conditions (Glogger-Frey et al., 2015) and has primarily been tested across a limited range of topics in mathematics (Loibl et al., 2017).
Guided Inquiry.
While worked examples plus practice and productive failure are well-defined approaches, guided inquiry is more ill-defined and suffers from imprecision in terminology. For example, depending on their specific implementation, one could categorize inquiry-based learning (Prince and Felder, 2006), problem-based learning (Dochy et al., 2003; Hmelo-Silver, 2004), case-based learning (Herreid, 2007), peer-led guided inquiry (Lewis and Lewis, 2005, 2008), and process-oriented guided-inquiry learning (POGIL; https://pogil.org; Farrell et al., 1999; Bailey et al., 2012) as types of guided-inquiry instruction. We acknowledge this variance in implementation and the fact that there are other structures used by instructors, such as hybrids of these techniques, that may be effective (Eberlein et al., 2008). However, for the purpose of this paper, we define guided inquiry as an approach in which students actively engage in solving problems to learn critical concepts and practices and are guided throughout the process (Hmelo-Silver, 2004). Guidance through this process ranges in level of explicitness based on the learner’s prior knowledge, but broadly consists of hints, prompts, questions, or even direct explanation from an instructor or learning assistant (Hmelo-Silver et al., 2007; Lazonder and Harmsen, 2016). Additionally, we consider guided-inquiry instruction to have the following characteristics: 1) students working together in small groups, 2) the instructor and learning assistants acting as facilitators of learning rather than as proprietors of knowledge, and 3) scaffolds or instructional supports that fade away as knowledge is built (van Merriënboer and Kirschner, 2007).
As defined, guided inquiry stems from social constructivism theory, which recognizes knowledge is built by the learner and is impacted by cooperative social interactions (Bodner et al., 2001; Eberlein et al., 2008). While proponents of worked examples plus practice criticize guided inquiry for ignoring the limitations of human working memory (Kirschner et al., 2006), guided-inquiry proponents argue that scaffolded guidance effectively manages students’ cognitive load (Schmidt et al., 2007). Because guided inquiry suffers from imprecision in terminology, it is challenging to characterize the evidence base for this approach. In the K–12 literature, guided inquiry has been shown to improve student learning outcomes compared with unguided inquiry (Lazonder and Harmsen, 2016). In higher education, students in a POGIL-style chemistry course scored as high as or higher on the final exams than students who had taken a more traditional lecture-based course from the same instructor (Farrell et al., 1999). Additionally, students in a peer-led guided inquiry–style chemistry course experienced improved performance on the ACS Exam compared with students in more traditional lecture-based courses (Lewis and Lewis, 2008). Compared with traditional lecture, case-based learning in an introductory biology course improved exam performance, including performance on questions requiring application and analysis (Chaplin, 2009). Problem-based learning has also been shown to improve retention, application, and skill development compared with more traditional teaching methods (Dochy et al., 2003; Prince, 2004). Overall, the literature substantiates that guided inquiry–related approaches can improve student learning. However, as is evident from the preceding examples, the literature is limited due to the use of comparison groups that provide no guidance for problem solving or lecture only with no time for problem-solving practice.
Controversies and a Need for Comparison Studies
Worked examples plus practice, productive failure, and guided inquiry all have been shown to enhance student learning, yet they have not been directly compared. This is an important deficit in the literature. First, researchers have recently hypothesized unique advantages of each pedagogy for serving different learning outcomes (Kalyuga and Singh, 2016; Kapur, 2016). For instance, worked examples plus practice may be best for learning specific procedures and near transfer, while productive failure and guided inquiry may be best for promoting far transfer. Second, given that guided inquiry is a common instructional approach in biology and chemistry, it is of interest to the DBER community to compare guided inquiry with the other pedagogies. Third, research studies for all three pedagogies are limited due to weak comparison groups (e.g., no guidance, no time for problem-solving practice, weak forms of direct instruction, lecture only). Stronger comparisons among pedagogies involving guidance and problem-solving practice are more intriguing to researchers and educators. Finally, the majority of research studies highlighted earlier focus on domains in mathematics, such as algebra and statistics. The context-specific boundaries of this research base should be expanded to include domains like biology that rely heavily on conceptual knowledge. In this paper, we address these gaps in the literature with an investigation that directly compares worked examples plus practice, productive failure, and two forms of guided inquiry in the context of biochemistry.
Chosen Context of Biochemistry
We purposefully chose the context of biochemistry for this comparison. Introductory biochemistry courses play an important role in STEM undergraduate curricula because they 1) are required for many STEM majors, 2) include content that is critical for health professional entrance exams, and 3) integrate the disciplines of biology and chemistry. Biochemists agree on a set of core concepts that define the discipline (Loertscher et al., 2014; American Society for Biochemistry and Molecular Biology, 2020). One particularly challenging concept for students is the physical basis of noncovalent interactions (PBI). PBI builds on students’ general chemistry and introductory biology content knowledge to bring together the idea that noncovalent interactions occur due to the electrostatic properties of biological molecules (Cooper et al., 2015). Students with expertise in this concept recognize that although interactions are given different names (i.e., ionic interactions, hydrogen bonds, van der Waals forces), they are all based on the same electrostatic principle of attraction due to opposite charge (Loertscher et al., 2014). PBI is so central to biochemistry that once students deeply understand it, their view of the discipline is transformed (Loertscher et al., 2014). PBI is a content area ripe for instructional design because of known student difficulties with causal mechanisms of how noncovalent interactions arise (Becker et al., 2016; Halmo et al., 2018). Although problems that deal with PBI are difficult and challenging for undergraduate students, incoming biochemistry students have prior knowledge in biology and chemistry that could be activated to help them solve these problem types.
Cross-Disciplinary Research Question
Researchers in both DBER and educational psychology assert that direct comparisons of distinct evidence-based pedagogies could resolve outstanding questions in each field and enable the optimization of student learning of persistently troublesome biology concepts. By drawing upon the strengths and shared goals of educational psychology and DBER (McDaniel et al., 2017), we aim to test general learning mechanisms within a specific disciplinary context that is persistently troublesome for students and, thus, advance both research and practice. Specifically, we address the following research question: What are the comparative impacts on student learning of PBI for methods of instruction that vary in the nature and timing of guidance, namely, worked examples plus practice, productive failure, and two forms of guided inquiry?
METHODS
Study Design
We compared the impacts on student learning of four instructional approaches: worked examples plus practice, productive failure, and two forms of guided inquiry (unscaffolded and scaffolded guidance). To do this, we recruited students from the two prerequisite courses for Introductory Biochemistry and Molecular Biology (Introductory Biology and Modern Organic Chemistry I). in Spring 2018 and Spring 2019. Due to logistical constraints, our study used an unbalanced incomplete block design, with semester of data collection (Spring 2018 and Spring 2019) serving as the block effect (Figure 2). Our study is unbalanced, because there are unequal sample sizes for each treatment across blocks. Our study is incomplete, because we did not test all treatments in each block: productive failure was tested in Spring 2018 and Spring 2019, worked examples plus practice and unscaffolded guidance were tested in Spring 2018 only, and scaffolded guidance was tested in Spring 2019 only. Students who agreed to participate and completed a basic knowledge pretest were randomly assigned to one of the conditions tested in each block. Each condition involved a 35- to 45-minute lesson about PBI. After instruction, participants completed an assessment of basic knowledge and transfer. We describe the participants, data collection, and data analysis in detail in the following sections.
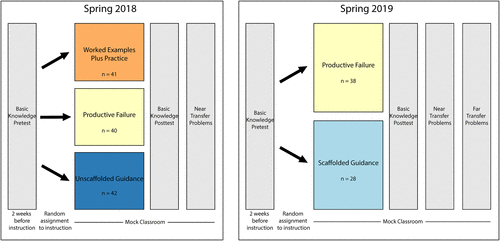
FIGURE 2. Study design for the comparison of impacts on student learning of four instructional approaches: worked examples plus practice, productive failure, unscaffolded guidance, and scaffolded guidance.
Participants
Data collection for this study took place over the course of two semesters (Spring 2018 and Spring 2019) at a research-intensive university in the southeastern United States. Spring 2018 participants were enrolled in one of three sections of Introductory Biology taught by one professor. Spring 2019 participants were enrolled in either Introductory Biology taught by one professor or Modern Organic Chemistry I course taught by two different professors. The researchers purposely chose to recruit students from Introductory Biology and introductory Modern Organic Chemistry I. courses at the end of the Spring semester, because these courses are required prerequisites for introductory biochemistry, and students at the end of these courses reflect the incoming biochemistry student population.
In Spring 2018, the researchers announced the study to 416 Introductory Biology students through in-class announcements. The instructor also allowed us to contact all 416 students through email. One hundred fifty-four of the 416 contacted students agreed to participate and completed the basic knowledge test (described under Basic Knowledge Test). We excluded data for 31 of these students, because they did not complete the entire study. One hundred twenty-three (30%) of the 416 contacted students completed the entire study. We randomly assigned these participants to one of three instructional conditions: 41 participants to worked examples plus practice, 40 participants to productive failure, and 42 participants to unscaffolded guidance. The 123 participants who completed the entire study received 10 points of extra credit toward their final Introductory Biology course grades (2.5% of the total possible points) as an incentive.
In Spring 2019, the researchers used in-class announcements to announce the study to 931 Introductory Biology and Modern Organic Chemistry I students. The instructors allowed us to follow up via email with students who gave us their names and email addresses. Two hundred twenty-seven students provided their names and email addresses. Ninety-five of the 227 contacted students agreed to participate and completed the basic knowledge test. We excluded data for 29 students from analyses, because they did not complete the entire study. Sixty-six (29%) of the 227 contacted students completed the entire study. We randomly assigned these participants to one of two instructional conditions: 38 participants to productive failure, and 28 participants to scaffolded guidance. The 66 participants who completed the entire study received $25 cash as an incentive.
The UGA institutional review board approved this study under exempt status (STUDY00000660 and PROJECT000000090). Demographic information of the participants can be found in Supplemental Table 1.
Data Collection
Development of Instructional Materials.
The authors developed all instructional materials. We designed them to help students achieve learning objectives pertaining to PBI and focused, in particular, on known student difficulties (e.g., Halmo et al., 2018). The materials are intended for use in introductory biochemistry courses. Three experts who are biochemistry instructors and discipline-based education researchers provided feedback on the worked examples plus practice, productive failure, and unscaffolded guidance materials. Three experts on the method of guided inquiry, who are also discipline-based education researchers, provided feedback on the scaffolded guidance instructional materials. We pilot tested the productive failure and scaffolded guidance materials in two focus groups. Four Introductory Biology students participated in the productive failure focus group in Spring 2018, and six Introductory Biology students and five Introductory Biochemistry and Molecular Biology students participated in the scaffolded guidance focus group in Spring 2019. We revised the materials based on expert feedback and pilot testing. We provide the finalized lesson materials used in this study, including handouts, instructor slides, and notes in the Supplemental Material.
Instructional Conditions.
To compare the impact of worked examples plus practice, productive failure, unscaffolded guidance, and scaffolded guidance, we randomly assigned participants to one of the conditions tested in each block. Each lesson lasted 35–45 minutes and took place in a SCALE-UP classroom (Beichner and Saul, 2003). SCALE-UP classrooms have several round tables with nine seats per table and are designed to facilitate student–instructor and student–student interactions. One of the authors (P.P.L.) taught all three lessons in Spring 2018, while a different instructor (trained by S.M.H.) taught both lessons in Spring 2019. We randomly assigned participants in each session to seats in the SCALE-UP classroom. The materials used and type of instruction experienced by participants differed depending on the instructional condition:
Worked examples plus practice condition: The instructor reviewed the learning objectives, introduced participants to a problem, and presented a worked example solution to the problem (i.e., an explicit explanation). She then gave participants time to practice a similar problem independently for several minutes, and then asked them to compare their solutions with the two people sitting closest to them. The instructor did not assist participants during independent problem solving or group sharing. In total, participants went through two rounds of this worked example–problem practice pairing.
Productive failure condition: The instructor reviewed the learning objectives and introduced participants to the same four problems that the worked examples plus practice participants practiced. However, the instructor provided no solution. Instead, she asked students to explore the problems with the participants at their table by comparing and contrasting the problems and generating as many possible solutions as they could (i.e., prompts). During this exploration, the instructor and two peer learning assistants in 2018 (one in 2019) walked around the room and noticed student work. They did not comment on the correctness of students’ ideas or direct them to a solution. Instead, they repeatedly asked students to explain what they were doing and pushed them to expand their thinking to all four problems. The instructor and peer learning assistants quickly conferred on the common ideas students were expressing. Then, after problem exploration, the instructor commented and built upon students’ ideas (Loibl et al., 2017). For example, the instructor and peer learning assistants noticed that students frequently compared the differences among having C, H, N, or O atoms in an amino acid R group. The instructor pointed this out to students and said, “That’s a good problem-solving step. The way to think about differences among atoms for this problem is to consider their differences in electronegativity and what this means in terms of full/partial and permanent/temporary charges.” After building on students’ solutions, the instructor presented two back-to-back worked examples (the same ones from the worked examples plus practice condition).
Unscaffolded guidance condition: In this condition, the instructor and two peer learning assistants provided guidance, but the instructional materials were not scaffolded (i.e., did not have supports that faded away as knowledge was built). We chose this approach to implement a guided-inquiry condition that was comparable to worked examples plus practice and productive failure in the number of problems covered and in overall session length. Thus, after the instructor reviewed the learning objectives, she gave participants the same four problems that the worked examples plus practice and productive failure participants received. However, she did not provide any solutions in the form of worked examples, and participants had the entire class period to work on the four problems with people at their tables. During this work time, the instructor and two peer learning assistants circulated and addressed participants’ questions. The instructor/peer learning assistants aimed to provide support based on a students’ prior knowledge. When interacting with students, they first diagnosed the students’ prior knowledge (e.g., by looking at participants’ work or asking them to explain their thinking). If possible, the instructors/peer learning assistants provided simple prompts (e.g., “Look at this aspect of the problem.”), but if participants’ prior knowledge was more limited, they provided explicit guidance (e.g., explanations of a concept). For example, if a student was stuck on hydrogen bonds, the instructor would ask probing questions like, “What is a hydrogen bond?” Next, the instructor might ask leading questions such as, “Do hydrogen bonds involve charges? If so, where do they come from?” At that point, if it was clear that participants had the knowledge they needed to proceed, the instructor would leave them to work. However, if it was clear that participants were unfamiliar with key ideas, the instructor would provide an explicit explanation. Anytime an explicit explanation was provided, participants were encouraged to use that explanation to help their work on subsequent problems.
Scaffolded guidance condition: In this condition, the instructor and four peer learning assistants provided guidance, and the instructional materials were scaffolded (i.e., had supports that faded away as knowledge was built). We chose this approach to implement a guided-inquiry condition that was comparable to worked examples plus practice and productive failure in session length, yet presented students with problems that progressed from simple to complex. The instructional materials included a total of 24 problems. These 24 problems encompassed the same four problems that participants in other conditions saw, but the problems were strategically broken down into component parts that built on one another. By the end of the problem set, students were solving a problem without any support. To start the session, the instructor reviewed the learning objectives. Then she gave participants the entire instruction time to work on the problem set with the participants at their tables. During this work time, the instructor and four peer learning assistants circulated the room and addressed participants’ questions. They followed the same principles of interaction as the unscaffolded guidance condition.
Assessments of Student Learning.
We used three assessments of student learning, which are described in the following sections. We administered all assessments through the Qualtrics (SAP, Walldorf, Baden-Württemberg, Germany) online survey platform. We provide all assessment items used in this study in the Supplemental Material.
Basic Knowledge Test.
The basic knowledge test was developed as part of a separate longitudinal study on student learning (same institution, N = 913). The test consists of 19 multiple-choice and multiple true-false items and addresses key terminology, the use of terms in context, and interpretation of common visual representations associated with PBI. We present five of the 19 items in Figure 3A and the full 19-item test in the Supplemental Material. A key for this test is available from P.P.L. upon request. We scrutinized the psychometric properties of the test, including dimensionality and reliability (unpublished data). In so doing, we used an item response theory (IRT) model. IRT is a probabilistic approach wherein a correct response to an item is defined as a function of person (i.e., ability) and item parameters based on unidimensionality and local independence assumptions (Embretson and Reise, 2000). We used a two-parameter logistic model. In the two-parameter logistic (2-PL) model, the correct response to an item is defined as a function of the student’s ability and the item’s difficulty level and discrimination power. The BILOG software was used to estimate person and item parameters (Zimowski et al., 1996). Results showed that the empirical reliability was acceptable at a value of 0.67 (Kline, 2000; Du Toit, 2003). We used the obtained item parameters from this 2-PL model to estimate students’ ability in the current study. For the current study, participants completed the basic knowledge test before and after instruction (Figure 2). Participants took the pretest on their own time. Participants took the posttest immediately after instruction in the same classroom where they received instruction. The pretest and posttest were identical, except they referred to proteins that differ in appearance (i.e., Protein Z for the pretest and Protein X for the posttest).

FIGURE 3. Three measures of student learning of PBI. Selected items from the basic knowledge posttest (A) and examples of a near-transfer problem (B) and a far-transfer problem (C) used in this study.
Near-Transfer Problems.
The three near-transfer problems used in this study are based on the Protein X problem published in Halmo et al. (2018) and were further revised based on interviews with one Introductory Biology student and two Introductory Biochemistry and Molecular Biology students in Spring 2016. These problems require students to make a prediction and explain that prediction (Figure 3B and Supplemental Material). As a reliability measure, we calculated Cronbach’s alpha between the three problems to be 0.75, which indicated good internal consistency (Kline, 2000). The near-transfer problems resemble the problems used during instruction, but present proteins that differ in appearance and involve different amino acids. Participants completed the near-transfer problems immediately after instruction following the basic knowledge posttest (Figure 2). The near-transfer problems were presented to students in random order.
Far-Transfer Problems.
The authors developed three far-transfer problems based on the previous work of Werth (2017). Six Introductory Biology students and five Introductory Biochemistry and Molecular Biology students in a Spring 2019 focus group provided feedback on the far-transfer problems, and we revised the problems as needed. These problems require students to make a prediction and explain that prediction (Figure 3C and Supplemental Material). These far-transfer problems draw upon the same conceptual knowledge as the near-transfer problems, but the context differs from the problems provided during instruction and thus requires a different solution structure. As for the near-transfer problems, we used Cronbach’s alpha as a reliability measure. Cronbach’s alpha (0.78) indicated good internal consistency (Kline, 2000). Due to the logistical constraints described earlier, only Spring 2019 participants (not Spring 2018 participants) completed the far-transfer problems, and they did so immediately after instruction (Figure 2).
Data Analysis
We downloaded from Qualtrics (SAP, Walldorf, Baden-Württemberg, Germany) all participant responses to the assessment items. We collected data on the basic knowledge pre- and posttests and written responses to the near- and far-transfer problems.
Scoring of Basic Knowledge Test.
As described in the previous section, we used the 2-PL IRT model to estimate item parameters with responses from 913 students. We estimated students’ ability for pre- and posttest in this study using those item parameters. The ability parameter in the IRT model can be interpreted as a z-score (M = 0; SD = 1). Hereafter, we refer to students’ ability estimates as “basic knowledge performance.”
Analytical Coding of Near- and Far-Transfer Written Responses.
Three of the authors (S.M.H., P.R., and O.S.) first read all written responses from both rounds of data collection. We developed an analytical codebook, informed by knowledge of published descriptions of student thinking about PBI (Loertscher et al., 2014; Cooper et al., 2015; Becker et al., 2016; Halmo et al., 2018), to capture common ideas. The authors (S.M.H., P.R., and O.S.) who served as coders and developers of the codebook were blind to condition during this phase of the research. We independently applied codes from the codebook to the written responses in a deductive manner and inductively created new codes as needed. The researchers then deductively applied these new codes to all written responses. The finalized codebook was applied to all written responses by two coders (S.M.H. and P.R.). After independent coding, we calculated intercoder reliability for all codes used in subsequent analyses (described in the next paragraph) using Cohen’s kappa (Gisev et al., 2013). Cohen’s kappa coefficients ranged from 0.21 to 0.96 for near-transfer coding and from 0.45 to 0.93 for far-transfer coding. The overall average Cohen’s kappa values for near-transfer and far-transfer coding were 0.74. and 0.76, respectively, which both indicated substantial agreement (Viera and Garrett, 2005). The researchers discussed all discrepancies in coding until complete agreement was reached. We provide the finalized analytical codebook for each problem in the Supplemental Material.
After the written responses were coded to consensus, three of the authors (S.M.H., P.R., and P.P.L.) and a team of undergraduate researchers developed a rubric that enabled us to assign a score to each near- and far-transfer problem based on the analytical codebook. The researchers involved in this scoring process were blind to condition. The scoring rubric for each problem is available in the Supplemental Material. The rubric captured the quality and correctness of predictions and the supporting evidence. The rubric also credited participants for attempting to support a prediction with evidence regardless of the quality or correctness of the prediction and evidence provided. More specifically, we awarded up to three points based on the quality and correctness of the prediction. We awarded up to three points based on the quality and correctness of the evidence. We awarded up to one point if both a prediction and evidence were provided, regardless of whether either was correct. We applied the scoring rubrics to all analytically coded written responses, resulting in a score from zero to seven for every written response item. To calculate overall near- and far-transfer performance, we summed the scores on the three problems for each participant and divided that sum by the highest possible score, generating an overall near- and far-transfer score.
Statistical Analyses
We separately analyzed basic knowledge posttest performance, near-transfer performance, and far-transfer performance. We set our alpha level at 0.05 for these three independent statistical tests. We analyzed basic knowledge posttest performance using a type II sum of squares analysis of covariance (ANCOVA), with semester of data collection serving as the block effect, basic knowledge pretest performance as a covariate, and instructional condition as the independent variable. We analyzed near-transfer performance using a type II sum of squares ANCOVA, with instructional condition as the between-subject variable, semester of data collection serving as the block effect, and basic knowledge pretest performance as the covariate. We analyzed far-transfer performance using type II sum of squares ANCOVA, with instructional condition as the between-subject variable and basic knowledge pretest performance as the covariate. When instruction type had a significant effect on the omnibus test (p < 0.05), Tukey’s least-squares means post hoc test was used to perform multiple comparisons. We determined the estimates of adjusted group mean differences to be statistically significant if p < 0.05. To calculate effect sizes for significant differences, we estimated Cohen’s d by dividing the adjusted group mean difference by the square root of the MSError from the analysis of covariance. All statistical analyses were conducted in R (R Core Team, 2019).
RESULTS
Prior Knowledge, Not Method of Instruction, Predicts Performance on the Basic Knowledge Test
We first evaluated whether there was a difference on pretest performance among instructional conditions. analysis of variance results indicated no significant differences on pretest performance among the four conditions, F(3, 184) = 0.19, p = 0.90. Previous work showed that worked examples plus practice, productive failure, and guided inquiry all enhance student learning. Thus, we did not expect differential advantages of different instructional methods on basic knowledge posttest performance. As anticipated, no instructional group outperformed the others on the basic knowledge posttest (Figure 4). The means for posttest performance (adjusted for pretest performance) were −0.48 for participants in the worked examples plus practice condition, −0.61 for participants in the productive failure condition, −0.48 for participants in the unscaffolded guidance condition, and −0.68 for participants in the scaffolded guidance condition (Table 1). The negative values do not indicate that no learning occurred (see Supplemental Table 2). The negative values only indicate that students in the current study performed below the population mean of the 913 students used for IRT analysis (see Methods). For basic knowledge posttest performance, we first tested to see whether there were significant interaction effects between condition and pretest performance. The interaction effect was not significant, F(3, 180) = 2.49, p = 0.06, so we removed the interaction from the model and report the ANCOVA results without the interaction. Specifically, using a type II ANCOVA, we found no significant effect of instruction type on basic knowledge posttest performance, F(3, 183) = 0.48, p = 0.69. We also found no significant effect of semester of data collection on basic knowledge posttest performance, F(1, 183) = 1.12, p = 0.29. Additionally, when the data were filtered to look at participants in the productive failure condition only, the semester block (either Spring 2018 or Spring 2019) did not have a significant effect on posttest performance, F(1, 76) = 0.52, p = 0.48, suggesting no semester block differences. However, there was a significant effect of basic knowledge pretest performance on basic knowledge posttest performance, F(1, 183) = 16.68, p < 0.001. In sum, worked examples plus practice, productive failure, unscaffolded guidance, and scaffolded guidance did not differentially impact basic knowledge posttest performance (Figure 4).

FIGURE 4. Basic knowledge posttest performance by instruction type. For each box-and-whisker plot, the black horizontal line represents the median basic knowledge posttest performance (unadjusted for pretest performance), the hinges represent the first and third quartiles, and the whiskers extend to the highest and lowest values that are within 1.5 times the interquartile range of the hinge. Dots represent individual participants, and values can be interpreted as a z-score (M = 0; SD = 1). Positive and negative values do not indicate learning and no learning, respectively. Rather, positive values indicate that a student performed above the population mean of the 913 students included in IRT analyses, while negative numbers indicate a student performed below the population mean. WE, worked examples plus practice; PF, productive failure; UG, unscaffolded guidance; SG, scaffolded guidance.
Instruction | Mean | SD |
---|---|---|
Worked examples plus practice (n = 41) | −0.48 | 0.74 |
Productive failure (n = 78) | −0.61 | 0.60 |
Unscaffolded guidance (n = 42) | −0.48 | 0.74 |
Scaffolded guidance (n = 28) | −0.68 | 0.70 |
More than Unscaffolded Guidance Is Needed for Near-Transfer Problem Solving
While we found no significant effect of instructional condition on basic knowledge posttest performance, we did find a significant effect of instructional condition on near-transfer problem solving (Figure 5). Specifically, worked examples plus practice, productive failure, and scaffolded guidance outperformed unscaffolded guidance on near-transfer problem solving. The mean near-transfer score was 0.63 for worked examples plus practice, 0.58 for productive failure, 0.44 for unscaffolded guidance, and 0.66 for scaffolded guidance participants (Table 2). For near-transfer performance, we first tested to see whether there were significant interaction effects between condition and pretest performance. The interaction effect was not significant, F(3, 180) = 1.62, p = 0.19, so we removed the interaction from the model and report the ANCOVA results without the interaction. Using a type II ANCOVA with semester of data collection as a block effect and basic knowledge pretest performance as a covariate, we found a significant effect of instruction type on near-transfer problem-solving performance, F(3, 183) = 5.36, p = 0.001. Post hoc comparisons using the least-squares means Tukey adjusted test indicate that the mean near-transfer problem-solving performance for the unscaffolded guidance condition was significantly lower than the worked examples plus practice condition (d = 0.72), the productive failure condition (d = 0.65), and the scaffolded guidance condition (d = 1.08; Table 3). Basic knowledge pretest performance significantly affected near-transfer problem-solving performance, F(1, 183) = 4.21, p = 0.04, whereas semester block did not significantly affect near-transfer problem-solving performance, F(1, 183) = 1.43, p = 0.23. Additionally, when the near-transfer scores were filtered to look at participants in the productive failure condition only, the semester block (either Spring 2018 or Spring 2019) did not have a significant effect on near-transfer performance, F(1, 76) = 0.96, p = 0.33, suggesting no semester block differences. This result suggests that more than unscaffolded guidance is needed to help learners solve problems similar to those seen in instruction.

FIGURE 5. Near-transfer problem-solving performance by instruction type. For each box-and-whisker plot, the black horizontal line represents the median near-transfer performance (unadjusted for pretest performance), the hinges represent the first and third quartiles, and the whiskers extend to the highest and lowest values that are within 1.5 times the interquartile range of the hinge. Dots represent individual participants.WE, worked examples plus practice; PF, productive failure; UG, unscaffolded guidance; SG, scaffolded guidance.
Instruction | Mean | SD |
---|---|---|
Worked examples plus practice (n = 41) | 0.63 | 0.28 |
Productive failure (n = 78) | 0.58 | 0.28 |
Unscaffolded guidance (n = 42) | 0.44 | 0.24 |
Scaffolded guidance (n = 28) | 0.66 | 0.20 |
Comparison | Adjusted mean difference | SE | p value | Effect size (Cohen’s d) |
---|---|---|---|---|
Productive failure–scaffolded guidance | −0.11 | 0.06 | 0.32 | |
Productive failure–unscaffolded guidance | 0.17 | 0.06 | 0.02* | 0.65 |
Productive failure–worked examples plus practice | −0.02 | 0.06 | 0.99 | |
Scaffolded guidance–unscaffolded guidance | 0.28 | 0.09 | 0.008* | 1.08 |
Scaffolded guidance–worked examples plus practice | 0.10 | 0.09 | 0.71 | |
Unscaffolded guidance–worked examples plus practice | −0.18 | 0.06 | 0.007* | 0.72 |
Different Types of Failure Do Not Differentially Impact Far-Transfer Problem Solving
Given that more than unscaffolded guidance is needed for near-transfer problem solving, we investigated how the other forms of instruction impacted far-transfer problem solving. Only participants in Spring 2019 were asked to solve far-transfer problems, and we did not recruit enough participants in Spring 2019 to test three conditions. Therefore, we elected to test productive failure and scaffolded guidance, but not worked examples plus practice, because it was unknown whether productive failure and guided inquiry differentially impact far-transfer problem solving (Kapur, 2016). In our experiment, scaffolded guidance and productive failure did not differentially impact far-transfer performance. The mean far-transfer score was 0.51 for participants in the productive failure condition and 0.58 in the scaffolded guidance condition (Table 4). For far-transfer performance, we first tested to see whether there were significant interaction effects between condition and pretest performance. The interaction effect was not significant, F(1, 62) = 0.83, p = 0.37, so we removed the interaction from the model and report the ANCOVA results without the interaction. Using a type II ANCOVA with basic knowledge pretest performance as a covariate, we found no significant effect of instruction type, F(1, 63) = 0.96, p = 0.33, or basic knowledge pretest performance, F(1, 63) = 0.06, p = 0.81, on far-transfer performance. Our data suggest that explicit guidance after problem solving (i.e., productive failure) and guidance distributed throughout problem solving in the form of scaffolded materials and instructor support (i.e., scaffolded guidance) do not differentially impact far-transfer problem solving.
Instruction | Mean | SD |
---|---|---|
Productive failure (n = 38) | 0.51 | 0.25 |
Scaffolded guidance (n = 28) | 0.58 | 0.24 |
LIMITATIONS
Readers should consider the following limitations when evaluating our findings. First, the nature of the participant recruitment and data collection in this study led to an incomplete block design. While every instruction type was not represented in each block, there was overlap from Spring 2018 to Spring 2019 of the productive failure instructional condition. We accounted for block in our statistical analyses and found no significant differences. A second limitation related to the first is that low recruitment in Spring 2019 necessitated a reduction in the number of treatments for that block. The research team prioritized the testing of productive failure and scaffolded guidance, which prevented us from investigating worked examples plus practice in our analysis of far-transfer performance. Researchers hypothesize that worked examples plus practice may lead to lower performance on far transfer compared with productive failure or scaffolded guidance (Kapur, 2016). Future research should test this hypothesis. Third, we were limited by sample size. One hundred eighty-nine (14%) of the 1347 students recruited to participate in the study actually participated, suggesting the students who did participate may have differed from a typical student population. For example, our sample was disproportionately female (see Supplemental Material). In addition, the average posttest scores were below the mean for the instrument (see Table 1), suggesting the students in this sample differ from the students used in the 2-PL IRT model. One possible explanation for the below-average basic knowledge scores is the fact that participants in this study took the test outside class time, whereas participants whose data were used to generate the 2-PL IRT model took the test during class time and therefore might have taken it more seriously. Also, we cannot rule out the possibility that the students who did participate may have had greater motivation for learning the material or for the extrinsic rewards offered as incentives. Therefore, future work should investigate similar research questions in an authentic classroom setting. Additionally, given the sample size constraint, a sensitivity power analysis with alpha set to 0.05 and power set to 0.85 reveals a minimal detectable effect of 0.26 for the basic knowledge and near-transfer analyses and a minimal detectable effect of 0.37 for the far-transfer analysis, indicating that we could at minimum detect a medium (0.25–0.40) or large (>0.40) effect. Smaller differential effects may exist, but we did not have the statistical power in our data set to detect them. Replicating the study with an increased sample size could resolve this issue. Fourth, to minimize participant burden and increase compliance, we administered assessments immediately following instruction. Therefore, we cannot answer the question of whether the results obtained persisted for longer periods of time. Fifth, our measures of basic knowledge, near transfer, and far transfer may not have been sensitive enough to detect differences that did exist. Finally, we investigated only one content area (PBI), so our claims are not generalizable to other content areas.
DISCUSSION
Given the calls for second-generation research on active learning (Freeman et al., 2014) and cross-disciplinary collaborations between biology education and educational psychology (McDaniel et al., 2017), we set out to determine the comparative impacts of worked examples plus practice, productive failure, and two forms of guided inquiry (i.e., unscaffolded and scaffolded guidance) on student learning of a challenging concept in biochemistry, PBI. We discuss our findings in the context of past research, explore their significance, and propose future directions.
The Nature and Timing of Guidance May Not Matter for Near Transfer
We show that multiple, but not all, instructional methods can achieve comparable learning gains. Prior research on worked examples plus practice, productive failure, and guided inquiry suffered from the use of weak controls (e.g., minimal guidance or weaker forms of explicit instruction such as lecture only; Glogger-Frey et al., 2015; Kapur, 2016). Pedagogies with some form of guidance unsurprisingly outperformed those with no guidance (Mayer, 2004), but how do guided pedagogies measure up to one another? Until now, the literature lacked a direct comparison of worked examples plus practice, productive failure, and guided inquiry (Figure 1). In this work, we conducted a head-to-head comparison to show that worked examples plus practice, productive failure, unscaffolded guidance, and scaffolded guidance led to comparable basic knowledge outcomes (Figure 4). We also show that worked examples plus practice, productive failure, and scaffolded guidance led to comparable near-transfer problem solving that is significantly better than unscaffolded guidance (Figure 5). Finally, we show that productive failure and scaffolded guidance produced comparable far-transfer problem solving (Figure 6). These novel findings shed light on the debate in educational psychology about the nature and timing of guidance (Kirschner et al., 2006; Hmelo-Silver et al., 2007; Kapur, 2016) and suggest that instructors have some flexibility in choosing among the tested approaches.

FIGURE 6. A comparison of far-transfer problem-solving performance between productive failure (PF) and scaffolded guidance (SG). For each box-and-whisker plot, the black horizontal line represents the median far-transfer performance (unadjusted for pretest performance), the hinges represent the first and third quartiles, and the whiskers extend to the highest and lowest values that are within 1.5 times the interquartile range of the hinge. Dots represent individual participants.
We did not detect differences among worked examples plus practice, productive failure, or scaffolded guidance on near-transfer performance. Likewise, for far transfer, we did not detect differences in learning for productive failure compared with scaffolded guidance. Taken together, these findings suggest that at least near transfer can be achieved whether the nature of guidance involves explicit explanations (i.e., worked examples plus practice and productive failure) or scaffolded instructional materials (i.e., scaffolded guidance). Transfer can also be achieved whether guidance is early, late, or distributed throughout problem solving, although future research should compare worked examples plus practice, productive failure, and scaffolded guidance for far-transfer problem solving (Kapur, 2016).
We stress one important caveat regarding the nature of guidance. If guided inquiry is used, scaffolded instructional materials seem to be important. Our scaffolded guidance materials sequenced problem solving into increasingly complex questions, while the unscaffolded guidance condition simply provided problems for participants to solve. Even though the unscaffolded guidance condition was designed to provide just-in-time support, perhaps this was not sufficient, because only some students requested help, and there were not enough members of the instructional team to help students break down the problem into components pieces. The unscaffolded guidance condition involved two learning assistants, while the scaffolded guidance condition involved four learning assistants. Unscaffolded guidance can occur unintentionally among instructors who aim to create active-learning environments. These instructors may give students problem sets, but not break them down into manageable chunks that lead students from simple to complex thinking. This could happen even if instructors stop lecturing and give students plenty of time to work in class. Along these lines, note that our implementation of unscaffolded guidance would be categorized as student centered by the Classroom Observation Protocol for Undergraduate STEM (COPUS) protocol (Smith et al., 2013; Lund et al., 2015), multiple-voice by the Decibel Analysis for Research in Teaching (DART) method (Owens et al., 2017), and high structure (Eddy and Hogan, 2014). Unscaffolded guidance participants engaged in problem solving for nearly the entire class period, supported by an instructor and two learning assistants. Despite this design, near-transfer learning for the unscaffolded guidance condition was inferior to the three conditions receiving more guidance.
The general comparability of pedagogical approaches observed in the present study is somewhat surprising given the heated debates among their proponents. However, all the pedagogies tested have been shown to positively impact learning, so maybe their differences are not as important as was previously suspected. Alternatively, the instructional approaches we implemented may not differ enough from one another. We may have inadvertently omitted critical features from one or more of the methods that would have led to differential impacts on near- or far-transfer problem solving. For example, perhaps unscaffolded guidance could be successful if the number of learning assistants was increased. Another alternative explanation is that the dosage of instruction was not adequate. We might have detected differences if the instructional sessions lasted longer or if instruction spanned over multiple lessons, although a previous meta-analysis on instructional guidance indicates that the length of an instructional study does not impact its effect size (Lazonder and Harmsen, 2016). Finally, the limitations of our study may mask differential impacts (see Limitations).
Implications for Active-Learning Instructors
Instructors new (and even somewhat new) to active learning frequently want to know whether one instructional method is better than another or whether there are things not to do. Our data provide much-needed guidance for these instructors. First, our data suggest that some variability in the nature and timing of guidance may be just fine for student learning. For example, instructors who struggle to see themselves going from straight lecture classrooms to guided inquiry (in which most of the class period is spent in student work) may find productive failure as a potentially easier transition that appears to be equally effective. With productive failure, an instructor must carefully craft a challenging problem for the start of class and follow it by connecting students’ solutions with the varieties of ways experts solve the problems (Kapur, 2016; Loibl et al., 2017). Crafting these problems may be challenging for a new active-learning instructor, but explicitly explaining the problem to students should feel familiar. Second, our data suggest that unscaffolded guidance should be avoided. Even though unscaffolded guidance looks and feels like active learning, it did not maximize near-transfer problem solving in our study. Getting students talking and working more in class, while a great first start, is not sufficient to implement successful active learning. Instructors may experience less than optimal student outcomes if they only add clicker questions or challenging problems to their lessons. Instead, instructors should aim to create well-scaffolded lesson materials that break problems down into manageable pieces, sequence them carefully from the start to finish of a lesson, and consider how they will introduce and follow up questions. Finally, and unsurprisingly, what students know coming into a classroom setting matters, and instructors should remember that eliciting students’ prior knowledge is a worthwhile endeavor.
Potential Implications for Classroom Climate and Other Noncognitive Factors
Although our study did not investigate classroom climate or other noncognitive factors, future research should take these factors into consideration. First, different instructional methods may be better suited for building classroom equity. Classroom equity refers to promoting fairness in the classroom so that all students have the opportunity to participate, think, pose ideas, construct their knowledge, and feel welcomed into the intellectual discussion (Tanner, 2013; Miller and Tanner, 2015). In our study, only the scaffolded guidance condition provided all students with access to guidance from instructional materials along with opportunities to construct knowledge through interactions facilitated by the instructional team. Worked examples plus practice and productive failure provided all students with guidance through the problem-solving process. However, students had limited opportunities to pose ideas or to receive feedback from the instructor. On the other hand, the unscaffolded guidance condition provided all students with the chance to pose ideas and questions, construct knowledge, and participate in intellectual discussion. Yet the instructional materials themselves offered no guidance, only prompts to solve a challenging problem. These differences may impact students’ perceptions of classroom equity. Second, various instructional methods may differentially interact with noncognitive aspects of student development. The amount of struggle and level of challenge that students experience in worked examples plus practice, productive failure, and guided inquiry likely varies. Worked examples plus practice reduces challenge and provides explicit explanations and support, so little to no struggle is experienced. In contrast, productive failure and guided inquiry force students to struggle with the material and even to fail at solving problems correctly. Noncognitive aspects of student development that may interact with these instructional methods include, but are not limited to, motivation to learn, self-efficacy, and resilience (Trujillo and Tanner, 2014; England et al., 2019; Henry et al., 2019). A potentially fruitful area of research would be to compare the impacts of each method on classroom equity and other noncognitive factors.
CONCLUSIONS
This work serves as a model of research that draws from theoretical and empirical work in educational psychology to inform classroom practice in biology, while refining context-specific boundaries for worked examples plus practice, productive failure, and guided-inquiry approaches. Importantly, this work provides the first direct comparison of these approaches while simultaneously extending previous work on these pedagogies to the conceptual domain of biochemistry. The biochemistry lesson materials developed for this study target known student difficulties and help students craft explanations for near- and far-transfer problems. While this work advances both the fields of educational psychology and DBER, there is still more cross-disciplinary work to be done. Lessons developed here can be further improved by incorporating other known principles from educational psychology, like drawing to learn (Van Meter and Garner, 2005; Ainsworth et al., 2011; Quillin and Thomas, 2015; Fiorella and Zhang, 2018). Additionally, future work can address the question of who benefits most from these different pedagogies.
ACKNOWLEDGMENTS
We would like to acknowledge the participants for their time and effort. We kindly thank Sarah Baas Robinson, Erin Dolan, Julie Dangremond Stanton, Regina Frey, and Jennifer Loertscher for their helpful feedback during the development of our lesson materials. We thank Sarah Baas Robinson, Kush Bhatia, Jamie Pham, Justin Rubin, and Ave Fouriezos for their assistance with data collection. We also thank Vanessa Alele and Grace Snuggs for their assistance with coding and scoring. Special thanks to Dan Hall and Huimin Hu of the University of Georgia, Athens (UGA) Statistical Consulting Center and Hye-Jeong Choi for their advice with statistical analyses. We also express gratitude to our supportive colleagues in the Biology Education Research Group at UGA for their helpful feedback on earlier drafts of this article. P.P.L. would like to thank the UGA Study in a Second Discipline program for the fellowship that led to collaboration with L.F. S.M.H. would like to thank David Live for introducing her to L.F.’s research and the ARCS Foundation for its financial support. This material is based upon work supported by the National Science Foundation under grant no. DRL1350345.