Student Behaviors and Interactions Influence Group Discussions in an Introductory Biology Lab Setting
Abstract
Past research on group work has primarily focused on promoting change through implementation of interventions designed to increase performance. Recently, however, education researchers have called for more descriptive analyses of group interactions. Through detailed qualitative analysis of recorded discussions, we studied the natural interactions of students during group work in the context of a biology laboratory course. We analyzed multiple interactions of 30 different groups as well as data from each of the 91 individual participants to characterize the ways students engage in discussion and how group dynamics promote or prevent meaningful discussion. Using a set of codes describing 15 unique behaviors, we determined that the most common behavior seen in student dialogue was analyzing data, followed by recalling information and repeating ideas. We also classified students into one of 10 different roles for each discussion, determined by their most common behaviors. We found that, although students cooperated with one another by exchanging information, they less frequently fully collaborated to explain their conclusions through the exchange of reasoning. Within this context, these findings show that students working in groups generally choose specific roles during discussions and focus on data analysis rather than constructing logical reasoning chains to explain their conclusions.
INTRODUCTION
Environments that allow for learning are naturally social in nature. According to social cognitive theory, learning occurs in and cannot be separated from a social context (Bandura, 1977; Vygotsky, 1978). Furthermore, within social learning contexts, the ways students engage with one another can impact whether they are able to generate conceptual explanations for and derive meaning from the content they learn (Chi, 2009). Ultimately, when students work together in groups, grapple with different interpretations of data, and construct conclusions or models collaboratively, their learning is deeper. Historically, these collaborative interactions have been called “argumentation,” a method of reasoning in which consensus about a claim is reached through using evidence to explain a rationale for drawing a conclusion (Toulmin, 1958; Erduran et al., 2004, Osborne, 2010). When instructors encourage collaborative practices that lead to argumentation, students can learn to engage in critical thinking, problem-solving, and scientific communication, through which they develop better understanding of scientific concepts (Cavallo, 1996; Cavallo et al., 2004; Johnson and Lawson, 1998; Berland and Reiser, 2009; National Research Council [NRC], 2012) and their ability to use logical and scientific reasoning (NRC, 2007; American Association for the Advancement of Science [AAAS], 2011). However, there is also evidence that students generally do not choose to exchange reasoning during in-class discussions without prompting or training (Zohar and Nemet, 2002; Lubben, 2009; Knight et al., 2013, 2015).
Students who have the opportunity to participate in collaborative discussion and engage in argumentation show an increase in knowledge and in their ability to use reasoning (Johnson et al., 1993; Johnson and Johnson, 1999). Engaging in argumentation also encourages students to think scientifically and exchange reasoning with peers (Kuhn, 1993; Koslowski, 1996; Zohar and Nemet, 2002; Asterhan and Schwartz, 2009) and to perform better on tasks that require the use of reasoning (Boa et al., 2009; Osborne, 2010). For example, Felton et al. (2015) showed that students who engaged in argumentation with the intention of reaching consensus were more likely to construct knowledge but also to increase the quality of their arguments. However, variation in the ways that students choose to interact can also have negative implications both within their current groups and on future individual performance (Weldon and Bellinger, 1997; Blumen et al., 2014; Barber et al., 2015; Marion and Thorley, 2016). Thus, understanding how students interact when solving problems in a group and exploring how reasoning is used in such settings may inform ways to implement peer discussion to generate high-quality learning experiences (Repice et al., 2016; Leupen et al., 2020).
Many studies have shown that student interactions are modulated by circumstance and context. For example, in the process-oriented guided-inquiry learning (POGIL) instructional approach, assigning students to take on specific roles during discussion can positively affect a group’s productivity (Moog and Spencer, 2008). These assigned roles target students to a particular task, such as leading (manager or captain), presenting final conclusions (presenter or spokesperson), recording final conclusions (recorder), and reflecting on performance (reflector, document control, checker; Farrell et al., 1999). When implemented effectively, this approach can promote individual participation and cultivate functional groups (Simonson, 2019) and improve performance and retention. For example, undergraduates enrolled in a chemistry lab and biomechanics courses using the POGIL approach earned higher course grades than those in a non-POGIL course and were more likely overall to successfully complete the course (Farrell et al., 1999; Simonson and Shadle, 2013). An outstanding question is whether students need to be assigned to task-oriented roles to be successful. Eddy et al. (2015) showed that students may naturally gravitate to certain behaviors when placed into a group setting, but Farrell et al. (1999) noted that when students self-selected roles in group discussions, they usually chose behaviors with which they were most comfortable, possibly preventing them from challenging themselves.
The goal of this study was to characterize natural group interactions in relatively stable groups over time and to specifically explore the components of discussion that affected students’ use of reasoning. We used the ICAP framework (Chi and Wylie, 2014) to provide theoretical backing for this work. The ICAP distinguishes between different types of active learning and describes the likely cognitive levels of understanding that can be achieved in each. Interactive is defined as multiple students collaboratively make inferences, while in constructive, students make inferences, enabling problem solving. These are distinguished from active, in which students receive information and connect it to prior knowledge, and passive, in which students only receive information. Chi and Wylie suggested that only when students engage in an interactive or constructive manner can they achieve higher-order learning. When interactive, students are both constructive and engaged with one another, working to create new ideas and meaning in a way that would not be possible individually. This is supported by other work (Osborne and Patterson, 2011) showing that when students act interactively, they can transition from using simple explanations to using reasoning to support their ideas. Small-group student discussions, which we used in this study, have the capacity to be interactive; however, they are by no means automatically so. Thus, there is a need to explore how students choose to engage in such settings, and how their engagement affects their use of reasoning.
In this study, we used a mixed-methods approach to explore natural student interactions in an introductory biology laboratory setting with three major goals: 1) characterize the behaviors of students engaged in unguided group discussion in which social and scientific interactions were expected but not explicit; 2) characterize student-chosen roles, their permanence, and their impact on other group dynamics; and 3) Characterize the use of reasoning in groups and whether it changes based on group interactions.
METHODS
Characterization of the Course and Students
All students were enrolled in a course-based undergraduate research experience (CURE) laboratory: Drug Discovery through Hands-on Screens, in which students in each section of the course worked as teams to screen for novel antibiotics using thousands of compounds from a small-molecule library and the bacterium Salmonella as a model system. The lab met in 2-hour sessions, twice a week for 15 weeks. There were six sections of 18–23 students per section, for a total of 121 students. In each of the sections, students interacted with one another, a faculty coordinator, and teaching assistants. Students self-selected into groups of three to five during the first week of class and generally maintained these groups over the course of the semester. For consenting students, we collected the following demographic data: gender, race/ethnicity, year, underrepresented minority (URM) status, first-generation status, and incoming standardized test scores (ACT or Scholastic Aptitude Test [SAT]; Table 1). Because we had a relatively low number of individual races and ethnicities reported, we grouped individuals not identifying as “white” under the acronym BIPOC (Black, Indigenous, and persons of color).
Sex | Percent | Race/ethnicity | Percent | Status | Percent | Incoming performance | Average score |
---|---|---|---|---|---|---|---|
Female | 72.2% | White | 71.1% | First year (freshman) | 60.8% | ACT Total | 27.86 |
Male | 27.8% | BIPOC | 28.9% | URM status | 12.4% | ACT Math | 28.81 |
First generation | 16.5% | SAT Total | 1256.27 | ||||
SAT Math | 633.05 |
Assignments and Discussions
We developed a set of six assignments for this study (Figure 1); two individual, used as pre–post measures, and four used for group discussions during lab sessions over the course of the semester. All assignments presented experiments and data similar to the content of the course. We used an individual pre–post assignment to establish baseline and endpoint measures for each student, realizing that group discussions might not reflect how individuals process information by themselves. Students completed the pre assignment online before any group discussions and again online as part of one of their final assignments for the course (post). Each question included 1) an introduction with background information; 2) data in the form of a graph and raw numerical data; and 3) a prompt to provide claims, evidence, and reasoning (Figure 2).

FIGURE 1. Order and timing of each assignment. All six assignments presented data from drug screens conducted on different bacterial strains.
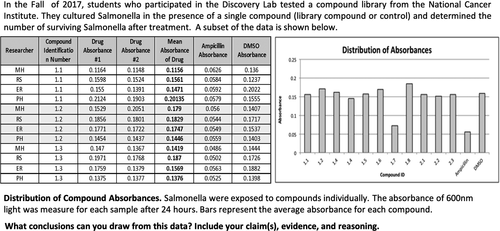
FIGURE 2. Pre and post assignment. Students completed the pre and post assignments individually at the beginning (week 1) and end (week 15) of the semester.
For the in-class group discussions over the semester (Figure 3), each assignment was provided to students as a printed worksheet. Students were not specifically guided in any way before these discussions; thus, their responses were untrained with regard to producing claims, evidence, and reasoning during data analysis. Students audio-recorded themselves as they discussed the assignment, and the instructor collected the recordings as part of normal course work for participation credit. Of the 121 students enrolled in the course, 98 students in 30 groups agreed to have their assignment recordings used for research purposes (University of Colorado Institutional Review Board protocol 16-0511). We did not analyze recordings from groups with nonconsenting students. After the conclusion of the lab course, we transcribed the recordings verbatim. In most recordings, all students could be heard for the entirety of the discussion; however, due to seat location and/or voice projection, occasionally some turns of discussion were not audible and could not be included in the analysis. Only one discussion was mostly inaudible and thus could not be analyzed.
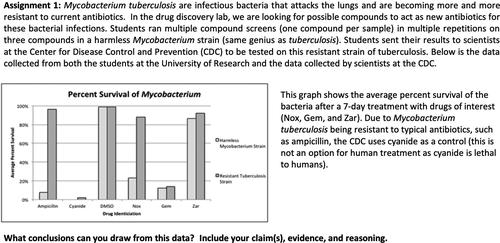
FIGURE 3. Example of a discussion assignment. A physical copy of the assignment was given to each student group, along with an audio recorder, at the beginning of the lab period. Students were asked to discuss the data and answer the questions while audio-recording their discussions.
Data Analysis
We took a mixed-methods approach to data analysis, collecting quantitative measures on performance and participation and constructing detailed qualitative analyses of student interactions during discussion (Maxwell, 2013; Patton, 2009). All students were given pseudonyms.
Quantitative Features.
We scored each student’s individual answers (pre and post; n = 45) as correct or incorrect and also noted the number and nature of reasoning statements made, as an indication of the student’s ability to construct an argument (Table 2) For each of the group assignments, we determined the length of each discussion (minutes) and an individual’s time spent talking to determine an individual’s participation index (time the individual spent speaking/total time of discussion). We excluded off-topic turns-of-talk in the analysis.
Scoring students pre–post assignment responses | ||
Correct answera | Observed student reasoning statementsb | |
Pre–post assignment | Compound ID 1.7 is the most effective at killing Salmonella. | Because… it has the lowest absorbance values it is similar to the + control/ampicillin it does not cause increased growth it has been tested by multiple individuals it provides consistent results it is outside 2 standard deviations of the + control |
Assignment 1 | Compound Gem is the most effective at killing Mycobacterium tuberculosis. | Because… it has the lowest absorbance values it is similar to the + control/ampicillin it has been tested by multiple individuals it is outside 2 standard deviations of the + control |
Assignment 2 | Compound CA is the most effective at killing the Salmonella. | Because…. it has the lowest absorbance values it is similar to the + control/ ampicillin it was tested in triplicate it is outside 2 standard deviations of the + control |
Assignment 3 | Compounds Faid and Bran are the most effective at killing the Mycobacterium. | Because… they have the lowest absorbance values they are similar to the + control/ampicillin they have reliable standard deviations they do not cause increased growth |
Assignment 4 | Combinational treatments W2 +Vet and W6+Vet are the most effective at killing the Salmonella. | Because… they have the lowest absorbance values they are similar to the + control/ampicillin they continue to cause death over time |
Qualitative Features
Coding Student Behaviors and Roles during Group Discussion.
Rather than attempting to use a previously developed coding scheme that might have forced us to apply a certain lens to our data, we started with an open-coding approach to capture whatever features were present in student discussions, using iterative content analysis (Saldana, 2015). During this emergent coding process, three coders read the same discussion transcripts and discussed the themes of students’ language. We noted many features seen in our previous work on student discussions (Knight et al., 2013) as well as features of argumentation and problem solving described by others (Toulmin, 1958; Erduran et al., 2004, Osborne, 2010; Prevost and Lemons, 2016). Because no previously published set of codes directly captured the behaviors we were interested in, we developed categories reflective of previous work, but tailored to our own students’ responses. Using three raters, we determined possible codes, discussing and revising, until we settled on 15 unique behavior codes. Using this final set, we coded the same subsets of discussion transcripts independently, adjudicating differences and continuing to iteratively refine the definitions of each code until we were satisfied. To establish reliability, all three raters then coded 10% of the remaining transcripts with an interrater agreement of 97% overall. We calculated interrater reliability for each code using Cohen’s kappa (Gisev et al., 2013). Kappa coefficients ranged from 0.49 to 1, with an overall average across all codes of 0.78, indicating substantial agreement (Viera and Garrett, 2005). We then coded the remaining transcripts individually.
To establish student roles, as no prior published roles fit our data set, we used an iterative process similar to what we described earlier, noting styles of interaction and typical individual behaviors during each discussion. In the first cycle of role development, we identified 14 possible roles, which we reduced to 10 after a second cycle of coding and discussion. For each student, we also tallied their different behaviors in each discussion and used the predominance of a particular behavior to help guide the selection of a role for this student. With additional qualitative review of each transcript, students were then assigned to individual roles. A.P., J.K.K. and K.G. assigned roles to students in 17 discussions, reaching greater than 80% agreement. Kappa coefficients, calculated separately for each role, ranged from 0.58 to 0.9, with an overall average Cohen’s kappa of 0.78, indicating substantial agreement. The remaining 74 discussions were coded individually.
Exchange of Quality of Reasoning.
To describe engagement in reasoning, we used the Exchange of Quality Reasoning scale (Knight et al., 2013; Table 3). This scale was developed from Toulmin’s components of argumentation (Toulmin, 1958) along with more recent work (e.g. Erduran et al., 2004) to generate a scale that privileged the participation of multiple students in developing an argument. As shown in Table 3, a level 0 discussion has no reasoning, while a level 3 discussion has more than one student exchanging reasoning tied to a claim with evidence (a “warrant”). We used the fine-grained behavioral coding scheme described earlier, tracking incidences of claims with reasoning, to give each discussion a score on the Exchange of Quality Reasoning scale. For this rating, all three coders rated all discussions, compared ratings, and reached consensus.
Levela | Definitionb |
---|---|
0 | No students made reasoning statements. |
1 | Only one student used reasoning, which could include a warrant (no exchange). |
2 | Two or more students exchanges reasoning, but neither or only one included a warrant. |
3 | Two or more students exchanged reasoning, including warrants. |
RESULTS
Students Show Improvement in Correctness and Reasoning from Pre to Post
Students were asked to complete an individual assignment at the beginning of the course and again at the end to be used as baseline and endpoint measures of their ability to analyze data and use reasoning; 45 of the 98 consenting students completed this assignment. Students were scored for correctness (0/1) on the individual pre–post assignment. For the students who completed both assignments, there was a significant increase in individual performance, with 47.8% of students answering the pre assignment correctly, and 97.8% answering the post assignment correctly (exact McNemar’s test, p < 0.001; Cramer’s V = 0.14; small effect size).
In addition to scoring correctness, we noted the number of reasoning statements, if made, in each student’s individual answer. For the students who completed both assignments, there was an overall significant increase in the number of reasoning statements from an average of 0.54 statements pre to an average of 0.85 post (Table 4A). Almost equal proportions of students never used reasoning or stayed the same in their use of a single reasoning statement. Twenty-six percent of students who showed an increase in reasoning did so by a single statement from pre to post (Table 4B). We further describe the types of evidence students used as reasons in Table 5. Of the 45 students who completed both the pre and post assignments, 23 provided the same piece of evidence to support their claims both pre and post, while about half either used different pieces of evidence or added more evidence to back their claims on the post. For example, Karah used the same evidence pre and post (comparing the absorbance of new compounds to the absorbance for the known antibiotic ampicillin) as her reason for choosing compounds as potential new antibiotics (a “hit”; Table 5A). On the other hand, Laura (Table 5B) used the positive control of ampicillin to explain her choice on the pre assessment and expanded her reasoning on the post assessment to include validating a compound as a hit, because it fell below 2 SD from DMSO, the chemical serving as the negative control. Similar to Laura, John further added in his post response that the results were reliable, because they were tested by multiple students.
A. The average number of reasoning statements used in the individual pre and post assignments for students who completed botha | |
---|---|
Pre | 0.54 (0.66) |
Post | 0.85 (0.07)* |
B. Change in number of reasoning statements pre to post | |
Never used reasoning | 23.9% |
One reason pre and post | 28.3% |
Increased | 34.8% |
Decreased | 13.0% |
A. Examples of students who used similar reasoning on both the pre and post assignments (n = 23) | |||
---|---|---|---|
Pre assessment reasoning statements | Post assessment reasoning statements | ||
Karah | Compound 1.7 may be a potential antibiotic for salmonella because it had a similar light absorbance to ampicillin, an antibiotic. | Karah | Compounds 4.2 and 5.3 might be hits because their absorbance values are close to that of ampicillin, the positive control. |
B. Examples of students who used different reasoning on the post assignment (n = 22) | |||
Pre assessment reasoning statements | Post assessment reasoning statements | ||
John | Ampicillin and compound 1.7 had low absorbance rates … The low absorbance indicates that the compound was able to kill more of the bacteria, leaving less bacteria behind to absorb the light. | John | I think that it is safe to say that compounds 4.2 and 5.3 are statistical hits, since they have a similar absorbance to ampicillin and would most likely fall below two standard deviations of the negative control (DMSO) if it were to be calculated. Since they were separately tested by four students, there are enough replicates for the results to be trustworthy. |
Laura | Any other compound that may be a successful antibiotic should have an absorbance rate after culturing close to that of ampicillin. Looking at the graph, the only compound with an absorbance close to that of ampicillin is compound 1.7. | Laura | Looking at compounds that may be hits, the way to determine if they are indeed hits is to find the mean of the DMSO and subtract two standard deviations from it. If the compound absorbance values are below this, they are hits. Since we don’t have this information, looking at the graph we can just guess that compounds 4.2 and 5.3 will be below this value, especially since their absorbance values are close to that of ampicillin. |
Individual Student Behaviors within Discussions
Table 6A shows the set of detailed codes we developed to describe natural (unprompted) student behaviors during group discussions. Discussions frequently started with students either making a claim or beginning to analyze data. During this time, students noticed which compounds served as controls in the experiment or what kind of data were being presented. This was commonly followed by further data analysis and claims, sometimes supported with a reasoning statement. Students occasionally described future possible extensions to experiments, usually near the end of their discussions. Most students engaged in a repetitive pattern of analysis and claims with minimal reasoning; only a few groups exchanged claims with multiple statements of reasoning. Overall, students most frequently used analysis in their discussions (18.2%), followed by noticing or recalling information (9.7%) and making claims (9%; Table 6B).
A. Code definitions and frequenciesa | ||
---|---|---|
Code | Definition | Average % of turns of talk per discussion |
Analyze | Using numbers or direct reference to the graph | 18.2 (0.1) |
Recall | Pointing out or recalling information needed to solve the problem | 9.7 (0.1) |
Repeat | Restating something that was already stated previously. Clarifying by restating. | 9.3 (0.1) |
Claim | Stating answer to applicable problem | 9.0 (0.1) |
Extend | Suggesting further analysis of experiments | 5.0 (0.1) |
Reason | Defending a claim/statement/idea with a reason connected to evidence | 4.8 (0.1) |
Question | Asking a question in order to better understand. General statements of confusion. | 4.0 (0.1) |
Affirm | Making statements of agreement | 3.7 (0.1) |
Focus attention | Reading questions or asking to move on | 2.1 (<0.1) |
Teach | Explaining something to another student | 2.1 (<0.1) |
Drive discussion | Keeping discussion on track. Asking questions of others to facilitate discussion | 1.6 (<0.1) |
Agree | Reaching a final consensus after disagreement | 0.7 (<0.1) |
Disagree | Stating disagreement with a claim/statement/idea | 0.7 (<0.1) |
Divert | Pulling attention away from the main content of the question | 0.7 (<0.1) |
Other | Off-topic | 26.3 (0.2) |
aMore than one code could be used to describe each turn of talk. The frequency of each behavior was calculated as a percent turns of talk for each discussion, then averaged across the 91 discussions. On average, discussions contained 21.3 (±10) turns of talk. Standard Deviation is shown in parentheses. | ||
B. Transcript from one group discussion, with associated codes for each turn of talk | ||
Student | Dialogue | Code |
Kelly | The negative control is DMSO. | Recall |
Jessica | The negative control is DMSO and ampicillin is the positive control because ampicillin shows low average absorbance and DMSO shows a high average absorbance. | RecallAnalyze |
Samuel | So it initially looks like the compounds, Faid and Bran maybe were like the best ones at killing the mycobacterium um, because as shown in the graph, they had the least amount of absorbance. They were the most similar to ampicillin after the incubation period. | ClaimReason |
Heather | Regg had the highest absorbance; it was even higher than the DMSO. So that would prove to not be a good potential compound to killing the bacteria, and ... | ClaimReason |
Kelly | Um and because each compound was tested in triplicate by two students, like that’s pretty good. But for the ones that showed that they were hits, that they were low, they should probably be tested a few more times just to be sure that they are killing the bacterium. | Extend |
Group Composition
Students self-selected their groups, limited only by who else was in their lab section. We describe groups as homogeneous or heterogeneous in terms of gender and race/ethnicity (Table 7). The majority of students chose to form groups that were heterogeneous for race or gender (60% for race; 63.3% for gender); 37% of these were heterogeneous for both. When students formed homogeneous groups, they were usually all white (11 of the 12 homogeneous race groups) and all female (9 of the 11 homogeneous gender groups).
Heterogeneous for gender | 19 | ||||
Homogeneous for gender | 11 | All male | 3 | All female | 9 |
Heterogeneous for race/ethnicity | 18 | ||||
Homogeneous for race/ethnicity | 12 | All white | 11 | All BIPOC | 1 |
Heterogenous for both | 11 | ||||
Homogenous for both | 3 | All White | 3 | All Female | 3 |
Total groups | 30 |
Exchange of Quality Reasoning Varies by Group
Using the Exchange of Quality Reasoning scale (Table 3), we characterized the use of reasoning during each discussion (Figure 4A). Most discussions (72%) did not use reasoning (level 0) or had reasoning by only one student (level 1). Some discussions contained exchanges of reasoning statements without directly tying their reasoning to a claim (level 2: 18%), and only 5% of all discussions reached level 3, in which at least two students tied their claims directly to the supporting evidence with reasoning. Over the course of the four discussion assignments, group reasoning scores varied significantly (going either up or down), but on average, most groups stayed at level 1. Of the 30 groups, 14 always engaged in low-quality discussions, two always engaged in high-quality discussions, and 14 fluctuated between higher- and lower-quality discussions (Figure 4B).
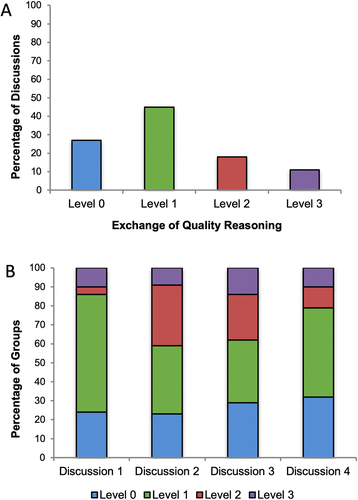
FIGURE 4. Distribution of Exchange of Quality Reasoning scale scores. (A) The majority of group discussions had low-quality reasoning (level 0 or 1) across all assignments, with only 5% reaching level 3. (B) Using a chi-squared test for association, groups vary significantly across the four discussions; χ2(9, N = 91) = 41.849, p = 3.502e-06; Cramer’s V = 0.18; small effect size.
Figure 5 shows two different discussions that represent typical examples of low reasoning versus high reasoning. In the level 0 discussion (Figure 5A), students focused on extracting information about the data presented in the graph. They primarily listed what they observed rather than answering the question using claims and reasoning. They made a single claim: “W6 plus Vet really seems to improve the compounds,” but provided no justification for this claim, failing to connect it to evidence presented. The students engaged in thinking about future directions and made comments on the quality of the data, but never circled back to a final conclusion. In contrast, a different group (Figure 5B) made multiple claims along with justifications for each of these claims. Altogether, they made a total of four different claims and a final conclusion statement with justification. Students in this example also engaged in analysis, but ultimately focused on drawing a conclusion with supporting evidence.

FIGURE 5. Example transcripts of low and high Exchange of Quality Reasoning discussions. A. A low-level discussion in which students primarily list observations about the data, discuss future experiments, and do not make a clear claim. B. A high-level discussion in which students make a variety of claims with justifications.
As shown in Table 8A, the frequency of specific behaviors was clearly different between groups who engaged in high- quality reasoning versus low-quality reasoning. In high-quality discussions, students made claims and provided reasoning at significantly higher frequencies than students in low-quality discussions. On the other hand, students in low-quality discussions used analysis at a significantly higher frequency than those in high-quality discussions.
A. Prevalence of specific behaviorsb | |||
---|---|---|---|
Behavior | Low | High | |
Claim | 8.1% | 11.9%** | |
Analyze | 20.8%** | 13.2% | |
Reason | 2.9% | 9.7%** | |
B. Prevalence of common rolesc | |||
Roles in low-quality discussions | Roles in high-quality discussions | ||
Analyst | 36.2% | Reasoner | 22.6% |
Generalist | 19.3% | Generalist | 22.6% |
Minimalist | 18.3% | Minimalist | 17.2% |
Reasoner and Analyst | 7.8% | Reasoner and Analyst | 16.1% |
Reasoner | 6.4% | Analyst | 11.8% |
Differences in group composition (Table 7) can also affect the level of reasoning. For instance, we observed that groups heterogeneous for gender had significantly higher-quality discussions than homogeneous gender groups of either all male or all female (p < 0.01, two-sample t test; Cohen’s d = 0.84; large effect size). On the other hand, there was no difference in quality of reasoning between groups that were homogeneous versus heterogeneous for race/ethnicity (Figure 6).

FIGURE 6. Group Heterogeneity and Quality of Reasoning. Average Exchange of Quality Reasoning scores for groups that were homo- or heterogeneous for either gender or race/ethnicity; n = 30 groups. Students in heterogeneous groups in terms of gender showed significantly higher scores (**p < 0.01, two-sample t-test; Cohen’s d = 0.84; large effect size.
Finally, despite variations in their exchange of reasoning, groups overall performed similarly on all assignments, with a range of 79–87% of groups providing a correct answer for assignments 1–4. A chi-square test of independence showed that there were no significant differences in performance across the four group assignments.
Students’ Behaviors Classified Them into Roles
Using the codes ascribed to each individual, we established roles that captured the characteristics of an individual’s behaviors during discussion. Of the 10 roles we identified, four were most common: analyst, reasoner, generalist, and minimalist (Table 9). A minimalist was defined as someone who spoke only once or twice in a discussion and did not contribute meaningfully to completing the assignment. The other common roles are presented with examples.
Role | Definition | Number of times individuals take on a role (%) | Number of groups that included a role (%) |
---|---|---|---|
Analyst | Interprets data by describing tables or graphs, and thinks of alternative interpretations or experiments | 129 (36) | 75 (82) |
Reasoner | Explains the reasons behind a claim; justifies the final answer; may also make claims and notice | 72 (20) | 53 (58) |
Generalist | Engages in a variety of behaviors in a relatively equal mix; does not provide any major contributions | 60 (17) | 44 (48) |
Solver | Only makes claims without any reasoning | 14(4) | 13 (14) |
Observer | Only notices or recalls information | 7 (2) | 6 (7) |
Discussion driver | Promotes conversation by focusing attention of group members and driving the discussion forward | 5 (1) | 6 (7) |
Affirmer | Rewords previous claims or makes statements of agreement | 5 (1) | 6 (7) |
Knowledge facilitator | Drives development of understanding by teaching others | 5 (1) | 2 (2) |
Questioner | Asks clarification questions or requests explanations | 2 (>1) | 1 (1) |
357 total individual roles | 91 total group discussions |
The role of analyst was well illustrated by Andrew. Andrew primarily analyzed data, including describing the graphical data presented and identifying the percent survival of each strain. Andrew stated:
A lot of the resistant tuberculosis survived but not a lot of harmless Mycobacterium survived. But with the cyanide practically nothing survived … DMSO, Nox, and Zar had at least 80% survival in the resistant strain … Gem only has 20% survival in the resistant strain … Gem is definitely more successful than Nox and Zar.
During a different discussion, Rakel took on the role of reasoner, responding to the question prompt with:
You can’t use AC [...] as a for sure hit because you only have one trial. Then the next one we looked at that could possibly be a hit was CA […], that one looks like the most reliable because it has very consistent ampicillin values and it has consistent DMSO values and the drug. It’s kind of off a little bit, but for three trials it’s pretty good. For BB, yah, for BC too, um, […] similar ampicillin and similar DMSO, and that’s kind of the same thing with CB. These ones are not hits, but, um, are more reliable data.”
Rakel used a series of “because” statements when referring to the reliability of the data for compound AC and again when she explained why CA could be a hit by comparing it to the values of the controls. The use of these statements qualified Rakel as a reasoner: someone who made multiple claims with justification statements.
The role of generalist was defined as one who engaged in multiple different behaviors, with no clear theme. For example, Gina asked a question: “How can cyanide be a control?”; then made a claim: “The least effective one would be this one [referring to the drug Mas]”; later analyzed data: “the resistant strain looks like it especially resistant to the ampicillin”; and finally, drove the discussion: “Do you want to say more?” Her varied contributions are typical of those classified as generalists.
Several additional less-common roles also provide insight into student behaviors. A knowledge facilitator was someone who engaged in teaching other students by helping them understand the data. This role is demonstrated by Noel’s dialogue in response to a question about a control:
So, the DMSO, essentially, the point of it, by testing DMSO on its own you know that it is not actively harming any bacteria that it is in because then you wouldn’t know how efficient the actual compound is. So, in a sense cyanide, ampicillin, and DMSO are all controls, ‘cause ampicillin you want to know actually works on a non-resistant strain and that it no longer works on a resistant strain.
The role of driver was the least common (only five instances total). Students who took on this role engaged in leadership, in which they focused on asking questions of others to direct the conversation forward. For example, Jess served as a driver when she attempted to refocus the attention of her group members to the problem by requesting information through a series of statements: “[Does] anyone want to make a claim?” and “Does anyone want to mention Mas or Bran?”
Some Students Show Preference for a Specific Role, but Many Change Roles
We grouped students into three categories by their likelihood to maintain the same role over the four discussions. Although we use the term “preference” for these categories, there is no evidence that students are conscious of their role choices. In the “no preference” category, students took on a different role for each discussion; in “some preference,” students took on the same role for more than 50% of the discussions; and in “strict preference,” students always took on the same role for all discussions in which they participated (Table 10A). Among the students who showed a role preference, the most common was analyst (Table 10B).
A. | Role selectiona | Percent of students (n = 109) | |||
---|---|---|---|---|---|
No preference | 49.2% | ||||
Some preference | 27.4% | ||||
Strict preference | 23.4% | ||||
B. | Roles students chose to assume when they had strict role preference (n = 29) | ||||
Reasoner | Analyst | Minimalist | Other | ||
6 | 14 | 6 | 3 | i.e., driver, affirmer, observer |
Although it was common for students to vary their roles, it is noteworthy that, in some cases, students appeared to change their roles in response to the absence of a formerly dominant individual. We defined dominators as students whose participation index was greater than 50%, where the participation index is student’s total minutes spent talking/total time of discussion. There were dominators in 19 of 91 discussions. In most cases, this behavior was exhibited by different individuals in different groups, and by both males and females equally. However, in four groups, a single individual (a male in three cases, a female in one case) consistently exhibited dominant behavior. In each of the instances when this dominant individual was present, they contributed most or all of the arguments used by the group to draw conclusions. Thus, all of those discussions were coded as a 1 on the Exchange of Quality of Reasoning scale. However, when the dominant individual was not present in a particular discussion, as occurred on two occasions for two different groups, the remaining students increased their use of reasoning, with one group reaching a level 2 and the other, a level 3 on the Exchange of Quality of Reasoning scale. This change is represented by Nathan’s group, shown in Figure 7. Nathan generally dominated the discussion, as seen by his introductory statement:
Cyanide is the control that they used in this experiment and definitely has the lowest survival in both the harmless mycobacterium and the resistant tuberculosis strain, but that one you are not able to use. It is not an option for human treatment. Gem looks really promising, it is less than 20% for both the harmless mycobacterium and the resistant tuberculosis strain over 7 days.
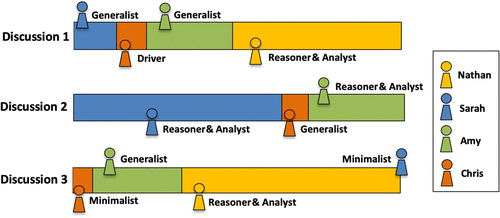
FIGURE 7. Roles and contributions can change across discussions. The roles taken by different members of a single group over three discussions (students were absent for the fourth discussion). The participation index for each student is shown as a bar and is labeled with their respective role(s).
Here, Nathan acted as a reasoner and analyst, noticing components of the question, analyzing data, making a claim, and justifying his claim by providing data to support it. Perhaps in response to the strength of his statements, the other three group members took on supporting roles of generalist (two group members) and driver (one group member) in both discussions 1 and 3. However, when Nathan was absent in discussion 2, the student interactions were different. The other students now took on the roles Nathan had previously performed, and all contributed equally to the discussion. For example, Sarah now took on the role of the reasoner, in which she made a claim and then justified her choice by comparing the compound she chose to the control:
What we see is AC is the most effective. It is even more effective than ampicillin … The least effective was AA. The ampicillin and DMSO looked pretty even for that so I would say that is actually not too effective.
Chris also took on the role of reasoner by making similar statements:
Yah, the only drugs I see that were effective, like way below the DMSO, were AC, BA, and CA […]. For CA, the data is pretty consistent so I would say that is probably the best drug of the bunch.
These examples illustrate that a dominator can negatively affect a group’s exchange of reasoning, but that students can also shift their roles in the absence of a dominator.
DISCUSSION
Limitations
We collected data in the form of written individual responses and recorded group discussions across four different time points in the context of a CURE laboratory course. These data allowed us to draw conclusions about how students in this specific environment interacted with one another when completing data-analysis assignments. However, students in different laboratory environments, or even the same laboratory environment but with different group assignments, may choose to behave differently.
Our study was also limited by sample size and relative homogeneity. Although we were able to gather rich qualitative data from recorded group interactions, our sample did not allow us to determine the statistical significance of all of our observations or explore all possible questions pertaining to gender and race/ethnicity.
Finally, the individual pre–post assignment and the group discussion assignments were graded for participation rather than for correctness. The purpose of this was to capture ideas and more organic discussion with less pressure of reaching a correct conclusion; however, students may have chosen not to participate (as in the case of the post assignment) or participated with less organization and effort during the in-class assignments if they were not motivated to perform to the best of their ability. Thus, we may not have captured students’ full capacity for collaboration and reasoning.
Students Need Explicit Instruction in Reasoning
Science process skills like data analysis and reasoning are an important part of scientific endeavors such as solving problems, designing experiments, and communicating results (DebBurman, 2002; Dirks and Cunningham, 2006; Lubben, 2009; Coil et al., 2010). These skills are not intuitive for most students, and the act of providing reasoning during problem solving is difficult for students at all levels (Kitchen et al., 2003; Erduan et al., 2004; McNeill and Krajcik 2008). Past efforts to improve students’ science process skills have suggested that practice and hands-on laboratory experience foster an environment that allows for the development of reasoning skills (Kanari and Millar, 2004; Kitchen and McDougall, 1999).
In this study, we found that, despite being in a laboratory environment, students focused primarily on analyzing data without using reasoning. We measured their exchanges of reasoning as one indication of their ability to be collaborative, finding that only 53.2% of student claims were backed by reasoning, and only 58% of groups contained a reasoner (Table 9). This shortage may indicate that the data presented to students were not complex enough to draw out reasoning (Paulus, 2005) and/or that students were not comfortable providing evidence to support the claim(s) they were making (Erduan et al. 2004; McNeill and Krajcik, 2008). Students may easily recognize evidence but may not understand how to use evidence in constructing reasoning (Sampson et al., 2011; Zembal-Saul et al., 2012). If they do not understand what constitutes a reason in a science setting (McNeill and Krajcik, 2007; Sandoval and Reiser, 2004), they are likely to provide descriptions of data rather than explanations (Driver et al., 2000; Sandoval and Millwood, 2005; McNeill et al., 2006). From the observations we collected, it seems likely that students are unsure about what qualifies as reasoning. In several instances, students state that they are done with the assignment, because they have already supplied reasoning, when in fact they have only made a series of claims and observations. Thus, there is a disconnect between students’ perception of providing reasoning and the actual use of reasoning as defined by instructors.
Previous studies (Johnson et al., 1991, 1993; Johnson and Lawson, 1998; Krejins et al., 2003; Tanner et al., 2003; Osborne, 2010; Premo et al., 2018) have also shown that putting students into groups is not necessarily sufficient to generate collaboration. Our findings support this observation: most of the students in our study participated in group work without engaging in the interactive or constructive ways promoted by Chi and Wylie (2014) that would transition them from simple explanations to generative thinking that includes reasoning. Thus, it seems clear that, even in a group laboratory setting, where students are theoretically free to explore their ideas and ask one another questions, they still need explicit instruction in how to use reasoning, from what reasoning is to how to implement it during data analysis and discussion, and frequent reminders to employ such reasoning.
Heterogeneity is Beneficial for Group Discussions
Previous studies have shown that an individual’s gender may influence his or her participation (e.g. Tolmie and Howe, 1993). In one study of college students, males often reported taking on leadership roles during group discussions, while female students preferred to be collaborators (Eddy et al., 2014). Similarly, in a study of South African middle school students, males took on a more authoritative approach to the problems, and females focused more on democratic approaches and reaching consensus (Lubben, 2009). In the current study, mixed-gender groups had relatively equal participation (measured by turns of talk), and, overall, an equal number of males and females took on dominating roles in discussions. Nonetheless, when a group consistently had a dominator, it was a male in three of the four groups. These results support the previous findings that males and females may interact differently in their groups, potentially depending on the makeup and dynamic of the group. We also found that mixed-gender groups reached higher-quality of reasoning levels than homogeneous gender groups (Figure 6A), although some homogenous (all female) groups were also highly collaborative. Due to the strength of the effect size for the higher reasoning levels of heterogeneous gender groups, instructors may wish to monitor the makeup of groups and encourage students to form, or assign students to, mixed-gender groups. By doing so, instructors can promote equity and an environment more conducive to sharing reasoning.
As far as racial and ethnic makeup of groups, prior studies have shown a positive impact of heterogeneity in these cases as well, demonstrating the value of having various opinions and perspectives to generate novel solutions (Howard et al., 2002; McLeod and Lobel, 1992). In the current study, we did not observe any differences between the ways in which racially heterogeneous groups engaged compared with racially homogeneous groups (Figure 6A). However, because the majority of students were white (Table 1) and many groups were homogenous with respect to race/ethnicity, there were likely not enough examples to observe potential benefits. Thus, we still strongly support promoting heterogeneous racial and ethnic groups when possible.
Students’ Natural Role Choices Are Not Necessarily Optimal
Assigned roles, such as manager, presenter, recorder, and checker from POGIL, focus primarily on the format of discussion rather than the exchange of scientific ideas. Such organizational roles are clearly helpful, especially for long group discussions or long-term projects (Moog et al., 2006; Moog and Spencer, 2008). Students in the current study, who engaged in shorter discussions only four times over the semester, did not naturally adopt any of these roles except, rarely, the role of driver (most similar to the POGIL role of manager). This suggests that when students are unguided with regard to role selection, they are likely to engage in the quickest path to content understanding, preferentially taking on the role of analyst rather than roles that are focused on organization and/or equity of participation.
In addition to not taking on organizational roles, students often switched their roles from one discussion to the next: in fact, 40% switched roles in each discussion (Table 10). However, this malleability may have benefits. For example, students may change roles if they become more confident with content or more comfortable with the other students in the group, allowing them to shift from a more supplemental role such as generalist or minimalist to a more critical role like reasoner (Figure 8). Students may also change roles to compensate for a missing group member who is usually more vocal or dominant in the discussion (Figure 7), consciously or unconsciously reacting to the needs of the group. Similarly, a student may choose to shift from monopolizing the discussion to playing a minimalist role, thus allowing a different member of the group to use new skills. All of these shifts, even if unconscious, support the social cognitive theory of learning: that students generally do not act solely from an individualistic perspective, but rather are attuned to their group members and the social nature of learning. Nonetheless, given students’ overall narrow preference for the role of analyst, instructors would be well served to help guide students into roles that promote deeper thinking.

FIGURE 8. Distribution of observed student roles for each of the four group discussions. The percent of students who took on each role is shown for each discussion. According to a chi-squared test for association, the groups are not significantly different from one another χ2 = (12, N = 91) = 17.51, p = 0.1267. Across the four discussions, the distribution of student roles differed slightly, although not significantly/ Although the role of Analyst was always the most prevalent, fewer students were classified as Generalists and more as Reasoner after Discussion 1.
In addition, we suggest that some of the less commonly enacted roles of driver, knowledge facilitator, and questioner might be adopted by more students if they were aware of the value of such roles and more conscious of the social dynamics of their groups. A student who is aware of the benefits of cueing other members of the group to use reasoning (Knight et al., 2013) may be able to make such suggestions, stimulating argumentation within the group. Similarly, a knowledge facilitator, essentially acting as an instructor, can act to promote better discussion by engaging with others to help everyone explain their own ideas more fully (Webb et al., 2002; Beichner, 2007; Jensen and Lawson, 2011; Knight et al., 2015). Finally, the role of questioner can also stimulate more thorough understanding and exploration. By asking one another “why” or “how” they reached their conclusions, or even expressing statements of confusion, students can elicit responses from one another that stimulate a conceptual explanation and the use of reasoning.
APPLICATIONS FOR INSTRUCTION
We end with several suggestions that instructors can implement when students are engaged in group work, particularly if the group work is long term and intended to be generative.
Promote Specific Behaviors and Roles to Fully Engage Students in the Social Aspects of Learning
Providing examples and explanations of productive behaviors and specific roles may aid students in realizing there is value in working collaboratively. If students have an understanding of how to act as a reasoner, driver, knowledge facilitator, and questioner, and how these behaviors can create collaboration and interaction, they may be more likely to choose to engage in these roles. One way to promote this understanding would be to have students practice taking on a set of specific roles to accomplish a group goal and then discuss how each role helped to create a positive social dynamic as well as to construct a solution. This exercise could help students learn beneficial group behaviors without requiring them to step into preassigned roles. If students further practice switching roles and again discussing how their perspectives changed when they adopted different roles, they may feel more confident in adopting more than one beneficial role under unassigned conditions.
Promote Argumentation
Students need better tools to help them focus more on generating and exchanging reasoning while engaged in discussions, as they more naturally engage in explanations and analysis than in reasoning (Zeidler, 1997; Walker et al., 2012; Walker and Sampson, 2013; this study). One example from the literature that could be useful is argument-driven inquiry, a multistage instructional model for improving argumentation (Poock et al. 2007; Schroeder and Greenbowe, 2008; Walker and Sampson, 2013; Walker et al., 2012, 2019). Another less formal approach would be to provide instructions that explicitly prompt students to focus on exchanging reasoning in support of developing an argument. We suggest that a combination of verbal and written cues need to be given to students on a regular basis to establish the practice of reasoning. If students are not fully aware of how to connect evidence to claims or how to recognize whether others are using reasoning, they need to explicitly practice these skills and receive feedback from one another and instructors. After such practice, reminders to use the principles of argumentation may be enough to promote collaboration and complete rationales to support their claims. Implementing such tools and others will require further study.
ACKNOWLEDGMENTS
Thank you to Kestrel Green for his assistance in coding recorded discussions, to Lisa Corwin for advice, to members of the Corwin and Knight labs for feedback, and to the students who participated in these studies. This work was funded by a grant from the National Science Foundation (DUE 1711348).