Why Students Struggle in Undergraduate Biology: Sources and Solutions
Abstract
Students’ perceptions of challenges in biology influence performance outcomes, experiences, and persistence in science. Identifying sources of student struggle can assist efforts to support students as they overcome challenges in their undergraduate educations. In this study, we characterized student experiences of struggle by 1) quantifying which external factors relate to perceptions of encountering and overcoming struggle in introductory biology and 2) identifying factors to which students attribute their struggle in biology. We found a significant effect of Course, Instructor, and Incoming Preparation on student struggle, in which students with lower Incoming Preparation were more likely to report struggle and the inability to overcome struggle. We also observed significant differences in performance outcomes between students who did and did not encounter struggle and between students who did and did not overcome their struggle. Using inductive coding, we categorized student responses outlining causes of struggle, and using axial coding, we further categorized these as internally or externally attributed factors. External sources (i.e., Prior Biology, COVID-19, External Resources, Classroom Factors) were more commonly cited as the reason(s) students did or did not struggle. We conclude with recommendations for instructors, highlighting equitable teaching strategies and practices.
INTRODUCTION
Many students who enter higher education with the intent to pursue a career in the biological or biomedical sciences abandon this goal because they struggle in introductory “gatekeeper” science courses (Gainen, 1995; Gasiewski et al., 2012). Promoting student retention in science, technology, engineering, and mathematics (STEM) is essential to our national efforts to produce graduates who meet the growing need for a trained and diverse workforce (President’s Council of Advisors on Science and Technology, 2012). This goal may be addressed through mitigation of student struggle in STEM courses, because struggle can significantly undermine students’ academic abilities and performance (Batz et al., 2015; England et al., 2017). While the term is ill-defined in the literature, internal and external factors can contribute to students’ experiences of struggle.
Internal Factors
Academic success and struggle are influenced by students’ content knowledge as well as a host of internal, or affective, factors (Trujillo and Tanner, 2014). One influential internal factor is student mindset when encountering struggle (Dweck, 1999, 2006), which has been shown to contribute to performance outcomes (Yeager et al., 2019). Additionally, previous work shows that students’ beliefs about the degree to which intelligence is a stable trait is an influential factor impacting student struggle (Dweck, 1999; Limeri et al., 2020). Specifically, Dweck (1999) found that students who perceived intelligence as an unchangeable trait, or an innate ability, were more likely to interpret struggle as an indication that they were not intellectually capable of success. However, student abilities are not fixed, because individuals’ mindsets develop and change throughout their lives (Aronson et al., 2002; Yeager et al., 2019). To address how mindset changes over time and how it can be influenced by STEM course work, Limeri et al. (2020) used latent growth modeling to demonstrate that students who reported they had struggled in a course also increasingly viewed intelligence as an unchangeable trait over a semester.
Other internal factors that may cause students to perceive struggle in relation to their undergraduate biology education include study habits, motivation, self-determination, and grit (passion and perseverance), as well as sense of belonging in STEM (Johnson et al., 2007; Cromley et al., 2016; Ye et al., 2016; Flanagan and Einarson, 2017; Ryan and Deci, 2017, 2020; Sithole et al., 2017; Dyrberg and Holmegaard, 2019; Sheshadri et al., 2019; Wilton et al., 2019; Meaders et al., 2020).
External Factors
Sources of struggle can also be due to external factors beyond students’ control. External factors that may cause students to perceive struggle include course format (e.g., regular use of undisrupted lecture or unstructured group work); reliance on high-stakes exams to evaluate students; and large class sizes, particularly in introductory courses (Armbruster et al., 2009; Gasiewski et al., 2012; Freeman et al., 2014; Ballen et al., 2017; Corkin et al., 2017; Scott et al., 2017). Two other external factors contributing to students’ perceived struggle are previous educational experiences and access to resources, which play a central role in student incoming academic preparation for higher education and overall success in entry-level college courses (Freeman et al., 2007; Salehi et al., 2019, 2020).
We include incoming preparation as an external factor to emphasize its reflection of the opportunity gap in primary and secondary education. For example, access to high-quality curricular materials, evidence-based instruction, and technology all contribute to gaps in academic preparation that are subsequently reflected in student grades in introductory courses that fail to provide students with equal opportunities to demonstrate proficiency in course content knowledge (Salehi et al., 2019, 2020). Specifically, several analyses of performance outcomes in STEM at multiple institutions revealed that differences in student performance appeared to be due to differences in Scholastic Aptitude Test (SAT), American College Test (ACT), and pre-semester concept inventory scores (Salehi et al., 2019, 2020). Thus, we may predict a strong relationship between measures of incoming preparation, course performance, and perceptions of struggle. To our knowledge, this relationship has not been studied in undergraduate biology.
While incoming preparation is often linked to performance outcomes, it has also been well demonstrated that some instructional practices and classroom factors can expand or reduce (or eliminate!) incoming gaps in academic preparation. For example, instructors who use evidence-based and equitable teaching strategies can decrease performance gaps in undergraduate STEM courses (Freeman et al., 2007; Ballen et al., 2017; Cotner and Ballen, 2017; Salehi et al., 2020; Theobald et al., 2020).
Finally, a unique potential source of external struggle for students in Spring 2020 was the emergency transition to remote learning amid the COVID-19 pandemic. At its onset, higher education institutions required students to shelter in place for an indefinite period of time, functionally cutting students off from essential learning resources on campus. The transition to emergency remote learning dramatically impacted how students prepared for and took exams (Driessen et al., 2020a; Beatty et al., 2022), participated in the classroom setting (Ali et al., 2020; Wester et al., 2021), and developed social relationships with both peers and instructors (Smoyer et al., 2020; Supriya et al., 2021; Wut and Xu, 2021). The transition to remote learning also created practical challenges for faculty, many of whom resorted to asynchronous online videos or a combination of asynchronous and synchronous work, with little to no instructor–student interaction (Supriya et al., 2021; Wolinsky, 2021). While pedagogical decisions were the result of dire circumstances, the impacts of those decisions were still consequential to student learning and, potentially, perceptions of struggle in the course.
Research Questions
The emergency transition to remote learning amid the COVID-19 pandemic created an opportunity to study ways in which students attribute their success and struggle in biology during one “typical” semester and one in which a significant disruption took place. Importantly, we did not define struggle for the students, but rather used their open-ended responses and course performance to better understand their views/interpretations of struggle while addressing the following research questions:
RQ1: (a) What factors influence struggle in introductory biology? (b) How do performance outcomes correlate with students encountering and overcoming struggle in introductory biology?
RQ2: (a) To what do students attribute their struggle (or lack of struggle) in introductory biology? (b) What sources of struggle were students most likely to overcome?
While previous literature provided several factors influencing student experiences and performance, student struggle is ill-defined in the literature. Our study expands on this body of literature by asking students to define their struggle, providing a holistic view of student challenges in undergraduate biology. We take this further by identifying sources of struggle that students are most commonly able to overcome. Through examination of sources of struggle that students are most likely to overcome, we are able to make instructor recommendations that can potentially have a significant, rapid impact on students within a course.
METHODS
Courses and Instructors
We examined data from a survey of 965 students across three different introductory biology classes (hereafter Courses 1–3 in order of sequence) with six different sections during the Fall 2019 and Spring 2020 semesters (Table 1). We consider these courses introductory because they are taken by mostly first- and second-year undergraduate students as prerequisites for upper-level biology courses. Participating courses were offered by the biology department at a large, primarily white public research university in the southeastern region of the United States. Enrollment for each lecture section ranged from 159 to 376 students. The courses surveyed were taught by five instructors, who varied in terms of teaching experience and demographics, but all instructors used active-learning components. Two of the five instructors co-taught, however as they did not teach any other course included in this survey independently we include them as a single instructor in Table 1 and in subsequent analyses to maintain anonymity of the instructors. Following Driessen et al. (2020b), we define active learning as “an interactive and engaging process for students that may be implemented through the employment of strategies that involve metacognition, discussion, group work, formative assessment, practicing core competencies, live-action visuals, conceptual class design, worksheets, and/or games” (p. 6). The specific strategies instructors employed varied, ranging from flipped classroom to group work with iClicker questions. Instructor teaching experience varied between 0 and 18 years.
Section | Instructor | Semester | Course number | Participating studentsa | Total students |
---|---|---|---|---|---|
1 | 1 | Fall 2019 | Course 1 | 205 | 327 |
2 | 2 | Fall 2019 | Course 1 | 107 | 159 |
3 | 2 | Fall 2019 | Course 1 | 135 | 260 |
4 | 4 | Spring 2020 | Course 2 | 172 | 237 |
5 | 4 | Spring 2020 | Course 2 | 137 | 194 |
6 | 3 | Spring 2020 | Course 3 | 209 | 376 |
Data Collection
Data collection and research was approved by Auburn’s Institutional Review Board no. 18-349 EP 1811. Before data collection, we recruited instructors via email and other personal communications. From the consenting instructors’ classes, we collected data from their enrolled undergraduate students through the use of an online survey created in Qualtrics (Supplemental Table S1). We posted the survey on the Canvas page for each of the participating classes during the last week of the semester for the Fall 2019 and Spring 2020 semesters. Each instructor made students aware of this optional survey and offered bonus points for participating. Students either did not participate, participated by taking the survey and consenting as a participant, or participated by not consenting to taking the survey through Qualtrics. We used only the data from the consenting participants. At the end of the semester, we collected institutional information for students enrolled in the six classes participating in this study. This information included student grades, ACT and/or SAT scores, high school grade point average (GPA), college GPA, sex (i.e., male or female), and race/ethnicity as obtained from the Office of Institutional Research.
The survey instrument opened with an informational letter to participants detailing the purpose of the study. The survey then prompted students to either consent or not consent before moving on to several research questions. The remainder of the survey collected demographic information (e.g., gender identity and race/ethnicity) and open-ended responses to the following questions: 1) “Did you encounter struggle in introductory biology this semester?,” and if so, 2) “Were you able to overcome struggle that you encountered?” (Supplemental Table S1). The survey questions used in this research were previously developed and implemented with 875 students in an Organic Chemistry II course as detailed in Limeri et al. (2020). Students followed logic questions, an advanced survey option that allows the creator to include “rules” within the survey (L. Limeri, personal communication, September 8, 2020). By implementing logic, students advanced to further questioning that was specific to their earlier responses (Figure 1). We operationalized perceived struggle using two “yes/no” questions, ensuring our interpretations of student responses to these questions accurately reflected their experiences by presenting our interpretation to students and asking them to comment on it in a constructed-response question. For example, students who answered “no” to the first question were then asked, “Your response suggests that you did not encounter struggle in [biology course] this semester. Please explain why this is or is not an accurate description of your experience.” Written responses to these questions were reviewed during the qualitative coding process (see RQ2:Qualitative Analysis), and any written responses that contradicted the binary yes/no responses were removed from the analysis.
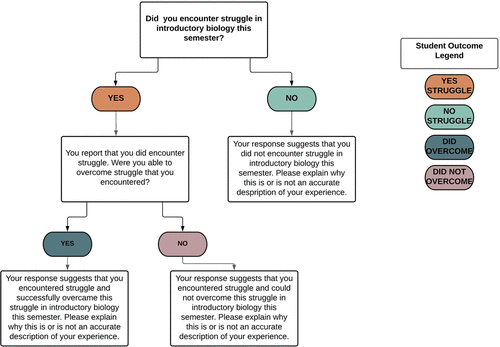
FIGURE 1. Logic question progression based upon student responses to the question: “Did you encounter struggle in introductory biology this semester?” The resulting responses led to the following student outcomes: 1) No, I did not struggle, or 2) Yes, I did struggle. Students who indicated “yes” were then asked: “Were you able to overcome struggle that you encountered?” The resulting responses led to the following outcomes: 3) No, I did not overcome my struggle, or 4) Yes, I did overcome my struggle.
Of the 1453 students enrolled, we received 965 total survey responses, with 130 students taking the survey both in Fall 2019 and Spring 2020 for different courses and three students taking the survey twice in the same semester for different courses. In the total data set of 965, five responses were removed, as their open-ended responses contradicted their binary responses (e.g., students selected no, indicating they did not experience struggle, but they then went on to write responses suggesting they did struggle). The remaining 960 responses were used in our quantitative analysis. Of the 960 responses from the quantitative data set, only 745 responses contained complete open-ended qualitative responses. Of the 745 responses, 38 were discarded because their open-ended responses could not be confidently coded. This left us with 707 student responses for our qualitative analysis. A portion of the 707 entries with open-ended qualitative responses included duplicate responses by students in different courses (82 students; see Limitations section for more information). For a summary of the demographics of the enrolled and participating students from the cumulative six sections, see Supplemental Table S2.
RQ1: Quantitative Analysis
RQ1A. What Factors Influence Struggle in Introductory Biology?
First, we were interested in the factors correlated with struggle in introductory biology courses. We focused on how Incoming Preparation (i.e., access to resources), Instructor, and Course were correlated with struggle.
We used a principal component analysis (PCA) to collapse two measures of Incoming Preparation into one variable. Incoming Preparation measures include a standardized test score (ACT scores or SAT scores converted to ACT scores) and high school GPA. SAT scores were converted to ACT scores following the ACT/SAT concordance tables provided by ACT.org. In the PCA, PC1 explained 76% of the variance in the data set and was extracted for use as a single measure of incoming preparation.
To determine the impact of Incoming Preparation, Instructor, and Course on student struggle, we used generalized linear mixed-effects models (GLMMs). We used model selection on multiple GLMMs to determine the best-fit structure of both fixed effects and random effects. The fixed effects we used in model selection included our principal component of Incoming Preparation (PC1), Instructor, and Course. In the case in which there were two instructors for a single section (alternating instructors), we treated both instructors as a single instructor, as the students remained the same for that course, and neither instructor taught independently in courses surveyed in this study. The random effects we included in model selection to account for variation in the data set include Student ID (to account for having duplicate entries from 130 individuals in our data set), Student Year Rank (first year, second year, third year, fourth year), Section (to account for potential differences between sections for a single instructor), and Semester (to account for the expected variation that existed between a pre-COVID semester and a semester with COVID and the emergency transition to remote learning). Following best practices outlined by Theobald (2018), we determined the best fixed-effects structure first without including random effects, and subsequently determined the best random-effects structure while holding the fixed effects constant. The best-fit model was selected using Akaike’s information criterion (AIC).
RQ1B. How Do Performance Outcomes Correlate with Students Encountering and Overcoming Struggle in Introductory Biology?
We were interested in understanding how student experiences of struggle impacted overall performance outcomes in the course when accounting for factors that are known to influence performance such as Incoming Preparation, Instructor, and Course. We used linear mixed-effects modeling to determine the impact of encountering and overcoming struggle on performance in the course. We additionally used model selection to determine the best-fit model. Fixed effects included in model selection were Incoming Preparation and encountering or overcoming struggle. While we were not directly interested in the relationship between Incoming Preparation and Performance, we included this factor to account for the known relationship between these variables. We included Incoming Preparation as a fixed effect, as it is a continuous numerical variable and as such cannot be treated as a random factor. We determined the best-fit model using AIC score comparison as outlined in RQ1A. Random effects included in model selection were Student ID, Student Year Rank, Instructor, Course, Section, and Semester. Further explanation on why each factor was included in model selection can be found in RQ1A.
RQ2: Qualitative Analysis
After downloading the answers to the open-response question (Figure 1), nine of the coauthors (A.E.B., J.E.P., T.L., J.D.B., C.B., I.C.F., C.C.J., T.S., C.J.B.) individually reviewed a set of 40 student responses to the open-ended question and generated codes using inductive coding (created codes from data rather than creating codes a priori; Saldaña, 2013) and qualitative content analysis (i.e., a tool used to determine the presence and frequency of certain codes within the open-ended responses; Morgan, 1993). They also took detailed analytic notes at that time (Birks and Mills, 2015). They then met together to compare their codes and create/revise the codebook. The researchers used constant comparison methods to ensure quotes within a code were not so different that they warranted the creation of a new code (Glesne and Peshkin, 1992). This process was repeated until the group was confident with their codebook, developing one unified codebook with detailed descriptions and examples. The final 10 codes in the codebook were: 1) Prior STEM (other than biology), 2) Prior Biology, 3) COVID-19, 4) External Resources, 5) Classroom Factors, 6) Study Habits, 7) Innate Ability, 8) Time Management, 9) Preference for Biology, and 10) Anxiety. During the code creation process, we noticed students referenced the code Classroom Factors frequently, so one of the coauthors (T.L.) further broke down the Classroom Factors code into six subcodes: 1) Content, 2) Exams, 3) Format, 4) Group Work, 5) Instructor, and 6) Workload. Sources of struggle and reasons students did not encounter struggle were seamlessly categorized in the same way, and always represented two sides of the same coin (i.e., two separate parts of the same category). For example, students may describe their struggle because they were unfamiliar with biology or STEM content, or they did not struggle because they had a strong background in biology or STEM; or students may have mentioned that they did struggle because of the large workload, or they did not struggle because they had a manageable workload.
After the 10 main codes were established, the same nine coauthors used axial coding (Saldaña, 2013) to group the codes into one of two categories: internally or externally attributed struggle. Codes 1–5 were considered to be external attributes (i.e., outside the students’ control), while codes 6–10 were categorized as internal (i.e., within the students’ control). Student responses to their reported struggle and ability to overcome reported struggle were assigned to all appropriate codes, meaning that a single response could fit into more than one category.
Once the codes, subcodes, and categories were established, three of the coauthors (E.P.D., C.B.T., T.L.) completed the coding independently in three sections: 1) students who did not struggle, 2) students who did struggle but did not overcome, and 3) students who did struggle but did overcome. For each section, each of the three coders independently coded responses in “blocks” ranging from 40 to 169 responses and then collaboratively coded to 100% consensus. This resulted in a total of seven different blocks, with an average initial percentage agreement of 69.5% for the main codes and 69.8% for the Classroom Factors subcodes. We calculated percent agreement by dividing the total number of codes agreed on by the number of codes agreed on plus the number of codes not agreed upon. We calculated percentages for each code by dividing the total number of responses assigned for each code by the total number of student responses for each category.
RESULTS
Descriptive Statistics
After downloading the Qualtrics survey results, we noted the percentage of students who did or did not encounter struggle, and of those who experienced struggle, we reported those who did and did not overcome it. Of the total 960 responses, 253 (26%) reported that they did not encounter struggle and 707 (74%) reported that they did encounter struggle. Of the 707 who encountered struggle, 190 (27%) reported they did not overcome their struggle, and 515 (73%) reported that they did overcome their struggle, and 2 did not respond concerning whether they overcame their struggle or not.
Of the 707 responses that included complete open-ended qualitative responses, 560 (79%) reported encountering struggle and 147 (21%) reported they did not encounter struggle. Of the 560 who struggled, 412 (74%) reported overcoming their struggle, while 148 (26%) reported not being able to overcome their struggle (Supplemental Figure S1). For a complete breakdown of sample sizes by semester for both data sets, see Supplemental Table S3.
RQ1: Quantitative Analysis
RQ1A. What Factors Influence Struggle in Introductory Biology?
The best-fit model for predicting whether students encountered or overcame struggle was determined by identifying the model with the lowest AIC score (Supplemental Tables S4 and S5). The best-fit model for encountering struggle included the interaction between our measure of Incoming Preparation (i.e., the results of a principal component analysis described in Methods; hereafter PC1) and Course. Student Year Rank was included as a random effect. During model selection, we also included Instructor, Student, Section, and Semester as possible additional factors, but the best-fit model did not include these factors as random or fixed effects (see Methods for more detail on model selection). The best-fit model for overcoming struggle included PC1 and Instructor as fixed effects and included student as a random factor.
Incoming Preparation had a significant effect on encountering and overcoming struggle (Supplemental Tables S5 and S6). The model showed that students with higher measures of Incoming Preparation were less likely to encounter struggle and more likely to overcome struggle if they did encounter it. Specifically, with each point increase in Incoming Preparation, students were 0.66 times less likely to encounter struggle and 0.25 times more likely to overcome their struggle.
Course and Instructor also both had significant effects, where Course was significantly correlated with encountering struggle and had an interaction with Incoming Preparation (Supplemental Table S6 and Supplemental Figure S2), and Instructor was significantly correlated with students overcoming struggle (Supplemental Table S7 and Supplemental Figure S2). Specifically, students in Course 2 and Course 3 were less likely to encounter struggle (1.1 times and 1.4 times less likely, respectively) than students in Course 1 (Supplemental Table S6 and Supplemental Figure S2). We contextualize these findings by pointing out that Course 1 is the first introductory biology course that most students take their first semester of college and is a prerequisite for Course 2 and Course 3. Additionally, Student Rank (first year, second year, third year, fourth year) was included in the best-fit model for encountering struggle as a random factor. The model accounting for both Student Rank and Student ID had a ΔAIC of 2, indicating that they were identical in fit, so we present the most parsimonious model.
RQ1B. How Do Performance Outcomes Correlate with Students Encountering and Overcoming Struggle in Introductory Biology?
We next wanted to determine whether students’ experiences of struggle correlated with their final scores in the course, controlling for Incoming Preparation. The best-fit model was determined by comparing AIC (Supplemental Table S8) and included two fixed effects (Encountering Struggle and Incoming Preparation (PC1)) and two random effects (Instructor and Student ID):
We found a significant effect of encountering struggle on performance in the course when also accounting for variation due to Incoming Preparation (PC1) (Figure 2 and Supplemental Table S9). Students who encountered struggle scored 3.7 points lower on their final grades than students who did not struggle.
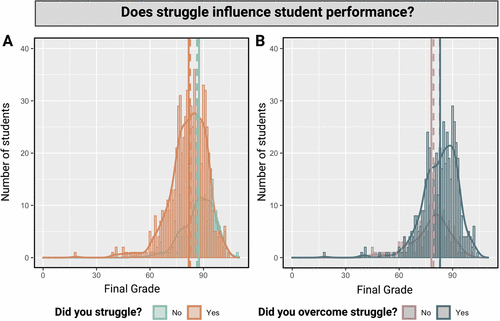
FIGURE 2. Histogram of final scores grouped by (A) whether students did or did not encounter struggle, and (B) whether students who encountered struggle did or did not overcome their struggle. Hashed lines represent the estimated marginal means in final scores for each group, and solid lines represent raw means in final scores for each group. The estimated marginal mean is the mean of the final score for each group of struggle (Y/N) or overcome struggle (Y/N) at the mean value of Incoming Preparation (PC1) based on the model.
We then examined the effect of overcoming struggle on final score while accounting for effects of Incoming Preparation, as well as Instructor and Student ID as random effects. Using AIC comparison, we found the best-fit model was (Supplemental Table S10):
There was a significant effect of overcoming struggle on performance in the course when accounting for variation due to Incoming Preparation (PC1) (Figure 2 and Supplemental Table S11). Students who reported overcoming struggle had final grades 3.6 points higher than students who reported they did not overcome their struggle.
Overall, students who reported not encountering struggle performed slightly better when accounting for Incoming Preparation than students who reported encountering struggle; students who reported encountering and overcoming struggle performed slightly better than students who encountered but could not overcome struggle when accounting for Incoming Preparation (Figure 2). This indicates that students who encountered struggle and those who could not overcome struggle received lower grades than students who did not encounter struggle and those who reported overcoming struggle.
RQ2: Qualitative Analysis
For ease of interpretation of the qualitative results, we developed a qualitative codebook for the 10 codes, as binned into the broader two categories of external and internal attributes, complete with code explanations and both a positive and negative example of student use (Figure 3). For example, if students said they did not struggle or overcame their struggle because of something, then that would be a positive example of the category. However, if students said they struggled, their response would be a negative example of the category. Additionally, we provided a breakdown of the subcodes of Classroom Factors with explanations of the codes and both positive and negative examples (Figure 4). We recommend referral to Figure 3 and Figure 4 to understand the codes and examples. We present results from both semesters (e.g., Fall 2019 and Spring 2020) separately, as there were distinct circumstantial differences between the two (e.g., different instructors, different students, different semesters, and the pivot in Spring 2020 to emergency remote learning due to the COVID-19 pandemic).
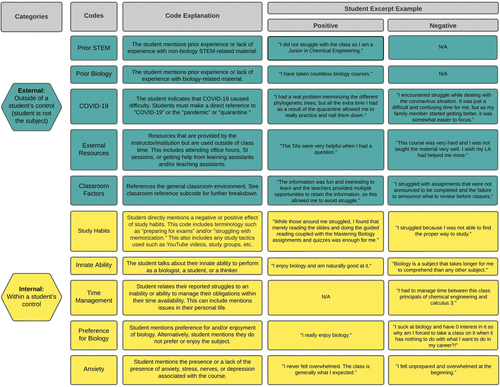
FIGURE 3. Explanations of 10 qualitative codes with examples of a positive and negative student response for each code.

FIGURE 4. Breakdown of six Classroom Factors subcodes with examples of a positive and negative student response for each.
RQ2A. To What Do Students Attribute Their Struggle (or Lack of Struggle) in Introductory Biology?
When we asked students to elaborate on their experiences, we found explanations differed based on whether students did or did not struggle (Figure 5A). Across both semesters, we found students who did not face struggle largely attributed their lack of struggle to Classroom Factors (31% Fall, 34% Spring), Study Habits (29% Fall, 27% Spring), and Prior Biology (29% Fall, 16% Spring). One response that typifies a positive reference to Classroom Factors is: “The information was fun and interesting to learn and the teachers provided multiple opportunities to retain the information, so this allowed me to avoid struggle” (Figure 3).
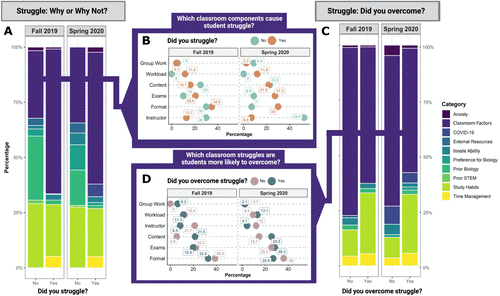
FIGURE 5. Fall 2019 and Spring 2020 student responses as categorized into the 10 codes. (A) Student responses indicate the source of struggle (Yes) or reason for a lack of struggle (No) across semesters. (B) Breakdown of category Classroom Factors as a source of struggle or lack of struggle into six subcodes displayed in ascending order. (C) Of students who reported struggle, responses reflect source of struggle among those who overcame (Yes) and could not overcome (No) across semesters. (D) Of students who reported struggle, breakdown of category Classroom Factors as a source of struggle among students who could overcome and those who could not into six subcodes displayed in ascending order.
Among students who reported facing struggle, the most often cited reasons for struggle were Classroom Factors (65.6% Fall, 59.7% Spring) and Study Habits (23.5% Fall, 21.8% Spring). A response that typifies a negative reference to Classroom Factors is: “I struggled with assignments that were not announced to be completed and the failure to announce what to review before classes” (Figure 3). However, the Classroom Factors code had a wide variety of responses that led to a more detailed breakdown of this code into subcodes (Figure 4). When comparing students who did not struggle with those who did struggle, we observed that students who did struggle mentioned Classroom Factors more, Prior Biology less, Time Management more, and Study Habits less (Figure 5A).
We found that students—both those who did and did not struggle—largely referred to external factors (e.g., Prior STEM, Prior Biology, COVID-19, or Classroom Factors) more than internal factors (e.g., Anxiety, Innate Ability, Preference for Biology, Study Habits, or Time Management; Figure 6). However, students who did not struggle referred to internal reasons (26% Fall, 32% Spring) more often than students who did struggle (18% Fall, 19% Spring).
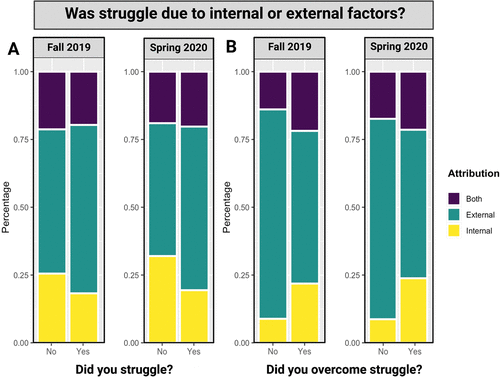
FIGURE 6. Fall 2019 and Spring 2020 student responses as collapsed into the two overarching categories: externally or internally attributed factors leading either to (A) students reporting they did not experience struggle (No) or that they experienced struggle (Yes). (B) Of students who experienced struggle, students reporting they were not able to overcome struggle (No) or that they could overcome struggle (Yes). Of note, external factors include Prior STEM (other than biology), Prior Biology, COVID-19, External Resources, and Classroom Factors codes; and internal factors represent Study Habits, Innate Ability, Time Management, Preference for Biology, and Anxiety. External factors are those outside students’ control, and internal factors are those within students’ control.
Classroom Factors Reference Breakdown. When comparing referenced classroom factors between students who did and did not struggle (Figure 5B), major differences included students who struggled more often cited Workload, Exams, and Format as sources of their struggle. In contrast, students who did not struggle more often cited the Instructor, Group Work, and Content as a reason for their lack of struggle. When referencing Classroom Factors, the most cited reasons for not struggling were the Instructor (30% Fall, 52.6% Spring), the Format (30% Fall, 7% Spring), and the Content (25% Fall, 22.8% Spring). For example, students who cited Instructor as a reason they did not struggle (i.e., a positive reason) often indicated a response similar to “I felt my professor prepared us and I worked very hard outside of class” (Figure 4). The most often cited reasons for struggle when referencing Classroom Factors were the Format (34.8% Fall, 30% Spring), Exams (20.6% Fall, 27.3% Spring), and Content (16.1% Fall, 21.8% Spring; Figure 5B). One example of a response in which Format was a source of struggle (i.e., a negative reason) was “It was very difficult for me to learn in a classroom that was flipped.”
RQ2B. What Sources of Struggle Were Students Most Likely to Overcome?
Reasons for struggle largely varied depending on whether the student could overcome their struggle or not (Figure 5C). For example, students who did not overcome their struggle most often cited Classroom Factors as their source of struggle (76% Fall, 68% Spring). Students who did overcome their struggle most often cited Classroom Factors (62% Fall, 57% Spring) and Study Habits (28% Fall, 27% Spring) as their source of struggle.
When examining sources of struggle as external factors (e.g., Prior STEM, Prior Biology, COVID-19, or Classroom Factors) and internal factors (e.g., e.g., Anxiety, Innate Ability, Preference for Biology, Study Habits, or Time Management), we found that students who overcame their struggle cited internal factors as their reason for struggle (22% Fall, 24% Spring) more than students who did not overcome (9% Fall, 9% Spring; Figure 6).
Classroom Factors Reference Breakdown. In both semesters, there were not major differences in sources of struggle attributed to the classroom among students who did or did not overcome struggle (Figure 5D). For example, the most cited sources of struggle for students who did not overcome were the Format (38.9% Fall, 36% Spring), Exams (22.2% Fall, 25.3% Spring), and Instructor (21.1% Fall, 12% Spring). Similarly, the most cited sources of struggle for students who did overcome were Format (32.8% Fall, 26.9% Spring), Exams (19.8% Fall, 28.3% Spring), and Content (21.5% Fall, 25.5% Spring). The largest differences between students who did and did not overcome their struggle were that students who did not overcome cited Format and Instructor more, while students who did overcome their struggle cited Content more.
DISCUSSION
RQ1: Quantitative Analysis
What Factors Impact Struggle and How Does Struggle Impact Overall Performance?
The linear models show a significant effect of Incoming Preparation and Course on encountering struggle and Incoming Preparation and Instructor on overcoming struggle. We also found a significant effect of encountering and overcoming struggle on performance, even when accounting for Incoming Preparation. Here, we make sense of these findings by placing each of these factors in the context of previous research.
Incoming Preparation was significantly correlated with encountering and overcoming struggle. This was not surprising because of the established relationship between incoming preparation and STEM course performance (Salehi et al., 2019, 2020). Additionally, while it appears at face value that struggle explains performance, students’ perceptions of struggle are likely driven by their performance in the course (though with our current data, we cannot disentangle directional impacts). As our measure of Incoming Preparation relates to precollege educational resource availability, and students with lower incoming preparation experience struggle disproportionately, our results provide additional evidence that “gateway” courses differentially impact subsets of students. Future areas of research should explore effective teaching strategies to help students overcome challenges in introductory biology courses, as well as strategies students use to cope with obstacles and struggle.
Our results also support previous work showing student perceptions of their capacity to succeed within a discipline are driven by performance outcomes in STEM (Seymour and Hunter, 2019). Some students will underestimate their abilities based on grades compared with similarly performing students (Marshman et al., 2018). Mentions of performance arose multiple times in students’ open-ended responses, with students often expressing the sentiment that they felt they knew the material but performed poorly on the exams. Students also often cited poor grades on exams when asked why they struggled in the course. Many works have demonstrated the importance of grades to undergraduate students (Sabot and Wakeman-Linn, 1991; Lewis et al., 2017; DeFeo et al., 2021), which likely carries over into their perceptions of struggle. One student responded to the open-ended prompt saying “I made a C on the first test and did very well on everything else. Completed all assignments and did well on the next tests and still looks like I’ll finish with a B,” and while to many this would sound like a student who encountered struggle and overcame it, this student categorized themselves as someone who encountered struggle and did not overcome it, demonstrating a strong link between grades and perceptions of struggle. Other students viewed struggle differently: one student expressed a similar sentiment of struggling on the first test but doing better on the rest of the exams and thus identified as not encountering struggle.
Our results also support the idea that STEM classes appraise students’ abilities to understand science, and this appraisal is related to how well their previous schooling prepared them for tertiary education. This puts capable but academically underprepared students at a serious disadvantage (Salehi et al., 2019, 2020). Intentional or not, the practice of using STEM courses to weed out students seeking degrees in STEM is problematic, because it hinders efforts to attract students who have been historically underrepresented in those fields, such as persons excluded because of their ethnicity or race (PEERs), first-generation college students, and women (Mervis, 2011).
To address gaps in incoming preparation, instructors should not assume that students already possess significant content knowledge and should provide greater resources such as supplemental instruction programs or peer-tutoring study groups (Batz et al., 2015; Salehi et al., 2019; Meaders et al., 2020). Another alternative that may help make introductory biology courses more accessible across different preparation levels is an emphasis on low-stakes formative assessments. Shifting focus to lower-stakes assessments has been shown to provide a mechanism to assess student knowledge without significant performance gaps that are often apparent in high-stakes assessments among genders or students historically excluded in science because of their ethnicity or race (Sambell and Hubbard, 2004; Cotner and Ballen, 2017).
We also found a significant correlation between course and encountering struggle. The course in which students were more likely to encounter struggle was Course 1, which is the first biology course that most biology majors take in college, often in the very first semester. This sentiment was prevalent in students’ open-ended responses, where many related their sources of struggle to study habits, not knowing how or what to study for exams, and adjusting to college courses in general (see in RQ2 for further discussion on study habits). Our results suggest instructors can help mitigate struggle experienced by students by spending class time early in the semester discussing study habits and time use both in and out of class and by setting clear expectations for the course, particularly in introductory courses. This recommendation is echoed in Seymour and Hunter (2019), who through extensive student interviews found that good teachers and teaching had several characteristics such as course structure that was organized and coherent, and instructors who showed concern for student learning.
RQ2: Qualitative Analysis
According to our qualitative results, students reported several sources of struggle, including experience in Prior STEM or Prior Biology courses, COVID-19 (only during Spring 2020), External Resources, Classroom Factors, Study Habits, Innate Ability, Time Management, Preference for Biology, and/or Anxiety. As Spring 2020 was a tumultuous semester for many due to the emergency transition to remote learning secondary to the onset of COVID-19 pandemic midsemester, it was not necessarily reflective of a normal or repeatable semester. Further, Spring 2020 results were very similar to Fall 2019 results, aside from the novel code COVID-19. For these reasons, this Discussion will focus on the results from Fall 2019.
We will first focus on the top three most mentioned codes: 1) Classroom Factors, 2) Study Habits, and 3) Prior Biology. Then, we will follow with a discussion of bigger picture trends in codes grouped as either internal or external sources of struggle.
Classroom Factors
Overall, both students who faced struggle and those who did not face struggle elaborated by citing Classroom Factors, demonstrating how specific factors can be advantageous to some students while disadvantaging others. Within this category, the two most mentioned subcodes were Instructor and Format.
First, students commonly cited Instructor as a reason they did not face struggle (e.g., “I felt my professor prepared us, and I worked very hard outside of class”), while a much smaller percentage cited the Instructor as a reason why they did struggle (e.g., “The professor just didn’t click with me”). However, sources of struggle that students were least likely to overcome were also Classroom Factors, including Instructor and Format. Previous literature highlights the importance of the instructor, demonstrating that STEM professors with fixed mindsets caused students to feel less of a sense of belonging and more stereotyped based on their gender as opposed to those instructors with growth mindsets. This ultimately negatively impacted women students and their performance in the course (Canning et al., 2021). Canning et al. (2019) showed professors’ beliefs about the fixedness of ability were associated with racial performance gaps that were twice as large as gaps in courses taught by faculty who held growth mindsets. Seidel et al. (2015) created an “Instructor Talk framework” to assist instructors in reflecting on the learning environments they create through noncontent language in classrooms. When using their framework with two different instructors, Seidel et al. (2015) discovered specific subcodes of Instructor Talk may play a critical role in constructing inclusive environments, allowing the potential for students to overcome stereotype threat (i.e., boosting self-efficacy, revealing secrets to success, and promoting diversity in science). One example of Instructor Talk focusing on promoting diversity in science from the Seidel et al. (2015) study reads:
We absolutely know, we have lots of stories that say the kinds of people who do science affect the kinds of questions that get asked, affect the kinds of data that gets acknowledged, and the kind of data that gets ignored. So, that’s why it’s really important to have a diverse group of people doing science. (p. 6)
Further research examining Instructor Talk across multiple classroom contexts showed both positively and negatively phrased Instructor Talk has lasting impacts on students’ perceptions of the classroom environment (Harrison et al., 2019; Ovid et al., 2021). We recommend instructors signal an inclusive learning environment for all students by using the framework detailed in Seidel et al. (2015) to monitor and improve the messages they convey to their students.
Second, both students who did struggle and those who did not struggle mentioned Format in their responses. Of those students who cited Format as a source of struggle, fewer overcame this struggle. This finding may be explained by a preference for or against active learning, the dominant pedagogy deployed across classrooms in the current study. This is supported by previous literature that demonstrated undergraduate students learn more in classrooms that use active learning than those that use traditional lecture strategies, even though they perceive they learn less (Deslauriers et al., 2019). This hypothesis is well supported by the following quote obtained from a student facing struggle in the Fall 2019 semester: “I, along with many of my peers, did not like the ‘flipped classroom’ curriculum. It was said that many students learn better this way, but the average test scores do not support this. A regular lecture-based classroom is a better way for students to learn. Teaching ourselves provides a workload that isn’t sustainable for the average college student.” Student comments along these lines were common during the Fall 2019 semester.
Alternatively, this finding could demonstrate that the given format chosen by a professor advantaged some students while disadvantaging others. This is in line with previous literature that demonstrated common active-learning practices, such as group work (Driessen et al., 2020b), increase student performance on average (Springer et al., 1999; Knight and Wood, 2005; Carmichael, 2009; Chaplin, 2009; Daniel, 2016; Marbach-Ad et al., 2016; Yapici, 2016; Donovan et al., 2018; Weir et al., 2019) but may disadvantage LGBTQIA+ students (Cooper and Brownell, 2016) and students with disabilities (Gin et al., 2020). Therefore, it is important for instructors to be deliberate about their teaching practices and aware of their students’ needs when making pedagogical decisions.
Study Habits
Students who did and who did not face struggle frequently mentioned Study Habits in their explanations; among those who struggled over the semester, students who overcame more often cited Study Habits as the reason they struggled than those who did not overcome. Students who could not overcome attributed their struggle to academic performance in the class. Given how important study habits are to performance outcomes (Numan and Hasan, 2017), the citation of Study Habits by both those who struggled or those who did not struggle makes sense, as more prepared students did better on exams, and exam scores were the bulk of students’ grades. In introductory biology courses, previous work has shown the impact of incoming preparation on student performance (Salehi et al., 2020), and part of academic preparation relates to studying effectively for college-level exams. Future work will be needed to clarify the extent that a lesson on study habits and expectations have on students’ experiences of struggle, academic performance, and anxiety.
Prior Exposure to Biology
Students with prior biology courses and experiences often did not face struggle in our sample; however, previous literature concerning prior biology knowledge and student performance yields mixed messages. For example, Bone and Reid (2011) demonstrated prior experience with only high school biology did not predict performance in a first-year biology course. That is, students who completed biology at the senior high school level did perform better than those who had not, but only if they also completed chemistry (Bone and Reid, 2011). Similarly, Johnson and Lawson (1998) suggested reasoning ability contributes more to student performance in biology than prior biology knowledge. On the other hand, Loehr et al. (2012) claimed student performance in introductory college biology is positively associated with advanced high school science and mathematics course work, an emphasis on a deep conceptual understanding of biology concepts, and a prior knowledge of concepts addressed in well-structured laboratory investigations. Similarly, Ozuru et al. (2009) showed undergraduate students’ prior biology knowledge was positively correlated with their overall comprehension of their college biology text.
Regardless of the mixed findings from previous literature, it was clear in this study that students often attributed their lack of struggle to prior preparation in biology, and if students did not struggle, they were more likely to perform higher than predicted. This means that students’ varying levels of exposure to biology could be contributing to a difference in ultimate performance, becoming more an effect of their social and academic capital from the time they were children than an effect of college instruction (Marjoribanks, 1997). Previous research conducted by Salehi et al. (2019) demonstrated this to be true in the case of performance in standard introductory calculus–based mechanics. That is, incoming preparation predicted 20–30% of the variation in student exam performance. We recommend instructors design their courses and teaching methods to better match the actual preparation level of their incoming students, rather than potentially assuming their students have previously learned the information in high school. This can be accomplished through a pre-course concept inventory, and the use of this information by the instructor may eliminate performance gaps while improving the success of all students.
Internal versus External Attributions of Struggle or Lack of Struggle
Overall, students more commonly cited external sources (i.e., Prior STEM, Prior Biology, COVID-19, External Resources, and Classroom Factors) as the reason(s) they did or did not struggle rather than internal factors (i.e., Study Habits, Innate Ability, Time Management, Preference for Biology, and Anxiety). While the majority of student responses on the cause of struggle pointed to external factors, more students reported overcoming internal (rather than external) factors.
These results are largely consistent with previous literature on both mindset (“growth mindset” vs. “fixed mindset”) and grit (perseverance and passion for long-term goals; Duckworth et al., 2007; Limeri et al., 2020). The way students view their capacity for academic growth and change likely plays a large role in how they approach struggle, particularly in STEM fields, in which many students think of learning as an innate skill.
Grit theory predicts that student success is not only due to their capacity to learn but is largely predicted by measures of a student’s determination. We acknowledge that this theory has serious limitations in its application, and we do not intend to shift responsibility away from situational factors that legitimately challenge students (Ris, 2015). Additionally, we recognize that this study is conducted at a school that disproportionately serves middle- to upper-class students and is among the lowest in enrollment of students from the bottom 20% in income (Aisch et al., 2017). Instead, we recognize that introductory courses are required for progress within the major for science students. Because we observed that students are more likely to overcome internal struggle, we suggest that interventions targeting mindset and/or grit may improve students’ ability to overcome challenges they face (Harackiewicz and Priniski, 2018; Binning et al., 2019, 2020; Limeri et al., 2020).
Additionally, these divisions among students based on whether they experienced challenges of introductory courses and whether they could overcome them reflects well-documented research on persistence and loss in undergraduate STEM education. For example, Seymour and Hunter (2019) described different types of processes that accounted for student decisions to leave STEM majors. Tracking students over time revealed a “push–pull” decision-making process, wherein students simultaneously experienced “push” factors, such as problems in precollege and college experiences, as well as “pull” factors, or perceived attractions of alternative majors or career trajectories. First, they showed that students’ decisions to switch majors were due primarily to external factors such as problems in course design, teaching, and negative classroom culture; these are the same external factors to which our students largely attributed their sources of struggle. Second, Seymour and Hunter (2019) observed that students who persisted in a major developed coping strategies to help them persevere. Because our study does not investigate the coping strategies used by students who overcame their struggle, exploring coping strategies in biology among students who overcame struggle is the next logical step of the current research.
LIMITATIONS
This study has several limitations. First, we assumed students interpreted the word “struggle” in the same way. An advantage of leaving the term open for interpretation was that students communicated in their responses how they interpreted struggle and how they viewed struggle in the classroom. Based on student responses, struggle may have been interpreted as challenges impacting performance in the course or the ability to learn.
As this study took place over two consecutive semesters with some courses that are often taken consecutively (Introductory Biology I in Fall 2019 and Introductory Biology II in Spring 2020), there were 130 students with duplicate survey entries (260 entries out of 965), and thus our data set does not have completely independent samples between semesters. While this does not represent completely independent sampling, we chose to retain all duplicate entries for our study and to control for duplicate student responses in the quantitative portion by including student ID as a random effect.
Finally, while struggle can significantly undermine students’ academic abilities and performance (Batz et al., 2015; England et al., 2017), struggle disproportionately affects PEERs, who already face unique challenges resulting from social isolation and discrimination (Bertrand and Mullainathan, 2004; Hurtado et al., 2010). We acknowledge that the students who participated in our study are largely from overrepresented backgrounds (Supplemental Table S2). Future work will expand beyond our single institution to examine struggle in other institutional contexts and student populations.
CONCLUSIONS
Students reported struggle across introductory biology for a variety of reasons, as demonstrated by our findings. Quantitative and qualitative analyses revealed that students’ interpretation of struggle was interwoven with their perceptions of performance or learning in the class. We found that obstacles experienced by some students served as life rafts for other students. For this reason, we hope this work promotes awareness of the common pitfalls introductory biology students face, so instructors can intervene with recommended strategies, creating more equitable classrooms where all students can succeed, regardless of prior experience with biology, knowledge of effective study habits, and classroom elements.
ACKNOWLEDGMENTS
The qualitative survey used for this study was adapted from a survey developed by Dr. Lisa Limeri, who also provided interesting discussion on the project in an Introduction to Discipline-Based Education Research course taught by C.J.B. We would also like to thank Dr. Todd Steury for his wisdom concerning quantitative analyses. Finally, we would like to thank all of the members of the Ballen lab for their feedback on the paper. Graphs were created in R, and many figures were formatted with BioRender.com. This work was supported by National Science Foundation DUE-2120934 awarded to A.E.B. and C.J.B. and DUE-2011995 and DBI-1919462 awarded to C.J.B.