Students’ Experiences and Perceptions of the Scientific Research Culture after Participating in Different Course-Based Undergraduate Research Experience Models
Abstract
Undergraduate students interact with the culture of scientific research when they participate in direct mentorship experiences and laboratory courses such as course-based undergraduate research experiences (CUREs). Much work has been done to explore how CUREs impact the interest, motivation, and retention of undergraduate students in science. However, little work has been done exploring students’ experiences and perceptions of the culture of scientific research in the CURE context, and how different CURE models representing different subfields of science impact these experiences and perceptions. This study explored which cultural aspects of scientific research students experienced after participating in a CURE and whether their perceptions of those cultural aspects differed based on students’ participation in a bench-based or computer-based research project. Students discussed the Practices and Norms/Expectations of scientific research most frequently. Students in the bench-based and computer-based project areas mentioned different cultural aspects as important to their experiences. Bench-based and computational students also had different perceptions of some of the same cultural aspects, including Teamwork, Freedom & Independence, and Persistence & Resilience. These results suggest that different CURE models differentially impact students’ experiences and perceptions of the culture of scientific research, which has implications for examining how students move into scientific research.
INTRODUCTION
Becoming an expert in science requires people to move into the culture of scientific research. This process, called border crossing, generally begins when undergraduate students first interact with scientific research through their lab courses or direct mentorship opportunities (Anzaldúa, 1987; Lave and Wenger, 1991; Aikenhead, 1996; American Association for the Advancement of Science [AAAS], 2011). Course-based undergraduate research experiences (CUREs) are one context in which students are introduced to scientific research culture and can begin to learn how to border cross. Designed to mimic direct mentorship opportunities and provide undergraduates with research experience, CUREs allow undergraduates to interact with the culture of scientific research by engaging in the work scientists do to produce knowledge (Lave and Wenger, 1991; Lunetta et al., 2007; AAAS, 2011; Auchincloss et al., 2014). Many studies have shown that participating in CUREs can increase student interest and retention in science as well as their confidence and motivation (Shaffer et al., 2010; Dolan, 2016; Olimpo et al., 2016; Shanle et al., 2016). However, there is a paucity of work exploring the cultural aspects of scientific research that students experience in the CURE setting and how those cultural aspects may support or challenge students’ border crossing (Dewey et al., 2021).
Different models of CUREs have been developed, including bench-based, field-based, and computer-based models, that represent different subfields within the sciences (Kirkpatrick et al., 2019). Reports of student outcomes from each of these CUREs suggest that these different models provide students with similar cognitive, affective, psychosocial, and behavioral outcomes (Dolan, 2016; Kirkpatrick et al., 2019). However, no studies to date have explored whether students experience different cultural aspects of scientific research in different models of CUREs and whether those aspects present different barriers or entry points to students trying to border cross into scientific research. Thus, this study explores the cultural aspects of scientific research that students experience after participating in a CURE and how students’ experiences and perceptions of those cultural aspects differ as a function of project type (i.e., bench-based vs. computer-based projects).
The Culture of Scientific Research
Academic scientific research has its own culture made up of distinct aspects that help identify it and distinguish it from other academic fields, such as history (Taras et al., 2009). Through a systematic literature review and expert validation, the core cultural aspects related to the work performed by scientists to produce scientific knowledge were identified and summarized in the Culture of Scientific Research (CSR) Framework (Dewey et al., 2021). The framework is comprised of 31 cultural aspects categorized as Practices, Norms/Expectations, and Values/Beliefs (Phelan et al., 1991; Figure 1). Practices are the day-to-day activities or actions of a group. Norms/Expectations are the standards that influence how a group and its members think and behave. Values/Beliefs are the broad ideas that are held in esteem by a group and that are used to define the group (Phelan et al., 1991). Each aspect has been given a shorthand code in Figure 1. Practices are P1–P13, Norms/Expectations are NE1–NE9, and Values/Beliefs and VB1–VB9.
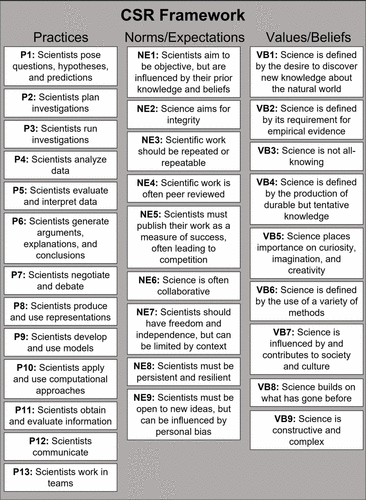
FIGURE 1. The culture of scientific research framework. Adapted from Dewey et al., 2021.
Previous work has described how the culture of scientific research is reflective of the dominant class in science, which both historically and still today, reflects white, male, Western worldviews (Aikenhead, 1996; Seymour and Hewitt, 1997; Carlone and Johnson, 2007, 2012). As such, the cultural aspects in the CSR Framework also reflect white, male, Western worldviews (Dewey et al., 2021). However, rather than advocating for the persistence of this culture, the CSR Framework was developed to identify the current scientific research culture and allow for work toward change. The core cultural aspects identified in the CSR Framework are distinct from, but can be influenced by, broader layers of culture such as broader societal cultures, institutional climates, departmental cultures, and microcultures within research labs (Thoman et al., 2017; Reinholz et al., 2019). The CSR Framework also does not include forms of discrimination such as racism or sexism as cultural aspects, although they are commonly experienced within scientific research (Clancy et al., 2014; Dutt, 2020). The core cultural aspects identified in the CSR Framework should be seen as one layer of culture that can be influenced by many other layers of culture. This layered approach allows for work that examines the impact of broader layers of culture and various forms of discrimination on experiences of border crossing into the scientific research culture and interacting with its core cultural aspects. Ultimately, this type of work will allow for the identification of ways to change the culture of scientific research to make it more inclusive.
Border Crossing into the Culture of Scientific Research
The process of moving between different cultures is called border crossing (Anzaldúa, 1987; Aikenhead, 1996). Some cultures are easier to border cross between than others, and the similarities and differences between cultures can greatly impact this process (Aikenhead, 1996). Scientific research is a field that undergraduates are asked to border cross into as they start participating in research experiences. To successfully move into the world of scientific research, undergraduates must learn about and interact with the Practices, Norms/Expectations, and Values/Beliefs that make up the culture of scientific research (Phelan et al., 1991; Aikenhead, 1996; Lunetta et al., 2007; Dewey et al., 2021). Border crossing is commonly achieved through legitimate peripheral participation, which describes how a newcomer becomes part of a community (Lave and Wenger, 1991). The newcomer starts on the periphery of the field, observing the experts. Over time, the newcomer begins working directly with experts in a community of practice, participating in the legitimate practices of the field. This mentored participation helps newcomers eventually become part of the community of practice in that field (Lave and Wenger, 1991).
Undergraduates act as legitimate peripheral participants most commonly during direct mentorship opportunities. By engaging with experts in the field and interacting with its culture, students can learn how to border cross into the field of scientific research. During these opportunities, undergraduates work with experts (e.g., graduate students, postdoctoral researchers, or principal investigators), learning how to think, act, and talk like scientists while performing different scientific practices. These experiences help undergraduates learn how to define themselves as members of the field of scientific research (Lave and Wenger, 1991; Thiry and Laursen, 2011). However, direct mentorship opportunities have limited availability, and consequently not all undergraduate students are able to participate. Therefore, different types of laboratory course experiences have been developed to provide an avenue for a larger population of students to participate in research (Auchincloss et al., 2014; Bangera and Brownell, 2014).
Laboratory courses can take a wide variety of forms. Traditional laboratory courses differ the most from direct mentorship experiences, because they have predefined topics and methods, step-by-step instructions, and an outcome that is known to students and instructors (Auchincloss et al., 2014; Brownell and Kloser, 2015). In inquiry laboratory courses, students more often do not know the outcome of the course activities and may be asked to produce their own methods (Auchincloss et al., 2014; Ballen et al., 2017, 2018). However, instructors still know the expected outcome of students’ investigations, and therefore students are often not engaging in the discovery of new knowledge (Ballen et al., 2017; Goodwin et al., 2021). For both traditional and inquiry laboratory courses, the main mentor students interact with is the course instructor.
CUREs tend to be the lab courses that are most similar to direct mentorship. Students work in groups to ask and address research questions for which the outcomes are unknown and of interest to the broader field (Auchincloss et al., 2014; Brownell and Kloser, 2015; Dolan, 2016; Goodwin et al., 2021). CUREs are generally designed to involve students in discovery through novel questions, broadly relevant work, collaboration, and iteration while engaging in scientific practices (Auchincloss et al., 2014). The instructor in the CURE often acts as the primary mentor for students. However, depending on the design of the CURE, students may also have access to additional members of the scientific community who act as mentors, such as graduate students, postdocs, and more senior undergraduate students (Kirkpatrick et al., 2019). Overall, the structure of CUREs is expected to provide students with more opportunity to act as legitimate peripheral participants compared with inquiry or traditional laboratory courses. It is important to note that CUREs are still formal courses within a term and can take on varying designs (Brownell and Kloser, 2015; Goodwin et al., 2021). Some CUREs function more like a class than a research lab, with students completing pre- and post-lab assignments, receiving credit for attendance, and having designated class times where they work and collect data (e.g., Brownell et al., 2015; Olimpo et al., 2016; Sarmah et al., 2016; Gin et al., 2018). Other CUREs are designed to give students more freedom to come and go when performing research while still completing course work, such as the Freshman Research Initiative at the University of Texas at Austin (Beckham et al., 2015). However, while students in CUREs work within course expectations, the structure of CUREs provides students with the opportunity to participate in the legitimate practices of scientific research and suggests that they will experience other cultural aspects of scientific research and begin the process of border crossing into the field. Given that CUREs tend to provide students with the closest approximation of legitimate peripheral participation outside direct mentorship experiences, these courses are an important context for understanding students’ experiences as they encounter the culture of scientific research. However, little work has investigated which cultural aspects of scientific research students report experiencing in CUREs.
Why Investigate Students’ Experiences of Culture?
Research into peoples’ experiences of culture differs from research exploring cognitive, psychosocial, behavioral, and attitudinal outcomes. Studies focused on outcomes often look for changes in or gains made by students (increases in content knowledge, science identity, etc.). In contrast, focusing on students’ experiences from a cultural standpoint provides a perspective on the underlying interactions that students have with scientific research and their feelings about those interactions. While some studies report no changes in students’ reported outcomes through participation in research (e.g., no change in science identity; Shanle et al., 2016), students may still have learned about the culture of scientific research and developed perceptions of the culture based on their experiences. Additionally, even if students report gains in outcomes such as science identity (e.g., Estrada et al., 2011), they could still be experiencing specific cultural barriers that they may have a hard time overcoming if they continue in science. Exploring students’ perceptions of the culture of scientific research may help explain some cognitive, psychosocial, behavioral, and attitudinal outcomes and will provide additional information regarding students’ experiences of scientific research.
Different Models of CUREs
CUREs have been designed using a variety of different models. Some field-based experiences have been developed that allow students to ask questions about both terrestrial and aquatic ecology (e.g., Kloser et al., 2013; Thompson et al., 2016). However, bench-based and computational models are more commonly described in the literature. Bench-based experiences using bacteria, zebrafish, and other model systems allow students to ask questions about experimental evolution, toxicology, and genetics (e.g., Brownell et al., 2015; Olimpo et al., 2016; Sarmah et al., 2016; Kirkpatrick et al., 2019). Computer-based experiences wherein students perform research investigations using previously collected data and ask questions regarding bioinformatics and genomics have also been developed (e.g., Shaffer et al., 2010; Brown, 2016; Kirkpatrick et al., 2019). Reports from these CURE models suggest that the different models provide students with similar outcomes (Dolan, 2016). Across CURE models, students participating in CUREs have been shown to have increased content knowledge, analytical skills, self-efficacy, persistence and motivation in science, project ownership, and sense of belonging (Dolan, 2016). Attitudinal outcomes have also been compared between students running computer-based projects and bench-based projects within the same course (Kirkpatrick et al., 2019). Kirkpatrick et al. (2019) found that students in the computer-based project area showed equal or higher interest, sense of achievement, and satisfaction with the course compared with students in the bench-based project areas. Overall, these results suggest that students can have similar outcomes from running computational projects versus bench-based projects. However, no work has specifically explored students’ perceptions of the culture of scientific research across these two different CURE models.
Differences between Bench-Based and Computer-Based Scientific Research
Bench-based and computer-based CUREs emulate broader fields and subfields within science. Addressing research questions within subfields of the life sciences, such as microbiology, molecular biology, and experimental evolution, often requires bench-based experimental approaches. These include cell culture, staining, microscopy, polymerase chain reaction, gel electrophoresis, cloning, and competition assays, among many others (Aneja, 2007; Kawecki et al., 2012; Surzycki, 2012). On the other hand, fields such as genomics and computational biology rely heavily on computational practices such as machine learning and simulations to address research questions, (Eraslan et al., 2019; Chelly Dagdia et al., 2021). The approaches to addressing research questions also differ. Differences in practices and approaches can impact how the cultures of these subfields of science look and function (Chelly Dagdia et al., 2021). The differences in the cultures of bench-based and computational-based science fields suggest that students participating in bench-based or computational-based CUREs may have different experiences and perceptions of the culture of scientific research that may impact their border-crossing experiences. This study investigates three research questions:
Which cultural categories and aspects do students experience through participation in a CURE?
How do students’ experiences compare across bench-based and computer-based project areas?
How do students’ perceptions of the cultural aspects they experienced compare across bench-based and computer-based project areas?
METHODS
Study Context
This study was conducted in the context of an introductory biology laboratory course that enrolls between 250 and 350 students per semester at a large midwestern R1 university (10% underrepresented minority [URM], 60% women). The university defines URM as African American/Black, Asian/Pacific Islander, Hawaiian, Hispanic/Latinx, or Native American/Alaska Native. This course is the second in a two-semester sequence that is required of all biology majors at the university, and it is designed as a CURE (Kirkpatrick et al., 2019). Students use scientific practices, collaborate, ask broadly relevant and novel questions, and are given the opportunity for iteration throughout the course. They work in small groups (three to six people) to design and conduct an independent research project over the course of the semester. Additionally, students are given the opportunity to choose one of four project areas and are encouraged to come up with novel research questions within their chosen project areas. These project areas are based on research areas of faculty at the university, adding to the broad relevance of the work students are doing. Three of these project areas are bench-based: Experimental Evolution, in which students use Pseudomonas fluorescens to study questions about evolution; Environmental Toxicology, in which students use zebrafish (Danio rerio) to study the impacts of toxins on behavior and development; and Microbiome, in which students study the impact of various chemical, molecular, and environmental factors on the gut microbiota of zebrafish. The fourth project area, Computational Microbiology, is computer based and allows students to ask questions about the human gut microbiome. All four project areas run concurrently.
Class sections of the CURE course (composed of approximately 20 students per section) are taught by graduate teaching assistants and meet once a week for a 2-hour discussion. In these discussion sections, students learn general techniques they need to complete their projects, such as consideration of experimental design features, proper data management, data analysis, and scientific communication. Students are expected to work on their research projects outside this 2-hour discussion period and to schedule their project work based on their availability and project needs. Students in the bench-based project areas work in a dedicated lab space and are provided with open lab hours (50–60 hours/7 days per week) during which they collect their own data using model systems such as bacteria and zebrafish. Students in the computer-based project area can work anywhere, use publicly available data sets, and focus on learning coding and other analytical strategies to answer their research questions. At the end of the semester, students are required to participate in a public poster session where they present their work. In addition, each student turns in an individual research paper. Students are graded on their effort and presentation rather than the success of their investigations (Kirkpatrick et al., 2019). This study was approved under the University of Minnesota Institutional Review Board no. STUDY00003109.
Participant Recruitment
Participants for this study were recruited using convenience sampling at the required end-of-semester poster sessions. Five different poster sessions are scheduled during a week near the end of each semester. Students choose the poster session at which they will present based on their class schedules. Students can present their posters alone or with group members depending on their schedules. At each poster session, between 17 and 27 posters are arranged around the room with one to three student presenters at each poster. Non-presenting students choose which poster presentations to attend and complete an evaluation rubric on the presentations. Some groups did not have students listening to their presentations for short periods of time. These unoccupied students or groups were approached by the interviewers and asked if they wanted to participate in the study. The computer-based project area tends to have the fewest students enrolled. However, the interviewers tried to recruit students equally across the project areas, even though there was unequal enrollment in the project areas.
In total, 124 interviews were conducted with 192 undergraduates (58% women, 42% men) who agreed to participate in this study in either the Spring 2018 (n = 98) or the Fall 2018 (n = 94) semester. Sixty-one of these interviews were with a single student, while the other 63 interviews were with multiple students presenting at a time. Nearly equivalent percentages of the interviews with the computational and bench-based students were individual versus in groups (computational: 58% individual, 42% group; bench-based: 47% individual, 53% group). Of the 192 student participants, 158 were in one of the three bench-based project areas, and 34 were in the computer-based project area. Given the lower enrollment in the computer-based project area, the 34 students interviewed from the computer-based project area likely represent a greater proportion of the overall population of students in that project area. Although there is a greater proportion of women in the computer-based project area sample, the general makeup of students across the four project area samples was similar for race/ethnicity (Table 1). The 192 participants in this study represented approximately a third of the students across all the poster sessions.
Factor | Project area | |||
---|---|---|---|---|
Experimental Evolution (n = 61) | Environmental Toxicology (n = 55) | Microbiome (n = 42) | Computational Microbiology (n = 34) | |
Gender | ||||
Women | 35 (57%) | 27 (49%) | 25 (60%) | 24 (71%) |
Men | 26 (43%) | 28 (51%) | 17 (40%) | 10 (29%) |
Race | ||||
White | 45 (74%) | 37 (67%) | 30 (71%) | 23 (67%) |
Asian | 9 (15%) | 14 (25%) | 5 (12%) | 7 (21%) |
Black | 5 (8%) | 4 (7%) | 3 (7%) | 2 (6%) |
Hispanic | 2 (3%) | 0 | 1 (2%) | 1 (3%) |
Hawaiian | 0 | 0 | 1 (2%) | 1 (3%) |
Native American | 0 | 0 | 1 (2%) | 0 |
Unknown | 0 | 0 | 1 (2%) | 0 |
Data Collection
Data were collected through interviews conducted during the poster sessions with students who agreed to participate in the study. Students were asked to give their full presentations and answer three interview questions: 1) What did you like most about your experience? 2) What did you find the most challenging about your experience? 3) What will you take away from your experience? Over the course of the two semesters of data collection, there were seven people who helped facilitate student interviews, none of whom were associated with grading. At the beginning of each poster session, an announcement was made to introduce this research project and the associated interviews to the students. The project was presented as a way to help improve the course for future students. It was emphasized that the interviewers were not associated with grading and that any communication of results to instructors would be done after grading was completed and in such a way that students could not be identified. All interviewers participated in a short training session before the poster sessions led by the first author (J.D.). Interviewers were coached on how to approach student presenters, ask for consent from all students present at a poster, and record relevant information (student names, project area, etc.), and when to start the audio recording of the presentation. Interviewers asked all students each of the specific interview questions after students had given their full poster presentations, allowing for responses to be associated with specific individuals. They could ask questions related to content throughout the student presentations.
The three interview questions were purposefully broad and designed to surface aspects of scientific research that students experienced and considered salient without the framework of the educational researchers influencing their responses (Turner, 2010). This open-ended approach was chosen over an approach that included the word “culture” in the interview questions or asked students to identify which of the aspects in the CSR Framework they experienced to avoid biasing students’ responses (Turner, 2010; Creswell, 2013). Additionally, the questions elicited students’ perceptions of their experiences in two ways. The first two interview questions asked for both positive (i.e., the “like” question) and negative (i.e., the “challenge” question) responses to elicit the emotional valence of students’ experiences. The third interview question was designed to elicit what students felt they had learned and found valuable (i.e., the “takeaway” question). The word “takeaway” was used rather than asking students directly what they had learned to avoid biasing responses toward content. The interviews were audio-recorded and transcribed verbatim.
Coding
During coding, a response was defined as a single students’ answer to a single interview question. Because there were three interview questions, each student had the opportunity to give three responses during an interview. Seven students did not provide a response to one of the questions in the interview, resulting in a total of 569 responses.
To analyze students’ interview responses, a codebook was developed based on the CSR Framework (Dewey et al., 2021). Each of the 31 aspects within the CSR Framework was turned into a code with a definition, descriptions of when to use and when not to use the code, and examples from student interviews (Supplemental Tables S1–S3). The codebook was developed collaboratively by the first author (J.D.), who has a doctoral degree in STEM education, and the second author (A.E.), an undergraduate research assistant. The codebook was used to deductively code students’ responses (Miles et al., 2013). A student response could receive multiple codes (e.g., Communication, Freedom & Independence, Persistence & Resilience), but a single code (e.g., Communication) could only be recorded once per response. Responses were able to be associated with specific individuals when multiple students were interviewed at a time. In cases in which students may have been talking over each other or interjecting (32% of the group interviews), the responses were separated, and a new code was only used for individual students if they added additional thoughts to the responses. For example, if Student 1 mentioned “Communication” and a group mate, Student 2, simply agreed, Student 2’s response was not given the Communication code. However, if Student 2 had built on the ideas related to Communication, then the response would be given that code. This conservative approach was used to ensure that each idea that was coded was an independent thought from the students, even though it could have resulted in an undercount of ideas from students in group interviews. However, there were no major differences in the types and frequencies of ideas mentioned by students who did group interviews and students who did interviews alone, and therefore these data were combined.
The first and second authors (J.D. and A.E., respectively) independently coded the interview transcripts, overlapping on 36% (N = 69) of the interviews. Students made references to their project areas in their responses, so coders were not blinded to the project area of each student. After the student response data had been coded, they were transformed into dichotomous data for each of the 31 codes (0 = absent, 1 = present). Given that the majority of these data were 0s, interrater reliability was calculated using Finn’s coefficient, because it accounts for low variance (or high agreement) between raters (Finn, 1970). Percent agreement was also calculated, because Finn’s coefficient is a less commonly used measure of reliability. The two coders had an average Finn’s coefficient of 0.94 (range of 0.8–1.0) and an average percent agreement of 97% (range of 90.6–100%), both of which are very strong. After the interrater reliability was determined, the two coders discussed and resolved any coding disagreements on the interviews on which they overlapped.
Ninety-three percent of students’ responses (529/569) received at least one code. Forty responses (7%) did not receive a code, because they were either a statement of agreement with another student, were unclear, or discussed ideas that fell outside the scope of the coding framework. In total, 785 codes were used, representing an average of 1.4 codes per student response.
Data Analysis
To examine which culture category was most frequently mentioned by students, the coded responses for all interview questions were grouped within the three culture categories of the CSR Framework (i.e., Practices, Norms/Expectations, Values/Beliefs). The percent of total responses for each culture category was calculated. To determine which aspects were most frequently mentioned (independent of category), the coded responses were summed across the interview questions without grouping by category. The percent of total responses for each aspect was calculated.
Next, to explore whether there were differences in students’ reported experiences based on their project area, the coded responses were analyzed by project area. Responses were first sorted by cultural category and summed across the interview questions and cultural aspects within each category to determine whether there were differences in the prevalence of each culture category based on project area. A chi-square test was used to determine whether differences found between the cumulative bench-based responses and the computational responses were statistically significant. Cramer’s V was used to determine the effect size of the chi-square comparison. Then, responses coded as each cultural aspect were summed across the interview questions, and the percent of total responses for each aspect for each project area was calculated to determine the most common aspects that were mentioned by students in each project area. The data for the three bench-based project areas will be presented separately, because while students in these project areas had similar experiences, there were differences.
Finally, to determine whether students’ perceptions of specific cultural aspects differed by project area (i.e., did students talk about specific aspects more as something they liked or found challenging, or as something they learned?), responses that were coded as specific aspects were separated by interview question and project area. The percent of responses coded as a specific aspect for each interview question was calculated for each project area: [(number of responses with code for an interview question/total number of responses with that code) * 100]. For example, of the 26 responses coded as Teamwork for the Experimental Evolution students, 11 were in response to the first interview question (what students liked), [(11/26) * 100] = 42.3%. This calculation allows students’ perceptions of a specific aspect to be compared within each project area (i.e., do students find Teamwork to be more enjoyable or challenging?).
RESULTS
The results section addresses each research question separately. Research question 1 is addressed by examining the overall responses from students, first broadly by cultural category (Practices, Norms/Expectations, Values/Beliefs) and second by cultural aspect. Research question 2 is addressed by comparing students’ responses across project areas, both broadly by cultural category and more specifically by cultural aspects. Research question 3 is addressed by examining students’ perceptions of specific cultural aspects across project areas.
Research Question 1: Which cultural categories and aspects do students experience through participation in a CURE?
Aspects within the Practices category (day-to-day activities or actions of a group) were the most commonly mentioned by students, representing 431 coded responses (54.9% of total; Figure 2). The Norms/Expectations category (the standards that influence how a group and its members think and behave) was the second largest category, with 282 coded responses (35.9% of total). Aspects within the Values/Beliefs category (broad ideas held in esteem and used to define a group) were rarely mentioned (72 responses, 9.2% of total).

FIGURE 2. Percent of student responses in each category of the CSR Framework (N = 785 coded responses). Each category is represented with student quotes.
Of the 31 aspects in the CSR Framework, only two aspects, Negotiation & Debate (Practice, P7) and Science Is Not All-Knowing (Value/Belief, VB3) were not mentioned in students’ responses (Table 2). The most frequently mentioned aspect was the Norm/Expectation of Freedom & Independence (NE7; 151 responses, 19.2% of total) which relates to how scientific researchers should have the freedom to choose what to work on and how to conduct their investigations, but this can be limited by context. The Norm/Expectation of Persistence & Resilience (NE8) was another common cultural aspect mentioned by students (88 responses, 11.2% of total) and relates to the ideas that failing is common in science and researchers must persevere through problems (Table 2). The remaining Norms/Expectations were mentioned in only a handful of responses (one to 11 responses).
Practices | Percent of total codes | Norms/Expectations | Percent of total codes | Values/Beliefs | Percent of total codes |
---|---|---|---|---|---|
Run Investigations (P3) | 16.8% | Freedom & Independence (NE7) | 19.2% | Discovery (VB1) | 3.3% |
Teamwork (P13) | 12.4% | Persistence & Resilience (NE8) | 11.2% | Influenced by/Contributes to Society (VB7) | 3.1% |
Computational Approaches (P10) | 6.6% | Open to New Ideas (NE9) | 1.4% | Curiosity/Imagination (VB5) | 0.8% |
Plan Investigations (P2) | 5.8% | Collaborative (NE6) | 1.4% | Constructive and Complex (VB9) | 0.6% |
Communication (P12) | 5.1% | Integrity (NE2) | 1.0% | Builds on What Has Gone Before (VB8) | 0.5% |
Produce Representations (P8) | 1.9% | Peer Review (NE4) | 0.8% | Empirical Evidence (VB2) | 0.5% |
Obtain and Evaluate Info (P11) | 1.8% | Repeat Investigations (NE3) | 0.6% | Durable but Subject to Change (VB4) | 0.3% |
Analyze Data (P4) | 1.7% | Objective (NE1) | 0.1% | Variety of Methods (VB6) | 0.1% |
Pose Questions (P1) | 1.3% | Publish as Measure of Success (NE5) | 0.1% | Cannot Answer all Questions (VB3) | 0% |
Evaluate and Interpret Data (P5) | 1.1% | ||||
Generate Arguments, Explanations, Conclusions (P6) | 0.3% | ||||
Develop and Use Models (P9) | 0.1% | ||||
Negotiate and Debate (P7) | 0% |
The Practice of Running an Investigation (P3; 132 responses, 16.8% of total) was the second most frequently mentioned aspect overall. This aspect encompasses ideas related to the procedures and techniques students used to collect data as well as the overall process of collecting data (Table 2). Another Practice that comprised more than 10% of the coded responses was Teamwork (P13), which encompasses the actions taken to work in a group to conduct scientific research (97 responses, 12.4% of total; Table 2). Applying and Using Computational Approaches (P10) was also frequently mentioned, with many comments related to coding in R (51 responses, 6.6% of total). Planning Investigations (P2; 45 responses, 5.8% of total) and Communication (P12; 40 responses, 5.1% of total) were other Practices that appeared frequently within students’ responses.
Of the nine aspects in the Values/Beliefs category, students most commonly talked about how Science Is Defined by the Desire to Discover New Knowledge about the Natural World (VB1; 26 responses, 3.3% of total) and Science Is Influenced by and Contributes to Society and Culture (VB7; 24 responses, 3.1% of total; Table 2). These aspects relate, respectively, to how a driving force in science is the discovery of new, sometimes unexpected information, and how science affects and is affected by the various elements and intellectual spheres of the culture in which it is embedded.
While most of the ideas in the CSR Framework were mentioned by students, there were also two common ideas that surfaced from students’ responses that did not fit within the framework: wanting background knowledge or preparation before starting an experiment (nine responses, 1% of total) and participating in research helped students clarify their interests (12 responses, 1.5% of total). While these two ideas are not included in the CSR Framework and are infrequently mentioned, they are still important to some students’ experiences in the specific context of this CURE, and their role could be investigated further using other educational tools.
Research Question 2: How do students’ experiences compare across bench-based and computer-based project areas?
When split by project area, Practices remained the category most commonly mentioned by students, followed by Norms/Expectations. However, there was a statistically significant difference with a moderate effect size between the computer-based and bench-based project areas regarding how frequently the categories were mentioned; χ2(2, 785) = 26.81, p < 0.001, Cramer’s V = 0.18. Practices made up more than 74% of the responses from students in the computational project area compared with an average of 50% (43% to 52%; Table 3) of the responses from students in the bench-based project areas. The proportion of the bench-based students’ responses coded as Norms/Expectations was approximately two times greater than the proportion of the computational students’ responses coded as Norms/Expectations (average of 40% vs. 19%; Table 3). The Values/Beliefs were not as commonly talked about by students in all project areas, representing between 6% and 16% of students’ responses in the bench-based projects and 7% of students’ responses in the computer-based project (Table 3). Within the bench-based project areas, the pattern of student responses was fairly similar, although the Values/Beliefs aspects comprised 16% of Microbiome student responses as compared with 9% for Environmental Toxicology and 6% for Experimental Evolution (Table 3). Given the small number of total responses that were coded as Values/Beliefs for each project area, subsequent results will focus on the Practices and Norms/Expectations categories.
Culture category | Project area | ||||
---|---|---|---|---|---|
Bench based | Average % bench based | Computer based | |||
Experimental Evolution (n = 246) | Environmental Toxicology (n = 219) | Microbiome (n = 177) | Computational Microbiology (n = 143) | ||
Practices | 52% | 55% | 43% | 50% | 74% |
Norms/Expectations | 42% | 36% | 41% | 40% | 19% |
Values/Beliefs | 6% | 9% | 16% | 10% | 7% |
There were also differences in the specific cultural aspects mentioned most often by students who had worked in the computer-based versus bench-based project areas (Table 4). Not only did computational students focus more on Practices overall, but they also mentioned different Practices than those mentioned by bench-based students. For example, computational students frequently mentioned Applying Computational Approaches (P10), while this aspect was never mentioned by the bench-based students. Computational students also mentioned practices such as Data Analysis (P4) and Producing Representations (e.g., images, graphs) (P8) that were infrequently or never mentioned by bench-based students. In contrast, bench-based students talked about Planning Investigations (P2), which was never mentioned by computational students, and Running an Investigation (P3), which was mentioned six to seven times more often by bench-based students as compared with computational students (Table 4). While there were these differences in the saliency of certain Practices across project areas, there were also two aspects that seemed to be equally salient to students regardless of project area: Communication (P12) and Teamwork (P13) (Table 4).
Cultural aspect | Project area | ||||
---|---|---|---|---|---|
Bench based | Average % bench based | Computer based | |||
Experimental Evolution (n = 246) | Environmental Toxicology (n = 219) | Microbiome (n = 177) | Computational Microbiology (n = 143) | ||
Plan Investigations (P2) | 7% | 9% | 6% | 7% | 0% |
Run Investigations (P3) | 22% | 19% | 18% | 20% | 3% |
Analyze Data (P4) | 1% | 1% | 1% | 1% | 6% |
Produce Representations (P8) | 0% | 0% | 0% | 0% | 9% |
Computational Approaches (P10) | 0% | 0% | 0% | 0% | 36% |
Communication (P12) | 7% | 4% | 4% | 5% | 5% |
Teamwork (P13) | 11% | 16% | 10% | 12% | 12% |
Freedom & Independence (NE7) | 22% | 15% | 28% | 22% | 9% |
Persistence & Resilience (NE8) | 12% | 15% | 8% | 12% | 8% |
Research Question 3: How do students’ perceptions of the cultural aspects they experienced compare across project areas?
To investigate students’ perceptions of the cultural aspects they experienced in the CURE, responses coded as specific aspects were analyzed by interview question (i.e., did students mention the aspects more as something they liked, found challenging, or will take away?). Student perceptions of the most prevalent aspects discussed within the Practices and Norms/Expectations categories are presented in the following sections, first by emotional valence, and then by what students valued learning.
Emotional Valence of Students’ Perceptions
During the interviews, students were asked two questions aimed at understanding which features of the students’ experiences they viewed in a positive or negative way: 1) What did you like most about your experience? 2) What did you find the most challenging about your experience? The responses to these questions provided insight into the emotional valence of students’ experiences of scientific research culture. This section will first discuss the emotional valence of Practices that computational and bench-based students mentioned with different saliency. Then, the practices salient to both groups of students will be compared. In representative student quotes, underlining highlights the most relevant portion of the quote.
Emotional Valence of Practices with Different Saliency to Computational and Bench-based Students.
Across both the bench-based and computational groups, students described the Practices that were most salient to them as both enjoyable and challenging. For the computational students, this finding applied to the Practices of Applying Computational Approaches (P10), Producing Representations (P8), and Data Analysis (P4). For example, in response to the question about what the students liked, one student said, “The learning curve [for using R] took a lot of time, but once I did get the hang of it, I could start busting out a bunch of stuff in time. So that was really nice. But it was also hard” (S7). This student enjoyed learning how to use R, but also found the process time-consuming and challenging. In contrast, the most salient Practices for bench-based students were Planning Investigations (P2) and Running an Investigation (P3). These were also described as both enjoyable and challenging. For example, in response to the question about what students liked about their experiences, one student said, “I enjoyed going in [to the lab] and just taking care of the fish and performing the daily routines. That was interesting and really cool to see” (S171, Environmental Toxicology). This student enjoyed being able to physically go into the lab and work on different parts of the project. However, various aspects of running the investigations also presented challenges to some students, with comments in response to the challenge question, such as “We had a lot of issues with contamination. So, we had to redo our entire experiment…and that took a long time” (S51, Experimental Evolution). This student found it difficult to deal with various challenges presented by the experiments, such as bacterial contamination or not knowing if things were being done correctly.
Overall, even though bench-based and computational students focused on different Practices, both groups of students talked about those Practices as both enjoyable and challenging.
Emotional Valence of Practices Salient to Both Computational and Bench-Based Students.
Two practices were mentioned with equal frequency by both computational and bench-based students: Communication (P12) and Teamwork (P13). However, the emotional valence of the perceptions across the two groups differed.
Communication (P12).
Communication (P12) was an aspect mentioned almost equally by students in all four project areas, representing between 4% and 7% of students’ total responses (Table 4). However, bench-based students mentioned this Practice in response to the questions regarding emotional valence (i.e., What did you like? What did you find challenging?), while computational students mentioned this Practice in response to the takeaway question (discussed later). Communication (P12) was mentioned most frequently as something that the bench-based students enjoyed (i.e., in response to the question about what they liked), and they enjoyed both verbal and written communication. For example, one student said, “Being able to come here and visit and talk about all of the work that we did is really rewarding for sure” (S21, Microbiome). Another student said, “I feel like my scientific writing really improved, especially when it comes to figures and writing figure captions and getting concise but still getting the point across” (S32, Environmental Toxicology). These students enjoyed being able to share their work at the poster sessions and develop their skills in scientific writing. A few students mentioned this aspect as a challenge, but this was infrequent (six out of 33 responses). Overall, bench-based students enjoyed the Practice of Communication (P12).
Teamwork (P13).
Teamwork (P13) was an aspect mentioned by students in all four project areas, representing between 10% and 16% of students’ total responses (Table 4). However, the patterns of when and how students talked about Teamwork (P13) varied across project areas. Many bench-based students seemed to enjoy Teamwork (P13). Both bench-based and computer-based students found Teamwork (P13) to be challenging, but they faced different challenges related to working in a group.
When examining all instances where Teamwork (P13) was mentioned, bench-based students often mentioned Teamwork (P13) in response to the question about what they liked (between 28% and 44% of responses mentioning Teamwork; Figure 3). For example, one bench-based student said, “I think it was good to have the experience of working with someone else in a lab. It was nice to come in with other people” (S55, Experimental Evolution). Another student said, “[I like that] through this project I was able to develop my teamworking skill. Because, as you can imagine there’s a lot of lab work that goes into this work. And having a collaborative team effort makes it easier and fun” (S132, Microbiome). These students enjoyed working in groups and learning how to make group work successful and effective in this class. In contrast, only 18% of the computational students’ responses coded as Teamwork (P13; three responses) were in response to the question about what they liked about their experience (Figure 3).

FIGURE 3. Comparison of students’ perceptions of Teamwork; N = total number of responses coded as Teamwork. Those responses were separated by project area, and the percent of those coded responses that were in response to each interview question was calculated.
Across all four project areas, approximately a third of the students’ responses that were coded as Teamwork were in response to the challenge question (Figure 3). However, the types of challenges that students faced differed. One computational student said, “Coding isn’t very conducive to group work essentially because you have to do it pretty individually to get any sort of results, so it’s kind of difficult to work in a group, but we managed it really well” (S177). Another student made a similar comment, saying, “Collaboration is kind of difficult in computational [work] as well, because some people understand how to communicate with one program more than others. Or the other problem is [that] everyone wants to contribute obviously, but some people’s programs don’t run” (S188). These students found that it was difficult to run a computational project as a group, because coding and using different programs tended to be very individual practices. In comparison, bench-based students tended to have more difficulty with group coordination. For example, one student said, “Trying to be flexible with time [was challenging], because if one person says ‘oh, I can’t actually make it into the lab today,’ then everyone’s like ‘oh, I’m in class too,’ and it’s hard to work around that” (S4, Environmental Toxicology). A different student said, “I would say coordination with group members can be hard, because I found in my group that… it kind of seemed like most people weren’t very willing to put in extra work when there were challenges. So, that was a little frustrating” (S97, Experimental Evolution). Bench-based students focused more on the difficulties associated with making sure people went into the lab to complete the experiments. Overall, bench-based students enjoyed Teamwork more than computational students. Both groups of students found Teamwork to be challenging, but they faced different challenges when working in a group.
Emotional Valence of Norms/Expectations.
There were two main Norms/Expectations mentioned by students in all four project areas: Freedom & Independence (NE7) and Persistence & Resilience (NE8). However, there were important differences in students’ perceptions of these two aspects.
Freedom & Independence (NE7).
Freedom & Independence (NE7) represented between 15% and 28% of the responses from bench-based students and 9% of the responses from computational students (Table 4). The bench-based students enjoyed Freedom & Independence (NE7) more than the computational students, and the bench-based students reported less frequently than computational students that Freedom & Independence (NE7) was challenging.
Over half of the time that students in the Experimental Evolution and Environmental Toxicology project areas mentioned Freedom & Independence (NE7), it was in response to the question about what they liked about the research experience (Figure 4). For example, one student said, “I definitely liked the freedom that we had to develop our own experiment. I thought that was really exciting” (S149, Environmental Toxicology). Another student said, “It was interesting to be able to do our own thing instead of being told what to do” (S65, Experimental Evolution). Thirty-four percent of Microbiome students’ responses containing this aspect were in response to the like question. One Microbiome student said, “I liked being able to come up with my own question, something that was interesting to me and being able to choose where I wanted to go” (S127, Microbiome). In contrast, only 15% of computational students’ responses were in response to what they liked: “[I like that] there’s a lot of flexibility in what you can study” (S189).
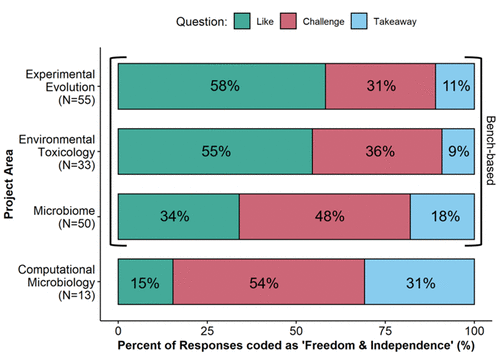
FIGURE 4. Comparison of students’ perceptions of Freedom & Independence; N = total number of responses coded as Freedom & Independence. These responses were separated by project area, and the percent of those coded responses that were in response to each interview question was calculated.
Over half of the computational students’ responses that were coded as Freedom & Independence were in response to the challenge question (Figure 4). One student said, “A lot of the stuff that we had to do we weren’t explicitly taught in class. We were just expected to figure it out, which was fine because there is a lot of information to teach about this stuff. But that was definitely frustrating at times” (S139). This sentiment was common, in that students found it very challenging to be asked to learn how to code and run a computational project more independently. Of the responses that were coded as Freedom & Independence for bench-based students, only between 31% and 36% of those from the Experimental Evolution and Environmental Toxicology groups were in response to the challenge question (Figure 4). One student said, “I would say [it was a] challenge to kind of just be thrown in the lab. It’s your own experimental design. You just take it and run with it” (S75, Environmental Toxicology). In contrast, in the Microbiome project, approximately 48% of responses touching upon this aspect were in response to the challenge question. One Microbiome student said, “I like the independence, but with that comes challenges. If you have a problem, the TAs are there but you have to kind of figure it out yourself. So, there were a lot of times where we would kind of be delayed a couple of days because we did something incorrectly” (S111, Microbiome). These students also found it challenging to have so much independence when working in the lab. Overall, students in the bench-based projects enjoyed Freedom & Independence (NE7) and students in two of the bench-based groups mentioned this aspect as challenging less frequently than the computational students.
Persistence & Resilience (NE8).
Persistence & Resilience (NE8) represented between 8% and 15% of students’ responses across the four project areas (Table 4). Computational students found Persistence & Resilience (NE8) to be more of a challenge compared with the bench-based students. Neither group of students mentioned this aspect as something they liked very frequently.
Two-thirds of the computational students’ responses that were coded as Persistence & Resilience (NE8) were in response to the challenge question (Figure 5). For example, one student said, “You run through errors that you don’t really expect to run into. You type out the code and you miss a comma or a period or something. Something super common like that, and then it throws off the whole thing, and you have to look back at the whole thing [line by line], and kind of pick out what you did wrong” (S85). Another student said, “The troubleshooting was the most difficult because programming is so foreign that you don’t really know what to do, and you’re just doing a ton of Google search to try to problem solve” (S115). For these students, running into coding errors and having to work through them on their own was very challenging.
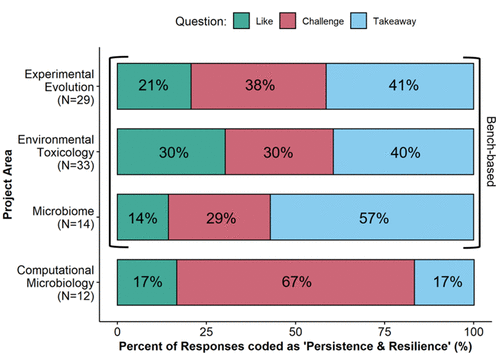
FIGURE 5. Comparison of students’ perceptions of Persistence & Resilience; N = total number of responses coded as Persistence & Resilience. Those responses were separated by project area, and the percent of those coded responses that were in response to each interview question was calculated.
Students in the bench-based project areas found Persistence & Resilience to be less challenging. On average, a third of the bench-based students’ responses that were coded as Persistence & Resilience were in response to the challenge question (average of 32% of responses mentioning Persistence & Resilience; Figure 5). One student said, “It can be challenging when you fail and you’re like ‘Okay, what did we do wrong? What can we do different? We still have time, so let’s try and make a change and get better results for our research paper’” (S8, Experimental Evolution). Another student said, “I found it most challenging when an error would occur, going back and thinking about what [happened]” (S84, Experimental Evolution). The bench-based students also found it challenging to deal with issues or mistakes when they happened. Overall, the computational students found Persistence & Resilience (NE8) to be more of a challenge than the bench-based students, and rarely did either group find this aspect to be enjoyable.
Students’ Perceived Valuable Learning Experiences
During the interviews, students were also asked a question that aimed to identify what they perceived to be valuable learning experiences (i.e., What will you take away from your experience?) The responses to these questions highlighted the aspects that students perceived as valuable. Overall, students mentioned many of the same aspects discussed earlier as takeaways as well, reinforcing the saliency of these aspects to students. When comparing across project areas, bench-based students mentioned Planning Investigations (P2) and Running an Investigation (P3) as Practices they valued learning, while computational students mentioned Producing Representations (P8) and Computational Approaches (P10) as Practices they valued learning. The remainder of the results will focus on the nuanced differences in how bench-based and computational students talked about the same aspects as takeaways.
Perceptions of Practices.
The two Practices that both bench-based and computational students mentioned as takeaways were Communication (P12) and Teamwork (P13).
Communication (P12).
Most of the responses from students that were coded as Communication (P12) were in response to the question about what students would take away from their experiences. In fact, every mention of the Practice of Communication (P12) from computational students was about it being a takeaway. Computational students made comments such as “Learning how to write scientifically” (S188) and “I’ll take away this presenting aspect, [because] I haven’t really had to present to a ton of people in the past” (S85), showing that they valued learning how to communicate their work to others. Bench-based students mentioned Communication (P12) as a takeaway half as frequently as computational students but made similar comments. Overall, computational students seemed to value learning to communicate a bit more compared with the bench-based students.
Teamwork (P13).
Computational students also seemed to value learning to work in teams more compared with bench-based students. Nearly half of the computational students’ responses that were coded as Teamwork were in response to the takeaway question (Figure 3). For example, in response to this question, one student said, “Since we weren’t actually doing something in a lab and it was all hands-on, it was very collaborative. We got really good at talking to each other, even outside of lab, to get things done” (S116). This student found value in the work done with the group to complete the computational research project and felt group members learned to be good at working with one another. In contrast, about a third of the responses from bench-based students that were coded as Teamwork (P13) were in response to the takeaway question (Figure 3). One student said, “Just learning how to work with other people and to keep an open mind, that’s the biggest thing” (S76, Environmental Toxicology). This student valued learning how to work in a group with different people. Overall, computational students mentioned Teamwork (P13) as something that they took away from the experience about twice as often as bench-based students.
Perceptions of Norms/Expectations.
Computational and bench-based students also differed in how frequently they mentioned different Norms/Expectations as something they would take away from their experiences.
Freedom & Independence (NE7).
Freedom & Independence represented a greater proportion of the computational students’ responses to the takeaway question compared with the bench-based students (31% for computational students vs. 9–18% for bench-based students; Figure 4). For example, in response to this question, one computational student said, “It was a good experience to know that we are able to do a more long-term project, kind of on our own. We did have guidance, obviously, but a lot of it was done by ourselves” (S79). This student acknowledged having the ability to work on a long-term project independently and valued that learning experience. While some bench-based students also mentioned this aspect as a takeaway, it was less frequent. One student said, “I was going to say, just like actually doing an experiment on your own and not being instructed on what you’re doing is a big takeaway. Figuring out yourself… Just kind of, instructing yourself” (S68, Experimental Evolution), indicating the student also valued learning to run an experiment independently. However, overall, the computational students found this aspect to be more of an important lesson that they took from the experience compared with the bench-based students.
Persistence & Resilience (NE8).
On average, bench-based students talked about Persistence & Resilience (NE8) as a takeaway three times more than computational students (Figure 5). Between 40% and 57% of the bench-based students’ responses that were coded as Persistence & Resilience (NE8) were about this aspect being valuable to learn, while computational students only mentioned this aspect as a takeaway twice. One bench-based student said, “I think [I’ll take away] the idea that things don’t always go to plan… And it’s kind of a realization that this happens in the real world, too. Scientists have to redo things a lot, and their hypotheses are going to be wrong. It just happens” (S25, Environmental Toxicology). Another bench-based student said, “It’s okay to fail as long as you keep trying” (S129, Microbiome). Unlike the computational students, bench-based students reported that they learned that there was value in failing and trying again.
DISCUSSION
This paper explored the cultural aspects of scientific research that students experience through participation in a CURE and how undergraduates who participated in bench-based and computer-based CUREs experienced and perceived the culture of scientific research. The results of this study show that a small number of cultural aspects were especially salient to students. Additionally, this study shows that different aspects were salient to students depending on the CURE model in which they participated. Finally, this study showed that students also had different perceptions of the cultural aspects of scientific research that they experienced.
Only a Few Cultural Aspects of Scientific Research Were Salient to Students
Of the 785 coded responses collected from students in this study, 90% were about either the Practices or Norms/Expectations of scientific research, and students talked about Practices 1.5 times more frequently than Norms/Expectations (Figure 2). This finding is not surprising, considering the context for the study was an introductory biology laboratory course. While this was the second of two required courses, students were still learning a variety of techniques for the first time and practicing skills that they may not have used before (e.g., presenting a poster or writing a full scientific paper). When starting in a new field, the Practices would be the first thing students learn about and try to master before learning about the broader standards (Norms/Expectations) and defining characteristics (Values/Beliefs) of that field (Lave and Wenger, 1991; Auchincloss et al., 2014).
Of the 31 aspects in the CSR Framework, only two were not mentioned by students in this study: The Practice of Negotiation & Debate (P7), which relates to how scientists justify, evaluate, revisit, and rebut claims, discuss observations, listen to criticism, and engage in persuasion to resolve disagreements when trying to reach explanations of data, and the Value/Belief that Science Is Not All-Knowing (VB3), which relates to how science cannot answer all questions (Dewey et al., 2021). This finding suggests that these CURE experiences do generally reflect the culture of scientific research as experienced by academic scientific researchers (Dewey et al., 2021). Of the 29 aspects that were mentioned by students, seven aspects represented nearly 78% of students’ coded responses: Freedom & Independence (NE7), Running an Investigation (P3), Teamwork (P13), Persistence & Resilience (NE8), Computational Approaches (P10), Planning Investigations (P2), and Communication (P12) (Table 2). This result implies that many of the cultural aspects of scientific research were not very salient to most students after the CURE experience.
However, students may still have experienced those aspects. For example, all students in this course had the opportunity to engage in the Practice of Negotiation & Debate (P7) with their classmates when discussing the data they collected and interpreting the data. There may not have been much disagreement among students, resulting in limited debate. Alternatively, students may not have realized that they were negotiating and debating with their group mates when they were discussing interpretations of data. For either of these reasons, Negotiation & Debate (P7) might not have been recognized as a salient part of the students’ experiences. Similarly, all students in this course were expected to analyze the data they collected from their investigations; however, Data Analysis (P4) was only mentioned in a small proportion of students’ responses (1.7% of total; Table 2). The salience of this aspect may have been diminished, because it is a common Practice in laboratory exercises (Buck et al., 2008; Auchincloss et al., 2014) and something that students may expect to occur and thus was not as surprising or memorable to them when it did occur. Finally, while students found Teamwork (P13) to be very salient to their experiences, they did not talk very frequently about the Norm/Expectation of Collaboration (NE6), which is a broader expectation of the scientific community at large and involves cooperation at many different levels across disciplines and fields. Students may not have found this idea to be salient because they mainly worked with classmates in their small groups on their projects. They did not work with other small groups to design projects or share data. Broader collaboration, for example, working with course coordinators or others to obtain necessary materials for their projects, may not have been interpreted as collaboration, or happened infrequently and was therefore not salient.
The top four aspects mentioned by students were Freedom & Independence (NE7), Running an Investigation (P3), Teamwork (P13), and Persistence & Resilience (NE8) (Table 2). CUREs are designed to involve students in the use of scientific practices, discovery, broadly relevant work, collaboration, and iteration (Auchincloss et al., 2014). Therefore, students’ focus on running experiments, working in a group, and having to work through difficulties or problems aligns with the goals of CUREs. Similar results have been shown by others using the Laboratory Course Assessment Survey, a tool that specifically measures students’ perceptions of if and how frequently they participated in three of the design features of CUREs (collaboration, discovery, and iteration; Corwin et al., 2015; Cooper et al., 2019; Goodwin et al., 2021; Lo and Le, 2021). However, while the five design features of CUREs are helpful for distinguishing them from other laboratory courses, they provide a limited view of students’ potential experiences in a CURE. The CSR Framework provides a broader theoretical framework based on the literature that can be used to examine and compare students’ research experiences across multiple contexts. As this study shows, this broader framework captures the design features of a CURE, but also identifies other aspects students may experience (e.g., Norms/Expectations such as scientists must be Open to New Ideas [NE9]) that may not have been considered within the narrower lens of CURE design features. The broader framework also identifies aspects of the culture of scientific research that many students are not recognizing as salient but that might be desired as salient course outcomes (e.g., Develop and Use Models [P9] or many of the Values/Beliefs aspects).
Bench-Based and Computational Students Did Not Have the Same Experiences and Perceptions of Scientific Research
There were some similarities regarding the aspects mentioned by bench-based and computational students (i.e., Teamwork [P13], Communication [P12], Freedom & Independence [NE7], Persistence & Resilience [NE8]). The biggest difference between students’ experiences was that bench-based students discussed the practices of Planning Investigations (P2) and Running an Investigation (P3) in the context of doing hands-on lab work, while computational students focused instead on the practices of Data Analysis (P4), Producing Representations (P8), and Computational Approaches (P10) in the context of doing computational work. While computational students did still plan out and run an investigation using publicly available data, these practices were not very salient to the students and were instead overshadowed by the computational practices required for their investigations. Running an Investigation (P3) is more typically associated with hands-on work done in a lab to complete experiments, so the computational students in this study may not have realized they were still running their own investigations (Kirkpatrick et al., 2019). Additionally, computational students were using previously collected data, which may have contributed to the reduced saliency of planning and running an investigation. Bench-based students, like computational students, were asked to produce representations of their data and perform basic analyses of the data they collected. However, these were not as salient to the bench-based students and were overshadowed by their hands-on experiences of planning and running their investigations.
These findings are not surprising, given the fact that bench-based and computer-based subfields of science, on which the different CURE models are based, use very different practices to address their research questions (Aneja, 2007; Kawecki et al., 2012; Surzycki, 2012; Eraslan et al., 2019; Chelly Dagdia et al., 2021). However, these findings are different from much of the previous work investigating student outcomes in CUREs. Previous work, including a study conducted in the same context as this study, has found that students have similar attitudinal, cognitive, affective, and behavioral outcomes between bench-based and computer-based CURE models (Dolan, 2016; Kirkpatrick et al., 2019). However, while students across project areas may have similar attitudinal outcomes from this course, computational students may walk away from the course with a different impression of what it means to do scientific research, given their focus on computational practices. While these Practices could have been examined separately from a culture framework, the CSR Framework identifies Practices that are considered relevant across a broad spectrum of the sciences. Additionally, all of the Practices in the CSR Framework were considered to be part of biology researchers’ understanding of the culture of scientific research across subdisciplines of biology (Dewey et al., 2021). Therefore, using the CSR Framework allows for the consideration of which Practices students are experiencing as salient in different contexts and courses.
Given the variable design of CUREs, it is possible that the responses collected from students in this CURE would differ from responses from students participating in different computational and bench-based CUREs. For example, students participating in a CURE with substantially fewer opportunities for student independence and a more consistent weekly structure might discuss Freedom & Independence (NE7) less frequently than the students in this study. Additionally, students participating in a different computational CURE that has less emphasis on coding might talk about Computational Approaches (P10) less frequently or in different ways and might see their work as more of an investigation than the computational students in this study. Ultimately, more work needs to be done to compare students’ experiences of the scientific research culture across different CURE courses.
Students’ perceptions of the aspects they found salient, both regarding their emotional valence and what they valued learning, also differed across bench-based and computational students. Differences in the emotional valence of students’ experiences are important to consider, because the positive and negative experiences that students have of specific cultural aspects may indicate where students are experiencing entry points or barriers to border crossing in these courses (Aikenhead, 1996). For example, bench-based students enjoyed the Practice of Teamwork (P13) more than computational students, potentially suggesting that working with others was more of an entry point for bench-based students. Both groups of students found Teamwork (P13) to be challenging but in different ways, suggesting that Teamwork (P13) could act as a barrier in different ways for students depending on which CURE model they are enrolled in, either through interpersonal interactions or the mechanisms required to perform the research. Computational students also found the Norms/Expectations of Freedom & Independence (NE7) and Persistence & Resilience (NE8) to be more challenging than the bench-based students (Figures 4 and 5). This suggests that computational students may have experienced these aspects as more of a barrier than the bench-based students. While some students’ responses could have reflected their self-selection into a project area (e.g., students who wanted to learn how to code chose the computational project area and therefore talked about this Practice frequently), we do not believe this impact is widespread. Students would not have known how the different project areas would impact their experiences of aspects such as Freedom & Independence (NE7) or Persistence & Resilience (NE8), and therefore their perceptions of these aspects would not reflect their choice of project area. Previous work in the same context also showed no difference in interest, sense of achievement, or course satisfaction between students who chose their project area and students who were placed into a project area (Kirkpatrick et al., 2019). To further explore whether the differences seen between computational and bench-based students were due to the project area or individual differences independent of the project area, future work could investigate whether these differences hold up when students do not have a choice of project area.
It is also important to consider which aspects students considered takeaways, because those are the aspects that students have internalized and recognized as learning experiences. Students will likely take these aspects with them into their future experiences of scientific research, and they could potentially be leveraged to help students’ border crossing. For example, computational students valued learning about Teamwork (P13) and Freedom & Independence (NE7) more than the bench-based students. Computational students reported that they were able to work through the challenges posed by working with others and having more freedom and recognized that they learned from those experiences more than the bench-based students. In contrast, the bench-based students valued learning about Persistence & Resilience (NE8) much more compared with computational students. The bench-based students may have moved from recognizing the challenge of pushing through failure to recognizing that they learned from those experiences, while the computational students might be stuck on the challenge of pushing through failures and have not quite managed to reconcile it into a learning experience (Henry et al., 2019). Computational and bench-based students may move into their next research experiences with different understandings of scientific research and different strengths based on their participation in different CURE models.
IMPLICATIONS FOR COURSE DESIGN
This paper describes the first use of a cultural border-crossing lens to explore which aspects of the culture of scientific research students experience in a CURE and how different CURE models may impact students’ experiences and perceptions of those cultural aspects. CUREs have the potential to provide a larger, more diverse population of students with the opportunity to become legitimate peripheral participants in scientific research. However, this study has shown that students may not find many of the cultural aspects of scientific research to be salient after participating in a single CURE. For example, most students in this study did not find the Values/Beliefs of scientific research to be very salient. These results have implications for thinking about the design of individual CURE courses as well as the incorporation of CUREs in the undergraduate curriculum. If a major goal of a CURE is to engage students with many of the cultural aspects of scientific research as they exist in the current system, efforts need to be made to make these aspects more salient for students. This could be done through discussions and reflections in class. However, it is impossible to expect students to learn about the entirety of the culture of scientific research in a single class. One possible solution could be expanding CUREs throughout the undergraduate curriculum and designing them in ways that help students build up their understanding of these aspects over time. This could provide a viable alternative to direct mentorship that may persist for multiple semesters.
The different experiences that students had in the computational and bench-based project areas are also important to consider when thinking about course design and student outcomes. While some CUREs may be designed to meet specific goals, constraints such as time, space, and money often dictate how CUREs are designed (Kirkpatrick et al., 2019). Students in this study were given the opportunity to choose the type of research they wanted to perform (i.e., bench based or computer based). However, most CUREs are designed using only one of these models, and students in these courses generally cannot choose a specific CURE model. These findings suggest that curriculum designers may find it beneficial to include multiple CURE models throughout the curriculum and require students to take one of each type. The challenges highlighted by students in the different project areas suggest that instructors may need to provide different supports within the curriculum for students based on the type of research they are conducting. Students with different backgrounds and interests may experience different cultural barriers and entry points when participating in bench-based or computer-based CURE models, which ultimately may impact their border crossing into scientific research. Future work identifying the cultural barriers and entry points that different groups of students in different CURE models experience could help in designing targeted supports within the curriculum to improve the border crossing and success in scientific research for all students.
MOVING MORE CRITICALLY TOWARD CHANGE
The Implications section describes ways to use the CSR Framework to guide CURE design. There is value in this approach, because it provides a way to help more students successfully move into the current system of scientific research. However, only using the CSR Framework to guide CURE design will result in the perpetuation of the current system and will not address the underlying systemic problems within the culture of academic scientific research (systemic racism, sexism, etc.). We do not believe that this should be the ultimate application of the CSR Framework. Instead, we propose that the CSR Framework be used in multiple ways. Changing the design of CUREs to help students move into the current culture of scientific research can be used as a stopgap measure while other work progresses toward changing the system itself. The CSR Framework can be used in a more critical, layered way along with analytical tools and frameworks that examine broader layers of culture, mentoring, and forms of discrimination to identify the problematic aspects of the scientific research culture that should be changed to help make scientific research more inclusive. Identification of the aspects that students are experiencing in CUREs and how they are experiencing them will ideally lead to the identification of both supportive cultural aspects as well as problematic, exclusive cultural aspects of scientific research. Then, the field should work toward actionable ways that these problematic aspects could be changed to create an inclusive culture of scientific research.
LIMITATIONS OF THIS STUDY
This study was done in the context of a single CURE course at one institution, limiting the generalizability of the results. Future work should be done to determine whether students taking CURE courses at other institutions would have similar or different experiences and perceptions of the culture of scientific research. Additionally, the student participants in this study were largely white, reflecting the dominant racial group in science. It will be important for future work to investigate similar questions with more diverse student populations to determine how the experiences and perceptions of students highlighted in this work compare with other groups.
Data for this study were collected through interviews with students using broad, open-ended questions. Students were not asked specifically about the culture of scientific research or specific cultural aspects within the CSR Framework, but rather were asked questions to elicit the most salient parts of their experiences. Future work using surveys that specifically ask students about the cultural aspects of scientific research would provide additional insight into students’ experiences. Additionally, the public nature of the interviews could have impacted who decided to participate in this study and could have made some students less comfortable talking about their experiences with us. This in turn could have reduced the number and types of ideas that students chose to share during the interviews. Future work using both similar and different methods of data collection could help to parse out the impact of the interview environment on students.
This study did not explore students’ experiences and perceptions based on their identities (e.g., gender identity, race/ethnicity). Understanding similarities and differences between the experiences and perceptions of students with different identities, as well as the experiences of students with intersecting identities, is important for understanding the interactions between students’ home cultures and the culture of scientific research. Understanding individualized experiences is especially important for identifying barriers and entry points experienced by students with different identities and understanding undergraduates’ experiences of border crossing into the culture of scientific research which reflects white, male, Western worldviews (Anzaldúa, 1987; Aikenhead, 1996; Seymour and Hewitt, 1997; Carlone and Johnson, 2012). Work exploring the intersection between students’ identities and CURE models would help tease apart students’ experiences more completely. Currently, students’ gendered experiences and perceptions of the culture of scientific research in this CURE are being examined, and alternative approaches are being developed to gather data from students from additional underrepresented backgrounds.
CONCLUSION
The application of the CSR Framework in this study is the first step in a process of examining experiences of the culture of scientific research and identifying ways to make it more inclusive. The critical examination of the important and nuanced ways that students experience the culture of scientific research in courses such as CUREs can provide guidance on how to help students enter the current system of scientific research. Concurrently, more work needs to be done using the CSR Framework in conjunction with other frameworks to identify aspects of the scientific research culture (and thus aspects of the system) that need to be changed to create a more inclusive system for students to enter. This layered approach will ideally lead to an increase in the success of all students entering the scientific research field both now and in the future.