Simulating a Computational Biological Model, Rather Than Reading, Elicits Changes in Brain Activity during Biological Reasoning
Abstract
The creation and analysis of models is integral to all scientific disciplines, and modeling is considered a core competency in undergraduate biology education. There remains a gap in understanding how modeling activities may support changes in students’ neural representations. The aim of this study was to evaluate the effects of simulating a model on undergraduates’ behavioral accuracy and neural response patterns when reasoning about biological systems. During brief tutorials, students (n = 30) either simulated a computer model or read expert analysis of a gene regulatory system. Subsequently, students underwent functional magnetic resonance imaging while responding to system-specific questions and system-general questions about modeling concepts. Although groups showed similar behavioral accuracy, the Simulate group showed higher levels of activation than the Read group in right cuneal and postcentral regions during the system-specific task and in the posterior insula and cingulate gyrus during the system-general task. Students’ behavioral accuracy during the system-specific task correlated with lateral prefrontal brain activity independent of instruction group. Findings highlight the sensitivity of neuroimaging methods for identifying changes in representations that may not be evident at the behavioral level. This work provides a foundation for research on how distinct pedagogical approaches may affect the neural networks students engage when reasoning about biological phenomena.
INTRODUCTION
Modeling is a skill that allows scientists to explore complex biological systems, synthesize scientific concepts, test hypotheses, generate causal explanations, and identify gaps in knowledge (Odenbaugh, 2005; Nersessian, 2009; Svoboda and Passmore, 2013). Modeling and model literacy are considered core science competencies for primary to postsecondary students in the United States, and training in modeling and model-based reasoning is emphasized strongly in national standards for science education (American Association for the Advancement of Science, 2011; Brown et al., 2018). While models may take multiple forms, simulation-based models are gaining popularity in undergraduate life sciences education, because they allow students to experiment with mathematical and computational manipulations that expose the complex, nonlinear dynamics of biological systems (Abou-Jaoudé et al., 2016). For instance, students might construct a representation of a genetic operon on the computer, then manipulate components of the operon, viewing real-time changes to the system dynamics in graphical and numerical format as a result of their manipulations. While recent reviews have examined the implications of modeling and model-based learning experiences for students’ behavioral understanding of scientific concepts and systems, including their drawn or written work related to modeling (Louca and Zacharia, 2012; Seel, 2017), there are few studies on the neural mechanisms of scientific reasoning, let alone modeling (Brewe et al., 2018; Nenciovici et al., 2019). The current study addressed this gap by comparing the functional neural activity of undergraduate life sciences students who were briefly exposed to a modeling simulation activity versus traditional, reading-based instruction.
Modeling-based instruction (MBI) is a knowledge-building endeavor wherein students generate hypotheses about the mechanisms of scientific phenomena either through expert-provided, preconstructed models, or by generating their own testable models, and then interpret the results relative to the biological mechanisms (Windschitl et al., 2008). Coupling modeling with simulations allows students to evaluate the results of their model manipulations against expected outcomes, that is to say, to retrieve prior relevant knowledge and connect it to new knowledge (Soderberg and Price, 2003; Seel, 2017; Dauer et al., 2019). Theoretically, then, modeling can lead to deep disciplinary understanding as students integrate existing and new knowledge structures to build more sophisticated, connected representations of biological systems and their interactions (Smetana and Bell, 2012; Mulder et al., 2016; Seel, 2017). Accordingly, limited research suggests that undergraduate science, technology, engineering, and mathematics (STEM) students exposed to MBI curricula show improved levels of conceptual understanding, more sophisticated inquiry-based reasoning, and improved levels of knowledge about models, including their scientific purpose and utility (Bray Speth et al., 2009; Brewe et al., 2009; Brewe and Sawtelle, 2018; Ogan-Bekiroğlu and Arslan, 2014; Shen et al., 2014; Helikar et al., 2015). Much of the research on MBI has been qualitative and lacking a control or comparison population against which relative impacts or effect sizes can be compared. There is a need for rigorous research both to quantify the effects of MBI relative to other pedagogical techniques and to articulate the mechanisms by which MBI might enhance student learning.
Previous research on MBI found that students initially focus on components, that is, the individual nodes within the models that describe the organisms or molecules that are interacting (Hmelo-Silver, 2004). With repeated modeling practice, students increasingly focus on the relationships among components within the model as they shift toward the interactive dynamics of these components (Hmelo-Silver, 2004; Dauer et al., 2013; Bergan-Roller et al., 2020). In a study comparing student dyads who constructed computational models with those who ran simulations with preconstructed computational models, there were few differences in postlesson conceptual models between the groups (King et al., 2019a). Instead, differences emerged in the cognitive processes employed during the lesson. Model simulation groups tended to rely on surface-level cognitive processes like paraphrasing and analyzing and focused their discussions on identifying components and relationships of the model rather than determining the causal mechanisms from the relationships. Model construction groups, in contrast, focused more on the underlying causal relations among system components, suggesting that the application of more complex inductive and evaluative reasoning about system dynamics can be fostered through modeling-based pedagogical techniques. Overall, then, findings suggest that the effects of MBI are not always evident in simple measures of student performance on conceptual modeling tasks, but instead may manifest in the engagement of different strategies or modes of reasoning.
The Roles of Hypothesis Generation and Causal Reasoning in Modeling
Both hypothesis generation (“the outcome of a system perturbation will be X because of Y”) and causal reasoning (“when X changes, Y and Z change because X is linked to Y and Z through…”) are critically relevant to student modeling of complex biological systems (Sweeney and Sterman, 2007; Windschitl et al., 2008). In the context of modeling, causal reasoning encompasses the idea that changes in the abundance of components or strength of relationships within a biological system will have direct and indirect effects on other system components and on overall system function (Grotzer et al., 2017). Causal reasoning is often the first step in understanding how system components interact (Fugelsang and Mareschal, 2014). From a cognitive neuroscience perspective, causal reasoning encompasses flexible attentional shifting (from perturbation to target effects), retrieval of prior knowledge about system components and relationships, and dynamic processing of system observations in relation to prior knowledge (Nenciovici et al., 2018). Modeling-based instructional activities allow students to explore the outcomes of perturbations through manipulations of system components and therefore are likely to inspire the use of causal reasoning as students evaluate and explain these effects.
Similarly, hypothesis generation using models involves predicting system behaviors and observing outcomes based on these predictions. Students must retrieve knowledge of the system, infer or retrieve relationships among components in the system, and evaluate the consequences of the associations. Löhner et al. (2005) compared student groups who used graphical interfaces with those who read about physics phenomena and found that the former developed more qualitative hypotheses and follow-up experiments, while the latter tested hypotheses, but failed to update these hypotheses based on observations. Behaviorally, students were better prepared to generate hypotheses if they practiced this skill as opposed to just receiving an explanation of what hypothesis testing involves (Kwon et al., 2009). These studies suggest that active model manipulation and testing encourages students to engage in hypothesis generation to a greater extent than simple, direct instruction.
Learning Transfer from System-Specific Contexts to System-General Contexts
Although existing literature suggests that MBI inspires the use of more complex forms of reasoning and hypothesizing with respect to the modeled system, an unanswered question is the extent to which MBI promotes the transfer of learning to new, unencountered biological systems. Theoretically, the emphasis of MBI on the dynamic interactions among system components should encourage students to generalize understanding of complex inhibitory or excitatory transactions to novel systems. By learning about inhibitory feedback loops in one genetic system, for instance, students may be more likely to understand how inhibitory feedback loops regulate biological systems at a more general level. This transfer of information from one system to another likely places high demands on analogical reasoning, or the appreciation of underlying relations between two systems that appear different at the surface level (Luo et al., 2003; Fugelsang and Mareschal, 2014; Vendetti et al., 2015). Such analogical reasoning relies on the ability to align representations or relationally map the novel and familiar systems and abstract the commonalities across these systems (Gentner and Colhoun, 2010). The question of whether model-based learning encourages such relational abstraction is critical, given that transfer of learning to new contexts is a central goal in undergraduate education.
Neuroimaging as a Tool to Evaluate the Effects of STEM Instructional Interventions
While intensive qualitative analysis of behavioral data has yielded insight into the effects of MBI on students’ use of different strategies and reasoning processes, neuroimaging offers a novel means of understanding how experiences with MBI may impact student processing at the more direct level of the brain. Functional magnetic resonance imaging (fMRI) is a particularly powerful tool for evaluating changes in the spatial distribution of neural activity in response to instruction. Characterization of the neural networks involved in scientific reasoning is a relatively new endeavor, although studies from the last 25 years have established the ability of fMRI to provide insight into the neural bases of scientific reasoning and the effects of different instructional formats on these neural mechanisms (e.g., Masson et al., 2014; Kontra et al., 2015; Mason and Just, 2015; Bartley et al., 2019; Schwettmann et al., 2019). For example, Kontra et al. (2015) used fMRI to examine the impact of different instructional methods in physics. Undergraduates who actively manipulated objects not only showed greater behavioral performance, but also increased blood oxygenation level–dependent (BOLD) signal in the sensorimotor, superior parietal, superior and inferior frontal, and superior temporal regions, relative to those taught using traditional, expository methods. The researchers argued that the experience of manipulating objects afforded greater representation of the dynamic aspects of torque and angular momentum in sensorimotor neural regions, which then aided retrieval. This study illustrates the promise of fMRI for helping to clarify the mechanisms that make particular instructional techniques effective.
More recent studies have begun to examine the effects of MBI on students’ neural representations (Brewe et al., 2018). In one study, undergraduates exposed to an MBI-based physics curriculum subsequently showed increased BOLD activity in the posterior cingulate, left dorsolateral prefrontal cortex (PFC), angular gyrus, and frontal poles when answering physics problems. Because these areas have been linked to working memory and higher-level reasoning, the authors suggested that MBI might support students’ use of mental simulation and prediction generation. Moreover, students used different neural networks during sequential phases of reasoning, drawing initially on brain regions associated with higher-level working memory and proceeding to regions linked to visual information processing and memory retrieval (Bartley et al., 2019). Notably, this study involved pre- and postsemester MRI scans, as opposed to evaluating the effects of MBI relative to other forms of instruction. Nonetheless, the findings present the possibility that MBI may promote increased activity in prefrontal neural regions during subsequent model-based reasoning.
Potential Neural Mechanisms of Model-Based Instruction
Studies consistently have implicated lateral prefrontal areas in causal reasoning and inference (Fugelsang and Dunbar, 2005; Mason and Just, 2004). A meta-analysis of several reasoning and problem-solving studies indicated that these tasks generally activate a network encompassing the dorsolateral PFC, anterior cingulate and anterior insular regions, and posterior parietal regions (Bartley et al., 2018). In a study of undergraduate students’ brain activity in response to conceptual reasoning in physics, students showed activity bilaterally in the dorsolateral and lateral orbitofrontal PFC, although several other regions, including the posterior parietal, retrosplenial, posterior cingulate, and lateral occipito-temporal regions, also were implicated (Bartley et al., 2019). Complex analogical reasoning tasks that involve the integration of multiple sources of information elicit activity in the most rostro-lateral regions of the PFC (Green et al., 2006; Krawczyk et al., 2011; Watson and Chatterjee, 2012). While fewer studies have examined the neural bases of hypothesis generation, experts in biological hypothesis generation show greater functional connectivity than novices across the middle frontal, superior and middle temporal, middle occipital, parahippocampal, and lingual cortex regions (Lee, 2012). Moreover, undergraduate students showed increased activity in the left inferior and superior frontal gyri after training in hypothesis generation relative to a control group (Kwon et al., 2009). Given behavioral evidence that MBI can facilitate causal reasoning and hypothesis generation, we hypothesized that exposure to a modeling-based instructional intervention would elicit greater activity in lateral prefrontal regions that consistently have been associated with these cognitive processes relative to a traditional, reading-based intervention.
Against this background, we examined the behavioral and neural impacts of simulating a computational model versus reading about the dynamics of a biological system. We asked two behavioral research questions: 1) Do students who read about a biological system perform differently than students who simulate the system on a short-term system-specific recall and reasoning test? 2) Do students who read perform differently than students who simulate on a test requiring them to generalize understanding of dynamic system properties to an abstract system? We also asked one primary neural research question: Are there differences in the neural responses of students who read vs. those who simulate when subsequently performing system-specific and system-general tests?
Given the short-term, accuracy-based nature of our behavioral assessments, we expected few behavioral differences between the two instructional groups, further exacerbated by the small sample sizes inherent to MRI studies. Based on previous behavioral findings (King et al., 2019a), however, we expected there would be differences in cognitive processing during the lesson that would manifest as neural response differences during subsequent biological reasoning. Given the high demands that modeling places on hypothesis generation and causal reasoning, we expected that model simulation would lead to differences in the use of lateral prefrontal neural regions linked to these cognitive processes. We also expected the Simulate group to show greater transfer of reasoning from the specific genetic system they studied to more abstract, generalized modeling concepts. At the neural level, we hypothesized that this increased transfer would manifest as increased activity in the rostro-lateral PFC in this group, given the reliance of transfer upon analogical reasoning.
METHOD
Participants
Participants included 30 undergraduate students enrolled in an introductory life sciences course (LIFE 120) at the University of Nebraska–Lincoln in the United States. Students were recruited through class announcements. All activities took place between weeks 4 and 10 of the semester, after students had been introduced to the computational modeling platform but before they had covered the specific instructional content used in the study. Students were carefully screened to ensure that they did not have a learning disability, attention-deficit/hyperactivity disorder, experience of concussion or another neurological diagnosis; that they were right-handed; and that they had no conditions that contraindicated MRI. In terms of describing our sample, 60% of those recruited were first years, 33% were sophomores, and 3% were seniors. Thirteen percent were first-generation students. The majority of students (87%) were white, two (7%) were African American, one (3%) was Asian, and one (3%) was Hispanic. All students were native English speakers, and 47% were male. Reported ACT scores ranged from 19 to 34 (M = 27). Students were randomly assigned to the model simulation (Simulation) or control (Read) conditions, as described later. Only two students (one per condition) indicated they had previously taken biology or anatomy courses. As shown in Table 1, groups did not differ significantly in their demographic characteristics.
Group | |||
---|---|---|---|
Read (n = 15) | Simulate (n = 15) | p | |
N (%) male gender | 8 (57) | 6 (43) | 0.25 |
N (%) white, non-Hispanic | 12 (80) | 14 (98) | 0.36 |
N (%) first year statusa | 8 (53) | 10 (67) | 0.45 |
M (SD) ACT scoreb | 26.6 (3.63) | 27.3 (3.79) | 0.61 |
Procedure
Procedures were approved by a university institutional review committee (IRB 20170917322 EP), and all participants provided written, informed consent to participate. Students were paid $40 upon completion of the study. Students attended a 2-hour appointment at the university’s imaging center, where one of the authors (C.A.C.C.) explained lesson activities to students and provided them with necessary materials (see Supplemental Material for these activities). Students then completed the lesson module independently in a quiet room. Students were allowed approximately 75 minutes to work on lesson activities. The lesson used in this research study is nearly identical to the published version (Crowther et al., 2018) minus the student-constructed model portion. The two instructional conditions were as follows:
Read condition: Students in the Read group were provided with an introductory reading about prokaryote gene regulation, specifically the lac operon. The reading outlined the learning objectives for the module and detailed key concepts of gene expression (e.g., the idea that some gene products are regulated in their abundance and timing). The reading then provided information about the components of the lac operon and the way that they interact to support lactose metabolism. Students were provided with a table in which they identified the positive and negative regulators (also called activation/inhibition mechanisms) within the system and explained their relationships. Thereafter, students read the answers to several example scenarios that applied understanding of the lac operon. For example, students were provided with a model and scenario in which only lactose was present, followed by a summary of how the presence of lactose would affect other components within the lac operon.
Simulate condition: Students in the Simulate group were provided with an introductory reading and positive/negative regulators table identical to those supplied to the Read group. However, rather than reading a written summary of the effects of manipulations to the lac operon system, they used the online Cell Collective platform (https://cellcollective.org; Helikar et al., 2012, 2015) to interact with and test the model. The Cell Collective software was designed to make computational modeling and simulations broadly accessible in life sciences research and education, regardless of the user’s prior modeling experience. The home page of the software allows students to select and access either the research or the education side of the platform. The education-focused area of Cell Collective provides access to scaffolded, interactive modeling and simulation activities focused on nearly 15 different topics (Bergan-Roller et al., 2017; King et al., 2019b). Students in this study used a computational model of the lac operon (Crowther et al., 2018) to make predictions, test scenarios, and respond to questions about model components and interactions. For example, Cell Collective allowed students to manipulate levels of lactose and glucose within the computational model and test the effects of mutations to specific genes. As they worked, students received diagrammatic feedback from the simulation regarding the effects of their manipulations on component activation, like lac operon transcription. Students wrote responses to questions regarding the effects.
Magnetic Resonance Imaging Protocol.
Following the completion of instructional activities, students underwent MRI in a 3 Tesla Siemens Skyra scanner (Siemens AG, Erlanger, Germany). Students were provided with ear protection, directed to recline on the scanner bed, and fitted with a 32-channel head coil. A mirror on the head coil allowed students to view task stimuli on a projection screen. First, a T1-weighted single-shot magnetization prepared rapid-acquisition gradient echo-pulse sequence was acquired (TR = 1 s, TE = 2.95 ms, voxel size = 1 mm3, flip angle = 9°, field of view [FOV] = 270, 176 sagittal slices). This was followed by two T2*-weighted echoplanar imaging runs (TR = 1 s, TE = 25 ms, 3 mm3 voxels, flip angle = 90°, FOV = 224 mm), during which students answered questions using a response pad.
Functional Magnetic Resonance Imaging Task.
There were two runs of scanning, the first comprising the system-specific task, which included questions about the lac operon, and the second comprising abstract, system-general questions about broader modeling concepts. In both tasks, students read and answered questions (trials) about model dynamics (hereafter referred to as model-based trials) and also completed control trials. For each model-based trial, students saw a diagram of and read a question related to the system-specific or system-general model over a period of 16 seconds (see Figure 1 for task description). During this time, it was not possible for students to make a response, but the question and response options were visible and outlined in a gray-colored box. The 16-second reading interval was based on pilot tests, which showed that students required a lengthy time period to read and process stimuli. Thereafter, the box turned green and students were allocated a maximum of 30 seconds to press a button on the right or left side of a response pad corresponding to their answer. For both the system-specific and system-general tasks, model-based trials were administered in the same order for all participants, although two versions of the tasks with varying orders for the control trials were created.
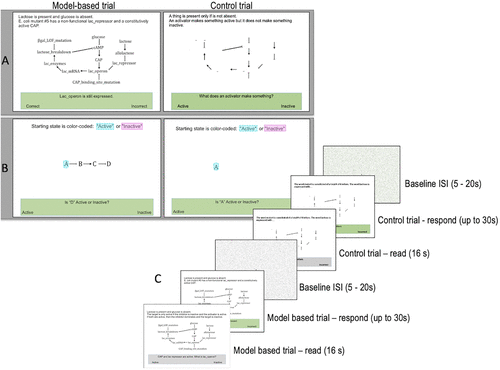
FIGURE 1. Description of the fMRI paradigm used in the study, showing (A) examples of system-specific model-based and control trials, (B) examples of system-general model-based and control trials, and (C) a general timeline for the trials. ISI: inter-stimulus interval.
System-Specific Task.
The eight system-specific model-based trials involved thinking about manipulations to the previously studied lac operon system (e.g., the effects of a mutation on a system component) and required a two-choice response (Active/Inactive or Correct/Incorrect; see Supplemental Material for a complete list of questions). Three of the trials were in a format asking students to either recall the relationship or perform simple direct reasoning between components, whereas five questions required more sophisticated reasoning about why a particular component would be active/inactive. Students also completed control trials that included questions with similar vocabulary as the model-based trials and a requirement to respond using the button box. However, the control trials did not include any biology- or model-based reasoning. Given the visual complexity of the model-based trials, we also input the model-based and control trial stimuli into MATLAB and randomized the pixels in each image to generate meaningless baseline stimuli that had color and luminance properties similar to those of the model-based trials. These baseline stimuli were presented between trials for jittered time intervals ranging from 5 to 20 seconds to enhance efficiency and mitigate trial anticipation effects (Poldrack et al., 2011).
System-General Task.
The second task focused on students’ ability to transfer their reasoning about system dynamics to a more abstract, general system of interacting components. Model-based trials were organized similarly to the system-specific trials, with increasing numbers of components, interactions, and “distance” between perturbation and effect. The structure of questions in the system-general task differed somewhat from the specific task, as the requirement was to transfer the reasoning about how interactions of excitatory and inhibitory relationships result in active/inactive components. Instead of using known components from the computational modeling lesson (e.g., lactose, CAP), letters of the alphabet (e.g., “A” and “B”) were substituted and arrow representations (e.g., inhibitory, activation) remained the same. Letters were highlighted with color to indicate whether they represented active or inactive components, and students were required to determine whether other letters within the model would be active or inactive based on the feedback dynamics depicted (see Figure 1 and Supplemental Material). Control trials also comprised highlighted letters but required students to simply answer whether the component the letter represented was active or inactive, based on the letter’s color. Baseline trials were created in the same way as for the system-specific trials. For each student, depending on response times, between 443 and 497 volumes of data were acquired for the system-specific task and between 496 and 517 volumes were acquired for the system-general task after removing the first five volumes of each task to adjust for steady-state magnetization.
Statistical Analyses
Group differences (Read vs. Simulate) in behavioral accuracy, measured as correct (1) and incorrect (0) trials, were analyzed for each task using a generalized linear regression model with a logit link function and binomial error distribution. A Wald test was used to determine whether students selected a correct response at levels greater than chance. One student in the Read group achieved accuracy scores of 0 for all model-based trials in the system-specific task and was therefore excluded from both behavioral and fMRI analyses for this task. We did not evaluate differences in response time, given that all students were prevented from making a response for 16 seconds until cued. A statistical threshold of p = 0.05 was used for behavioral analyses.
MRI data were analyzed separately for each fMRI task using the FMRIB software library v. 6 (Jenkinson et al., 2012). Preprocessing involved skull stripping the T1 images using the Brain Extraction Tool (Smith, 2002), realignment, boundary-based registration to the structural T1 image (Jenkinson and Smith, 2001), high-pass filtering at 60 s, linear registration with 12 df to the Montreal Neuroimaging Institute (MNI) 2-mm template, and smoothing to a 5-mm Gaussian kernel.
The statistical analysis of task-related fMRI data typically entails a “mass univariate approach,” to the general linear model (GLM), where, for each small, three-dimensional segment (called a “voxel”) in each volume of the brain, the temporally organized BOLD signal measurements are regressed on timing parameters for each trial of the task, that is, the trial onset and duration. To provide a more authentic characterization of the hemodynamic response to sensory stimuli, trial onsets are convolved with a prototypical inverted U-shaped function. In this study, we used a gamma function and its temporal derivatives. We modeled the first 16-second reading phase of each trial separately from the phase in which students were cued to make their response. Response phases were treated as nuisance regressors of no interest so that analyses concentrated on equivalent 16-second time periods for each trial. Estimates of the parameters used to align each fMRI volume relative to the middle volume of the run, known as motion regressors, also were included in the subject’s GLM design matrix to statistically correct for subject motion. In addition, we used FMRIB’s (Jenkinson et al., 2012) motion outliers function to identify time points with large motion artifacts (>0.5-mm framewise displacement) and remove their effects from the GLM design matrix.
For each participant, we derived regression parameters for brain activity during the reading phase of model-based trials relative to baseline (model > baseline). We also conducted a more stringent contrast of estimates for model-based trials versus control trials (model > control trials). Note that these parameters provide different information regarding neural effects. The first contrast provides a measure of change in brain activity during model-based trials relative to baseline brain activity. These estimates therefore incorporate all neural activity related to reading, visual processing, and anticipating a response. The model-based > control trial contrast provides an estimate of brain activity for the model-based trials over and above brain activity associated with reading and response anticipation processes that also were embedded in the control trials. This latter contrast therefore provides a purer estimate of neural activity associated specifically with processing biological models.
The resulting estimates for each participant were then passed to a second-level, group phase of analysis. Group analyses were carried out using FMRIB’s Local Analysis of Mixed Effects tool. In all of the group-level mixed models, participant self-reported gender and average accuracy for all model-based trials were included as mean-centered statistical covariates. The GLM therefore allowed us to identify spatial clusters where, on average, students’ BOLD responses differed significantly during model-based trials relative to 1) baseline or 2) control trials. The estimates for the Simulate and Read groups were compared using independent t tests. We also examined the relation of students’ average behavioral accuracy to their brain activity for each of the contrasts. We used a cluster-defining threshold of Z = 3.1 (p < 0.001) and a cluster-corrected significance threshold of p < 0.05 as the cutoff for significance. For illustrative purposes only, maximum parameter estimates shown in figures were extracted using FMRIB’s (Jenkinson et al., 2012) Featquery tool using cluster masks derived from the group analyses. The Talairach Client (Research Imaging Institute, 2009) was used to provide anatomical labels (within a range of 2 mm) associated with peak statistical coordinates.
RESULTS
Variation between Read and Simulate Groups
System-Specific Task.
Table 2 describes the behavioral accuracy of each group during the fMRI tasks. As shown, during the system-specific task, students correctly answered most control trials. For the model-based trials, students performed significantly better than chance (Z = 2.71 [df = 28], p < 0.001), and there was no significant group effect (Z = 0.57 [df = 28], p = 0.57) with students’ predicted probability of a correct response being similar across the groups (Read = 63% [confidence limit = 4.4%], Simulate = 66% [confidence limit = 4.5%]; Figure 2).
Group | |||
---|---|---|---|
Read M (SD) | Simulate M (SD) | p | |
System-specific model-based trials | 5.2a (1.47) | 5 (1.89) | 0.75 |
System-specific control trials | 6.53 (1.85) | 6.8 (1.26) | 0.49 |
System-general model-based trials | 4.36 (0.84) | 3.87 (1.30) | 0.24 |
System-general control trials | 8 (0) | 8 (0) | — b |

FIGURE 2. Predicted probability (±95% confidence interval, calculated as 1.96*SE) of correctly responding for Read and Simulate groups, distinguished by system-specific tasks related to the lac operon system and system-general task related to an abstract system (see Figure 1). Students performed significantly better than chance on the system-specific task and no different than chance on the system-general task.
For the fMRI system-specific task, the sample of students as a whole showed activation across widespread neural regions, including the cerebellum, middle and superior frontal gyri, and caudate nucleus for the model-based > baseline contrast (Table 3A and Supplemental Figure S1). For this contrast, the Simulate group showed greater BOLD activity than the Read group in the cuneus, as well as in the right postcentral gyrus, extending into the inferior parietal lobule (Table 3B and Figure 3). For the model-based > control trial contrast, the whole sample of students showed activation in the bilateral precuneus and lingual gyri, extending through parahippocampal regions (Table 3C and Supplemental Figure S2). However, there were no significant group differences for this more stringent model-based > control trial contrast (Table 3D). In summary, while there were no differences in behavioral accuracy between the groups, groups did show differences in neural activity relative to baseline when they processed system-specific model-based trials.
MNI coordinates | ||||||
---|---|---|---|---|---|---|
Contrast | Brain region | x | y | z | N voxels | Max Z |
Model based > baseline | ||||||
A. Whole sample | R. cerebellum | 6 | −74 | −20 | 20,669 | 7.51 |
L. middle frontal gyrus (BA 6) | −36 | 2 | 52 | 4981 | 6.68 | |
R. middle frontal gyrus (BA 9) | 48 | 32 | 34 | 1432 | 5.76 | |
L. medial frontal gyrus (BA 6) | −6 | 10 | 52 | 1258 | 6.41 | |
L. thalamus | −4 | −28 | −4 | 255 | 4.75 | |
R. cerebellum | 22 | −36 | −40 | 226 | 6.28 | |
L. caudate | −14 | 4 | 12 | 215 | 4.77 | |
L. cerebellum | −22 | −34 | −42 | 182 | 5.51 | |
R. claustrum | 32 | 24 | 4 | 181 | 6.54 | |
L. superior frontal gyrus (BA 10) | −28 | 52 | 16 | 110 | 4.12 | |
R. caudate | −22 | −26 | −4 | 104 | 4.09 | |
R. caudate | 34 | −32 | 2 | 83 | 4.07 | |
B. Simulate > Read group | ||||||
R. cuneus (BA 19) | 16 | −78 | 36 | 96 | 4.4 | |
R. postcentral gyrus (BA 2) | 62 | −22 | 38 | 81 | 3.97 | |
Model-based > control trials | ||||||
C. Whole sample | L. precuneus (BA 7) | −8 | −70 | 44 | 4025 | 5.8 |
L. lingual gyrus (BA 17) | −10 | −98 | 2 | 546 | 4.86 | |
R. cuneus (BA 18) | 18 | −100 | 14 | 541 | 4.66 | |
D. Simulate > Read group | n.s. |

FIGURE 3. Regions where students in the Simulate condition showed higher levels of activation than Read students for model-based trials in the system-specific task (model-based > baseline; Simulate > Read). Note that there were no group differences for the model-based > control trial contrast in this system-specific task. For illustrative purposes, bar graphs reflect the group average maximum parameter estimate (PE) in the cluster. Error bars reflect group standard deviations.
System-General Task.
During the system-general task, all students correctly answered all control trials. Students performed no better than chance for the model-based trials (Z = −0.37 [df = 28], p = 0.72), and there was no significant group effect (Z = 0.93, p = 0.35) with the predicted probability of a correct answer being similar across the two groups (Read = 48% [confidence limit = 4.6%], Simulate = 54% [confidence limit = 4.7%]; Figure 2).
During the system-general task, students showed activation across a number of neural regions for the model-based > baseline contrast, including in the medial and middle frontal gyri, posterior cingulate, and thalamus (Table 4A and Supplemental Figure S3). There were no group differences for this contrast (Table 4B). For the more stringent model-based > control contrast, both groups combined showed increased BOLD activity in middle and medial frontal regions, as well as in the insula and cerebellum (Table 4C and Supplemental Figure S4). The Simulate group showed a higher level of activity than the Read group in the right posterior insula, extending into the inferior parietal lobule, as well as in the left posterior cingulate gyrus (Table 4D and Figure 4). In summary, while there were no differences in the behavioral accuracy of the groups, groups did differ in the neural regions deployed when considering model-based relative to control trials.
MNI coordinates | ||||||
---|---|---|---|---|---|---|
Contrast | Brain region | x | y | z | N voxels | Max Z |
Model based > baseline | ||||||
A. Whole sample | R. cerebellum | 8 | −82 | −20 | 37,387 | 7.83 |
L. medial frontal gyrus (BA 6) | −4 | 18 | 48 | 8625 | 6.92 | |
R. middle frontal gyrus (BA 6) | −24 | 4 | 54 | 5093 | 6.25 | |
L. superior frontal gyrus (BA 10) | −30 | 58 | 8 | 1533 | 5.3 | |
R. claustrum | 32 | 22 | 0 | 421 | 7.02 | |
L. claustrum | −28 | 24 | 2 | 408 | 6.61 | |
L. medial frontal gyrus (BA 10) | −20 | 54 | −14 | 242 | 5.45 | |
L. posterior cingulate (BA 23) | −4 | −30 | 28 | 149 | 5.11 | |
L. thalamus | −18 | −30 | 0 | 91 | 4.18 | |
B. Simulate > Read | n.s. | |||||
Model based > control trials | ||||||
C. Whole sample | R. middle occipital gyrus (BA 18) | 32 | −84 | 10 | 25,117 | 6.93 |
L. middle frontal gyrus (BA 6) | −26 | 10 | 60 | 4034 | 6.16 | |
R. middle frontal gyrus (BA 6) | 28 | 16 | 62 | 3138 | 6.06 | |
L. medial frontal gyrus (BA 6) | 0 | 18 | 48 | 656 | 5.8 | |
L. superior frontal gyrus (BA 10) | 26 | 58 | 6 | 403 | 4.58 | |
L. cerebellum | −38 | −38 | −36 | 199 | 4.73 | |
L claustrum | −28 | 24 | 2 | 122 | 5.94 | |
R. insula (BA 13) | 34 | 24 | −2 | 108 | 5.55 | |
D. Simulate > Read | R. insula (BA 13) | 44 | −32 | 26 | 85 | 4.23 |
L. cingulate gyrus (BA 31) | −14 | −24 | 40 | 83 | 4.05 |
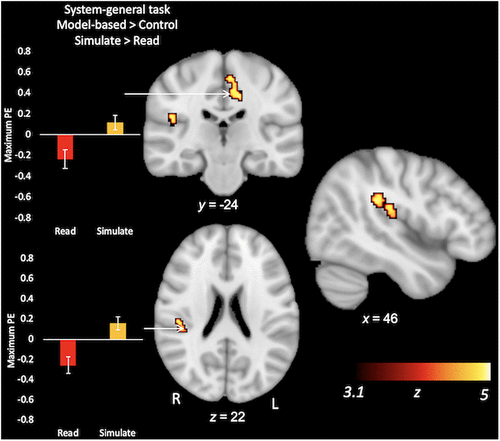
FIGURE 4. Regions where students in the Simulate condition showed higher levels of activation for model-based > control trials in the system-general task (model > control; Simulate > Read). Note that there were no group differences for the model-based > baseline contrast in this system-general task. For illustrative purposes, bar graphs reflect the group average maximum parameter estimate (PE) in the cluster. Error bars reflect group standard deviations.
Variation in Individual Students’ Behavior and Neural Patterns
Students’ mean behavioral accuracy for the model-based trials of the fMRI tasks was included as a covariate in the GLM analyses for each task, allowing us to evaluate the association of accuracy with brain activity. Independent of instructional group, this regressor was associated with students’ BOLD response patterns during the system-specific task. Specifically, for the model-based > control trials, higher mean accuracy was related to increased activity in bilateral middle frontal regions (Figure 5 and Supplemental Table S1). In contrast, behavioral accuracy for the system-general task did not relate significantly to brain activity during performance of that task.

FIGURE 5. Correlation between students’ accuracy and their BOLD activity for model-based > control trials in the system-specific task. For illustrative purposes, scatter-plot values reflect maximum parameter estimates (PE) for the model-based > control contrast extracted for each participant from this cluster and plotted against students’ percent accuracy for model-based task trials.
DISCUSSION
Teaching university biology steeped in system dynamics requires knowledge of how students develop their abilities to conceptually relate the components of biological systems and the dynamics of the system, processes that likely draw on hypothesis generation and causal reasoning skills that have been linked to lateral prefrontal brain regions (Nenciovici et al., 2018). This study makes a unique contribution to knowledge on educational neuroscience and life sciences instruction by showing that a short, modeling-based instructional intervention produced differences in functional brain activity, even when behavioral measures of learning were similar between the instructional groups. Group differences in neural activity were evident when students were evaluating the specific system they had learned about, as well as in a task that involved transferring this learning to general biological system dynamics. These differences, however, were not in the hypothesized lateral prefrontal regions. Instead, students’ behavioral accuracy during the system-specific task correlated with brain activity in bilateral middle frontal regions independent of mode of instruction, highlighting a need for research to understand this interstudent variation and how it can be leveraged to support effective teaching.
Behavioral and Neural Differences between Read and Simulate Groups during the System-Specific Task
The computational modeling lesson was designed to support student exploration of the lac operon system and evaluation of the likelihood of specific events as a result of manipulations of that system. The system-specific fMRI task challenged students to decide between plausible and implausible causal explanations of environmental conditions and perturbations to that same system. Students completing the computational modeling lesson invested differently from the Read group in understanding the system dynamics, as they systematically manipulated the lac operon model and sought explanations for the effects of these manipulations during the simulation lesson. Based on their more active exploration of model dynamics, we expected students in the Simulate group to show greater hypothesis generation and causal reasoning about why a perturbation resulted in the observed phenomena that would manifest as increased activity in lateral prefrontal regions. Students on the whole did show robust patterns of task-related lateral prefrontal activity, in line with previous studies relating these regions to complex reasoning (Fugelsang and Dunbar, 2005). They also showed activity, specifically during the model-based trials, in the superior parietal and posterior cingulate regions, areas where students showed increased activity after an intensive modeling-based physics course (Brewe et al., 2018). Contrary to our hypotheses, however, there were no group differences in the activation of prefrontal regions. Instead, the Simulate group showed higher levels of activity in the cuneus, as well as in the postcentral gyrus, extending into the inferior parietal lobule.
The MBI literature has highlighted the connection between physical and mental models, suggesting that the physical practice of modeling or interacting with external models encourages students to build and revise their internal, mental models of phenomena (Clement, 2000). Over the course of a semester, for instance, Brewe et al. (2018) determined that an MBI curriculum encouraged the use of different mental models by physics students when answering system-specific questions. Although the students in our Simulate group did not show the hypothesized pattern of greater activity in lateral prefrontal regions, they may have been drawing on different mental models from the Read group to reason through their responses, reflected in their different neural response patterns. Both Bartley et al. (2019) and Lee (2012) found that students used an array of posterior brain regions, including posterior parietal, lingual, and parahippocampal areas, when reasoning about physical and biological systems, suggesting that educational strategies designed to support these reasoning processes may affect neural networks extending beyond lateral prefrontal areas. Given the role of Brodmann area 2 in somatosensory processing (Grefkes et al., 2001; also see Supplemental Material, which includes a meta-analytic functional decoding analysis of our fMRI results), it is possible that students in the Simulate group were re-instantiating the more interactive sensory process of manipulating models when re-exposed to those models in the scanner. That is, their experience of modeling may have afforded different access points to those memories, which they drew upon during recall. It is also possible that, during recall, students in the Simulate group were studying the model to a greater degree than the control group to determine how system components were interacting. While our data indicate that the nature of processing differed for students exposed to MBI, it is important to note that we cannot draw conclusive inferences regarding specific cognitive processes or mental states based on correlational fMRI data (Poldrack, 2011). It is also important to acknowledge that group differences were confined to model-based trials and did not emerge when the general reading and response demands of the trials were controlled for (i.e., for the model-based > control trial contrast), raising the possibility that group effects were not specific to biological reasoning, but instead reflected differences in the deployment of more general processes.
Behavioral and Neural Differences between Read and Simulate Groups during the System-General Task
One goal for science instructors who teach biological systems is for students to recognize both the unity and diversity of these systems. That is, these systems have general principles that dictate how components interact to produce observable patterns, even while these principles are maintained differently in different systems and apply differently at hierarchical levels of molecules, cells, organisms, and communities (Wilensky and Resnick, 1999; Goldstone and Wilensky, 2008). In modeling parlance, that would mean students could proficiently change from diverse system-specific models to system-general models (Brewe and Sawtelle, 2018). Our system-general task was developed to determine students’ ability to transfer system-specific reasoning to more abstract contexts and incorporated similar inhibitory and excitatory feedback loops while increasing the number of components and interactions. Despite efforts to make the tasks similar, participants evidently viewed the system-general task as different from the system-specific task, as accuracy levels for the system-general task were low. It may be the case that students drew on more familiar, automatic representations for the system-specific task, with meta-analytic decoding hinting that patterns of activity during the task may reflect the use of episodic memory processes (see Supplemental Material). In contrast, the system-general task may have demanded greater use of neural networks associated with effortful, higher-level working memory and reasoning processes (Niendam et al., 2012; Bartley et al., 2018; Schwettmann et al., 2019), especially if the students did not infer the link between the principles in the task and those of the biological system they had studied. This evidence for limited transfer across the tasks replicates classic studies in cognitive psychology describing students’ failure to transfer problem-solving strategies to tasks with different surface features (Novick and Holyoak, 1991; Green et al., 2012) and underscores a need for educational strategies that scaffold the abstraction of system dynamics to novel contexts and scenarios.
There were no behavioral differences between the instruction groups during the system-general task, although there were qualitative performance differences between groups on specific trials. While the sample size limited our capacity to analyze per-question differences with sufficient statistical power, a descriptive analysis of the data indicated that both groups performed poorly on questions incorporating negative or positive feedback loops (less than 30% correct in each group), with Read performing better on a positive feedback question and Simulate performing better on the negative feedback question. Students in both groups were able to perform well (Read = 64% correct, Simulate = 95% correct) when ancillary positive and negative feedback loops were included as distractors, highlighting the participants’ ability to focus on the necessary component interactions.
We hypothesized that the Read and Simulate groups would differ in their recruitment of rostral prefrontal regions linked to analogical reasoning during the system-general task. This hypothesis was not supported. However, the Simulate group did show higher activity in the posterior insula extending into supramarginal cortex, as well as in the posterior cingulate. Again, meta-analytic decoding suggested that group differences corresponded with regions involved in somatosensory and motor processing, perhaps indicating variation in the sensory representations that groups were drawing on to perform the task. That is, the experience of actively modeling the biological system may have amplified encoding of sensory information in the Simulate group, which they could subsequently use to support the analysis of the interacting components within the models.
Individual Differences in Neural Activity Contribute to Variation in Behavioral Performance
While instructional group differences were evident at the neural level, it is important to call attention to the variability in behavioral performance within the instructional groups. Although the practice of simulating biological models did not lead to hypothesized differences in students’ recruitment of lateral prefrontal brain regions, there was a correlation between students’ mean behavioral accuracy for the system-specific model-based trials and their level of BOLD activity in middle frontal brain regions. These findings are consistent with previous studies linking lateral prefrontal activity to more advanced or expert scientific reasoning (Brault Foisy et al., 2015; Mason and Just, 2015; Nenciovici et al., 2019). Regardless of instructional method, then, it seems that some students naturally draw on prefrontal networks specifically when evaluating biological models and that the use of these networks corresponds with better task performance. The frontal response patterns of these students provide a neural benchmark for instructional interventions to promote more effective biological reasoning. They also highlight a need for ongoing research to understand the potentially malleable cognitive characteristics, including error checking or motivation, that differentiate these students from their peers.
Limitations and Future Directions
Several limitations of our study should be noted. First, given the complexity of the stimuli and the time required by students to answer questions, we used an unusual fMRI paradigm with relatively few trials. It is possible that low trial numbers, coupled with a small number of participants in each group, limited our capacity to detect group differences. Relatedly, the control trials for the system-specific task were complex, as illustrated by the fact that students were not always accurate in their responses to these trials. This may have obscured our capacity to capture differences in neural activity between the model-based and control trials. We did not find group differences in behavioral accuracy, but it is important to note that students were confined to making binary responses to simplify the response demands in the MRI scanner. Thus, our measures of behavior were coarse and likely not sensitive to behavioral changes that may have been evident had students been able to express their reasoning verbally. We elected to use a reading exercise as the control condition in the study, as we felt that this control reflected the common practice of presenting complete visual models in textbooks or lectures. In the future, it would be interesting to extend comparisons to other modes of instruction, such as video or auditory lectures. It is also clear that there are individual differences that may drive neural effects, and careful analysis of students’ levels of engagement with and performance during the lesson activities would be useful in specifying the learning conditions that promote changes in neural activity. Notably, participants in this study had already completed two computational modeling activities in their biology labs earlier in the semester and therefore likely had overcome some of the challenges associated with orienting themselves to the software and interpreting data outputs. Prior exposure to MBI may also have obscured some of the effects of our brief instructional manipulation, as some students in the Read group may already have been drawing on these modeling activities to support their reasoning.
More generally, there often exists a gap between cognitive neuroscience studies, which rarely map onto the messiness of postsecondary classrooms, and postsecondary instructional methods, which rarely connect to the neurocognitive underpinnings of how people learn. This study sought to bridge that gap by blending the rigor of neurocognitive methods with the authenticity of cognitive psychology–informed postsecondary instruction to examine mechanisms of learning in university biology. Although we believe there is applied knowledge to be gained from such an approach, there are continued challenges in blending neuroscientific research with authentic educational practice (Masson et al., 2012). Although fMRI currently has the best spatial resolution for determining human brain processes in vivo, it is an artificial environment devoid of the usual peer interactions of the classroom and with different motivational features relative to the classroom. The dynamic process of modeling is also difficult to capture within an artificial MRI scanning environment that allows for limited motion, and our study instead concentrated on students’ retrieval of information, as opposed to the learning process itself. Longitudinal studies that track the effects of MBI dosage on student’s longer-term behavioral and neural response patterns are a key direction for future research. Optimally, these studies would incorporate detailed think-aloud paradigms to gain greater insight into student’s actual reasoning processes and how these align with individual differences in neural response patterns.
CONCLUSIONS
Student knowledge and understanding of complex system dynamics are central to undergraduate biology education, yet the complex hypothesis generation, error detection, and causal reasoning processes involved in such systems thinking are not easily assessed through traditional behavioral measures. University science students are developing their abilities to use models more like experts (Hester et al., 2018) through repeated modeling activities that build neural networks associated with these experiences. Instructional considerations that may further advance students toward “modeling like an expert” include providing more support to analogize between scenarios (Green et al., 2012; Mareschal, 2016) and encouraging hypothesis generation (Kwon et al., 2009) and model construction in multiple modalities. In this study, students who briefly engaged in a modeling simulation showed higher levels of activity than those who simply read about the system across parietal and occipital brain regions. These neural differences were present despite similar behavioral performance across the groups, indicating that model simulation exercises may alter the types of strategies students employ when reasoning about biological systems even in the absence of obvious behavioral change. Knowledge that such neural change is occurring even in the absence of behavioral evidence of learning is a helpful consideration for instructors. Much more research is needed to determine how these neural response patterns align with specific cognitive strategies and subsequent student achievement. Nonetheless, this study takes an important first step in addressing calls for research on the neural effects of instructional methods (Hayes and Kraemer, 2017; Owens and Tanner, 2017), which ultimately could inform understanding of which interventions are most effective for eliciting change in biology students’ neural representations.
ACKNOWLEDGMENTS
We would like to thank Lisa Briona, Gretchen King, and Ryan Hudnall, who contributed to the development of instructional activities and data collection. We would also like to thank the students who generously volunteered their time to participate in the research. The research was partially funded through DUE 1432001 and University of Nebraska–Lincoln Core Research.