Putting the Pieces Together: Student Thinking about Transformations of Energy and Matter
Abstract
Research on student thinking facilitates the design of instructional materials that build on student ideas. The pieces framework views student knowledge as consisting of independent pieces that students assemble in fluctuating ways based on the context at hand. This perspective affords important insights about the reasons students think the way they do. We used the pieces framework to investigate student thinking about the concept transformations of energy and matter with a specific focus on metabolism. We conducted think-aloud interviews with undergraduate introductory biology and biochemistry students as they solved a metabolism problem set. Through knowledge analysis, we identified two categories of knowledge elements cued during metabolism problem solving: 1) those about the visual representation of negative feedback inhibition; and 2) those pertaining to student focus on different metabolic compounds in a pathway. Through resource graph analysis, we found that participants tend to use knowledge elements independently and in a fluctuating way. Participants generally showed low representational competence. We recommend further research using the pieces perspective, including research on improving representational competence. We suggest that metabolism instructors teach metabolism as a concept, not a collection of example pathways, and explicitly instruct students about the meaning of visual representations associated with metabolism.
INTRODUCTION
Designing high-quality instructional materials and pedagogical approaches requires research on student thinking about life sciences concepts and how students interact with instruction to build their knowledge. We define student thinking as the knowledge students bring to the learning context, which includes a mixture of intuitions, experientially grounded notions, and scientific ideas (diSessa and Sherin, 1998; National Research Council, 2001; Clark and Linn, 2013). Research characterizing student thinking reveals the challenging process of knowledge refinement students undertake when learning new concepts. For example, research on student thinking about natural selection revealed that students tend to use the word “adapt” in an everyday way rather than a scientific way and that students apply more scientific ideas when solving problems about familiar organisms than unfamiliar organisms (Nehm and Schonfeld, 2008; Nehm and Ha, 2011). Research on student thinking about noncovalent interactions revealed that students approach the topic using definitions and heuristics and must be guided to use causal mechanistic reasoning (Cooper et al., 2015; Halmo et al., 2018). This type of research has improved instruction while also raising new research questions about learning (e.g., Williams et al., 2015; Halmo et al., 2020). Biochemistry offers a particularly useful context for student-thinking research, as it requires students to integrate knowledge from biology and chemistry. The work presented here addresses the critical need to investigate student thinking by examining the ideas students use to solve problems about a core concept in biochemistry.
We investigated student thinking in the context of biochemistry because of the crucial role biochemistry plays in life sciences education. Many undergraduate science students take biochemistry because of its importance to their career paths. Most pre–health professions students must take biochemistry as part of the admission requirements for professional school. Opportunities in engineering are abundant for students who are prepared to consider how the chemical principles of life can be leveraged to solve real-world problems. Biochemistry also sits at the junction of multiple areas of research that impact human health and the environment, making it a desirable course for students who plan to go to graduate school. Students take introductory biology and general chemistry as early as high school, yet they typically do not consider the chemical foundations of biology phenomena or the biological applications of chemistry concepts until their first biochemistry course. Biochemistry courses challenge students to think about molecules and systems of molecules by applying basic chemistry knowledge.
Transformation of energy and matter is the core concept that explains how biological systems grow and change through pathways of chemical transformation (American Association for the Advancement of Science, 2011; Brownell et al., 2014). This concept pertains to biochemistry due to the discipline’s central focus on metabolic pathway dynamics and regulation (Loertscher et al., 2014; American Society for Biochemistry and Molecular Biology, 2022), hereafter referred to as “metabolism.” Metabolism deals with the linkage of chemical reactions in pathways and how pathways interconnect and allow an organism to function (Loertscher et al., 2014). As students build their knowledge of metabolism, they improve their ability to make predictions about the dynamics of a pathway, including how a pathway will respond to fluctuations caused by changes in the cellular environment. Biochemistry students must integrate many ideas to solve problems about metabolism (Loertscher et al., 2014), including ideas about free energy and directionality of chemical reactions, enzymes and catalysis, and enzyme regulation. Students develop these ideas in general chemistry and introductory biology courses, sometimes as early as high school and continuing into introductory college classes. This study examines student thinking about metabolism among undergraduate life science students.
Theoretical Perspective: Student Thinking as Knowledge in Pieces
Investigating student thinking requires adopting a perspective on the characteristics of student knowledge. There has been a long debate in the literature about the extent to which students’ knowledge is coherent and theory-like versus fragmented and context dependent (Elby, 2000; Sherin et al., 2012; Clark and Linn, 2013; Maskiewicz and Lineback, 2013; Leonard et al., 2014; Lira and Gardner, 2020). Indeed, evidence exists for both views (Scherr, 2007; Sherin et al., 2012). On the one hand, evidence shows that students display coherent, context-independent, stable ideas that are resistant to instruction (e.g., Vosniadou and Brewer, 1992; Vosniadou et al., 2008; Sherin et al., 2012; Coley and Tanner, 2015). This body of work is referred to by multiple names (e.g., framework theory, coherence perspective; Scherr, 2007; Clark and Linn, 2013; Leonard et al., 2014; Gouvea and Simon, 2018), but we will call it the “misconceptions perspective” (Scherr, 2007). On the other hand, evidence shows that students’ ideas are more like independent elements assembled in the moment based on context (e.g., diSessa, 1988, 2017, 2018; diSessa and Sherin, 1998; Smith, 1992; Hammer, 1996; Hammer and Elby, 2003; Wagner, 2006, 2010; Nehm et al., 2012; Sherin et al., 2012; Gouvea and Simon, 2018). This body of work has been referred to by a variety of names, each with a different emphasis (e.g., phenomenological primitives, facets of knowledge, resources, conceptual dynamics; Minstrell, 1992; diSessa, 1993; Hammer, 2000; Southerland et al., 2001), but we will refer to it as the “pieces perspective” (Scherr, 2007).
The misconceptions and pieces perspectives share some ideas, yet they have different research agendas (Scherr, 2007; Clark and Linn, 2013). Both perspectives describe students’ knowledge as constantly developing and evolving (Clark and Linn, 2013). Research from the misconceptions perspective aims to identify conceptual frameworks, searching for the nonscientific models students use coherently and rigidly across contexts (Scherr, 2007; Clark and Linn, 2013; Lira and Gardner, 2020). In contrast, research from the pieces perspective aims to identify individual knowledge elements, the fine-grained ideas that contribute to understanding a visual representation, scientific phenomenon, or concept (Elby, 2000; Hammer and Elby, 2002). Pieces researchers search for these independent knowledge elements accessed during student reasoning and pinpoint how they interact within and across contexts. Pieces researchers assume that students have reasons for thinking the way they do and that an idea they express that may seem incorrect is intuitive or useful in another situation (Scherr, 2007).
The misconceptions and pieces perspectives also differ in their instructional agendas. The misconceptions model argues that students must dismantle and discard nonscientific ideas by becoming aware of the inadequacy of those ideas, discovering more robust ideas, and resolving the contradictions (Smith et al., 1994; Scherr, 2007; Nehm and Reilly, 2007; Kalinowski et al., 2010). This process is difficult but leads to permanent change. The pieces model promotes the notion of instruction as helping students refine their intuitions (Elby, 2001), which are not considered to be incorrect but inappropriately applied in a given context. Thus, instruction is meant to help students recognize the utility of their intuitions in some circumstances and the need to refine them for a given situation. Adaptation of ideas can come relatively easily according to the pieces model, but it may be unstable. That is, students may refine their intuitions and then later forget the importance of the refinement (Scherr, 2007; Robertson et al., 2015).
We approached this investigation from the pieces perspective, because we hypothesized that students’ ideas about metabolism are more independent, loosely connected, and context dependent than they are theory-like. We thought this because metabolism draws upon many ideas that are not typically linked together until late in the life sciences curriculum. Some ideas are taught more in general chemistry (e.g., free energy and the conditions of reversibility for a chemical reaction), while others are taught more in introductory biology (e.g., feedback inhibition). In fact, many students may never receive explicit instruction, even in a biochemistry course, that prompts them to recognize, integrate, and apply pieces from biology and chemistry to metabolism. Many biochemistry instructors possess tacit schemas about metabolism that they assume students also have (Meyer and Land, 2003; Loertscher et al., 2014). Only recently have biochemistry educators acknowledged this limitation and started to tease apart the many concepts students could and should coordinate when solving problems about metabolism (Loertscher et al., 2014; Villafañe et al., 2021). We also were compelled by the instructional stance that privileges student thinking as intuitive and rational, even if sometimes misplaced, and that focuses on deciphering the origin of students’ ideas and facilitating refinement (Robertson et al., 2015).
We further hypothesized that some of the most critical knowledge elements for metabolism problem solving would be the ones that contribute to representational competence, the ability to appropriately interpret and produce a set of disciplinary-accepted representations of real-world phenomena linked to formalized scientific concepts (Elby, 2000; Ainsworth 2006; Airey and Linder, 2009; Offerdahl et al., 2017). When solving metabolism problems, students must make inferences about symbols, including letters, arrows, and compound names to produce a dynamic mental construct of the phenomena being represented (Sherin et al., 2012; Lira and Gardner, 2020). They must then integrate this information with prior knowledge of chemical reactions and pathways brought to working memory from long-term memory (Sherin et al., 2012; Fiorella and Mayer, 2015). This process of interpretation may be more difficult for learners because of shortcomings in the design of visuals. Scientists often design visuals based on their intuition and extensive knowledge base, leading to unintended student interpretations (Tversky et al., 2000; Smallman and John, 2005; Novick and Catley, 2007). Indeed, biochemists lack agreed-upon conventions for visually representing metabolism Offerdahl et al., 2017), and representations of metabolism rely heavily on arrows to convey meaning, even though arrows are used inconsistently in biology (Wright et al., 2017). For these reasons, it is critical to uncover the knowledge elements students use when interpreting visual representations of metabolism.
Thus, we aimed to add to the body of literature on student thinking about core life sciences concepts (Nehm and Reilly, 2007; Hartley et al., 2011; Andrews et al., 2012; Wright et al., 2014; McFarland et al., 2016; Prevost et al., 2016; Halmo et al., 2018; Scott et al., 2019) by characterizing the knowledge elements students use during metabolism problem solving. Specifically, we investigated the following research questions: What knowledge elements do life sciences undergraduates use when thinking about metabolism? How do students assemble these elements during problem solving?
METHODS
We conducted a qualitative interview study using a think-aloud protocol in which participants answered a problem set that examined their understanding of metabolism. Participants answered each problem while thinking aloud, and we further probed their understanding for clarity. We analyzed participants’ responses using qualitative content analysis, and this led to the identification of two categories that describe the use of knowledge elements across the entire sample. We then created resource graphs (Wittmann, 2006; Rodriguez and Towns, 2019; Rodriguez et al., 2020) for three purposefully selected students to address how individual students assemble knowledge elements about metabolism to make predictions across three different problems.
Participants and Context
We recruited participants from an introductory biology course in Fall 2016 and introductory biochemistry courses in Fall 2018. All courses were taught at a large, public research university in the southeastern United States. One instructor in a single section taught all of the introductory biology participants. Multiple professors in multiple sections taught the introductory biochemistry participants. The introductory biology course serves as a foundational course for life sciences majors, and at the time of the interviews, general chemistry was a prerequisite. This course enrolls mostly first- and second-year students. We refer to participants from this course as “biology students.” The introductory biochemistry course focuses on the structure and function of biological molecules, enzyme kinetics, metabolism, and molecular biology and enrolls mostly second- and third-year students. We refer to participants from this course as “biochemistry students.”
Although this is a basic research study to discover the range and assembly of student knowledge elements for metabolism problem solving, we provide some information about the instructional approaches for the purpose of context. The introductory biology course from which participants were recruited focused on metabolism in terms of understanding the basics of reactants and products of each step in cellular respiration and photosynthesis. Students were taught to focus on the movements of ADP/ATP and the electron carriers. Biology students encountered many visual representations, in a wide variety of styles. Some visual representations gave an indication of how intermediates change in structure throughout the reaction, but even in those cases, students were directed in the related questions and discussion to focus on the incoming reactants and outgoing products instead. The course emphasized metabolism solely in the context of achieving specific ends to support life in organisms—most specifically energy processing.
The biochemistry participants received metabolism instruction focused on regulatory mechanisms of pathways, but they were generally covered in terms of “This molecule inhibits/activates this enzyme/process, so what happens when there is a lot of it or a little of it?” The specific ways regulation can occur were touched on but not the primary focus. The primary focus articulated for these students was understanding free energy and reaction favorability. Students were typically asked to work in depth with named and visually depicted pathways and enzymes in a more elaborate way than in the introductory biology course. Introductory biochemistry also used a diverse set of visual representations for metabolism, but the diversity was narrower than that in introductory biology.
Recruited participants had previously completed a 26-item problem set as part of their enrollment in either introductory biology or introductory biochemistry. This assessment measures student learning about noncovalent interactions in biomolecular structure and metabolism. The investigation reported here focuses on metabolism items, which we describe in Data Collection: Problem Set Development. Our investigation of the noncovalent-interaction items is reported elsewhere (Halmo et al., 2018, 2020). We recruited a total of 44 participants, including 22 participants from introductory biology and 22 participants from biochemistry. None of the biochemistry participants had previously completed the assessment during introductory biology. Of the 22 biology participants, 11 were male and 11 were female. Of the 22 biochemistry participants, 2 were male and 20 were female. Gender identities of the sample were obtained from the Office of Institutional Research. Students received a monetary incentive of $20 for their participation in this study.
This study was approved by the University of Georgia, Athens (UGA) Institutional Review Board under exempt status (STUDY00000660 and PROJECT000000090).
Data Collection
Problem Set Development.
We developed a metabolism problem set that was designed to uncover the knowledge elements students use when solving problems about metabolism. We aimed to address concepts that students learn in introductory biology and chemistry courses and build upon in biochemistry courses. After piloting three initial pathways with students and interviewing for construct validity purposes, we refined the problem set to the pathway used in this study that asks students about an unfamiliar pathway (Figure 1). Thus, students need to rely on their knowledge of metabolism and visual representations to make predictions about the pathway. In pilot interviews, when the pathways involved more familiar metabolic compounds, students used knowledge elements more related to the particular compounds (e.g., ATP or cholesterol) than to principles of metabolism. We piloted the problem set with students from both introductory biology and biochemistry and with PhD-level biologists, chemists, and biochemists. We also conducted follow-up interviews with students and PhD-level biologists, chemists, and biochemists to obtain detailed feedback and recommendations for improvement of the problem set.

FIGURE 1. The metabolic pathway referenced in the problem set students were asked to solve. (A) Thiamine phosphate is converted over several steps to DMA-CoA by enzymes A, B, and C. If there is an excess of the intermediate compound IV-CoA, some of it can bind to enzyme B to prevent the enzyme from working via negative feedback inhibition. This slows the rate of IV-CoA production. (B) This variation of the pathway introduces IB-CoA, which can be converted to IV-CoA (and vice versa) by enzyme D.
The finalized problem set contains six multiple-choice, three multiple true-false, and three constructed-response items for a total of 12 items. The complete problem set is presented in Supplemental Figure S1. All 12 items refer to the metabolic pathway presented in Figure 1. The objective items reference the image in Figure 1A, and the constructed-response items reference the image in Figure 1B. The 12 items probe a variety of aspects of metabolism, including the meaning of visual representations, reaction reversibility, flux, and negative feedback inhibition.
This paper reports results from our analysis of participants’ responses to a subset of five items, including one multiple true-false, one multiple-choice, and three constructed-response items. These items reveal knowledge elements about the concept of negative feedback inhibition, a mechanism that governs the dynamics of pathway regulation. Based on pilot studies with experts and students, we represented negative feedback inhibition using a dashed arrow and circled bar (Figure 1). There is no consensus on how to represent negative feedback inhibition. Negative feedback inhibition occurs when an excess of product accumulates and inhibits an enzyme earlier in the pathway. In the case of our problem, when IV-CoA accumulates, it binds to enzyme B at a site other than the active site (i.e., an allosteric site), inhibits enzyme B, and decreases the amount of IV-CoA produced. The levels of all metabolic compounds in the pathway are affected by negative feedback inhibition at a single step.
Interview Protocol.
All participants had previously completed the problem set as part of their course enrollment. In the interview, we asked participants to complete the assessment again using a think-aloud interview protocol (Ericsson and Simon, 1980, 1998; Keys, 2000; Cooper et al., 2013). This protocol reveals cognitive processes that are not normally available in written answers alone. Twenty-seven of the interviews focused on problems related to both noncovalent interactions and metabolism. The remaining 17 interviews focused entirely on problems related to metabolism.
We modeled our protocol after established protocols in other studies (Keys, 2000; Cooper et al., 2013; Halmo et al., 2018). At the start of the interview, we asked participants to think aloud while solving the problems presented to them, including anything they were reading and looking at in the figures. We limited interruptions to statements encouraging participants to “Please keep thinking aloud.” At breaks in the interviewee’s thinking, we occasionally prompted with: “Can you explain what you mean by [scientific term]?” or “Can you further elaborate on [idea].” Students viewed the problem set via Qualtrics. For the multiple true-false and multiple-choice items, we asked students to click their response in Qualtrics, which provided us with a record of their selections in addition to their verbal responses. For the constructed-response items, student responses were verbal, not written.
We video-recorded all interviews and applied pseudonyms. Pseudonyms that begin with B refer to biology participants, while pseudonyms that begin with C refer to biochemistry participants. Pseudonyms are gender-neutral, because we did not examine gender as a factor in our analysis.
Data Analysis
Student Performance.
We determined student performance on the problem set as an indication of the extent to which students think about metabolism in a scientifically accurate way. We evaluated student performance on the multiple true-false and multiple-choice items by comparing the answers students clicked in Qualtrics with the key we generated in advance. We evaluated student performance on the constructed-response items by categorizing the ideas within their verbal explanations as purely scientific (e.g., aligning with scientific explanations), purely nonscientific (e.g., different from scientific explanations), or a mixture of both.
Qualitative Content Analysis.
We conducted qualitative content analysis (Strauss and Corbin, 1998) of participant interviews using a process that aimed to ensure the trustworthiness of our findings (e.g., Elo et al., 2014). We aimed to identify the full range of knowledge elements students brought to the problem-solving space. First, we developed an a priori codebook consisting of ideas and problem-solving approaches we expected participants to express based on prior work (e.g., see Data Collection: Problem Set Development). Second, author K.B. refined the codebook by listening to all interviews to determine how well the a priori codebook aligned with the ideas presented by participants. Third, K.B. listened to all interviews again and made additional refinements to the codebook as new insights emerged. Throughout steps 2 and 3, K.B. consulted with author P.P.L. This process led to a coding scheme of 40 total codes that were derived from participant language rather than solely based upon our preliminary ideas. Fourth, authors K.B. and A.S. applied the coding scheme to all interviews. They independently coded interviews and then compared their coding to resolve all discrepancies. Fifth, K.B. and A.S reviewed all coded interviews and narrowed the focus to 26 codes that deal with students’ interpretations of the dashed arrow and circled bar and their attention to metabolic compounds in the pathway. At this stage, K.B., A.S., and P.P.L. also reorganized several codes to better capture the variability within the original codes. This process led to the 12 codes presented in the tables in Results: Knowledge Elements Cued During Metabolism Problem Solving. The process of refining our codes is illustrated for two of the final 11 codes in Supplemental Figure S2.
Resource Graph Creation.
A resource graph is a representation of linked knowledge elements (Wittmann, 2006; Rodriguez and Towns, 2019; Rodriguez et al., 2020). Resource graphs reveal student reasoning, because they display the coordination of individual knowledge elements that are activated when students solve a problem. We purposefully selected three students from our sample and created resource graphs of their solutions. Authors K.B. and P.P.L. selected three students (of 44 total) who used five of the seven distinct ideas about the dashed arrow and circled bar and focused to different extents on various metabolic compounds. Author K.B. returned to these students interviews and documented each distinct statement they made. Generally speaking, K.B. defined a knowledge statement as a sentence. For example, K.B. counted the following sentence from participant Charlie as a knowledge statement: “Without enzyme C, there would be barely any production of DMA-CoA.” While documenting knowledge statements, K.B. also documented the connections between knowledge statements, which he determined because statements were sequential or because the student used linking words that explicitly connect different knowledge statements. Authors K.B. and P.P.L. then organized knowledge elements into the resource graphs presented in Results: Assembly of Knowledge Elements into Solutions.
RESULTS
Here, we present results that address our research questions: What knowledge elements do life sciences undergraduates use when thinking about metabolism? How do students assemble these elements during problem solving? We focus on research participants’ interview responses to five items. We first report participants’ scientific accuracy on these items. Accuracy is not the point of our research, yet we provide these data to orient readers to the way professional biologists, chemists, and biochemists think about the problem set and to present an overview of the extent to which students think in this way. The subsequent two sections focus on the use of knowledge elements during metabolism problem solving. Knowledge Elements Cued During Metabolism Problem Solving presents categories of knowledge elements derived from qualitative content analysis, and Assembly of Knowledge Elements into Solutions presents resource graphs from a subset of participants to address the assembly of knowledge elements. Taken together these data illustrate that our problem set cued multiple independent knowledge elements that most participants assembled in a contextually fluctuating way.
Performance on a Metabolism Assessment
Participants in our study answered one multiple true-false item, one multiple-choice item, and three constructed-response items. The multiple true-false item asked about the meaning of the dashed arrow and circled bar (Figure 1). Participants could select one to seven different responses, with “negative feedback or inhibition” as the correct response and all other responses as false (Table 1A). In multiple true-false items, each true-false item is independent, so it is possible to capture multiple ideas simultaneously held by students. Biology participants responded to this item with variability. The majority of biology participants (N = 15) selected negative feedback or inhibition, but even more biology participants selected IV-CoA being added as a reactant (N = 16) or IV-CoA being recycled (N = 18). Biology participants also selected other responses (alternate pathway, etc.). In contrast, all biochemistry participants recognized the dashed arrow and circled bar for its intended purpose (N = 22), even though a small subset simultaneously thought of the arrow in other ways (Table 1A). These data provide initial evidence that the dashed arrow and circled bar activated multiple knowledge elements for biology participants in our study and most strongly activated the negative feedback element for biochemistry participants. We will more fully explore participants’ knowledge elements about the dashed arrow and circled bar in the next section.
A. Participant responses to a multiple true-false prompt corresponding to Figure 1A: “What do the dashed arrow and circled bar represent?”b | ||
---|---|---|
Multiple true-false prompt | Biology participants (N = 22) | Biochemistry participants (N = 22) |
Negative feedback or inhibition | 15 | 22 |
Positive feedback or activation | 4 | 0 |
Removal of IV-CoA | 2 | 3 |
IV-CoA being added as a reactant | 16 | 3 |
IV-CoA being recycled | 18 | 6 |
Reverse reaction | 3 | 3 |
Alternate pathway | 11 | 3 |
B. Participant responses to a multiple-choice prompt corresponding to Figure 1A: “If enzyme C were inhibited, how might this eventually impact the activity of enzyme B?”c | ||
Multiple-choice prompt | Biology participants (N = 22) | Biochemistry participants (N = 22) |
The activity of enzyme B would not be affected | 11 | 4 |
The activity of enzyme B would increase | 2 | 6 |
The activity of enzyme B would decrease | 9 | 12 |
Second, we asked participants a multiple-choice question about the outcome of a perturbation in the visualized pathway. Specifically, we asked them what would happen to enzyme B activity if enzyme C were inhibited. The scientifically accurate view of this perturbation is that IV-CoA would build up due to decreased conversion to DMA-CoA by enzyme C and that the increased concentration of IV-CoA would in turn decrease the activity of enzyme B. This would occur due to negative feedback inhibition. Nine biology participants selected the scientifically accurate response, while 11 selected that the perturbation would have no effect on the activity of enzyme B. This result aligns somewhat with the diversity of biology participants’ knowledge elements about the dashed arrow and circled bar. Participants who thought, for example, that the symbol represented IV-CoA being recycled, might have reasoned that inhibiting enzyme C would either increase the activity of enzyme B or have no effect. Twelve biochemistry participants selected the scientifically accurate response (i.e., the activity of enzyme B would decrease). This number is many fewer than the number from Table 1A who said that the dashed arrow and circled bar represent negative feedback and inhibition. Apparently some biochemistry participants who recognized the dashed arrow and circled bar as negative feedback inhibition did not use this knowledge element for the multiple-choice question. These data point to the importance of uncovering the range of knowledge elements activated by the visual representations used for metabolism and of helping students develop representational competence.
We also characterized participant performance on three constructed-response items. Each item presented participants with a different context, a particular perturbation to the pathway. For each perturbation, we asked participants to make and explain their prediction about the impact on flux through the pathway. For example, participants were asked about the impact on flux if IV-CoA could no longer bind to enzyme B. We focused our performance analysis both on participants’ decisions that flux is or is not affected and whether they used purely scientific explanations, purely nonscientific explanations, or a mixture of both. We observed a similar pattern for biology and biochemistry participants (Figure 2 and Supplemental Figure S3). Most participants stated that flux would be affected by the perturbation, which is the intended response. A few participants used purely scientific explanations, and a few used purely nonscientific ones, but the typical explanations were a mixture of scientific and nonscientific ideas. The only noteworthy distinction between biology and biochemistry participants was that biochemistry participants’ explanations were somewhat more scientifically accurate overall. These data suggest that, while participants may have recognized that perturbations affect pathway flux, their reasoning included many knowledge elements, some of which needed refinement. The primary purpose of this paper is to report the range of knowledge elements participants use when solving metabolism problems and to examine how participants assemble these ideas in a problem solution. We present these data in the following two sections.
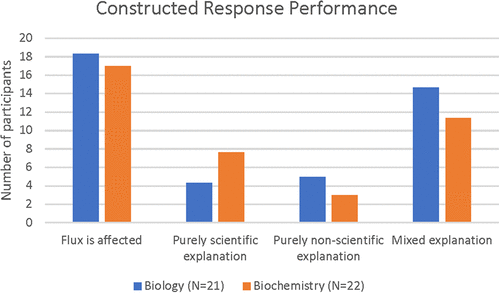
FIGURE 2. Participant performance across contexts in a metabolism problem set. Participants were asked to predict the outcomes of three perturbations to the metabolic pathway. They were asked to say whether or not overall pathway flux would be affected and scientifically explain their predictions. The leftmost set of bars shows the number of participants who predicted that flux would be affected by the perturbation. The remaining three sets of bars show the number of students who provided explanations that were purely scientific, purely nonscientific, or mixtures of both across all contexts. Blue bars correspond to biology participants (N = 21) and orange to biochemistry participants (N = 22). Item-level data are shown in Supplemental Figure S3.
Knowledge Elements Cued during Metabolism Problem Solving
Analyzing participant performance only tells a partial story. Identifying the variety of knowledge elements cued during problem solving explains participant performance and reveals future avenues for research and instruction. Our qualitative content analysis showed that participants’ solutions can be explained by two categories of knowledge elements: those pertaining to the dashed arrow and circled bar and those pertaining to the metabolic compounds students focus on. We describe these categories by providing examples of knowledge elements using quotes from the data. Quotes have been lightly edited for clarity and readability. We use gender-neutral pseudonyms for participants. Pseudonyms starting with the letter B indicate biology participants, and pseudonyms starting with the letter C indicate biochemistry participants. We begin with a look at knowledge elements activated by the dashed arrow and circled bar.
Knowledge Elements about the Dashed Arrow and Circled Bar.
As reported in Table 1A, participants could select one to seven choices about the meaning of the dashed arrow and circled bar. Participants’ interview explanations revealed which of these choices most strongly cued their thinking (Table 2). For a few biology participants and the majority of biochemistry participants, the dashed arrow and circled bar cued the knowledge element “negative feedback inhibition,” but the representation prompted other knowledge elements as well, including some not provided in the problem set (Table 2). This question provides the first opportunity within the interview for participants to exhibit representational competence regarding an important piece of the metabolic pathway.
Knowledge element, i.e., dashed arrow and circled bar represent … | Biology participants | Biochemistry participants | |
---|---|---|---|
Negative feedback inhibition | 7 | 17 | |
Intermediate going back to be part of the previous chemical reaction | Intermediate being “recycled” | 19 | 6 |
Intermediate being “reverted” | 8 | 5 | |
A reversible reaction | 2 | 2 | |
Negative feedback because of the negative sign | 10 | 11 | |
Intermediate binding to the enzyme | 9 | 4 | |
An electron donated to step B | 0 | 1 |
The most prevalent set of knowledge elements cued by the dashed arrow and circled bar, particularly among biology participants, was that the dashed arrow and circled bar represent IV-CoA going back to be part of the previous chemical reaction (i.e., the conversion of fatty acyl thioester to IV-CoA). Some participants described this notion as IV-CoA being “recycled” (Table 2). These participants explained a process in which IV-CoA serves as a necessary component for the conversion of fatty acyl thioester into IV-CoA. Consider Brooklyn’s description of what the dashed arrow and circled bar represent:
Brooklyn: [IV-CoA is] being added back in right here to B, so that it can somehow bond with the fatty acyl thioester and make some more of itself.
Similarly, participants described the dashed arrow and circled bar as IV-CoA being “reverted” back to a previous compound, usually fatty acyl thioester (Table 2). Some individuals thought that this was a necessary part of the process of creating IV-CoA. Still others saw the dashed arrow and circled bar as equivalent to a “reversible reaction,” to be used when the pathway needed more fatty acyl thioester. An example of this idea is shown here by Casey:
Casey: Then the reaction is just gonna essentially go back to [enzyme] B and the thioester because of the buildup of IV-CoA that’s going to push it back up the chain.
Participants’ retrieval of these specific knowledge elements (i.e., recycling, reverting, or a reversible reaction) aligns with the “what-you-see-is-what-you-get” (WYSIWYG) intuitive knowledge element (Elby, 2000). They see an arrow that points backward, so they interpret it as a backward reaction of some type. This is rational. In fact, the other “back” arrows shown in the pathway are intended to signify a backward reaction, and all participants understood this fact (unpublished data). The pieces perspective and literature on representational competence suggest that learners can build their ability to override the WYSIWYG intuitive knowledge element, and other intuitions, in favor of the intended scientific meaning (i.e., negative feedback inhibition) through instruction that explicitly prompts them to interpret these representations and tie them to scientific concepts (Volkwyn et al., 2020).
Another knowledge element activated by the dashed arrow and circled bar was “negative feedback because of the negative sign” (Table 2). This was true of both biology and biochemistry participants, including Bryce and Camron:
Bryce: I feel like the circled bar would have something to do with it being negative. So, in that thinking, I would say that this would be negative feedback or inhibition, and it would not be positive. And I’m only saying that because I see this as being a negative sign.
Camron: So the dashed arrow implies regulation of some sort of the metabolic compound on the activity of the enzyme. And the circled bar, since it’s a negative—a minus sign—it would be negative inhibition.
Participants’ reference to “negative feedback because of the negative sign” also aligns with the WYSIWG knowledge element (Elby, 2000). We designed the visual representation with a bar precisely because it signals “negative.” However, participants need to recruit knowledge elements beyond “bar means negative.” Students like Bryce and Camron need to build representational competence learning to override their WYSIWYG intuition and instead draw upon knowledge of the molecular interactions that underly negative feedback inhibition.
Interestingly, some participants did recruit a resource for the molecular mechanisms of negative feedback inhibition, showing a high degree of representational competence. These participants noted that the dashed arrow and circled bar indicate IV-CoA binding to and inhibiting enzyme B. We found this idea among both biology and biochemistry participants (Table 2). Consider Cheyenne’s description of the dashed arrow and circled bar:
Cheyenne: [IV-CoA] is attaching to this [enzyme B]. When a lot of [IV-CoA] is present, it’s signaling to enzyme B to stop working basically, so this reaction stops because you already have a lot of this product. So that’s allosteric inhibition.
Finally, the dashed arrow and circled bar caused one biochemistry participant to think of electrons. In another example of WYSIWYG, this student associated the circled bar with the negative charge of electrons and reasoned that an electron was being added back to reaction step B. This student used a knowledge element that is appropriate, and even expected, for biochemistry (e.g., in the context of full and partial charges on atoms; Halmo et al., 2020), but needs to be refined. A negative sign indicates electrons in some circumstances and negative regulation in others.
In summary, these data show that the dashed arrow and circled bar cued a variety of knowledge elements for students, including the expected one (negative feedback and inhibition), intuitive ones (e.g., negative because of the negative sign), and one that shows participants’ representational competence and mechanistic understanding (intermediate binding to enzyme B). These data suggest that most students need explicit instruction to interpret visual representations in ways that align with disciplinary concepts.
Knowledge Elements Pertaining to Student Focus on Different Metabolic Compounds.
Metabolic pathways like the one presented in our problem set are interconnected systems. Metabolic compounds are not lined up in the cell to react in order. Instead, they move around in cellular space like vegetables in soup. Reactions occur simultaneously. Perturbations in the concentration of one metabolic compound can impact all other aspects of the pathway as well as other pathways. With this reality in mind, the ultimate aim of our problem set was for participants to discuss how each metabolic compound would be affected by changes to a single pathway component. Yet participants mostly made predictions about pathway dynamics with a focus on single reactions and rarely dealt with impacts on the initial reactions of the pathway. Thus, a second category of knowledge elements we identified deals with students’ focusing their attention on particular metabolic compounds during problem solving: distal reactant (i.e., thiamine phosphate), proximal reactant (i.e., fatty acyl thioester), regulatory compound (i.e., IV-CoA), and end products (i.e., DMA-CoA and IB-CoA; Table 3).
Knowledge element, i.e., students focused their attention on the… | Biology participants | Biochemistry participants |
---|---|---|
Distal reactant: thiamine phosphate | 3 | 7 |
Proximal reactant: fatty acyl thioester | 5 | 9 |
Regulatory compound: IV-CoA | 15 | 22 |
End product: DMA-CoA | 16 | 21 |
End product: IB-CoA | 17 | 22 |
We found that the problem set most strongly cued students to focus on IV-CoA, which is the regulatory compound involved in every modification we asked students to consider, and the end products (i.e., IB-CoA, and DMA-CoA; Table 3). By end products, we mean those compounds found at the end when reading the pathway from top to bottom. All biochemistry participants and almost all biology participants described effects on the regulatory compound and the end products. Bobbi’s prediction about the impact of Enzyme C not working anymore typifies participants’ strong tendency to think about the problems from the vantage point of these two focal points:
Bobbi: [Enzyme C not working anymore] would affect only this pathway [indicates the reaction from thiamine phosphate to DMA-CoA]. So it wouldn’t affect the whole system. It would affect this way because if [enzyme C] stops working, then it’s gonna stop working to produce the DMA-CoA, but it won’t be affecting this one [indicates the pathway from IV-CoA to IB-CoA] since it’s using a completely different enzyme.
In contrast, few participants paid attention to thiamine phosphate and fatty acyl thioester, which are the distal and proximal reactants feeding into the regulatory compound (i.e., IV-CoA; Table 3). Even when the interviewer specifically asked about these compounds, participants were not prone to think that the impacts on thiamine phosphate and fatty acyl thioester were noteworthy. For example, the interviewer asked if introducing a branch point in the pathway would affect flux before the branch point. Camron and Cheyenne exemplify the responses in our sample:
Camron: No, it would only affect the concentration of DMA-CoA and IB-CoA, because it’s just working with how much IV-CoA is available at this predetermined place.
Cheyenne: Since the IV-CoA is now being distributed amongst two branches, there’s less of that in general, so there’s less of it to inhibit enzyme B. … So it’s not changing anything about the thiamine phosphate to the fatty acyl thioester, but it could be decreasing their concentrations, if anything.
Even when participants expressed there might be some change in the amounts of thiamine phosphate and fatty acyl thioester, they minimized the importance of that change. Cheyenne suggested a decrease in concentrations as an afterthought—a side effect that may or may not occur, and that would have no significance either way.
It is not surprising that participants focused on IV-CoA and the end products. We asked participants to consider modifications directly connected to IV-CoA, so using this knowledge element is expected and appropriate. Likewise, participants may have focused on the end products because their biology course and to some extent their biochemistry course encouraged them to think of metabolic pathways from this perspective (see Methods). Also, drawing pathways from top to bottom like we did may bias students to use an intuitive process of focusing on the compounds that come “last.” Yet it is important for students to focus on all intermediates in a pathway, because doing so opens them to the connectedness among different pathways and the impact of single changes on an entire system. Our data suggest the need for explicit instruction to support students to consider all of the metabolic compounds in a pathway.
Assembly of Knowledge Elements into Solutions
The previous section reported the knowledge elements participants used when thinking about metabolism. We also wanted to learn how participants assemble these resources during problem solving, which we addressed using resource graphs. Here, we present the resource graphs for three purposefully selected participants who used different knowledge elements about the dashed arrow and circled bar and focused to different extents on the various metabolic compounds. Each resource graph reveals a particular participant’s coordination of individual knowledge elements. We begin with Bailey and Carson to show the independent, fluctuating nature of participants’ resource use, which was most characteristic of our sample. We end with Charlie to show how student thinking can advance to greater coherence and stability, a rare occurrence in our sample.
Bailey: Independent, Fluctuating Knowledge Elements with a Focus on the Regulatory Compound and End Products.
We present biology student Bailey’s resource graph in Figure 3. Bailey’s resource graph shows utilization of a mixture of independent knowledge elements about the dashed arrow and circled bar, low representational competence, and a view of the pathway as a step-by-step process meant to make DMA-CoA and IB-CoA, rather than a multicomponent system controlled by feedback inhibition (Figure 3).

FIGURE 3. Resource graph for Bailey. White boxes indicate sections of the metabolism problem set that are the focus of this study. White triangles indicate specific questions within each section. Knowledge elements are shown as text within ovals. Arrows indicate the step-by-step flow of knowledge elements. Ovals with bold, colored outlines indicate different knowledge elements about the dashed arrow and circled bar: orange is for IV-CoA going back to be part of the previous chemical reaction, light blue is for negative feedback because of the negative sign, and dark blue is for negative feedback inhibition. The fill color of each oval represents different knowledge elements for student focus on metabolic compounds: gray fill is for focus on the regulatory compound or end products, and white indicates that the participant made no reference to a metabolic compound.
When Bailey was asked what the dashed arrow and circled bar mean, they drew upon four knowledge elements: negative feedback because of the negative sign, negative feedback inhibition, the intermediate being recycled, and the intermediate being added back as a reactant (i.e., reverted; Figure 3, left side). Bailey likened the dashed arrow and circled bar to a similar representation from their biology class, where one has to input some ATP to make more ATP product and have a net gain. Bailey also pointed out that the dashed arrow looks “sort of like a recycling sign.” Bailey appears to have used these knowledge elements independently. When they were later asked to make predictions about pathway perturbations, they abandoned the elements about negative feedback inhibition and only carried forward the elements about IV-CoA being added back to the previous reaction. For example, Bailey explained that when IV-CoA cannot bind to enzyme B, IV-CoA can no longer go back into enzyme B. They reasoned that because IV-CoA binding to B is essential for IV-CoA to be recycled, enzyme B will not work properly and the reaction will not proceed. Thus, they concluded that less IV-CoA, DMA-CoA, and IB-CoA will be produced and flux will be affected. Additionally, Bailey’s use of knowledge elements about the dashed arrow and circled bar fluctuated as they progressed through the three contexts. In the first context (i.e., introduction of a branch point), Bailey never considered the dashed arrow and circled bar. In the second context, when the problem explicitly cued them to think about binding between IV-CoA and enzyme B, Bailey did shift their attention strongly to the dashed arrow and circled bar. Yet in the third context (i.e., enzyme C no longer works), Bailey only used the recycling element at the very end of their solution.
In terms of their focus on the distinct metabolic compounds, Bailey only focused on the regulatory compound and end products (Figure 3). Bailey seemed to think of IV-CoA as being able to go in three directions, IB-CoA, DMA-CoA, or back to the fatty acyl thioester to IV-CoA reaction. Bailey gave some indication that metabolic compounds exist in pools of molecules (i.e., “There will be less DMA-CoA now with the introduction of the branch point”). Yet this notion fluctuated, because other times Bailey did not consider that less production of one compound would create the possibility of more production of a different compound (e.g., “Enzyme C not working will affect the DMA-CoA branch, but not the IB-CoA branch”).
In summary, Bailey discussed several knowledge elements about the meaning of the dashed arrow and circled bar, but they only saw the relevance of a couple of these elements, and only for one of the three problem scenarios. Bailey’s ideas about the dashed arrow and circled bar seem loosely held and specific to the context at hand. We would not expect Bailey to apply these ideas in a different context, for example, if negative feedback inhibition were visually represented in a different way. Even though Bailey initially connected the dashed arrow and circled bar with negative feedback, they did not possess the representational competence to apply this concept during problem solving. Bailey also did not see the relevance of the distal and proximal reactants. Instead, they thought of the pathway as primarily about the end products.
These results suggest that students like Bailey need explicit guidance to draw forth more knowledge elements about negative feedback inhibition, reconcile those elements with the notion of recycling and reversion, and practice using elements about negative feedback inhibition to explain how changes in any pathway component can affect every pathway component.
Carson: Independent and Linked Knowledge Elements with a Focus on All Metabolic Compounds.
Figure 4 shows the resource graph for biochemistry student Carson. Carson drew upon multiple knowledge elements about the dashed arrow and circled bar, some of which they linked and used variably during problem solving, and others of which they kept independent and eventually abandoned. Like Bailey, Carson’s representational competence was low. Yet Carson focused attention on every metabolic compound during problem solving (Figure 4).

FIGURE 4. Resource graph for Carson. White boxes indicate sections of the metabolism problem set that are the focus of this study. White triangles indicate specific questions within each section. Knowledge elements are shown as text within ovals. Arrows indicate the step-by-step flow of knowledge elements. Ovals with bold, colored outlines indicate different knowledge elements about the dashed arrow and circled bar: yellow is for an electron being added back to step B, orange is for IV-CoA going back to be part of the previous chemical reaction (i.e., recycling or added back as a reactant), yellow-orange is for the linkage of the electron idea and the idea of IV-CoA going back, and dark blue is for negative feedback inhibition. The fill color of each oval represents different knowledge elements for student focus on metabolic compounds: gray fill is for focus on the regulatory compound or end products, blue is for focus on the distal or proximal reactants, and white indicates that the participant made no reference to a metabolic compound.
Carson drew upon multiple knowledge elements when asked what the dashed arrow and circled bar mean: an electron donated to step B, IV-CoA being recycled, IV-CoA being added back as a reactant, and negative feedback inhibition. One of these ideas compelled Carson the most, and they linked it to two other knowledge elements. Carson kept the other knowledge element completely independent. Their solution unfolded in this way. As soon as Carson encountered the dashed arrow and circled bar, they stated that the representation looked like an electron being donated to reaction step B. Carson then considered the multiple true-false prompt “IV-CoA being recycled” and reasoned like this: because the charge (i.e., the electron) going back to reaction step B contributes to the entire pathway, the symbol potentially indicates recycling. Carson did something similar when considering the multiple true-false prompt “negative feedback inhibition,” stating that “an electron is negative; [therefore], this is negative feedback inhibition.” In contrast, when Carson read the prompt “IV-CoA being added as a reactant,” they generated an independent line of thought. Abandoning the intuitive knowledge elements of the electron, recycling, and negative feedback inhibition ideas, Carson stated that IV-CoA is the reactant for the IV-CoA to DMA-CoA reaction, so the dashed arrow and circled bar represent IV-CoA being added back as a reactant. Thus, with Carson we see expression of a variety of knowledge elements, some that they thought of independently and some that they linked.
Next, Carson went on to consider the impact of perturbations to the pathway. Here they carried forward the ideas of an electron being added to the previous reaction, but they did so with variability. When asked about the effect of adding a branch point, Carson stated that the electron being added is powering reaction B, but they did not use this idea to make a prediction about the branch point. When asked what would happen if IV-CoA can no longer bind to enzyme B, Carson drew upon the electron addition idea once again. They stated that when the electron is added to step B, the reaction goes back to fatty acyl thioester and back down, which is important for the pathway to function. This part of the process would be unable to function anymore (i.e., if IV-CoA can no longer bind to enzyme B), so the entire reaction pathway will not work. This would limit the reaction to IV-CoA, DMA-CoA, and IB-CoA and affect the flux through the pathway. However, when asked what would happen to the pathway if enzyme C does not work anymore, Carson set aside the electron donation idea and simply walked through the steps of the pathway.
In terms of Carson’s focus on distinct metabolic compounds, they considered all five compounds during problem solving (Figure 4). Unlike Bailey, who seemed to think of the pathway as existing for the formation of the end products, Carson considered every compound in their solutions. When thinking about the branch point problem, Carson pointed out that the conversion of fatty acyl thioester to IV-CoA is powered by an electron. When considering the impact of IV-CoA no longer binding to enzyme B, Carson predicted an effect on thiamine phosphate and fatty acyl thioester but not DMA-CoA. Finally, when considering what would happen if enzyme C does not work anymore, Carson noted that the steps catalyzed by enzymes A, B, and D would still be working. These results suggest that Carson viewed every metabolic compound as an important factor to consider.
In summary, Carson showed low representational competence in that they displayed a number of different knowledge elements about the dashed arrow and circled bar, some which they abandoned and others of which they linked and used variably during problem solving. Carson also used all five metabolic compounds during problem solving, showing the intuition that a metabolic pathway is a system where a change in one component can affect every other component.
These results suggest that students like Carson need encouragement to build representational competence and to continue refining their integration of disparate knowledge elements. For example, Carson would benefit from an instructor pointing out that, yes, negative signs often symbolize negative charge (i.e., electrons), but that they also symbolize negative regulation (i.e., decreasing activity). Like Bailey, Carson needs additional guidance about negative feedback inhibition, whether it be opportunities to build upon related ideas (e.g., the interaction between IV-CoA and enzyme B is like a ligand binding to a receptor) or opportunities to learn new terminology (e.g., the interaction between IV-CoA and enzyme B is an allosteric interaction). Unlike Bailey, who needs guidance to consider every pathway component, Carson only needs encouragement to continue this practice.
Charlie: Coherent, Stable Knowledge Elements with Attention to Most Metabolic Compounds.
Figure 5 shows the resource graph for biochemistry student Charlie. Charlie showed high representational competence, drawing upon a single knowledge element when asked about the dashed arrow and circled bar and using this element stably across all three problem contexts. Charlie also considered most of the metabolic compounds in the pathway.

FIGURE 5. Resource graph for Charlie. White boxes indicate sections of the metabolism problem set that are the focus of this study. White triangles indicate specific questions within each section. Knowledge elements are shown as text within ovals. Arrows indicate the step-by-step flow of knowledge elements. Ovals with bold, colored outlines indicate different knowledge elements about the dashed arrow and circled bar: dark blue is for negative feedback inhibition. The fill color of each oval represents different knowledge elements for student focus on metabolic compounds: gray fill is for focus on the regulatory compound or end products, blue is for focus on the distal or proximal reactants, and white indicates that the participant made no reference to a metabolic compound.
Charlie recognized the dashed arrow and circled bar as negative feedback inhibition. Unlike Bailey and Carson, Charlie did not state that the symbol represented any other thing (e.g., IV-CoA being recycled). Charlie then defined negative feedback inhibition using the context of the problem: “production of IV-CoA inhibits enzyme B.” Charlie also went further than other students in our sample and stated that with negative feedback inhibition “there must be an allosteric enzyme,” as this tends to be the mechanism by which negative feedback inhibition functions.
When asked to analyze perturbations in the pathway and their effect on flux, Charlie used their coherent understanding of negative feedback inhibition for reasoning and also focused attention on the pathway as a whole, not just the end products. With the introduction of the branch point, Charlie stated that the new branch would decrease the amount of free IV-CoA available. With less IV-CoA available, there would be less negative inhibition of enzyme B, resulting in an increased conversion of fatty acyl thioester into IV-CoA. In the second scenario, where IV-CoA can no longer bind to enzyme B, Charlie again used the idea of negative feedback inhibition, pointing out that it would be disrupted. Charlie then predicted the outcome on concentrations of fatty acyl thioester, IV-CoA, DMA-CoA, and IB-CoA. When asked about enzyme C not working, Charlie reasoned that there will be less production of DMA-CoA from IV-CoA, resulting in a buildup in the concentration of IV-CoA. They then pointed out that this would affect negative inhibition, as the buildup of IV-CoA would result in increased negative inhibition of enzyme B to slow the production of IV-CoA. Interestingly, Charlie never made a prediction about thiamine phosphate.
In summary, Charlie showed high representational competence regarding the dashed arrow and circled bar and negative feedback inhibition. While Bailey and Carson displayed multiple, competing ideas about the dashed arrow and circled bar and used only one or two during problem solving, Charlie stuck with their singular understanding through every problem scenario. All of Charlie’s statements converged on the underlying principle that IV-CoA negatively regulates enzyme B and, thus, controls pathway flux. Also, Charlie considered the impact on all metabolic compounds except thiamine phosphate.
Students like Charlie have refined their intuitions and learned to see the problem in a way that resembles that of experts. Thus, they respond in the scientifically expected way. However, students like Charlie certainly still have limits to their use of knowledge elements. Charlie could benefit from more complex problems. For example, how would Charlie respond if asked to consider the utilization of IV-CoA by other pathways (e.g., as a regulator of another enzyme with a different binding constant)? We predict that Charlie would draw upon additional knowledge elements and that they would need guidance to sort out their intuitions in this more complex situation.
Limitations
We were able to identify multiple knowledge elements cued by a metabolism problem set by interviewing 44 students at two undergraduate levels. The knowledge elements we discovered are unlikely to be the only ones about metabolism that exist among undergraduate life sciences students, because the problem set used in this study provides only one way of visually representing metabolism and only three problem-solving scenarios, all within the same pathway. The pieces framework predicts that the diversity of knowledge elements would increase with research using different problem sets and contexts. Additionally, students in other samples may bring additional knowledge elements to bear on metabolism problems. Finally, think-aloud interviews only reveal the ideas that participants say aloud. Participants may have used other knowledge elements that they did not express verbally.
DISCUSSION
In this study, we explored the knowledge elements introductory biology and biochemistry students used when solving problems about metabolism. We discovered a diverse array of knowledge elements that most participants used independently and with fluctuations across contexts. We view these results as the interaction among participants’ experientially grounded intuitions, scientific ideas, and the assessment. Here, we present several implications for research and instruction and conclude with a statement of the impact of research on student thinking.
Research Implications
Researchers who take the pieces perspective search for the independent, fine-grained elements of student thinking and how these elements interact within and across problem-solving contexts (Scherr, 2007; Gouvea and Simon, 2018; Rodriguez and Towns, 2019; Rodriguez et al., 2020; Lira and Gardner, 2020). Indeed, we found evidence for the fragmented, piece-like nature of student thinking about metabolism. Students in our sample used a wide range of knowledge elements to describe the dashed arrow and circled bar, not generally showing the representational competence to consistently link this symbol to the underlying scientific concept. They also focused mostly on end products rather than upstream components. Our sample consisted primarily of students like Bailey and Carson, who drew upon these knowledge elements independently and in a way that fluctuated with the problem context. With Charlie, we found one rare instance of coherent and stable use of knowledge elements and high representational competence. Charlie’s performance illustrates how students’ can refine their once-varied, fragmented, and fluctuating knowledge elements into a more unified set of scientific ideas that cohere and are stably applied.
Pieces researchers assume that students have good reasons for thinking the way they do and that students’ seemingly incorrect ideas are instead misapplied or indicative of ambiguous science instruction. We see rational reasons for the variety of knowledge elements students expressed in our study, some of which we described alongside our results. Consider the dashed arrow and circled bar. We intended for this symbol to represent negative feedback inhibition, and many participants gravitated toward this idea. Yet participants also thought that it represented the intermediate going back to be part of the previous chemical reaction. We argue that this is a WYSIWYG intuitive knowledge element (Elby, 2000); back arrows mean the reaction is going backward. Also, perhaps participants thought of recycling because the visual resembles the circular loop that denotes recycling in a student’s everyday life. Participants may have made a subconscious connection to recycling when they did not immediately recognize the visual. For many participants in our sample, the dotted, curved arrow simply was not a strong enough cue to indicate an entirely different process than that indicated by the solid, straight arrows. Further, consider participants’ focus on downstream versus upstream components in the metabolic pathway. The presentation of pathways in a stepwise pattern (fatty acyl thioester gets turned into IV-CoA, which gets turned into DMA-CoA) may play upon students’ natural biases (i.e., this pathway exists to make DMA-CoA). This could lead to underlying notions that every pathway has tangible goals and the end products are what matters. These findings warrant at least three lines of further research.
First, we need research aimed at improving students’ representational competence. This will involve 1) discovering the knowledge elements cued by various visual representations, 2) designing instructional materials based on what we learn, and 3) investigating whether these materials improve students’ representational competence. Conventions are lacking for visually representing concepts like metabolism (Kozma and Russell, 2005; Offerdahl et al., 2017; Wright et al., 2017). We designed the visual representations in our study based on feedback from experts, but experts did not agree on the best way to represent negative feedback inhibition. Some experts liked the representation we used, but others wanted a curved line ending in a flat, perpendicular line instead of an arrow. Yet even if experts agreed on the way to represent metabolism, their intuitions would be different from those of students (Tversky et al., 2002; Smallman and John, 2005). What we really need is basic research that investigates the knowledge elements cued by different visual representations, including ones commonly used in instruction and ones that are intentionally designed with attention to principles of human perception. Then, we should design instructional materials that respond to our research findings and ask whether representational competence is greater with the newly designed materials. Such work could follow a trajectory like that of Novick and Catley on cladograms (e.g., Novick and Catley, 2007, 2013, 2014; Catley and Novick, 2008). Novick and Catley discovered that tree-formatted cladograms promote better student learning than ladder-formatted cladograms. One cause for this difference is that human perceptual tendencies lead students to interpret ladder cladograms in a way that conflicts with the scientifically accurate segregation of taxa into nested levels (Novick and Catley, 2007). This research led to shifts in the presentation of cladograms in textbooks and instructional materials and, thus, to improved student learning (Novick and Catley, 2007; Schramm et al., 2021). We need this same type of systematic inquiry about visual representations of metabolism and also visual representations of concepts across biochemistry and the life sciences.
Second, research is needed that reveals students’ knowledge elements related to the causal mechanisms of negative feedback inhibition. It was rare in our study for participants to discuss the molecular-level process by which a compound like IV-CoA binds to the allosteric site on an enzyme, changes the structure of that enzyme, and thus regulates the enzyme’s catalytic activity. Another recent study on metabolism assessment showed the same finding (Villafañe et al., 2021). We hypothesize that students do, indeed, possess relevant knowledge elements. For example, the process of negative feedback inhibition is similar to that of a ligand binding to a cell surface receptor and triggering events inside the cell, which is commonly taught in introductory biology and physiology courses. We need research to discover how best to help students make connections like these and move beyond recognizing negative feedback (e.g., because of the negative sign), which many of our participants did, toward causal mechanistic understanding.
Third, and more generally, the pieces perspective should be applied to additional investigations of student thinking in the life sciences. Only a handful of pieces’ studies exist in biology education research (Nehm and Ha, 2011; Gouvea and Simon, 2018; Lira and Gardner, 2020; Slominski et al., 2020), even though the perspective has been productively used in physics (e.g., Hammer, 2000; Sayre and Wittmann, 2008; Scherr and Hammer, 2009; Weliweriya et al., 2019) and chemistry (e.g., Heisterkamp and Talanquer, 2015; Becker and Towns, 2012; Becker et al., 2017; Rodriguez et al., 2018, 2020; Rodriguez and Towns, 2019). The pieces perspective facilitated our discovery of many knowledge elements students used when solving metabolism problems, and the biology and biochemistry education communities are now better equipped to create instructional materials that support student learning. Biology education has much to gain from the pieces perspective, precisely because this perspective privileges and prioritizes student thinking.
Implications for Metabolism Instruction
The pieces model promotes the notion that instruction should help students refine their intuitions by recognizing the utility of their intuitions under certain circumstances and the need to refine their intuitions under others (Elby, 2001). Our results support this view, and we encourage instructors to take class time to hear what students are thinking and to use prompts, questions, and other scaffolds that help students reshape their knowledge (Robertson et al., 2015). Further, our findings lead to specific suggestions for instructors who teach metabolism.
Overall, our data suggest that metabolism should be taught as a concept in its own right, not as a collection of examples. Metabolism is typically taught by marching through a series of pathways, including glycolysis, cellular respiration, and photosynthesis. Our data illustrate that conceptual understanding of metabolism and problem solving requires integration of knowledge elements about visual representations and processes like negative feedback inhibition. Some students, like Charlie, may eventually refine and integrate these knowledge elements as they examine many example pathways. But a more expedient and successful approach would be to explicitly instruct students to apply and refine relevant knowledge elements (e.g., as discussed earlier regarding the causal mechanisms of negative feedback inhibition).
Our data also indicate that instructors should teach pathways as systems, not as step-by-step processes. Students in our sample tended to segment pathways and focus primarily on the downstream products. This suggests that students need help to focus on all metabolic compounds in a pathway. They need to be instructed that a change in an individual chemical reaction will influence all metabolic compounds in the pathway and possibly other pathways. This could be handled, for example, by simply asking students to describe how the concentrations of each metabolic compound in a pathway changes due to negative feedback inhibition.
As seen among our participants, the visuals in instruction and assessment may not adequately align with students’ knowledge elements. Students will likely build more coherent understanding of metabolism if they are explicitly instructed about the meaning of common visual representations. We recommend that instructors walk students through the visual representations used in a metabolic pathway, including the different types of arrows and other symbols, like our dashed arrow and circled bar, to bring to light common interpretations of the visuals. Many of these seemingly simple symbols represent a process with multiple steps, including diffusion of compounds within a cell, binding through noncovalent interactions, changes to the structure and catalytic activity of enzymes, and changes in flux through individual reactions and the pathway as a whole.
Inspired by our findings, we created a metabolism activity that we used in a biochemistry course (Supplemental Figure S4). The activity explicitly guides students about the meaning of visual representations, cues their relevant prior knowledge (e.g., reversibility of chemical reactions, binding through noncovalent interactions), promotes integration of ideas from chemistry and biology, and presents metabolic pathways as systems. The activity uses an example pathway. Yet the point of the lesson is not to learn the example, but to see the example as an illustration of ideas in play across all metabolic pathways.
The instructional interventions we suggest could be applied at the introductory biology or biochemistry level. Instructional level was not a critical distinction in our findings, although we saw some differences between the two samples, particularly in their selection of ideas about the dashed arrow and circled bar. Rather, our data suggest that students at both instructional levels would benefit from opportunities to refine and build on their intuitions about metabolism. That being said, it is worth considering the typical learning objectives of introductory biology compared with biochemistry. Given the breadth of material introductory biology must cover, metabolism will likely be limited to the examples of cellular respiration and photosynthesis. There may simply not be time to allow students to deeply explore the concepts of metabolic pathway regulation or pathways as systems. However, introductory biology instructors can be mindful of our findings and shift their focus even in small ways, for example, by carefully considering their use of visual representations or linking negative feedback inhibition to related phenomena commonly considered in introductory biology (e.g., enzyme–substrate interactions and ligand–receptor binding). In contrast, metabolism is a central focus in biochemistry courses, and these courses will need to do the heavy lifting on metabolism. Many biochemistry instructors may assume that students enter their class with well-developed, stable ideas about metabolism, but more than likely their biochemistry students resemble our participants, entering biochemistry with many pertinent but independent ideas about metabolism that they will need help to refine.
CONCLUSION
Our research revealed students’ knowledge elements about metabolism. Students used a diverse array of knowledge elements and assembled them in independent and fluctuating ways across problem-solving scenarios. Our findings show the impact of research on student thinking and point to the utility of the pieces framework and to research aimed at improving representational competence. Our findings also provide a model for using basic research on student thinking to guide instruction. Instruction can progress as data reveal the variety and rationale of the knowledge elements that comprise student thinking. Instructional materials should be created to provide scaffolding that maximizes students’ knowledge refinement and integration.
ACKNOWLEDGMENTS
We would like to acknowledge the study participants for their time and effort. We also express gratitude to our supportive colleagues in the Biology Education Research Group at UGA for their helpful feedback on earlier drafts of this article. We thank Stephanie Halmo for insightful feedback on later drafts of the article. We thank Matthew Lira for guidance on use of the pieces perspective. This material is based upon work supported by the National Science Foundation under grant no. DRL1350345. Any opinions, findings, and conclusions or recommendations expressed in this material are those of the authors and do not necessarily reflect the views of the National Science Foundation.