What College Biology Students Know about How Vaccines Work
Abstract
Vaccines are an important and societally relevant biology topic, but it is unclear how much college biology students know about how vaccines work and what inaccurate ideas they have about that process. Therefore, we asked more than 600 college students taking biology courses at various levels to explain, “How does a vaccine work?” in a free-response format. Based on authoritative sources and responses from immunology and other biology faculty, we created a rubric to gauge the basic knowledge and accuracy present in student responses. Basic knowledge was defined as knowing that vaccines mimic the pathogen, elicit an active immune response, and provide protection against future infection. Accuracy was defined as the absence of scientifically inaccurate ideas. We found that advanced biology majors score significantly higher in basic knowledge and accuracy when compared with all other student groups, but there were no differences between entering biology majors, pre–health majors, and non–pre-health majors. We also uncovered a variety of inaccurate ideas, with the most common being that vaccines contain the original, unmodified pathogen. These results provide a new way to gauge college student understanding of how a vaccine works and enrich our understanding of what college students know about this process.
INTRODUCTION
Vaccination is a critical issue that affects everyone and has important consequences for public health (Institute of Medicine, 2013). As society grows increasingly dependent on advances in science and technology, there have been many calls for educational institutions to prepare all students to understand science that is relevant for society (Rutherford and Ahlgren, 1990; American Association for the Advancement of Science, 2009; National Research Council, 2012). While many college undergraduates take a biology course, either as a stand-alone course or as part of a biology major, college biology students often have difficulty relating their biology knowledge to real-world issues. This disconnect is apparent in topics as disparate as genetically modified organisms (GMOs) and antibiotic resistance (Potter et al., 2017; Richard et al., 2017). College biology students will go on to make health decisions for themselves and their families, and some of them will also become healthcare workers who influence other people’s health decisions. Thus, it is important for them to be able to relate the biology they know to health issues like vaccination. However, it is not clear what basic knowledge college students have about the biology behind vaccines or what inaccurate ideas they hold. Education research shows that understanding students’ prior knowledge is critical for teaching them effectively (Sadler et al., 2013; Chen et al., 2020). Thus, in this paper, we develop a tool that instructors can use across a range of college biology courses to measure knowledge about how vaccines work. We then use this tool to understand how basic knowledge of how vaccines work relates to factors such as formal expertise level (where students are in their formal study of biology), confidence in one’s understanding, and inaccurate ideas about vaccines.
What does it mean for students to be knowledgeable about vaccines? A number of studies have attempted to measure how much people, including parents, healthcare professionals, students, and members of the general public, know about vaccines, but there is no consensus about how to define and measure vaccine knowledge. Some studies have asked general questions such as, “What do you know about vaccinations?,” or inquired about a variety of vaccine-related facts (Ho et al., 2017). Other studies have focused on specific aspects of vaccine knowledge, such as which vaccines protect against which illnesses (Wu et al., 2007; Mellon et al., 2014), which vaccines are recommended for which populations of people (Pavia et al., 2003; Mellon et al., 2014; Payakachat et al., 2018; Riccò et al., 2019; Shibli et al., 2019; Nguyen et al., 2020), how serious and prevalent vaccine-preventable diseases are (Salmon et al., 2004; Yudin et al., 2009; Shibli et al., 2017), and what side effects vaccines have (Lewis et al., 1988; Gellin et al., 2000; Maayan-Metzger et al., 2005; Apisarnthanarak et al., 2008; Yudin et al., 2009; Bauer et al., 2020; Belsti et al., 2021). These are all worthwhile pieces of knowledge to have about vaccines. However, because they only relate indirectly to the fundamental concepts in biology and immunology, it is difficult to adapt these questions to measure vaccine knowledge in the context of a biology classroom.
Another popular approach for measuring vaccine knowledge is through quantifying disagreement with inaccurate ideas about vaccines (Stecula et al., 2020a). (Although the term “misconception” is often used to describe any inaccurate idea, regardless of its source or how deep-seated or coherent it is, some people hold more narrow views of which inaccuracies should be called misconceptions [Singer et al., 2012]. Therefore, in this paper, we avoid the term “misconception” and instead use the phrase “inaccurate ideas.”) This approach is the one taken by the popular Zingg and Siegrist vaccine knowledge scale, which is based on a list of commonly held inaccurate ideas and has been used as a measure of vaccine knowledge in many other studies (Zingg and Siegrist, 2012). Tested ideas include, “vaccines are superfluous, as diseases can be treated (e.g., with antibiotics),” “many vaccinations are administered too early, so that the body’s own immune system has no possibility to develop,” and “vaccines cause autism” (Zingg and Siegrist, 2012; Stecula et al., 2020a).
However, an approach purely based on disagreement with inaccurate ideas has some drawbacks for measuring the vaccine knowledge of biology students. Some of the inaccurate ideas tested in popular scales have only a tenuous connection with fundamental biology topics. Also, any such approach to knowledge risks being incomplete or arbitrary. Dozens of inaccurate ideas about vaccines have been documented in the literature. There are ideas that relate more closely to the biology of vaccines, such as ones about what vaccines are made of, whether the immune system can handle vaccines, whether vaccines are effective, whether vaccines are preferable to natural disease, and in what ways a vaccine will permanently alter a person’s body (Jacobson et al., 2007; Amin et al., 2017; Gidengil et al., 2019). There are inaccurate ideas that are not actually about vaccines themselves but about vaccine-preventable diseases, such as them being rare or not serious (Jacobson et al., 2007). Still others relate to deeper biases about statistics, for example, the tendency to overestimate the risk of rare events like experiencing a serious side effect from a vaccine (LaCour and Davis, 2020). New inaccurate ideas appear and spread quickly, particularly on the Internet and social media (Stecula et al., 2020b; Center for Countering Digital Hate, 2021). Thus, an approach to knowledge that relies solely on measuring the number of inaccurate ideas a person holds is in danger of being incomplete, particularly regarding trending inaccurate ideas.
More fundamentally, an approach based only on inaccurate ideas risks missing the accurate knowledge students have, because accurate and inaccurate ideas can coexist. The widely used constructivist framework for learning holds that, because students construct new knowledge out of their existing prior knowledge, knowledge is not replaced but instead built upon or transformed. Indeed, some inaccurate ideas have kernels of information in them that students can use to create accurate ideas (Maskiewicz and Lineback, 2017). The knowledge-in-pieces perspective on constructivism points out that student ideas do not necessarily form a coherent framework and that students can simultaneously hold more accurate and less accurate ideas (Harlow and Bianchini, 2020). Thus, merely counting the number of inaccurate ideas a student agrees with may underestimate that student’s knowledge, and it does not directly inform instructors what existing student knowledge they can build on in their teaching.
Given the paucity of existing vaccine knowledge instruments that focus specifically on how vaccines work, we chose to create one that instructors could use to systematically measure their students’ knowledge of this topic. Understanding how vaccines work requires knowing fundamental concepts in immunology (Moser and Leo, 2010). The limited research that has been done suggested that there is a wide range of knowledge levels about this topic. On the one hand, one study found that most high school students in a particular classroom were able to correctly identify that the influenza vaccine contains inactivated influenza virus (Dumais and Hasni, 2009), and another found that middle school teachers, similar to professional virologists, knew that vaccines resembled the pathogen and acted to stimulate the immune system in service of fighting or preventing future infection (Jee et al., 2015). On the other hand, other studies have found that a significant fraction of middle school students incorrectly believe that vaccines contain “anti-virus” or “chemicals” that fight viruses directly (Jee et al., 2015) and that only half the adults in a diverse sample could provide any mechanistic information at all when asked to explain “how vaccines are supposed to work in the body to prevent disease” (Downs et al., 2008). Given that none of these studies involved more than 40 subjects and that they were conducted in a variety of different age groups, it is difficult to draw conclusions about what college students in general are likely to know or not know about how vaccines work.
Once we have a tool for measuring what biology students know about vaccines, we can apply it to better understand how various factors, such as formal levels of expertise and previous course work, affect students’ level of knowledge. An examination of various curricular standards and standardized assessments suggests that some but not all college students are required to receive instruction about how vaccines work. At the precollege level, none of the National Health Education Standards, the U.S. Next Generation Science Standards, or the AP Biology Curriculum Framework directly mentions anything about vaccines or the immune system (Joint Committee on National Health Education Standards, 2007; NGSS Lead States, 2013; College Board, 2020). Similarly, at the introductory college level, immunology ranked nearly last in a recent survey of what college instructors thought was “essential” in the first-year biology curriculum for majors, suggesting that few instructors teach this topic in introductory courses (Gregory et al., 2011). The topics of immunology and vaccines only begin to appear for more advanced course work for students who choose to learn about physiology, medicine, microbiology, or immunology. For example, the Medical College Admission Test, the Microbiology Concept Inventory, and the Human Anatomy and Physiology Society’s Comprehensive A&P exam test knowledge of how the immune system recognizes and fights against pathogens (Paustian et al., 2017; Human Anatomy and Physiology Society, 2019; Association of American Medical Colleges, 2020). Unsurprisingly, 95% of faculty who teach immunology devote at least a “small” amount of time to vaccines, with 40% giving it a “considerable” or “large” amount of time (Bruns et al., 2021). In addition, some studies have found that science literacy in general increases with the number of science courses taken and that science, technology, engineering, and mathematics (STEM) majors tend to have higher science literacy than non-STEM majors (Nuhfer et al., 2016; Shaffer et al., 2019). Given that only some advanced biology majors seem to be expected to learn about immunology and vaccines and that these students would be more likely to have higher general science literacy, one might expect that only advanced biology majors have much knowledge of how vaccines work. Indeed, one study found that people with a college education were not significantly more likely than other people to be able to explain how vaccines work (Downs et al., 2008). However, there are many informal ways to learn accurate information about vaccines, especially online, which may make up for a lack of formal instruction for students who are interested in biology or health. On the whole, we predict that formal course work in vaccines will increase students’ levels of knowledge and that advanced biology majors will know more on average than intro biology majors and pre–health majors, who will in turn know more than non-biology, non–pre-health majors.
It would also be informative for educators to learn whether confidence in one’s own vaccine knowledge correlates to actual knowledge, because if unknowledgeable students are nevertheless confident in their knowledge, they may not be motivated to learn more (Fischer and Sliwka, 2018). Research shows that students often do not accurately estimate their own level of knowledge (Tanner, 2012) and that, in the general public, many of the people who claim they have the most knowledge about social issues that relate to science, like GMOs, actually have the least (Fernbach et al., 2019). With regard to vaccine knowledge, some studies have found that people who believe certain inaccurate ideas about vaccines are more likely to say that they are knowledgeable or informed about vaccines (Motta et al., 2018; Romijnders et al., 2019). However, it is unclear if that inverse relationship will hold for knowledge of how vaccines work.
Finally, while inaccurate ideas about vaccines have been extensively documented in the literature (Jacobson et al., 2007; Gidengil et al., 2019), it would be valuable for educators to know which inaccurate ideas are commonly elicited when teaching about how vaccines work and the extent to which those ideas relate to having accurate knowledge about vaccines. As the constructivist and knowledge-in-pieces frameworks suggest, there can be a nuanced relationship between the accurate and inaccurate ideas in student minds: While some inaccurate ideas can hinder the learning of accurate information, others may be the result of or even aid the construction of accurate knowledge (Coley and Tanner, 2012; Maskiewicz and Lineback, 2017). Therefore, while it is important to know whether student responses contain any inaccuracies, it is also useful to uncover which inaccurate ideas students have and to understand what relation those ideas have with accurate knowledge. Once these inaccurate ideas are identified, instructors can work to reshape these student ideas and promote conceptual change (Maskiewicz and Lineback, 2017).
Thus, to address gaps in our knowledge of what college biology students know about how vaccines work, we first created a rubric to assess whether a student’s response to the question “How does a vaccine work?” contained the basic components of knowledge of how vaccines work and was fully accurate. Then, we used a mixed-methods approach relating student responses and rubric scores to address the following research questions:
To what extent do college biology students at various levels have a basic and accurate understanding of how vaccines work?
What factors, including having previous course work in vaccines, formal level of expertise, and confidence in one’s own knowledge, correlate with having a basic and accurate understanding?
What common inaccurate ideas do students have about how a vaccine works? To what extent do the presence of these inaccurate ideas correlate with students’ basic knowledge of how vaccines work?
METHODS
Survey Design
Given the lack of an existing instrument to address our specific research questions, we developed a novel written assessment tool to investigate undergraduate students’ understanding of how vaccines work. A summary of the items analyzed in this paper can be found in Table 1. (The full tool also contains many other prompts and is included as Supplemental Material 1, with slightly different versions for students and faculty.)
Question or challenge statement | Response format |
---|---|
If asked by another student in your major,a how would you respond to the following question … How does a vaccine work? | Open-ended response |
Please circle YES or NO in response to the following … I have taken one or more courses where I learned about how vaccines work. | Yes/No |
Please circle YES or NO in response to the following … I am confident in my understanding of how vaccines work. | Yes/No |
The primary item we analyzed was the vaccine knowledge prompt: “If asked by another student in your major, how would you respond to the following question… How does a vaccine work?” This question was intended to reveal each participant’s knowledge of how vaccines work, as opposed to other aspects of vaccine knowledge. Biology faculty (BF) participants were given a version of the survey that substituted the phrase “a professional colleague” for “another student in your major.”
We also analyzed some items relating to attitudes and background knowledge. The items analyzed in this study were “I have taken one or more courses where I learned about how vaccines work” and “I am confident in my understanding of how vaccines work.” These items could be answered by circling “Yes” or “No” to indicate personal agreement or disagreement with the statement. Although students were invited to explain their choices using one or two sentences, many students either did not write anything or did not elaborate much on their choices. Thus, we chose to analyze only the close-ended “Yes” or “No” responses.
Finally, we asked participants to fill out a demographic survey that asked for their major, class standing, gender, race or ethnicity, how many children they had, and whether they were part of the first generation in their family to go to college.
After we developed an initial version of this tool, a small-scale pilot study was conducted with advanced undergraduate and master’s students in biology to yield validity evidence based on response processes (Reeves and Marbach-Ad, 2016). These subjects completed the prompts as students would and as a group discussed how they interpreted the prompts and whether any prompts were confusing. None of the prompts analyzed here were changed as a result of the pilot study.
Participant Populations and Recruitment
We recruited four student participant populations for this study, all from a large, public, urban, master’s-granting university in a generally politically liberal geographic area. All data were collected in 2017–2018. Approval for this study was conferred by the San Francisco State University Human and Research Protections Protocol no. E17-257.
The four participant populations, which correspond to different levels of formal expertise, were advanced biology majors (ABM), entering biology majors (EBM), pre-nursing and pre–physical therapy majors (pre–health majors, PH), and non-biology, non–pre-health majors (NPH). ABMs were biology majors who had completed 1.5 years or more of biology courses for majors. EBMs were biology majors who were just starting the first term of introductory biology for majors. The PH and NPH were non–biology majors who were taking a nonmajors course focused on human health. This course was taken by many students in majors unrelated to health (NPH). However, this course was also one of the first biology courses taken by pre-nursing and pre–physical therapy majors (PH), because it was one of the first courses in a sequence of prerequisites. Students who were undeclared were classified as NPH. Because we were interested in both major and course work as components of “formal expertise,” students were excluded from the analysis if their majors did not match the classes they were in (e.g., an environmental science major taking introductory biology or a biology major taking nonmajors biology). More details about the demographic characteristics of these populations are given in Table 2.
Participant group | Number invited | Sample size | Participation rate | Participants’ racial/ethnic composition* | Participants identifying as female or other gender* | Participants who were first-generation college-going | Participants with children* |
---|---|---|---|---|---|---|---|
NBM– NPH | 189 | 183 | 97% | 25% Asian8% Black29% Latinx19% White16% Multiracial4% Other | 70% | 54% | 1% |
NBM–PH | 114 | 111 | 97% | 38% Asian6% Black30% Latinx13% White13% Multiracial1% Other | 77% | 49% | 2% |
EBM | 242 | 237 | 98% | 30% Asian5% Black42% Latinx12% White10% Multiracial1% Other | 74% | 59% | 1% |
ABM | 106 | 104 | 98% | 42% Asian6% Black27% Latinx18% White6% Multiracial1% Other | 66% | —a | 3% |
BF | 33 | 24 | 73% | 21% Asian46% White33% Otherb | 38% | 38% | 63% |
To recruit student populations, we contacted course instructors and obtained permission for a researcher to distribute and guide students in completing the written survey assessment during class time in the instructors’ courses. All students completed the assessment as an in-person classroom activity for the day but were given the opportunity to opt out of including their responses in the study. Because of an error in data collection, ABM students were not asked about items relating to attitudes, background knowledge, and first-generation college- going status.
We also recruited BF to obtain a comparable data set with presumably expert-level responses. We identified all BF at this university whose primary research or teaching focused on microbiology, physiology, immunology, or cellular and molecular biology and contacted them by email to request their participation. BF were not contacted if they were on leave or if they were present when their students were given the assessment during class time. BF participants were given a $25 Amazon gift card as an incentive to participate. Data from all BF participants were included in this study. More details about the characteristics of BF are given in Table 2. The lower participation rate for BF is to be expected given the different methods of recruitment.
Finally, we recruited a non-overlapping set of five immunology faculty (IF) to review the proposed rubric and common inaccurate ideas. These faculty were recruited because they previously conducted or currently conduct immunology research. They did not come from the same institution where the other data were collected. They were recruited by email without incentives.
Administering the Assessment Tool
Students were given this survey on paper as a part of a class activity during in-person courses. Instructors allowed researcher M.T.O. to give this survey on a day when the instructor would be absent or there were no regular classroom activities planned. The students were not told ahead of time what the survey would ask or that it was about vaccines. Right before giving the survey, the researcher informed student participants that the survey addressed vaccines, that it was not a test or assignment with any impact on the course grade, and that their responses would be anonymous and only identified with a “secret code.” The researcher also encouraged students to write their thoughts fully and completely, even if they did not know the answer to a particular question. Each survey item or group of items (such as the demographics survey) was printed on a separate piece of paper. The researcher distributed a particular page at a time to all students. They had 3–5 minutes to handwrite their responses to the items on that page, and then the next page was distributed to all the students. Therefore, students had little incentive to rush, because they could not start the items on the next page before the researcher allowed it. In most cases, students had finished writing by the end of the 3–5 minutes. BF participants were given the full survey using a similar protocol during in-person one-on-one meetings.
Creation and Validation of a Rubric to Assess Knowledge of How a Vaccine Works
To code the responses of study participants to the “How does a vaccine work?” prompt into discrete categories, we created a rubric. We decided to split our rubric into two pieces: basic knowledge, which would assess whether students understood the basic elements of how vaccines work, and accuracy, which would denote whether students only stated ideas that were consistent with current scientific understanding, as opposed to inaccurate ideas. We decided to analyze basic knowledge and accuracy separately, because many students stated both correct and incorrect ideas and, as suggested by the constructivist and knowledge-in-pieces frameworks of learning, the relation between correct and incorrect ideas can be complicated. Noting such correct and incorrect ideas separately also allowed us to easily analyze the relation between particular correct and particular incorrect ideas.
To make a rubric that would assess basic knowledge, we first made a preliminary list of the basic elements of how a vaccine works that was based on authoritative sources. The goal was for the rubric to be useful for both ABM and non–biology majors. Therefore, our sources were two best-selling undergraduate immunology textbooks (Janeway’s Immunobiology by Weaver and Murphy and The Immune System by Parham), which provide information at the level of an advanced biology major (Parham, 2015; Murphy and Weaver, 2017), and two websites for the general public created by two government agencies, the National Institute of Allergy and Infectious Diseases (NIAID) and the Centers for Disease Control and Prevention (CDC), which a non–biology major might use to understand vaccines (NIAID, 2011; CDC, 2018). In these sources, we looked for information about how vaccines work and listed all the main concepts discussed. We then made a tentative list of the ideas shared by all of our sources.
Next, we analyzed the responses of the BF participants for the presence of the information in the authoritative sources. We reasoned that the BF responses were a reasonable upper limit for the types of responses that knowledgeable undergraduate biology majors could be expected to write under similar conditions. Therefore, we decided that, for something to be a piece of “basic knowledge,” it would have to be present in at least two-thirds of the BF responses. Grounding our rubric in expert responses also constitutes further validity evidence based on test content (Reeves and Marbach-Ad, 2016).
At this point, we had a set of three components of basic knowledge that were present in all authoritative sources and nearly all BF responses. To refine our rubric, one coder, G.K., used the draft rubric to assess the responses of a random sample of 100 non–biology majors, as we hypothesized this would be the group of students with the lowest knowledge of biology and most variety in their responses. With every change to the rubric, responses were reassessed until all students were graded consistently. Afterward, we calculated for every student a basic component score, which consisted of the total number of basic knowledge components that student’s response contained.
We saw that many student responses contained at least one component of basic knowledge yet also contained scientifically inaccurate information. Therefore, we decided to also score responses for accuracy. Responses scored as “accurate” had no inaccurate ideas. We defined an “inaccurate idea” as an idea about vaccines or the immune system that was contrary to what is said by authoritative sources on vaccines.
To summarize students’ knowledge in a way that captured both their basic knowledge and their accuracy, we made a combined knowledge score. This score consisted of the response’s basic knowledge score plus a 1 if the response was fully accurate. Thus, a student who only mentioned prevention but who wrote no inaccurate information would receive a 2, while a student who mentioned all three components of basic knowledge but also mentioned an inaccurate idea would score a 3.
Immunology Faculty Review of Rubric Validity
To further provide validity for the rubric based on test content, we asked five IF to conduct a review of the rubric for basic knowledge. For each of the three components, we asked them to use a four-point Likert-like scale to rate how important each component was to measure a student’s knowledge of how a vaccine works. We also asked how clear the rubric items were. Finally, we asked the IF whether any important pieces of knowledge were missing from the rubric. If a piece of knowledge was cited by any IF as missing, we reviewed the BF responses to see how many of them contained that piece of knowledge.
Analysis and Immunology Faculty Review of Inaccurate Ideas
To analyze inaccurate ideas, we read all student responses scored as “inaccurate,” regardless of basic knowledge score or population type, and extracted all ideas that did not seem to be supported by authoritative sources. A single response could contain multiple inaccurate ideas. Then, we used a thematic analysis approach to group together similar ideas (Saldaña, 2016). We then made a list of proposed common inaccurate ideas that were present in more than 5% of all responses.
We then asked our IF whether each proposed inaccurate idea was in fact scientifically inaccurate and whether the description of that idea was clear. IF could answer “yes,” “maybe,” or “no.” When an IF indicated “maybe” or “no” for being scientifically inaccurate or for clarity, we looked at their comments to see whether the proposed inaccurate idea could be made more clearly inaccurate by a small change in wording of the description of the idea. We considered “small changes” to be adding a caveat or removing a phrase. If such a change was possible, we changed the wording as suggested. We removed any proposed inaccurate ideas that at least two-firths of the IF believed were not actually scientifically inaccurate and that could not be fixed with a small change in wording. The final list of common inaccurate ideas could then be used to identify responses with those particular ideas for further analysis.
Interrater Reliability
To measure interrater reliability, two researchers, G.K. and F.W., each independently coded a new random sample of at least 10% of the student responses, blind to population type, for each component of basic knowledge, overall accuracy, and each individual common inaccurate idea. All qualitative coding reached at least 80% consensus between the trained coders. We also calculated Cohen’s kappa, another measure of interrater reliability, for each component of knowledge, overall accuracy, and each individual common inaccurate idea.
Kruskal-Wallis and Chi-Square Analysis
Relations between a categorical and a numeric variable were analyzed with a Kruskal-Wallis test. This test was chosen because it is suitable for nonnormal data. In our analysis, the numeric variable was either the combined knowledge score or the basic component score, and the categorical variables were the level of formal biology expertise or whether a student had a fully accurate response. Statistical significance was calculated in R (R Core Team, 2019). Effect sizes were calculated using the epsilon-squared statistic (Tomczak and Tomczak, 2014). Dunn’s test was used for post hoc comparisons and was run using the dunn.test package in R (Dinno, 2017), with Bonferroni correction used to adjust p values.
Relations between two categorical variables were analyzed with chi-square tests. In our analysis, the comparisons were between level of formal biology expertise and the presence of particular components of basic knowledge or overall accuracy. Statistical significance was calculated by χ² analysis in Google Sheets (Google), Excel (Microsoft), or R (R Core Team, 2019), and a Bonferroni correction was used to adjust p values. Effect sizes were calculated using Cramér’s V in Google Sheets or Excel.
Linear and Logistic Regression Modeling to Relate Student Factors to Knowledge and Accuracy
To examine the factors that affected the combined knowledge score, we created a linear regression model in R. To make the linear regression model, we modeled the effect of many potential independent variables of interest to the combined knowledge score. We initially added these variables as potential independent variables: formal expertise level, whether a student reported taking course work about vaccines, whether a student reported feeling confident in their knowledge, gender, race/ethnicity, whether they were a first-generation college student, and their years in college. Years in college was treated as a linear variable, with freshmen designated as 0 years and seniors designated as 3 years. Because the ABM were not asked about many of these variables, we only conducted this analysis using the NPH, PH, and EBM student groups.
Similarly, to examine the student factors that affected whether a response contained any inaccurate statements, we used logistic regression modeling for overall accuracy. Logistic regression modeling is appropriate for categorical data with two outcomes, such as being or not being fully accurate (Theobald et al., 2019). The same independent variables were used as in the linear regression models (formal expertise level, whether a student reported taking course work about vaccines, whether a student reported feeling confident in their knowledge, gender, race/ethnicity, whether they were a first-generation college student, and their years in college). The logistic regression modeling was performed using the glm command in the base package in R.
We chose to analyze potential effects of gender, race/ethnicity, and college going–generation status (first-generation vs. continuing-generation college student) because previous education literature has shown “opportunity gaps” in biology performance along these axes (Eddy et al., 2014; Harackiewicz et al., 2014; Theobald et al., 2020). For gender, students who identified as trans were grouped with their stated gender. For race, students were grouped into the categories Asian, Black, Latinx, white, multiracial, and other. “Multiracial” consisted of students who selected or wrote in ethnicities consistent with two or more of the categories Asian, Black, Latinx, white, or Native American. “Other” consisted of students who declined to give their ethnicity and (for privacy purposes) those who chose ethnicities with n < 10.
Because we wanted to examine the effect of gender, race, years in college, and first-generation college student status, we excluded the small number of students who did not answer these questions, who identified as neither female nor male, or who chose a race or ethnicity classified as “other.” In addition, we excluded students who did not respond either “yes” or “no” to the two prompts asking yes or no questions (whether they had taken course work relating to vaccines, whether they were confident in their knowledge). Out of 531 students, a total of 20 students (3.8%) were excluded for any reason.
Model selection was then performed to find which combination of these independent variables fit the data best without overfitting (Theobald, 2018). To select which variables would be present in the final, best-fitting models, we used a “best-subset” approach that considered all possible combinations of independent variables and compared them on the basis of the Akaike information criteria with a penalty for small sample sizes (AICc; Barton, 2020). AICc is an estimator of the relative “goodness of fit” of models, with the lowest AICc indicating the best-fitting model (Theobald, 2018). The models with the lowest AICc were compared using analysis of variance. For models with small differences in AICc (<2) that were not significantly different, the more parsimonious model was used. To perform the best-subset selection, we used the dredge function in the MuMIn package in R (Barton, 2020). All models were fit using restricted maximum-likelihood. The p values were calculated using t tests with Satterthwaite’s method.
Linear and Logistic Regression Modeling to Relate Particular Inaccurate Ideas to Knowledge and Accuracy
To examine the extent to which the presence of various inaccurate ideas correlated to basic knowledge score, we created a linear regression model in R. To make the model, we initially used the presence or absence of the five most common inaccurate ideas as potential independent variables. All student responses were used in this analysis. Similarly, to examine the extent to which the presence of a particular inaccurate idea correlated to whether particular components of knowledge were present, we used logistic regression modeling. Model selection was performed as described in the section Linear and Logistic Regression Modeling to Relate Student Factors to Knowledge and Accuracy.
RESULTS
Here, we discuss the development of a simple rubric for the open-ended prompt “How does a vaccine work?” that can be used to assess a wide range of college biology students on their basic knowledge of how a vaccine works. Then, we use this rubric to show how student knowledge correlates with their level of formal biology expertise, whether they have taken a course addressing vaccines, their confidence in their knowledge, and demographic characteristics. Finally, we compile common inaccurate ideas college students have about how vaccines work and show how they relate to their basic knowledge.
Development of a Rubric to Measure Basic Knowledge of How a Vaccine Works
To develop a rubric to measure basic knowledge of how a vaccine works in response to the prompt “How does a vaccine work?,” our approach was to create a short list of a few “big ideas” that would represent broad concepts important for understanding vaccines. We took that approach instead of creating a longer list of finer details so that our rubric could be used across a wide range of undergraduate courses, including those for nonmajors, and could be used to score short responses like “minute papers.” First, we referenced authoritative sources that would span the range of how an undergraduate might learn scientifically accurate information about vaccines: two best-selling undergraduate biology textbooks and two government websites on vaccines. From those, we made a list of common “big ideas” that were in all four sources. Our initial list consisted of:
A vaccine contains all or part of a (modified) pathogen.
These modifications to the pathogen reduce or eliminate the risk of the vaccine giving or causing the disease.
Vaccines stimulate an immune response similar to that of the original pathogen.
“Immunological memory”
While we knew a rubric based on this list would be scientifically accurate, we wanted to make sure that it would be realistic to expect a knowledgeable undergraduate student to produce a response containing all of these ideas under the conditions we administered the survey: 3–5 minutes of writing time, without preparation. We reasoned that the BF responses would be a reasonable approximation of the upper limit of what knowledge undergraduates could produce. So, we checked the BF responses for the presence or absence of each of these four ideas. We found that although 96% (23/24) of BF expressed the idea that vaccines resembled pathogens (item 1), only 33% (8/24) explicitly stated that vaccines would not cause the disease (item 2). Therefore, we removed item 2 from the rubric. For item 3, all BF (100%, 24/24) stated some way in which vaccines stimulated an immune response, but only 37.5% (9/24) explicitly stated that the immune response elicited was the same or similar to that elicited by the original pathogen. Therefore, we removed the part “similar to that of the original pathogen” from item 3. For item 4, we found that only 58% (14/24) of BF responses used the words “memory” or “immunological memory.” However, a much higher percentage, 96% (23/24), expressed the idea that vaccines protect against future infection. Therefore, we altered this item to read “A vaccine can prevent disease caused by a pathogen or lessen the disease’s severity in the future.”
Next, we wanted to test how easily our rubric could be used to score student responses. We used our rubric to evaluate 100 randomly chosen student responses from non–biology majors, whom we assumed would have a greater variety of responses. From that analysis, we found that it helped graders to have a more precise definition of item 1, “A vaccine contains all or part of a (modified) pathogen.” Thus, we altered the rubric to read “A vaccine contains something that is part of or is shaped like the pathogen, including the pathogen itself or a weakened or modified version of it.” Thus, our rubric now consisted of three items:
A vaccine contains something that is part of or is shaped like the pathogen, including the pathogen itself or a weakened or modified version of it.
A vaccine stimulates an immune response.
A vaccine can prevent disease caused by a pathogen or lessen the disease’s severity in the future.
Finally, we recruited five IF to evaluate our revised rubric for basic knowledge for importance, clarity, and completeness. For importance, our IF all agreed that all three of the remaining rubric items were essential for measuring a student’s knowledge of how a vaccine works. For clarity, most of the IF thought that each of the items was clear. However, one IF chose “item needs minor revisions to be clear” for item 2, because they believed that some students who do not explicitly say that vaccines stimulate an active immune response and instead use language like “vaccines build your immune system” may nevertheless have an accurate idea of how a vaccine works. While that is sometimes true, it is not always true—students who say that vaccines “build your immune system” may instead believe that vaccines make the immune system generically stronger, which is different from activating a specific immune response. Therefore, we chose to keep item 2 as is. For item 3, one IF chose “needs minor revisions,” because the rabies vaccine can be used therapeutically. In response, we changed item 3 to read, “Vaccines function mostly to prevent disease caused by a pathogen or lessen the disease’s severity in the future” to acknowledge the small number of cases where vaccines are used as treatments. Finally, other IF chose “needs minor revisions,” because they wanted items to go into more depth: for item 1, one IF wanted the item to include the concepts of antigens and recognizing non-self, while for item 4, one IF wanted the item to include mentioning memory B and T lymphocytes or contrasting the adaptive and innate immune responses. We investigated these suggestions along with other IF suggestions in the next step, analyzing rubric completeness.
To assess the extent to which our rubric covered the most important knowledge relevant to a basic understanding of how vaccines work, we asked the IF whether they would recommend any additional items to measure student knowledge about how a vaccine works. The IF suggested 12 items, none of which were mentioned by more than one IF. We read through the BF responses again to see how many of them contained each of the suggested items. None of the suggested items was present in more than two-thirds of BF responses. Thus, none were added to the rubric. The full list of items the IF suggested is listed in Supplemental Table S1, along with the percentage of BF who mentioned that item.
Rubric for “How a Vaccine Works”
Our final rubric for responses to the prompt “How does a vaccine work?” contained three components of basic knowledge as well as a category for overall accuracy, as outlined in Table 3. Our detailed coding guide is included in the Supplemental Material, but a general description of how we coded responses is provided here. Overall, when analyzing whether a particular component of knowledge was present in a student’s response, we focused on whether it contained the general concept. If the student’s response contained both the component of knowledge and an inaccurate idea related to that component, we still said the student had that component. We chose to grade the responses in this manner, because constructivism holds that students can simultaneously hold accurate and inaccurate ideas and we were specifically interested in how inaccurate ideas and basic knowledge relate. (However, any response with inaccurate information would not get credit for accuracy.) Our focus on the general concept for the components of basic knowledge also meant that we did not focus on particular vocabulary. For example, students used a wide variety of ways to express the idea that vaccines would lower the chance of getting sick in the future, and all of them counted for prevention. Analyzing prompts in this matter helped us recognize knowledge in the responses of students who had clumsy word choices, such as those who were English-language learners. In addition, our focus on the general concept meant that a student’s explanation did not have to describe all possible scenarios relating to a component of basic knowledge to be given credit for that component. For example, if a student explained how vaccines work using the word “virus,” they could still be given credit for knowing components of basic knowledge, even though some vaccines work against bacteria. Finally, the focus on the general concept meant that we still gave points for components of basic knowledge even if students expressed a concept using anthropomorphic language, such as a vaccine “teaching” the body how to defend against a pathogen. It was often difficult to figure out whether students used such language literally or metaphorically, and there is evidence that even faculty use anthropomorphic language when discussing biology (Betz et al., 2019).
Criteriona | Raw agreement | Cohen’s kappab | |
---|---|---|---|
Basic knowledge | |||
Pathogen-likeness | A vaccine contains something that is part of or is shaped like the pathogen, including the pathogen itself or a weakened or modified version of it.“A certain vaccine contains a small dosage of the pathogen and it is injected into our bodies.”—PH student | 95% | 0.90 |
Immune activation | A vaccine stimulates an immune response.“I think vaccines are something like a weakened strain of a pathogen or their protein markers or something. The immune system detects them and starts making white blood cells and antibodies to combat it.” —PH student | 94% | 0.85 |
Prevention | Vaccines function mostly to prevent disease caused by a pathogen or lessen the disease’s severity in the future.“I guess the body familiarizes itself with it so if you face it again it won’t affect you.” —EBM student | 90% | 0.76 |
Accuracy | |||
Accurate | None of the ideas present are contradicted by authoritative sources on how vaccines work. | 91% | 0.69 |
The three concepts that are included under basic knowledge can be summarized as pathogen-likeness, immune activation, and prevention (Table 3). The first concept was pathogen-likeness: A vaccine contains something that is part of or is shaped like the pathogen, including the pathogen itself or a weakened or modified version of it. We also gave credit for pathogen-likeness if a student said that the “disease” was in the vaccine rather than a pathogen. We chose to do that, because although the pathogen and the disease it causes are different, it was extremely common for students to use the words “sickness,” “disease,” or “illness” when they actually meant the pathogen that caused the disease. For example, this EBM student seems to use the terms “disease” and “cell” (referring to the pathogen) interchangeably: “The vaccine contains the dead disease in it … and when given, your white blood cells build an immunity to the dead cell. So if the disease become[s] present later, your body will have already dealt with the cell, and know how to fight it.” Thus, if we had excluded mentions of “disease”-like terms, we would have greatly underestimated the prevalence of pathogen-likeness. Here are examples of the parts of student responses that were scored as expressing pathogen-likeness: “I have a basic understanding that a vaccine is a weaker strain of a virus” (EBM student), “A vaccine prevents disease by putting the smallest amount of the illness in your body” (NPH student), and “A vaccine works by injecting the virus or sickness into ones [sic] body” (PH student).
The second concept was immune activation: A vaccine stimulates an active immune response. Students could get a point for immune activation if they said that the immune system actively responded or reacted to the vaccine, such as this NPH student did: “The vaccine you’re getting a dose of a certain disease for your immune system to fight off,” or if they mentioned effects on specific parts of the immune system, such as creating antibodies or stimulating immune cells, as this EBM student did: “Your body then reacts to the foreign disease and produce[s] antibody and more white blood cells to combat the invader in your body.” Because this item specifically concerned creating an active immune response, responses that only said that vaccines had more passive effects like “strengthening” or “building” the immune system were not awarded this point. We observed that some students used these more passive or general ideas about “strengthening the immune system” to describe the effect of vitamins and healthy living. Thus, an EBM student who wrote, “The vaccines exposes [sic] the patient to a little of the disease and also something to strengthen the immune system,” might be thinking that the vaccine simply acts like a vitamin does to generically boost immune function instead of believing that the immune system actively responds to the antigens in the vaccine.
The third concept was prevention: Vaccines can prevent disease caused by a pathogen or lessen the disease’s severity in the future. Two student examples were: “[The vaccine] allows the immune system to build up a tolerance to the disease, therefore making it a low chance to actually contract the disease.” (NPH student) and “They put [the vaccine] to help your body know this is who you might encounter again later on. So it makes it easier for your body to fight it off” (PH student).
A basic component score was calculated by counting how many of the three basic components were present in a given response. For example, the following response contains all three components of basic knowledge:
Inject an inactive virus that was made in the lab into the body so that the body can build an immunity against the virus. The body will attack the inactive virus building antibodies against it. So, if it encounters the same virus again it can recognize the virus and attack it before it can invade your cells and replicate.—ABM student
The mention of “inactive virus” counts for pathogen-likeness, the mention of “building antibodies against it” counts for immune activation, and the discussion of attacking the virus if it encounters it again counts for prevention. Thus, the basic component score for this response was 3. In contrast, this next response only gets a 2:
A vaccine is an artificial or GMO version for a disease or virus that is introduced to one’s body in a controlled manner so that the body learns to recognize the disease & is able to fight it of [sic] instead of allowing you to catch the illness naturally which could possible [sic] be more threatening.—EBM student
The mention of “version of a disease or virus” counts for pathogen-likeness, and the discussion of learning to recognize the disease to fight it off instead of catching it counts for prevention. However, because the response only mentioned “the body” instead of the immune system or specific components thereof, it did not get immune activation.
As can be seen in several of the examples presented, many responses contained at least one component of basic knowledge yet also contained inaccurate ideas. Therefore, we decided to score accuracy separately from the components of basic knowledge. Responses scored as “accurate” had no scientifically inaccurate ideas, defined as ideas that are inconsistent with what is said by authoritative sources on vaccines. “Inaccurate” responses had one or more inaccurate ideas. As an example, this student response displays all three components of basic knowledge but also implies that vaccines contain the original, unaltered pathogen, which is an inaccuracy:
A vaccine is a concentrated virus injected into your body to better prepare your immune system. When the vaccine is injected, your white blood cells and T-cells are learning how to identify and fight that virus. This information is used in the future when the same/similar diseases are detected.—EBM student
In contrast, this next student response had none of the components of basic knowledge but also had no inaccurate information:
Vaccines could be injected, the doctor usually clears the area of skin with alcohol swab and then injects the shot with vaccine fluid that is inside the shot.—NPH student
To capture knowledge of basic components and accuracy together, we calculated a combined knowledge score, which was the basic component score plus a 1 if the student was fully accurate.
To see how well users could use the rubric, we conducted an interrater reliability analysis. Two researchers each independently coded a new random sample of at least 10% of the student responses, blind to population type, for each component of basic knowledge and for accuracy. All qualitative coding reached 90% or above consensus between the trained coders (Table 3). We also calculated Cohen’s kappa, another measure of interrater reliability that considers agreement by chance, for each component of basic knowledge and accuracy. It was 0.90 for pathogen-likeness, 0.85 for immune activation, 0.76 for prevention, and 0.69 for accuracy (Table 3). Cohen’s kappas of 0.80 or higher are generally considered to be in “excellent” agreement, and those between 0.60 and 0.80 are considered to be in “substantial” agreement (Landis and Koch, 1977).
Student Knowledge of How Vaccines Work
Using our rubric, we found that students at each of the levels examined had a wide range of knowledge about how vaccines work (Figure 1). While BF had a median combined knowledge score of 4 (out of 4); ABMs had a median score of 3; and EBMs, PH, and NPH students had a median score of 2. However, the scores for all student groups ranged from 0 to 4 (Figure 1). To see whether presumed level of biology expertise corresponded to vaccine knowledge, we compared combined knowledge scores as well as the scores for each component of basic knowledge and accuracy between all levels of presumed biology expertise (NPH, PH, EBM, ABM, and BF; Figure 1). Overall, there were significant differences between groups for combined knowledge scores (p < 0.001), each component of basic knowledge (p = 0.003 or less), and accuracy (p < 0.001). Effect size was moderate for combined knowledge score (epsilon-squared = 0.103), weak for pathogen-likeness (Cramér’s V = 0.20), moderate for immune activation (Cramér’s V = 0.23), weak for prevention (Cramér’s V = 0.16), and moderate for accuracy (Cramér’s V = 0.29; Rea and Parker, 1992). All groups of students (NPH, PH, EBM, ABM) had, on average, significantly lower combined knowledge scores than faculty (p < 0.001 by Dunn’s test; Figure 1A). ABM (n = 104) had significantly greater combined knowledge scores (p = 0.002 or less by Dunn’s test) than all other student groups (Figure 1A). When looking at the individual components of basic knowledge, it seems that the ABM advantage in combined knowledge score was primarily accounted for by pathogen-likeness and accuracy (Figure 1, B and E). ABM were more likely to know that vaccines are pathogen-like than any other student group (p = 0.019 or less; Figure 1B), and they were more likely to be fully accurate (p = 0.037 or less; Figure 1E). Effect sizes were in the weak to moderate range (Cramér’s V from 0.17 to 0.24). However, ABM did not differ from any other student group in knowing immune activation (Figure 1C), and they only differed from EBM in knowing prevention (p = 0.018, Cramér’s V 0.17, a weak association; Figure 1D). Surprisingly, though, we found no significant difference in combined knowledge score, any component of basic knowledge, or accuracy between EBM (n = 237), PH (n = 111), and NPH (n = 183; Figure 1, A–E). These findings suggest that EBM and PH do not necessarily know more than NPH about vaccines.
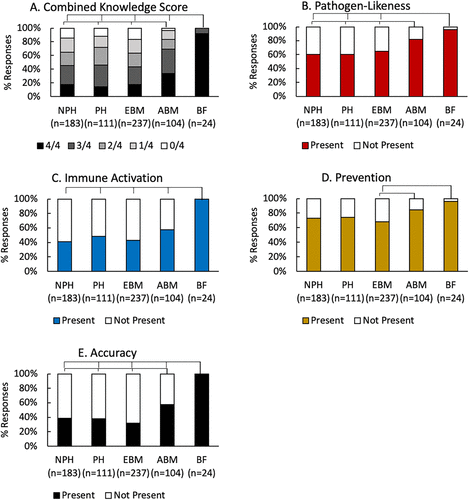
FIGURE 1. Scores for combined knowledge (A), pathogen-likeness (B), immune activation (C), prevention (D), and accuracy (E) across levels of expertise. Lines indicate Bonferroni- adjusted p < 0.05 by Dunn’s test for post hoc comparisons (A) or chi-square analysis (B–E).
How Other Factors Influence Understanding of How Vaccines Work
We sought to understand the relation between a student’s knowledge of how vaccines work and various possible contributing factors, such as whether a student has taken course work that covers vaccination, whether a student is confident in their knowledge of vaccines, years in college, and demographic characteristics, including gender, race/ethnicity, and college-going status. Therefore, we created a linear regression model that correlated these factors with the combined knowledge score. For more details on how we performed the modeling, please refer to the section Linear and Logistic Regression Modeling to Relate Student Factors to Knowledge and Accuracy. The initial model, before model selection, is included as Supplemental Table S2. The final model, discussed here, only includes the variables that most significantly correlate with our measures of knowledge.
The final model for combined knowledge score is shown in Table 4. Students who self-reported taking course work that addressed vaccines had, on average, a combined knowledge score that was 0.66 higher out of a possible total of 4 (p < 0.001), while students who reported being confident in their knowledge had a score that was 0.52 higher (p < 0.001). No other variables were significantly correlated with having a higher combined knowledge score.
Variable | Estimate (β) | SE | t value | p value |
---|---|---|---|---|
Intercept | 1.557 | 0.086 | 18.123 | <0.001 |
Course work | 0.659 | 0.114 | 5.791 | <0.001 |
Confidence | 0.520 | 0.116 | 4.472 | <0.001 |
Because confidence in one’s knowledge was significantly associated with the combined knowledge score, we wanted to further understand the relationship between confidence and knowledge. An examination of the distribution of combined knowledge scores shows that 30% (23 of 77) of the students whose responses contained none of the three components of basic knowledge were nonetheless confident in their knowledge (Figure 2A). Thus, not all students who reported confidence in their knowledge were actually knowledgeable. Similarly, 37% (143 of 345) of the people whose responses contained at least one inaccuracy claimed to be confident in their knowledge (Figure 2B). To better understand the relation between confidence and accuracy, we created a logistic regression model for accuracy with the same potential independent variables as our model for combined knowledge score. The initial, full model is available in Supplemental Table S3. The final model, after model selection, is shown in Table 5. “Confidence” did not end up being included as a significant variable. The only variables in the final model for accuracy were course work and years in college. Having taken course work was associated with a 1.98 times increase in the likelihood of having an accurate response (p < 0.001). For years in college, the odds of having an accurate response increased by 1.21 times for each additional year (beyond freshman year) a student was in college (p = 0.048).

FIGURE 2. Percentage of students who are confident or not confident in their vaccine knowledge by combined knowledge score (A) and accuracy (B).
Variable | Estimate (β) | SE | z value | p value | Odds ratio |
---|---|---|---|---|---|
Intercept | −1.107 | 0.164 | −6.746 | <0.001 | 0.33 |
Course work | 0.684 | 0.192 | 3.572 | <0.001 | 1.98 |
Years in College | 0.188 | 0.094 | 1.981 | 0.048 | 1.21 |
Inaccurate Ideas about How Vaccines Work
Because 62% (392/635) of student responses contained inaccurate ideas about how vaccines work (Figure 1E), often multiple ones, we wanted to categorize the most common inaccurate ideas and understand how they related to the components of basic knowledge. Using thematic analysis, we initially identified six proposed inaccurate ideas that were present in 10% or more of the inaccurate student responses.
We then asked five IF to review our descriptions of these inaccurate ideas to verify that they were truly scientifically inaccurate. Our initial set of proposed inaccurate ideas is listed in Supplemental Table S4. For one of the proposed inaccurate ideas, “Vaccines work through administering a small or non-harmful dose of the pathogen,” two IF believed it might not be inaccurate. Therefore, we discarded this proposed idea. For the remaining five proposed inaccurate ideas, we made small clarifications to the descriptions of these ideas in response to IF comments. For example, for the proposed idea “Vaccines are injected directly into the skin or bloodstream (as opposed to into a muscle),” one objection was that needles do go into the skin, and another objection was that some of the injected antigen may travel through the blood to reach an antigen-presenting cell. Therefore, we changed our description of this idea to “Vaccines are mainly injected directly into the bloodstream.” For another example, “A vaccine is a treatment or a cure,” two IF cited the rabies vaccines and cancer vaccines as counterexamples. Thus, we modified the wording to “Vaccines are primarily a treatment or cure.” The final versions of the common inaccurate ideas are listed in Table 6.
Misconceptiona | Raw agreement | Cohen’s kappab | % All student responses (n = 635) |
---|---|---|---|
A vaccine contains the pathogen in an unmodified form. “It’s a cocktail of different things, including whatever virus its meant to protect you from, gets injected into bloodstream so your immune system can begin to build antibodies against it.”—EBM student | 88% | 0.75 | 28% (n = 180) |
Vaccines are primarily a treatment or a cure. “It even helps to clean out an infection you might already have.”—EBM student | 92% | 0.67 | 11% (n = 67) |
A vaccine directly harms or fights the pathogen, not through the immune system. “A vaccine works by a doctor/nurse injecting fluid into your body. The fluid is the actual injection that fights off diseases.”—PH student | 95% | 0.70 | 6.9% (n = 44) |
Vaccines are mainly injected directly into the bloodstream. “A vaccine is injected through a vein which would mean that it goes through the inferior vena cava of the heart and eventually distributes from the aorta to the systemic circulation.”—ABM student | 92% | 0.58 | 6.9% (n = 44) |
A single vaccine provides immunity to all pathogens or diseases “Through there the body will take in the vaccine and is used to help take over the body so no other known disease can take over the body.”—EBM student | 92% | 0.25 | 6.8% (n = 43) |
The most common inaccurate idea, present in 28% (180 of 635) of all student responses, related to the composition of the vaccine: the idea that vaccines introduce an unmodified disease or virus to the body. An example student response was “A vaccine is basically a small amount of a certain virus or disease injected into your body.… And since it’s a small amount, your body should fight it off pretty fast” (ABM student).
Other inaccurate ideas concerned more general ideas of how vaccines protect against disease. Some 11% (67 of 635) of students thought that a vaccine is a treatment or cure given to a sick person, as highlighted in this response: “You can go to a doctor when you’re sick and they can give you a vaccine to cure your disease” (PH student). In addition, 7% (44 of 635) of students thought vaccines directly harm or fight the pathogen: “A vaccine works in ways where it fights off bacteria” (NPH student). Around 7% (43 of 635) of responses implied that a vaccine provides immunity to all pathogens or diseases, rather than a specific one, as shown here: “Vaccines work by helping your immune system fight off any disease you have or help prevent any from occurring. Without vaccines everyone would get sick, vaccines help with making sure you’re healthy and strong so you could survive” (EBM student).
Finally, 7% of students (44 of 635) thought vaccines are injected directly into the bloodstream, when in fact vaccines are usually injected into muscles. An example is shown here: “A vaccine works with a specified amount of medicine being placed into a shot and placing the needle into the arm around the area opposite of the elbow (forgot the name) and the vaccination being shot into the arm and covered to spread through the veins” (EBM student).
Next, we wanted to better understand how the common inaccurate ideas we found relate to the components of basic knowledge students have about how vaccines work. To do that, we first compared the basic component score of students who were or were not fully accurate. In this analysis, all students who had any inaccurate ideas related to vaccines or disease, including ideas that were not among the five common ones, were counted as having inaccurate responses. We found that students who had fully accurate statements were significantly more likely to have higher basic component scores (p < 0.001) by the Kruskal-Wallis test (Figure 3). The effect size as measured by epsilon-squared statistic was 0.079, a moderate correlation (Rea and Parker, 1992). We then looked at how stating particular inaccurate ideas was related to basic component scores. We ran a linear regression model for basic component scores with the presence or absence of the five most common inaccurate ideas as potential inputs. The initial, full model is included as Supplemental Table S5, and the final model after model selection is in Table 7. Unsurprisingly, students who stated that vaccines were treatments or cures, vaccines directly harm pathogens, or that a single vaccine could protect against all diseases had, on average, basic component scores that were between 0.73 to 1.00 points lower than students who did not have these ideas (p < 0.001 for all; Table 7). However, students who had the idea that vaccines use unmodified pathogens scored on average 0.55 points higher than others for their basic component score (p < 0.001; Table 7).
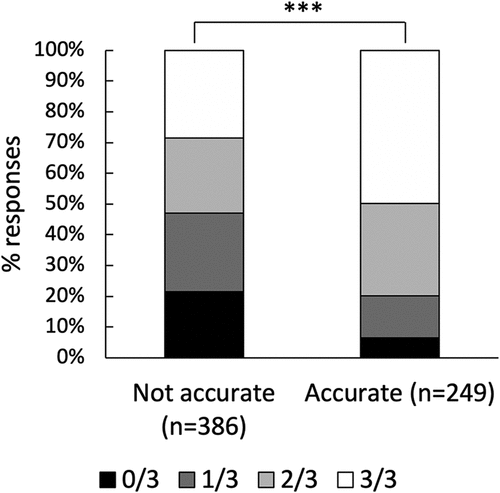
FIGURE 3. Basic component scores by accuracy. ***p < 0.001.
Variable | Estimate (β) | SE | t value | p value |
---|---|---|---|---|
Intercept | 1.911 | 0.048 | 39.621 | <0.001 |
Unmodified pathogen | 0.548 | 0.082 | 6.669 | <0.001 |
Vaccine is treatment | −1.001 | 0.127 | −7.859 | <0.001 |
Vaccine directly harms pathogen | −0.925 | 0.153 | −6.033 | <0.001 |
Single vaccine for all diseases | −0.728 | 0.143 | −5.092 | <0.001 |
Because the knowledge-in-pieces perspective of constructivism implies that learning occurs in bits and pieces, we hypothesized that specific inaccurate ideas may correlate with knowing or not knowing specific components of basic knowledge. Thus, we ran three logistic regression models with the presence or absence of the five common inaccurate ideas as inputs, one model for each of the three basic knowledge components. The initial, full models are in Supplemental Table S6, and the final models after model selection are in Table 8. Stating the inaccurate idea that vaccines use the unmodified pathogen was positively associated with knowing immune activation (odds ratio = 1.64, p = 0.009) and prevention (odds ratio = 1.70, p = 0.02; Table 8). (It was also strongly positively associated with knowing pathogen-likeness [odds ratio = 22.6, p < 0.001], because by definition, if someone thought that a vaccine contained the unmodified pathogen, then they knew it resembled the pathogen.) On the other hand, having the idea that vaccines largely cure or treat disease or that vaccines directly harm pathogens was associated with a significantly lower likelihood (p = 0.003 or lower) that a student would state the three basic knowledge components. Odds ratios ranged from 0.28 to 0.05, indicating roughly fourfold to 20-fold decreases in the probability that a student stating these ideas would demonstrate a given element of basic knowledge. Having the idea that a single vaccine is effective against all diseases was associated with a significantly lower likelihood of knowing that vaccines are pathogen-like (odds ratio = 0.15, p < 0.001) or that vaccines activate the immune system (odds ratio = 0.11, p < 0.001). Finally, the idea that vaccines are injected directly into the blood was not significantly correlated with knowing any of the components of basic knowledge.
Knowledge component | Variable | Estimate (β) | SE | z value | p value | Odds ratio |
---|---|---|---|---|---|---|
Pathogen-likeness | Intercept | 0.669 | 0.114 | 5.863 | <0.001 | 1.95 |
Unmodified pathogen | 3.116 | 0.472 | 6.598 | <0.001 | 22.6 | |
Vaccine is treatment | −2.281 | 0.424 | −5.385 | <0.001 | 0.10 | |
Vaccine directly harms pathogen | −2.340 | 0.597 | −3.920 | <0.001 | 0.10 | |
Single vaccine for all diseases | −1.911 | 0.418 | −4.568 | <0.001 | 0.15 | |
Immune activation | Intercept | 0.048 | 0.108 | 0.442 | 0.66 | 1.05 |
Unmodified pathogen | 0.496 | 0.189 | 2.626 | 0.009 | 1.64 | |
Vaccine is treatment | −2.625 | 0.608 | −4.320 | <0.001 | 0.07 | |
Vaccine directly harms pathogen | −3.025 | 1.029 | −2.939 | 0.003 | 0.05 | |
Single vaccine for all diseases | −2.163 | 0.540 | −4.009 | <0.001 | 0.11 | |
Prevention | Intercept | 1.169 | 0.120 | 9.717 | <0.001 | 3.22 |
Unmodified pathogen | 0.530 | 0.235 | 2.258 | 0.02 | 1.70 | |
Vaccine is treatment | −1.288 | 0.295 | −4.373 | <0.001 | 0.28 | |
Vaccine directly harms pathogen | −1.465 | 0.370 | −3.956 | <0.001 | 0.23 |
DISCUSSION
Here, we present a large-scale study on how college students believe vaccines work. Using our IF-validated rubric, we found that quite a bit of variability among college students with respect to knowledge about how vaccines work. We also found that many college students have a mix of accurate and inaccurate information about this topic and that formal education and confidence was positively associated with having more basic knowledge about vaccines.
Creation of a Rubric for Understanding How a Vaccine Works
From our analysis of BF responses and comments from IF, we believe that the rubric’s three points of “basic knowledge” (pathogen-likeness, immune activation, and prevention) capture the most important parts of the biology of how a vaccine works. Although our IF gave ideas for additional aspects of vaccine biology that could be included in the rubric, none were cited by more than two IF, and none were present in a supermajority of the BF responses, suggesting that these additional ideas were less fundamental. (However, these additional ideas could be the basis for a future rubric appropriate for more advanced students.) These three points of basic knowledge are also in accord with what interview studies have found are the major parts of adult mental models of how vaccines work. Jee et al. (2015) found that, when asked about vaccines, immunology experts and middle school teachers talked about the structure of the vaccine (corresponding to our basic knowledge component of pathogen-likeness), the function of the vaccine (corresponding to our component of prevention), and how vaccines work through stimulating the immune system (corresponding to our component of immune activation). Similarly, Downs et al. (2008) found that adults were generally aware that vaccines are protective against disease (prevention) and that some could further identify the mechanism by which vaccines provided that protection (pathogen-likeness and immune activation). Given that nearly all other published methods of measuring what people know about vaccines focus on other aspects of vaccine knowledge such as knowledge of vaccine recommendations (e.g., Mellon et al., 2014), knowledge about vaccine-preventable diseases (e.g., Salmon et al., 2005), or belief in inaccurate ideas (e.g., Zingg and Siegrist, 2012), our assessment and rubric might be a useful additional tool for probing what college students and other adults know about the basic biology of how vaccines work.
What Students Know and Do Not Know about Vaccines
Our study found that there is quite a bit of variability among college students with respect to their levels of basic knowledge about how vaccines work. We found that, although a majority of students stated that vaccines mimic pathogens and that vaccines provide protection against future infection, far fewer students knew that vaccines activate the immune system (Figure 1.) This finding agrees with the smaller interview study of Downs et al. (2008), who found that a substantial portion of the people interviewed knew that vaccines protected against disease but could not describe anything about the mechanism by which vaccines prevent disease.
At the same time, we found that many students at all levels had inaccuracies in their responses (Figure 1). This finding agrees with the results of nationwide survey studies that showed that, among the general public, there is a high rate of agreement with inaccurate ideas about vaccines (Stecula et al., 2020b). We found that the most common inaccurate ideas were that vaccines contain the pathogen in an unmodified form, vaccines are primarily a treatment or a cure, vaccines directly harm or fight the pathogen, vaccines are injected directly into the blood, and a single vaccine can provide immunity to all diseases (Table 6).
Curiously, three of the five most common inaccurate ideas we found (vaccines are a treatment or cure, vaccines directly harm the pathogen, and a single vaccine protects against all diseases) still imply that vaccines are effective against disease. Although one previous study did note that middle-school students often believed that vaccines fought infection directly (Jee et al., 2015), there is not much other documentation of these particular inaccurate ideas in the literature. In contrast, the one inaccurate idea we found that implies that vaccines might be harmful (vaccines contain the unmodified pathogen) has been documented several times in previous research (Benjamin and Bahr, 2016; Gidengil et al., 2019). We speculate this is because most studies of inaccurate ideas about vaccines, including one whose scale is widely used by other studies to measure vaccine knowledge (Zingg and Siegrist, 2012), focus solely on ideas that imply that vaccines are ineffective, unnecessary, or possibly dangerous. Thus, our study adds to our knowledge of inaccurate ideas about vaccines by revealing ideas held by students who may well believe vaccines are effective but have a less accurate understanding of how they work inside the body.
The Relationship between Basic Knowledge and Inaccurate Ideas
Although students who avoided inaccurate statements were significantly more likely to have more basic knowledge of how vaccines work, many students who cited all three components of basic knowledge nevertheless had one or more inaccurate ideas in their responses (Figure 3). This mix of accurate and inaccurate ideas is what one might expect from the perspective of the constructivist theory of knowledge: Students construct their own meaning out of what they have learned, which means that both accurate and inaccurate ideas can emerge.
The clearest example of how accurate and inaccurate ideas can coexist comes from analysis of the most common inaccurate idea we found, that the vaccine contains the pathogen it protects against in an unmodified form. This idea is inaccurate, because in reality, vaccines either use a weakened or killed version of the disease-causing organism or mimic the pathogen by introducing a component of it into the body (NIAID, 2011). At the same time, though, it is important to acknowledge that the students who believed this idea clearly know something about vaccines: By definition, they know that a vaccine resembles the pathogen it protects against (as a pathogen resembles itself). In addition, these students were also slightly more likely to know that vaccines activate an immune response and are used to prevent disease (Table 8), giving them an overall higher average basic component score (Table 7). One could speculate that these students learned how the body responds to natural infection and hypothesized that vaccines cause the same effects: induction of an active immune response followed by protection against future infection. That understanding would indeed give these students an understanding that is scientifically accurate in many respects.
On the other hand, we did not find that all inaccurate ideas were positively associated with higher basic knowledge. The ideas that vaccines are treatments or cures, vaccines directly harm the pathogen, and one vaccine can prevent all diseases were all associated with lower combined knowledge scores and a lower probability of knowing at least two out of the three specific components of basic knowledge (Tables 7 and 8). The presence of these ideas may signal that students misunderstand the basic principles by which vaccines work. The remaining common inaccurate idea, that vaccines are injected directly into blood instead of into a muscle or other tissue, was not significantly associated with basic component score or the presence of any individual component of basic knowledge (Tables 7 and 8). Students might have confused vaccine injections with blood draws or believed that any injection goes into a blood vessel. Nevertheless, our results suggest that this idea may neither interfere with nor contribute to learning how vaccines work.
Effect of Education on Understanding How Vaccines Work
We found that, in general, there was a positive effect of formal education on knowledge of how vaccines work. We found that ABM were more likely than other students to have higher combined knowledge scores, know each individual component of basic knowledge, and produce fully accurate responses (Figure 1). However, there was little difference between the other three student groups: EBM, PH, and NPH (Figure 1). Therefore, one cannot always assume that students who want to major in biology know more about biology or that students who want to major in pre–health disciplines know more about healthcare than other students. This finding agrees with evidence from national guidelines, previous studies, and curricula associated with standardized exams that suggest that immunology knowledge is usually reserved for more advanced college biology course work (Gregory et al., 2011; NGSS Lead States, 2013; College Board, 2020). It also agrees with some studies on other biology topics that found that EBM do not have more accurate biology knowledge than nonmajors (Coley and Tanner, 2015; Richard et al., 2017), although it is important to note that other studies disagree (Sundberg et al., 1994; Knight and Smith, 2010).
In addition, we found that, in our sample as a whole, self-report of taking a previous class that addressed vaccines was positively associated with knowledge. Having previous course work was significantly associated with higher basic knowledge scores, a higher probability of knowing each individual component, and a higher probability of having a fully accurate response (Tables 4 and 5). While educators hope that their students will remember and use what they are taught, not all studies have found a correlation between being taught a certain subject and better knowledge of that subject in different contexts. For example, studies have found that many ABM who have taken course work in evolution and genetics do not use their knowledge of natural selection to accurately describe antibiotic resistance (Richard et al., 2017) or their molecular biology knowledge to describe a GMO (Potter et al., 2017). However, there is evidence that students who are taught material in a real-world context are better able to apply their knowledge in that context (Danielson and Tanner, 2017). Perhaps instructors tend to teach about the immunology behind vaccines in the real-world context of protecting against disease.
Together, these findings imply that students may not be exposed to or learn much from informal sources about vaccines and that formal education is helpful in giving them basic knowledge about how vaccines work.
Relationship between Confidence and Knowledge in Understanding How Vaccines Work
We found that students’ self-declared confidence about their levels of knowledge of vaccines had a complicated relation with their levels of actual knowledge. Previous research on cognitive biases suggests that people are poor judges of their own knowledge (Kruger and Dunning, 1999). For example, in members of the general public, perceived understanding of genetically modified foods is negatively correlated with objective knowledge of genetics (Fernbach et al., 2019). In contrast, we found a positive correlation between students’ confidence in their knowledge and their actual levels of basic knowledge of how vaccines work (Table 4). Thus, with respect to vaccines, students may have some metacognitive awareness of their levels of knowledge—in other words, they may be somewhat aware of what they do or do not know. However, the findings are different when considering accuracy instead of basic knowledge: We found no significant correlation between student confidence and probability of having a fully accurate response (Table 5). It is unclear why there is a lack of correlation. Perhaps students with less knowledge struggle to identify inaccurate ideas, leading those students to be incorrectly confident about what they know. Or, perhaps students who are confident do not feel the need to examine their own knowledge for inaccuracies. The nature of the inaccuracy may matter as well. A study among the general public found that people who thought that vaccines cause autism were more likely to (incorrectly) declare that they knew as much about the causes of autism as doctors do (Motta et al., 2018); in other words, it found a negative correlation between confidence and accuracy. It may be that some inaccurate ideas are more deeply held or have more support from the Internet or other sources, which allows the students who hold them to be more confident, whereas other inaccurate ideas are more like guesses and reflect a lower level of confidence. Overall, our findings about students’ confidence and its relation to basic knowledge and accuracy echoes research that distinguishes being “uninformed” and being “misinformed” and shows that recognizing “misinformation” about scientific topics can be very difficult (Scheufele and Krause, 2019).
Implications for Vaccine Education
Overall, our study suggests that college students have incomplete knowledge about how vaccines work and that teaching them may improve their knowledge. It also implies that students who have inaccurate ideas may not be aware of it, so it may be necessary to directly confront those ideas.
Our study’s analysis of inaccurate ideas has further implications for teaching about vaccines. Research in the learning sciences supports the constructivist notion that inaccurate ideas can arise from genuine attempts by students to understand the world around them and that instructors can use these ideas as a “bridge” to more complete and accurate knowledge (Maskiewicz and Lineback, 2017). In our study, that was particularly evident with the most common inaccurate idea, that vaccines contain an unmodified version of the pathogen. It is important to challenge this idea, both because it is scientifically inaccurate and because it could lead to a belief that a vaccine could give a person the disease; for instance, an earlier study found many college students fear that the influenza vaccine will make them sick with the flu (Benjamin and Bahr, 2016). However, it is also useful to build on the fact that students with this idea nevertheless do have some accurate ideas about how vaccines work. Instructors could use the accurate idea of pathogen-likeness that is inherent in this idea by teaching students that, although vaccines resemble the actual pathogen and activate the immune system like an actual pathogen, they are really a modified version or a part of the pathogen that cannot make us sick with the disease. With the other inaccurate ideas that vaccines are a treatment or cure, that vaccines directly harm or fight the pathogen, and that a single vaccine can prevent all diseases, instructors could build on the idea that vaccines are effective against disease. However, these ideas were associated with lower levels of basic knowledge, so instructors who have students with these ideas might want to spend more time developing students’ models of the mechanism by which vaccines achieve that protection.
While our findings about student vaccine knowledge may be specific to our context, our assessment and rubric could be useful for instructors to quickly and simply uncover the extent to which their particular students know about the biology behind how a vaccine works. Research in education supports the idea that instructors who understand the prior knowledge of their students can teach more effectively (Chen et al., 2020; Sadler et al., 2013). The assessment itself consists of one free-response question, and respondents only need 3–5 minutes to handwrite their answer. This protocol is similar to how an instructor might administer a “minute paper,” a short active-learning writing activity in class (Miller and Tanner, 2017). The rubric only has four items: three items for basic knowledge and one for overall accuracy. Yet, despite the simplicity of this assessment and rubric, we were able to use it to differentiate, on average, between people with expert knowledge (faculty), people with moderate knowledge (advanced biology students), and presumed novices (EBM and non–biology majors; Figure 1).
Instructors can use the knowledge gained from this rubric to guide their instruction. For example, if an instructor does a minute paper using our prompt and finds that many of the students mention prevention but do not mention pathogen-likeness or immune activation, the instructor can have confidence that the students generally believe that vaccines prevent illness and focus teaching on the mechanism by which vaccines work. If many students do not even mention prevention, the instructor may instead focus on providing data and using strategies to engage students in demonstrating that vaccines work. Finally, if an instructor finds that students have a decent grasp of the three basic knowledge components but have many inaccurate ideas, the instructor can choose to provide only a cursory review of how vaccines work and instead challenge students more deeply about their inaccurate ideas. Because our assessment and rubric are simple, not tied to any particular course, and appropriate for use with students with a wide range of expertise levels, we believe that they could be appropriate for a wide range of instructors who want to know what their students know about how a vaccine works.
Limitations
Because our rubric requires users to make binary determinations, such as whether a component of basic knowledge is present or not, we could not use it to make finer distinctions about exactly how knowledgeable or correct students were. For example, it is more precise and accurate to say that a vaccine contains “a component of a pathogen” than “a piece of the virus” (not all vaccines work against a virus), which in turn is more precise and correct than saying that a vaccine contains “a part of the disease” (the pathogen is different from the disease it provokes). It is also more accurate and complete to say that a vaccine contains “a modified version of or a component of a virus or bacteria” than that it contains “a modified virus.” Nevertheless, we counted all of these phrases as expressing the basic knowledge component of pathogen-likeness. This simplification made the rubric easier to use and to some extent allowed us to separate students’ vaccine knowledge from their English writing skills. However, we do believe that there are probably differences in the knowledge level of students who express themselves in these various ways, which might be revealed in studies that use different methods such as interviews.
Similarly, we made the “accuracy” level binary, which meant that we could not make finer distinctions about “how wrong” students were. Students with very inaccurate ideas were grouped together with students who only had partially inaccurate ideas, and students who had many inaccurate ideas were grouped together with students who just had one. We chose this approach, because its simplicity eliminated many issues. We did not want to make judgments about which inaccuracies were “worse” than others or which inaccuracies were “complete” as opposed to “partial.” Also, we found it hard to determine whether various inaccuracies were truly distinct from each other, which would have been necessary for any approach that involved tabulating the number of inaccurate ideas per response. A tabulation approach would also unduly favor students who had fewer inaccurate ideas purely because they wrote less. Nevertheless, we acknowledge it would be good for future work to find a more nuanced way to approach measuring how wrong a student’s response is.
Another limitation is that our assessment used a very general question, so students may not have chosen to share every idea they have about how vaccines work. Because ideas can be accurate or inaccurate, omitting ideas would tend to decrease scores for basic knowledge but increase scores for accuracy. However, we tried to mitigate against this possibility by encouraging students to fully share their ideas and giving students enough time to finish writing. It is also likely that students wrote the ideas they felt were the most important for addressing the prompt, so our assessment probably still yielded valuable information about what ideas were most salient to students.
Also, our study only collected data from one university at a particular point in time. Although our study population was diverse in terms of race, gender, and first-generation college-going status (Table 2), it may not have been diverse in terms of other factors that may affect ideas about vaccines, such as political orientation (Baumgaertner et al., 2018). It would be useful for future work on vaccine knowledge to collect information on these other factors. It is also important to note that the data collection occurred before the COVID-19 pandemic, which meant that vaccines were much less salient for most college students. If our study were repeated today, when society is actively debating vaccines, it is not clear what the results would be. On the one hand, students might know more, because vaccines are more relevant for them and they are seeking this information out; on the other hand, they might have more inaccurate ideas, because misinformation about COVID-19 vaccines has spread widely on the Internet (Kricorian, Civen, and Equils, 2021).
In addition, our study focused on vaccines in general, and our results might not hold true for specific vaccines. One example is the influenza vaccine, which has a much lower uptake rate than traditional childhood vaccines (Benjamin and Bahr, 2016). In our study, less than 10% of students expressed the idea that vaccines might give someone the disease. However, a study among college students about the influenza vaccine found that at least 33% of students in general and nearly 50% of students who did not get a flu shot believed that the influenza vaccine could give the recipient the flu (Benjamin and Bahr, 2016). The level of knowledge college students have about vaccines might also be different with respect to vaccines that make use of newer technologies such as the mRNA COVID-19 vaccines (Kricorian et al., 2021).
Finally, our study does not address how students use their knowledge of vaccines to make decisions in the real world, such as whether to vaccinate themselves or their family members against particular diseases. The most widely used frameworks for predicting health behaviors, such as the health belief model, posit that the relation between scientific knowledge and health behaviors is indirect (Janz and Becker, 1984). Even though people who do not accept vaccines tend to hold inaccurate ideas about them (Zingg and Siegrist, 2012; Reich, 2016), studies have found that giving people scientifically accurate information about vaccines has little or sometimes even a negative effect on vaccine acceptance (Dubé et al., 2015; Nyhan et al., 2014; Pluviano et al., 2017). These studies, however, did not define vaccine knowledge in terms of understanding the biology behind how vaccines work. The one study that did probe parents’ understanding of how vaccines work implied that having a weak or nonexistent understanding of how vaccines work may leave parents, even those favorable to vaccination, vulnerable to misinformation (Downs et al., 2008). It would be interesting to see the extent to which knowledge of how vaccines work using a tool like ours correlates with vaccine acceptance and behaviors.
CONCLUSIONS
Here, we present a simple assessment and rubric that can help instructors better understand what their college biology students know about the basics of how vaccines work and uncover inaccurate ideas students have about this process. We used this rubric to reveal what a large sample of college biology students know and do not know about how vaccines work. We found that many college biology students have a weak understanding of the basics of how vaccines produce immunity, particularly students who are not in advanced biology courses, and that there are numerous common inaccurate ideas about what vaccines are made of and how they work. Consistent with constructivism, most inaccurate ideas were associated with lower levels of basic knowledge. However, the inaccurate idea that vaccines contain the unmodified pathogen was associated with higher levels of basic knowledge. Overall, these findings add to our understanding of what college students are learning about vaccines and suggest strategies for addressing certain inaccurate ideas.
ACKNOWLEDGMENTS
We would like to thank Kimberly Tanner and the SEPAL laboratory for their early support of the planning and data-collection phases of this project and Lyndsey Hightower for additional data collection. We also thank Sarah Bissonnette, Shannon Seidel, Keying Deng, SEPAL members, and the UC San Diego Biology Education community for stimulating conversations, comments, and feedback about this project and article. We are also very grateful to our faculty and student participants and to the instructors who allowed us access to their classes.