What Are They Thinking? Automated Analysis of Student Writing about Acid–Base Chemistry in Introductory Biology
Abstract
Students’ writing can provide better insight into their thinking than can multiple-choice questions. However, resource constraints often prevent faculty from using writing assessments in large undergraduate science courses. We investigated the use of computer software to analyze student writing and to uncover student ideas about chemistry in an introductory biology course. Students were asked to predict acid–base behavior of biological functional groups and to explain their answers. Student explanations were rated by two independent raters. Responses were also analyzed using SPSS Text Analysis for Surveys and a custom library of science-related terms and lexical categories relevant to the assessment item. These analyses revealed conceptual connections made by students, student difficulties explaining these topics, and the heterogeneity of student ideas. We validated the lexical analysis by correlating student interviews with the lexical analysis. We used discriminant analysis to create classification functions that identified seven key lexical categories that predict expert scoring (interrater reliability with experts = 0.899). This study suggests that computerized lexical analysis may be useful for automatically categorizing large numbers of student open-ended responses. Lexical analysis provides instructors unique insights into student thinking and a whole-class perspective that are difficult to obtain from multiple-choice questions or reading individual responses.
INTRODUCTION
The National Research Council recently outlined a vision for the future of biological science education that includes recommendations for sound foundations in chemistry and integration of chemistry with biology education, as appropriate (National Academy of Sciences, 2003, 2010). This call for integrating physical sciences and mathematics with biology is echoed by college instructors (Bialek and Botstein, 2004). Although there have been efforts at the undergraduate level to integrate chemistry and biology during laboratory exercises and attempts at combining chemistry and biology courses and/or curricula (Wolfson et al., 1998; Barreto, 2000; Schwartz and Serie, 2001; Reingold, 2004; Wenzel, 2006; Abdella et al., 2011), there have been few studies of students’ ability to transfer knowledge and/or skills between these specific disciplines. However, there are some reports that indicate potential problems students have with concepts such as free energy, oxidation–reduction, and equilibrium (Schwartz and Serie, 2001). Other studies have followed student learning progression in college chemistry (Claesgens et al., 2009). If curricular integration is to occur, it is critical to understand the concepts and principles that students have difficulty transferring between disciplines.
In contrast to the lack of research in students’ learning of biological chemistry, there has been considerable investigation into students’ difficulties with and mental models of acid–base chemistry in chemistry education (Lin and Chiu, 2007). Of particular importance for learning in biological chemistry is the finding that students often associate the chemical symbols of H and OH with acids and bases respectively, regardless of the chemical reaction (Furio-Mas et al., 2007), or consider the number of hydrogens in a chemical formula when determining acidic or basic properties of a molecule (Lin and Chiu, 2007). Students also have difficulty differentiating between atomic and ionic forms of compounds (Nakhleh, 1994; Furio-Mas et al., 2007). Some of the difficulties with understanding acid–base chemistry may be a result of a poor understanding of the particulate nature of matter (Nakhleh, 1994). Other reports show that students have difficulty producing accurate representations of chemical structures, without which it is nearly impossible to predict behavior of large biomolecules (Cooper et al., 2010). Indeed, students often find explanations of chemical phenomena difficult, despite instruction, and have difficultly performing tasks such as balancing chemical equations (for a review, see Krajick, 1991).
Based on the assumption that understanding biological systems requires some knowledge of chemical principles, many institutions set general chemistry as a prerequisite for general biology. However, in our introductory biology course, we have encountered students that still fail to apply basic chemistry concepts and have difficulty offering chemical explanations for biological phenomena (Wilson et al., 2006; Parker et al., 2007). If integration of biology and chemistry instruction is to happen, these fundamental problems need to be addressed. To address these problems, we need assessments that not only reveal students’ conceptions in these areas, but also provide insight into students’ thinking and possible mental models.
Constructed-response assessments (also called open-response assessments) require students to respond to questions using their own language and have the potential to reveal misunderstandings and conceptual barriers in ways that closed-response (e.g., multiple-choice) questions do not (Birenbaum and Tatsouka, 1987). In addition, students may have multiple ideas about a single topic, some of which may include both right and wrong concepts (or multiple wrong concepts). These heterogeneous ideas cannot be uncovered by multiple-choice instruments alone, but may be better revealed by constructed-response assessments (Nehm and Schonfeld, 2008). In addition, for students to undergo conceptual change (revising prior knowledge in light of new knowledge), it is important for both students and instructors to understand the different ideas that make up students’ prior knowledge (Chi, 2008). This level of detail is rarely revealed by closed-form assessments. However, resource constraints in large-enrollment, undergraduate, introductory science courses often discourage the use of constructed-response questions. Advances in technology provide opportunities for evaluating large numbers of student written responses quickly using a variety of computer techniques (Haudek et al., 2011; Nehm and Haertig, 2012; Nehm et al., 2012).
In previous work, we used lexical analysis software to analyze students’ constructed responses on topics relating to energy metabolism in an introductory biology class (Moscarella et al., 2008). We have extended this work to investigate students’ understanding of basic chemistry that may be related to conceptual problems students have in cellular biology. Further, we use this approach to reveal students’ heterogeneous ideas about chemical concepts in biology. These computerized lexical techniques are validated by using statistical classification functions to predict human scoring of student responses.
RESEARCH QUESTIONS
This report is centered on three research questions: 1) How well does lexical analysis of student writing uncover student mental models? We address this question by linking the lexical analysis of student explanations about biological groups’ acid–base behavior to literature on models and common concepts in biology and chemistry. 2) How accurately does student writing reflect their thinking about these ideas? To answer this, we compare students’ written responses with verbal explanations in face-to-face interviews. 3) How well does lexical analysis reflect human expert ratings of student writing? To investigate this, we generated statistical classification functions that use the lexical categories to predict human expert ratings.
METHODS
Course Description
This study was conducted in an introductory cellular and molecular biology class at a large public university during the Fall 2008, 2010, and 2011 and Spring 2009 semesters. Students enrolled in the course were mostly sophomores or juniors who had completed about 56 credit hours on average. More than 50% of the students were natural sciences majors, including biology and pre–health professional programs, and 50–60% of students enrolled were female. To enroll in the biology course, students must have completed a one-semester general chemistry course. More detailed demographic information is given in Table 1.
Fall 2008 | Spring 2009 | Fall 2010 | Fall 2011 | Interviews | ||||||
---|---|---|---|---|---|---|---|---|---|---|
Mean | SD | Mean | SD | Mean | SD | Mean | SD | Mean | SD | |
GPA | 2.96 | 0.58 | 3.02 | 0.6 | 2.99 | 0.58 | 3.06 | 0.69 | 3.13 | 0.64 |
Course grade | 2.25 | 1.23 | 2.27 | 1.12 | 2.28 | 1.13 | 2.29 | 1.15 | 2.63 | 1.06 |
Credit hours passed | 56.7 | 22.5 | 56 | 24.2 | 58.9 | 23.3 | 56.3 | 24.7 | 61.4 | 23.4 |
Percent female enrollment | 64.3 | 59.4 | 59.8 | 50.4 | 50 | |||||
Ethnicity (%) | ||||||||||
Asian | 4.2 | 5.5 | 4.2 | 6.7 | 0 | |||||
Black | 3.7 | 4.4 | 8.4 | 4.7 | 0 | |||||
Hispanic | 3.7 | 2 | 2.5 | 3.6 | 0 | |||||
White | 82.2 | 80.1 | 80.7 | 79.7 | 100 | |||||
Major (%) | ||||||||||
Pre-med/nursing/vet, etc. | 28.5 | 30.8 | 29.6 | 27.9 | 25 | |||||
Biology/human biology | 15.7 | 21.5 | 21.2 | 22.8 | 12.5 | |||||
Other natural science | 13.2 | 13.2 | 16.7 | 14.1 | 25 | |||||
Social sciences | 5.4 | 9.8 | 8.6 | 9.1 | 12.5 | |||||
Agriculture and animal sciences | 4.5 | 5.2 | 4.7 | 5.6 | 12.5 | |||||
Engineering | 3.6 | 10.6 | 10.1 | 10.9 | 0 | |||||
Chemistry and physical sciences | 2 | 1.3 | 3.7 | 2.6 | 12.5 |
Item under Investigation
Students were asked to complete an online homework question set for credit (approximately 0.01% of course grade per question). Students were awarded full credit for any genuine effort at responding, whether correct or not. The question set was designed to address topics common to the general chemistry and introductory biology courses. The assignment was given in the second half of the term, so students had seen the topics in both chemistry and biology courses. For this report, we focus on one question, further subdivided into two parts:
1a. Consider two small, identical, organic molecules in the cytoplasm of a cell, one with a hydroxyl group (-OH) and the other with an amino group (-NH2). Which of these small molecules (either, both, or neither) is most likely to have an impact on the cytoplasmic pH?
Compound with amino group [correct response]
Compound with hydroxyl group
Both
Neither
1b. Explain your answer for the above question.
Responses were collected in an online course-management system for analysis. The question was presented to students as a single Web page with multiple response boxes. A total of 1191 students answered the multiple-choice question (question 1a) and 1172 wrote something in the response box for an explanation (question 1b).
Scoring
Two expert raters with expertise in chemistry and biology independently evaluated all explanations from students who chose the correct multiple-choice response. Evaluations were made using the following rubric (an example student response is given for each scoring level as an example):
Level 1: Correct description of basic nature of amino group (e.g., “Amino group acts as a base as it can pick up an H+ from solution.”) | |||||
Level 2: Partially correct explanation (e.g., “The amino group acts as a base. It will lower the pH of the cytoplasm toward base.”) | |||||
Level 3: Totally incorrect or irrelevant explanation (e.g., “Amino group has two H atoms it may give up, but hydroxyl has only one OH molecule it may give up.”) |
For the analyses described here, only responses from students who selected the correct response to question 1a and attempted a written explanation were analyzed. Five students selected the correct multiple-choice answer but either did not answer question 1b or answered only with “I don't know.” These five responses were not subjected to further analysis.
Computerized Lexical Analysis
For lexical analysis of students’ constructed responses to question 1b, we used SPSS Text Analytics for Surveys version 4.0 (STAS; SPSS, 2010a), which allows the analysis of open-ended questions through the classification of responses into categories. The responses are imported into the software, which extracts key terms that are used to categorize the responses. To create categories for this project, we combined both linguistic and frequency algorithms in the STAS software. These computer-generated categories were further refined by using an expert answer. We took advantage of a custom library with biological terms we previously built in the software (Moscarella et al., 2008). Once the data were categorized, the individual responses and the associated categorized data were exported for subsequent data analysis. Responses that contained multiple relevant terms could reside in more than one category.
A category (denoted by italics in this text) in lexical analysis is the name of a group of similar terms and synonyms defined by either the software and/or the user. Categories can also contain functions using Boolean operators (“and,” “or,” “not”) that allow the user to specify very particular phrases or combinations of terms to include in that category.
SPSS Statistical Analysis
We used SPSS version 19 (SPSS, 2010b) to perform paired t tests, analysis of variance (ANOVA), nonparametric correlations, and discriminant analysis (Spicer, 2005). To test for word count and category count differences between interviewed students’ written and verbal explanation, we performed a paired t test with an alpha level of p = 0.05. To test for word count differences between responses in the three scoring levels, we performed a one-way ANOVA with an alpha level of p = 0.05. To examine correlations between written explanations and interviews, we calculated Kendall's tau-B for lexical categories of these two groups. For discriminant analysis, we used all the lexical categories generated from the lexical project as independent variables and expert rating as the dependent variable. Only responses on which the experts agreed after reconciliation were used for scoring. The discriminant analysis used a stepwise-forward, Wilks’ method with an Fin of 3.84 and Fout of 2.71. We used group sizes for prior probabilities and leave-one-out cross-validation.
Student Interviews
To confirm that student written responses accurately reflected student thinking on the question, eight students who correctly answered question 1a and completed the homework assignment from Fall 2011 were selected for interviews. These interviews occurred about 6 weeks after completion of the semester in which the students submitted their written responses. There were four female and four male students who were enrolled in various majors and had taken a similar number of credit hours. Students who participated in the interview had a slightly higher course grade than average for students enrolled in the course in Fall 2011 (Table 1).
At the beginning of each interview, each student was shown the question and asked to choose an answer and give an explanation. Student explanations were followed up with probes to clarify the meaning of terms used and to provide a more detailed description of students’ understanding of acid and base behavior in solution. Initial student explanations (before introduction of probes) were subjected to computerized lexical analysis as described in Computerized Lexical Analysis. In addition, the full interviews were qualitatively analyzed.
RESULTS
In previous semesters of this introductory biology course, students had difficulty predicting effects of functional groups and/or relating functional groups to acid–base behavior. Responses to the multiple-choice question (1a) show a similar problem (Table 2). Only one-third of the students could correctly identify an amino group as affecting the pH of a cell, while nearly half the students selected the incorrect response of hydroxyl.
Choice | Number | % |
---|---|---|
A. Aminoa | 399 | 33.5 |
B. Hydroxyl | 578 | 48.5 |
C. Both | 163 | 13.7 |
D. Neither | 51 | 4.3 |
To explore student thinking and difficulties about this topic, we prompted students to explain their multiple-choice answers. These written explanations were subjected to lexical analysis and concurrently scored by experts. Students’ written explanations were validated via face-to-face interviews. Finally, the variables generated from lexical analysis and expert rating were used in a statistical prediction model.
Lexical Analysis of Student Writing
Lexical analysis of all written responses produced 27 lexical categories. These categories included relevant terms that help reveal students’ understanding of acid–base chemistry and cellular biology (ionization, solution, hydrogen, base, etc.). Distributions of categories assigned to students’ responses are shown in Figure 1. Note that amino is the most frequently assigned category in all levels. This is likely because these are answers from students who chose the amino response (A) in question 1a. Our experience is that students often repeat key terms from the question in their constructed responses. The distributions of the categories hydroxyl and hydrogen are very similar between student responses scored in levels 1 and 3, while markedly different for level 2. While students scored in levels 1 and 3 use these terms with similar frequency, as we will see later, the co-occurrence of these categories with other categories provides important insight into the differences in responses across levels. On the other hand, the categories accept hydrogen, acid, base, and raise pH have very different distributions among the levels. All of the remaining categories appear in fewer than 20% of the student responses in both levels.
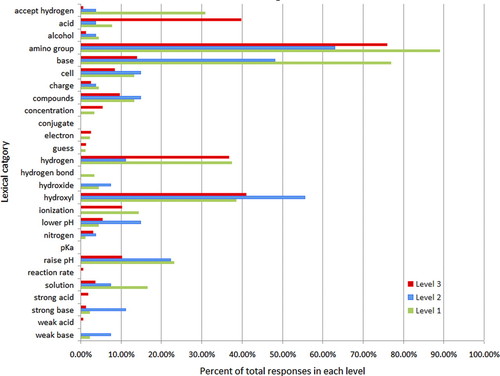
Figure 1. Percent of responses in a given rubric scoring level in each lexical category. Responses scored as level 1 by both experts (n = 91) are indicated by the green bar, those scored as level 2 (n = 27) by the blue bar, and those scored as level 3 (n = 166) by the red bar. Note that any one response may be in multiple categories and that the categories conjugate and pKa were created during the lexical analysis of all student responses, but did not appear in any of the responses in which the experts agreed on scoring.
Nearly all students used multiple ideas in their explanations. These multiple ideas can be identified and categorized by lexical analysis. For example, the following response was classified into the categories: amino, cell, hydroxyl, base, and raise pH:
#101: The amino group would have a greater effect on the pH of the cytoplasm rather then the hydroxyl group. Since the amino group is a base, it would make the ph of the cytoplasm increase [sic].
Whereas this response was categorized as: amino, compound, cell, and reaction rate:
#102: The amino group can break down compounds faster and can therefore change the pH of the cytoplasm.
Note that these explanations contain multiple concepts and share two lexical categories. However, it is the combination of these concepts that contributes to the evaluation by experts (see Expert Ratings of Written Responses) and overall scientific “correctness.” These multiple ideas cannot be revealed by multiple-choice questions, as both students selected the same answer in the multiple-choice question (i.e., that the amino group would affect the pH).
Lexical and Qualitative Analysis of Student Interviews
To determine whether students’ short written responses accurately reflected their thinking, face-to-face student interviews were used to validate their written homework responses. At the beginning of each interview, students were shown question 1a and 1b again and were asked to choose an answer and then explain their choice. Of the eight students interviewed that chose the correct answer to 1a on the homework, five students chose the correct answer in the interview as well. After the students responded to question 1a, they were asked to explain their choice (question 1b). These explanations were subjected to word count and lexical analysis along with their explanations from their homework (Table 3). Although students’ explanations in the interview setting included significantly more words, there was no significant difference in the number of lexical categories into which each response was classified. Both these measures were tested using a paired t test, with a significant difference (p < 0.05) noted only for word count between students’ written and interview explanations. This suggests that most of the additional words in the interview explanation hold little scientific meaning. We then performed nonparametric correlation tests of the lexical categories across each students’ written and interview responses (Table 4). Although a total of 27 lexical categories were created for all student responses (see Figure 1), only 16 of these categories were used by the subset of students chosen for interviews (see Table 4). Of these 16 categories, two of the categories were used in a student's written response but not in the interview (alcohol and solution) and three of the categories appeared in the interview and not the written explanation (cell, electron, and guess). However, there are high correlations (r = 0.745–1.0; p < 0.05) for seven of the lexical categories (Table 4), showing that students used the ideas consistently in their written and verbal explanations.
Written | Interview | |||
---|---|---|---|---|
Mean | SD | Mean | SD | |
Word count | 37.38* | 14.44 | 63.63* | 36.47 |
Lexical categories counted | 4.88 | 1.89 | 4.88 | 2.03 |
Lexical category | Kendall's tau-B coefficienta |
---|---|
Accept hydrogen | 1.000** |
Acid | 0.745* |
Alcohol | N/A |
Amino | 0.149 |
Base | 0.467 |
Cell | N/A |
Compounds | 0.333 |
Electron | N/A |
Guess | N/A |
Hydrogen | 1.000** |
Hydroxyl | 0.488 |
Ionization | 1.000** |
Lower pH | 1.000** |
Raise pH | 1.000** |
Solution | N/A |
Strong base | 1.000** |
In addition to lexical analysis of correct multiple-choice responses, student interviews were qualitatively analyzed to validate student thinking about acid–base chemistry revealed in written responses. Definitions and explanations used during the interviews closely align with student definitions and explanations in response to question 1b during the homework assignment. For example, one interviewed student was consistent in his use of the number of hydrogens on a molecule to determine the pH:
#122 Written: I choose the amino group containing more hydrogens. The more hydrogens in that is available [sic].
#122 Interview: My guess would be the amino group which would be NH2 has another or an additional hydrogen making it more acidic.
Another common explanation we observed in student writing describes a base or acid based on a pH range:
#103 Written: Bases cause pH to increase above 7. NH2 is a base and therefore a molecule with an amine will have a base >7.
Because we noticed this type of definition of a base, we further prompted students during interviews to define an acid and base. Two interviewed students used this model to define a base and an acid:
#118 Interview: A base is something with a pH of seven or greater, I guess.
Interviewer: And how would you define an acid?
#118 Interview: Less than seven.
#119 Interview: Um, well I know basic just means there is a higher pH.
It is important to note that these definitions represent previously observed mental models of acids and bases and their behavior that have been reported in the literature (see Lin and Chiu, 2007).
Interviews also supported our observations about student writing regarding the difficulty students had distinguishing between two forms of OH, the hydroxyl molecular form (R-OH) and the hydroxide ion form (OH−). During the interviews, we prompted students to distinguish between the two forms. While two of the eight students interviewed could readily distinguish between these two forms, the other six students either could not distinguish between them or needed further cues to do so. Four students made the distinction only after the cue “ion” was given to describe hydroxide by the interviewer.
Expert Ratings of Written Responses
For question 1b, a total of 323 responses that provided an explanation (and chose the correct answer to question 1a) were independently scored with a three-level rubric (see Methods) by two experts. Analysis of the raters’ scoring of the student responses showed very good interrater agreement. The Cronbach's alpha was 0.961 and the single-measure intraclass correlation was 0.926 (p < 0.000). The two raters were in absolute agreement on 284 of the 323 responses (see Table 5). For example, expert raters put written response #101 into level 1 and #102 into level 3 (examples shown above). Only student responses scored at the same level by both raters were used for word count, discriminant analysis, and web diagram generation.
Level 1 | Level 2 | Level 3 | Total |
---|---|---|---|
91 | 27 | 166 | 284 |
To investigate whether answer length was related to the expert ratings, we compared word counts for written responses in the three expert scoring levels using a one-way ANOVA. There were no significant differences across the three levels.
Level 2 Responses
It is important to note here that level 2 had the fewest agreed-upon responses. Of the 323 scored responses, only 27 were agreed upon as being level 2 by both raters. Twenty-five responses were scored as level 1 by one rater and level 2 by the other; 14 were scored as level 3 by one rater and level 2 by the other. This disagreement in scoring is in part due to the fact that responses in level 2 should be “partially correct.” One difficulty involved in human scoring using such a holistic rubric is what exactly constitutes “partially.” Even though the raters agreed on a rubric and trained on a subset of student responses, the exact interpretation of the rubric level was left up to each rater. For instance, at what level does a response become too “incorrect” and move into level 3, or how much “incorrectness” is tolerated in an otherwise perfectly correct response? Of the non–agreed-upon scores, all responses that were in disagreement between experts had a rating of level 2 from one of the experts. Therefore, the requirement that only responses for which raters agreed reduced the number of responses in level 2 more than responses in levels 1 or 3.
However, because level 2 represents “partially correct” understanding, more of these students have mixed models of acid–base behavior. Among the 27 agreed-upon responses that were placed into level 2, we identified subsets of responses grouped by a defining characteristic in the explanation (terms/phrases included in more than four responses). One characteristic noted in level 2 responses was that students correctly described hydroxyl group chemistry but did not address or only briefly addressed amino group chemistry. In fact, discussion of hydroxyls was more common in level 2 responses than in level 1 or 3 responses (see Figure 1). However, with explanations that only focus on hydroxyl groups, it is difficult or impossible to know what a student understands about amino group chemistry. A different characteristic of another subset of level 2 responses was classifying amino groups as strong bases (note strong base in Figure 1). These students may have given an otherwise reasonable explanation, but their classification of an amino functional group as a strong base led to their responses being rated as level 2. Still, these students demonstrate some understanding of acid–base behavior, but do not appear to understand the strong/weak classification. Other observed patterns in level 2 responses include assigning a pH to a compound and describing amino groups as amino acids.
However, due to the low numbers of agreed-upon responses in level 2, and the difficulty in drawing conclusions about responses in level 2, because of the required heterogeneity in the response (“partially correct”), we restrict our analyses and discussion to responses in levels 1 or 3 for the remainder of this report.
Discriminant Analysis
To test the utility of the lexical classifications, we used the lexical categories of each student's answer as independent variables in a discriminant analysis (Spicer, 2005), with the expert classification of the student answers as the dependent variable. Discriminant analysis is similar to regression in that it attempts to create linear functions that maximize the prediction of the dependent variable. However, discriminant analysis is used for categorical, rather than interval, dependent variables. For this analysis, we have a series of binary independent variables (presence or absence in a lexical category) that are combined in a linear function to maximize separation on the categorical dependent variables (expert rating). In this analysis, we use a two-category dependent variable (levels 1 and 3) that produces a single linear discriminant function. Discriminant analysis analyzes the covariance between independent variables, or whether the variables change together or not. Because of this, it is not the values of independent variables but the relationships among them that is critical in identifying key independent variables in the discriminant functions.
Like regression analysis, discriminant analysis can be implemented in a stepwise fashion. Stepwise discriminant analysis selected seven categories for prediction (see Table 6). The resulting discriminant function had good classification accuracy (Wilks’ lambda = 0.416, chi-square = 220.569, df = 7, p < 0.000). The group centroids (the multivariate means) for the two groups were 1.594 for level 1 and −0.874 for level 3. Table 6 shows the resulting standardized canonical discriminant function coefficients for each of the categories on the discriminant function (these coefficients are similar to beta weights in a regression analysis).
Category name | Coefficient |
---|---|
Accept hydrogen | 0.604 |
Acid | −0.433 |
Amino group | 0.200 |
Base | 0.799 |
Hydrogen | −0.326 |
Hydroxyl | −0.177 |
Raise pH | 0.228 |
For question 1b, we note that the largest (absolute values) coefficients are base, accept hydrogen (a property of a base), and acid (a negative coefficient; see Table 6). The large positive values of base and accept hydrogen are in good agreement with the scoring rubric, as it would be difficult for a student to achieve a high score without addressing the fact that amino functional groups behave as bases. These values move an individual toward the level 1 centroid (1.594). The large negative value of acid may be due to the fact that a number of students expressed the incorrect idea that amino groups existed only as amino acids (i.e., covalently linked to a carboxylic acid). Such an incorrect idea would obviously result in a lower score on the scoring rubric and may account for part of the strong negative value of acid in the analysis, which moves these individuals toward the level 3 centroid (−0.874).
It is also important to define the difference between the categories hydrogen and accept hydrogen, in that they give dramatically different coefficients; accept hydrogen is positive and has almost twice the weight of the negative coefficient for hydrogen. The category hydrogen is a more general category designed to include all responses that use the term “hydrogen” in any manner. The category accept hydrogen is a subset of responses in the hydrogen category. The accept hydrogen category only contains responses that include the term “hydrogen” and the terms “accept” or “pick up” (or variants thereof). The distance between the coefficients for these two categories exemplify the granularity of the lexical analysis and the ability of the classification functions to distinguish between levels.
To test the validity of discriminant analysis, we used a leave-one-out cross-validation classification in which each case is classified by the function derived from all cases other than that case. For this part of the analysis, we used all three rubric levels as dependent variables, which generated two discriminant functions. These two functions were used to predict level membership for each of the 323 responses that had been classified by the raters, whether they had agreed on the scoring of the response or not. Using all three rating levels for the computer prediction functions more closely aligns with the expert rating task. We then used the computer-predicted rating as another independent rater and calculated interrater reliability (IRR) measure among the two human experts and the computer prediction. The average intraclass correlation (Cronbach's alpha) between the three ratings was 0.899, showing exceptional IRR between the human experts and the statistical prediction. The single-measure intraclass correlation of 0.749 is a more conservative test of IRR and accounts for the error variance of each of the raters but is still above generally accepted IRR values (Shrout and Fleiss, 1979).
Web Diagrams
Discriminant analysis uses the covariance between independent variables (in this case, lexical categories) to generate the scoring functions. Another way to visualize the covariance is by using web diagrams. In these web diagrams, categories are represented by nodes, and lines connecting nodes represent the responses that contain those two categories. Figure 2 shows web diagrams of responses placed into levels 1 and 3 (Figure 2, A and B, respectively). For these web diagrams, we focused on the seven categories chosen as important in creating the discriminant function (see Table 6). In these diagrams, the node size represents the number of responses in the rubric level placed in that category. Therefore, smaller nodes represent less frequent ideas in the responses. These are the same frequencies shown in Figure 1. Lines between nodes represent responses that are shared between two categories. The thickness of the line connecting the two nodes represents the number of responses shared between the two categories. In addition, the type of line used (solid, dashed, dotted) represents the percentage of the responses in the smaller node shared with the larger node. For example, there is a solid line connecting hydrogen to amino in level 1 responses (Figure 2A). This means that more than 75% of the responses in hydrogen (because it is the smaller node) are also in the category amino. In contrast, the dotted line connecting accept hydrogen to hydroxyl shows that between 25 and 50% of responses categorized as accept hydrogen are also shared with the category hydroxyl (Figure 2A). No connection between nodes is shown if fewer than 25% of responses from the smaller node are shared between categories (see raise pH and base in Figure 2B).
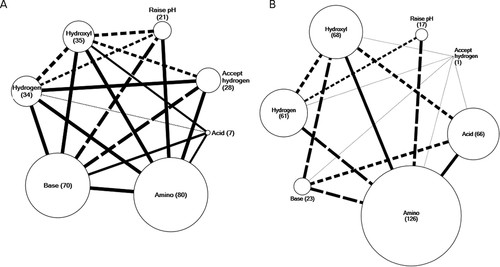
Figure 2. Web diagrams for responses in the eight categories used by the discriminant function. (A) Responses scored as level 1 by experts (n = 91); (B) responses scored as level 3 by experts (n = 166). Node size is proportional to the number of responses in that level contained in the category. A line connecting two nodes represents shared responses between the two categories. Line width reflects the number of responses shared by the two categories. Line type reflects what percentage of the smaller node is shared with the larger node. Solid line, 75–100% shared; dashed line, 50–74% shared; dotted line, 25–49% shared. If fewer than 25% of the responses in the smaller node are not shared with other categories, there is no line representing that connection.
Comparing the two web diagrams of level 1 and level 3 responses (Figure 2, A and B, respectively), we notice a few key differences. First, we see a drastic difference in node size for several categories: accept hydrogen, base, and acid. The larger proportion of responses in the acid node for level 3 responses is related to an incorrect idea regarding amino groups, in which they only exist in amino acid formation, as noted above. Both accept hydrogen and base are smaller nodes in level 3 compared with level 1, showing that more level 1 responses use these ideas in their explanations.
The other key difference represented in the web diagrams is the difference in connectivity between nodes. Level 1 responses show a complex pattern of multiple ideas, while level 3 responses show a less connected network of nodes. In addition to there being fewer lines (connections) in the level 3 responses, there are also fewer solid lines connecting nodes. This represents a smaller percentage of students using multiple ideas (as measured by these seven categories) in their responses. It is interesting to note the changes in the node hydrogen between levels 1 and 3. This node has relatively the same percentage of responses in levels 1 and 3. However, its connections differ drastically, as level 3 has fewer connections to base and accept hydrogen. This means that simply talking about hydrogen in a response is not indicative of a level 1 or 3 response, but the relationships to other ideas with hydrogen are critical for determining “correctness” of a response.
These diagrams help visualize the heterogeneity of student responses. Nearly all responses contained multiple ideas (see Table 3), some of which are more “correct” or relevant than others. This heterogeneity is revealed in the changing connectivity of these node networks. These diagrams show which ideas were being used (node size) and connected (lines) in a student's explanation. This information is useful for informed and detailed instruction that addresses misconceptions.
DISCUSSION
One key conclusion from this report is that students in introductory biology lack the ability to explain basic chemistry in a biological context, even after taking general chemistry as a prerequisite for the biology course. One possible explanation for this issue is that students may be more familiar with the typical acid–base molecules shown in introductory chemistry courses (e.g., OH− as a strong base). However, these acid–base molecules are different from the weak bases and acids typically present in biological functional groups, and students may be less familiar with the biological compounds (e.g., carboxyl). Indeed, nearly all acid–base chemistry as it relates to biology involves weak acids and bases, buffers, and equilibriums near neutral pH (with notable exceptions). Our interview data suggest such a focus in chemistry courses on strong bases is further complicated by students’ inability to distinguish OH in its ionic form (OH−) from its molecular form (R-OH; Furio-Mas et al., 2007). In fact, it was anecdotal evidence that students have difficulty applying chemical principles in biological contexts that prompted this study. As it relates to instruction, faculty should not assume that acid–base chemistry and biological functional groups are easy extensions of general chemistry or that students have sufficient understanding of chemistry to apply these ideas in new contexts. In fact, an overwhelming majority of the students in this study appear to lack the ability to apply acid–base chemistry in biology.
Implications for Instruction
It appears that students readily use some terminology associated with acid–base chemistry, such as base, hydrogen, pH, and acid. However, fewer students describe the mechanism by which acids or bases act, which may contribute to the problem of students being unable to classify the behavior of a weak base. Taken together, these observations show students have learned some fundamental terminology but struggle as they are asked to apply this knowledge and predict behavior of compounds in new situations. Illuminating what students do know (and their common errors) is essential for well-designed instructional interventions.
Although it is not our purpose in this report to detail such an intervention, we can imagine a scenario in which an instructor assigns a question as reported here for homework after one class period and has the results of lexical analysis before the next class period. With such insight (e.g., few students attempt to describe the molecule in question as a weak base), an instructor may spend time revisiting what makes an acid or base “weak.” There are several simulations available that address the difference between weak and strong acids; these could be used during a demonstration or assigned to students to complete on their own as part of laboratory exercises or homework (for one example, see Lancaster et al., 2011). Alternatively, an instructor concerned about students’ inability to distinguish between molecular and ionic forms of OH could begin the next class period reviewing the differences between such compounds. A third approach may be to help students move from a chemical context into a biological context. A classroom discussion about strong bases in solution may be a good starting point, since students seem to have a better understanding of this system. However, the instructor may prompt students to discuss the pH ranges they would expect to see in biological systems and, therefore, what sorts of properties and behaviors of molecules are expected in organisms.
The results of our research suggest that computerized lexical analysis can be used successfully to categorize student open-ended responses. We do not recommend that these classification functions be used to assign grades to individual students (e.g., high-stakes testing). However, we believe it would be feasible, for example, to design a series of questions in an online homework set containing open-response questions. Computer-predicted scoring functions could be used to evaluate student responses in real time, and students could be directed to a next question based on whether their predicted score is “correct” or “incorrect” and could receive individualized formative feedback about their written response. For example, a student who explained that a base would lower the pH of the cytoplasm would be taken to a follow-up question, textbook section, or tutorial about determining pH based on H+ concentration. This would allow homework sets to be tailored to individual students and would give students more relevant practice in topics in which they demonstrate a lack of understanding.
Instructor Gain from Lexical Analysis
In this report, we have highlighted one problem in introductory biology regarding functional group acid–base behavior. Although this fundamental problem is detailed in chemistry education research, this report expands the boundaries of this problem into biology and biological molecules. Such confusion over acid–base principles should not be ignored or minimized based on the assumption that students “learned about that” in their chemistry course. We believe that this student misunderstanding requires attention in biology instruction. As well, a critical step in conceptual change is that students themselves must recognize differences between their current knowledge and new knowledge (Chi, 2008). By using the results of lexical analysis, an instructor can see the misconnections students make in their explanations and can use this information to better address students’ prior knowledge with instruction tailored to students’ ideas.
We have also shown that multiple-choice questions do not fully reflect student thinking on a topic. Although roughly one-third of the students are able to select the correct multiple-choice response, less than half of these “correct” students could even give a partially sound scientific explanation. Therefore, one option for obtaining more accurate feedback regarding student understanding is to couple a multiple-choice question with a constructed-response explanation that can be subjected to lexical analysis. Feedback obtained solely from multiple-choice questions should be viewed with caution, as these results may overestimate the number of students who understand a given topic.
Finally, there also exists the opportunity for interested instructors to repeat this analysis in their own classrooms to uncover what their students think. We encourage interested instructors and researchers to collect their own student data from constructed-response questions and to explore their students’ writing. Such work is revealing, as it shows which concepts students attempt to connect and what ideas are absent. Lexical analysis is one tool that is useful to analyze any large number of responses. If nothing else, such a look into student thinking may cause instructors to be reflective in their teaching. Please visit http://aacr.crcstl.msu.edu to obtain the lexical resources from this study to perform your own analysis.
Research into Student Thinking
Students often have heterogeneous ideas about scientific concepts. Revealing this heterogeneity is difficult in a closed-form (i.e., multiple-choice) assessment, in that students are forced to choose from a limited number of possibilities. Evaluation of constructed responses via lexical analysis has the potential to better reveal this heterogeneity, while allowing faculty to use constructed-response assessments in large courses. In this study, we have used lexical analysis as a research tool to provide a different view of student understanding. This is a critical first step if instructors are to address problems with students’ mastery of concepts and content. As we gain additional insight into student understanding and describe specific content problems, better instructional tools can be developed and evaluated. Our results suggest that nearly all students attempt to connect multiple concepts in their responses. Some of these connections are viewed as valid or “correct,” while a large number of other students show a mix of “correct” and “incorrect” connections. Although this recognition of connections and heterogeneity can be accomplished by reading and coding individual responses, lexical analysis is a reliable and efficient alternative to uncover this heterogeneity of ideas in large numbers of responses.
Although we present the lexical and statistical analysis of only one question here, our larger effort is devoted to building research tools and resources to allow the analysis of many questions rapidly. One argument against lexical analysis of student responses as reported here is that it would be quicker for an instructor to read all the responses than to build the necessary computer resources. However, as noted by Ha et al. (2011), lexical and statistical resources for computer-assisted scoring continue to be built for additional biological concepts. Once there is a critical mass of these resources, analysis of responses to any of the studied questions becomes trivial and can be accomplished in a matter of minutes. These developed resources can then be reused in related questions and science domains and in subsequent courses and semesters. Such a communal effort to build these computerized resources could lead to richer assessment of student learning in science (Haudek et al., 2011).
The methods we have employed allow examination of open responses from large numbers of students. An accurate whole-class picture is difficult to obtain by reading student submissions in the traditional way (i.e., one cannot see the forest for the trees), especially if only a sample is chosen. Even if we assume a statistically representative subsampling of responses, it is unlikely that a reader could accurately synthesize a picture of correct and incorrect ideas and their interconnections without time-consuming qualitative analysis. The summarization and visualization tools provided by the software system provide these functions for the complete class population and without the impossible burdens of manual analysis. Furthermore, it is only with this accurate and valid whole-class picture of students’ ideas and thinking that suitable instructional interventions can be designed.
Limitations
In our analysis of student explanations to the question, we were able to use discriminant analysis based on expert raters to create classification functions that resulted in intraclass correlation coefficients of 0.749. It should be noted that the computerized prediction has somewhat lower agreement with expert raters than do the two expert raters with each other. However, this is likely due to the low number of human agreed-upon responses for scoring level 2. These problems can be addressed by collecting additional student responses in which to train the software (especially level 2), which should result in classification functions that more closely agree with expert raters.
Computerized Analysis
We believe that computerized lexical analysis can be a useful tool for instructors in evaluating constructed-response assessments. The ability to see connections between ideas for an entire class at once is valuable for an instructor to assess class understanding and modify instruction when necessary. Statistical analysis can help identify key concepts in student explanations and/or misconceptions. Coupling lexical analysis with computer-determined classification functions of student responses opens the possibility for the critical evaluation of large numbers of constructed-response items, allowing application in large STEM classrooms.
The Automated Analysis of Constructed Response (AACR) research group is a network of STEM education researchers who are exploring these techniques in multiple disciplines and sharing assessment items, lexical resources, and statistical scoring models in order to continue to improve assessment quality. If you are interested in more information about our work or becoming a participant in this network, visit our group website (aacr.crcstl.msu.edu) or contact the corresponding author. Lexical resources created during this project (library and categories) are available for free download (after registering for a free user account) on our website.
FOOTNOTES
‡Present address: Department of Biological Science, Florida State University, Tallahassee, FL 32306-4295.
ACKNOWLEDGMENTS
We thank Ron Patterson and Ryan Sweeder for help with data collection and scoring student responses, respectively. We thank other members of the AACR research group and two anonymous reviewers for their thoughtful comments regarding the project and manuscript. This work was funded through grants from the National Science Foundation (NSF; DUE 0736952 and DUE 1022653). Any opinions, findings, and conclusions or recommendations expressed in this manuscript are those of the author(s) and do not necessarily reflect the views of the NSF.