All Groups Are Not Created Equal: Class-Based Learning Communities Enhance Exam Performance and Reduce Gaps
Abstract
Having students work in small groups has been shown to promote better student outcomes, even in large lecture classes. On an institutional scale, the creation of learning communities that span multiple classes has also been proven to improve student outcomes. Research has shown that both interventions can help narrow the performance gaps that are often observed for students from certain demographic backgrounds. However, both have their drawbacks. When used solely for discussions in class, students in small groups rarely continue working together outside lecture in an intentional manner. Grading based on group performance leads to the perception of unfairness due to “weak” or “poor” members or disproportionate workloads. Learning communities, on the other hand, require a fair amount of administrative support to implement. Here, we describe a novel course and incentive structure that allows individual instructors to create sustainable learning communities in their classes. This course structure is relatively easy to implement, requiring very few changes to existing courses, and is adaptable to a variety of contexts, including remote teaching. Finally, we show that such learning communities provide additional learning gains for students and demonstrate that these class-based learning communities help narrow performance gaps for minoritized students.
INTRODUCTION
The number of students earning STEM bachelor’s degrees has increased significantly, by 49–141% between 2000 and 2019 (NSF, 2022). Simultaneously, states have almost universally cut their spending on higher education, with an inflation-adjusted drop of almost $7 billion between 2008 and 2018 (Center on Budget and Policy Priorities, n.d.). As a result of this, and other associated factors, colleges and universities have been forced to increase their class sizes, especially in lower-division science, technology, engineering, and mathematics (STEM) gateway courses. Such large classes have several drawbacks, including creating anonymity, lacking discussion opportunities, and contributing to disproportionate outcomes for minoritized students (Gibbs et al., 1992; Treisman, 1992; Carbone and Greenberg, 1998). Studies have shown that larger class sizes can have a significant negative impact on student performance, which is especially pronounced for lower-performing students (Gleason, 1986; Paola et al., 2013). In addition, large classes might exacerbate the performance gaps observed across student demographic groups, especially performance gaps due to gender (Lindemann et al., 2016; Ballen et al., 2018). This might be due to the culture in large STEM lecture classes, which can increase feelings of alienation and discourage participation, particularly for women of color (Johnson, 2007). Earlier work has also documented how first-generation college students in large classes are less likely to form relationships with student peers and have low engagement with faculty (Terenzini et al., 1996; Pascarella et al., 2004). Even for students who perform well academically in large lecture classes, it appears that their knowledge decays quickly, and they can still hold deep misconceptions about concepts that were covered in the lectures. A successful approach to using a small learning group includes active involvement, heterogeneity, subject-specific activities, and individual participation (Cooper, 1995). Small-group activities can be introduced into large lecture classes without sacrificing content with the added benefit of enhancing comprehension, promoting student accountability, and reducing anonymity (Yazedjian and Kolkhorst, 2007). Small groups have also been found to enhance individual achievement, group task performance, and positive attitudes toward group work (Lou et al., 2001; Gaudet et al., 2010). They are also likely to benefit students with lower grades the most, while not sacrificing performance by students with higher grade point average (GPAs; Tessier, 2007). Moreover, using small groups has been shown to improve outcomes for students in minoritized groups. For example, one study found that implementing peer-led team learning, an approach involving small-group interactions, drastically reduced the disproportionate failure rate among underrepresented minority students in an introductory biology course (Snyder et al., 2016). Another study found that implementing small groups increased confidence in subject matter and completion rates among Hispanic, Native American, and female students (DePree, 1998).
In practice, the use of small groups in undergraduate education can be broadly classified into two categories. In most large lecture courses, instructors use small groups for discussions and activities in class (Lou et al., 2001; Kalaian and Kasim, 2014), with students generally self-selecting where they choose to sit in class, and therefore, who they end up interacting with. After each class period, there is generally no continued collaboration between group members, at least in any instructor-intentioned manner. For the purposes of this paper, we refer to such groups as temporary in-class groups (TIGRs). In another category of classes, for example, in laboratory classes, students are assigned (or self-select) into groups in order to complete a task (or set of tasks), and this requires them to collaborate outside regular class times. We refer to such groups as task-based small groups (TABs). Grades of individual students within a TIGR are generally not dependent on the performance of the other students in the group, while some aspect of the final grade in TABs is generally dependent upon the overall group performance. For example, a class might have a group of students submit a single paper that was worked on collaboratively, with everyone in the group receiving the same grade (Cooper, 1995). A similar approach by Summers and Volet (2010) included a group grade for a presentation, while Kinsella et al. (2017) used a group project that helped determine the final grade. These two categories are not pure, but exist on a spectrum. For example, some TIGR classes might use group exams to determine part of a student’s final grade in class (Giuliodori et al., 2009; Gilley and Clarkston, 2014), an approach that improved student performance in exams. Alternatively, lab classes might have students submit individual analyses of their experiments, with no points being taken off for experiments that did not yield the expected results, so that an individual student’s grade would ultimately not be impacted by whether the group worked well or not. While TIGRs are relatively easy to implement, they do not (at least intentionally) foster the formation of student learning groups outside lecture time, so students are not forming longer-term learning communities. Merely forming learning study groups outside the classroom, without explicit connections to in-class activities, also does not appear to be very useful in terms of improving student outcomes (Rybczynski and Schussler, 2011). On the other hand, while TABs encourage collaboration outside the classroom, they are often perceived negatively by students due to their incorporation of a group grade, with students believing that this penalizes the harder-working students in a group (Barfield, 2003; Labeouf et al., 2014; Aivaloglou and Meulen, 2021). Being able to have small groups working together in class, while also having them collaborate outside lecture times as a learning community, but in a way that does not include a group grade, would therefore allow one to leverage the advantages of both TIGRs and TABs without students opposing these practices because of perceptions of unfair grading practices. Our definitions of TIGRs and TABs have parallels with the distinctions between incorporating unstructured, unguided collaborative learning in a class, as opposed to developing truly cooperative learning environments (Johnson et al., 2014).
An emerging issue with the use of small groups—both TIGRs and TABs—is the psychological impact that they might have on some marginalized student populations. There has been documentation of racist and classist microaggressions by members of groups who are working together on a group project (Sarcedo et al., 2015). Cooper and Brownell (2016) found that increased interactions between students may increase negative experiences for LGBTQIA students. Active-learning techniques, including group discussions, can also increase anxiety among students (Cooper et al., 2018; Hood et al., 2021), leading to worse outcomes for these students. Thus, it is important to provide students with choices for how they want to engage and participate in the classroom if we want to make large classes inclusive. An excellent compendium of resources addressing various aspects of using group work in classes has been developed by Wilson et al. (2018) and delves into many of the issues associated with using groups in classes, including optimal group sizes, group composition, and examples of group activities.
Another approach, usually involving institutional programs (rather than course-based interventions) is the formation of learning communities in which students are typically put into small groups that take a series of classes together. Even when they are enrolled in larger classes, these small groups of students will meet in their cohorts, oftentimes with the support of a peer educator of some sort (Tinto, 2003, 1998). Beyond this general idea, these programs are implemented in a variety of ways—for example, as a first-year experience program to improve student outcomes for biology majors (Xu et al., 2018; Solanki et al., 2019)—with each adopted to the specifics of the institution, but in general, they have positive outcomes for students, including increased retention, higher academic achievement, and increased student engagement (Andrade, 2007). While beneficial, such institutional learning communities depend upon coordination among a number of different administrative personnel and are not a practical solution for the individual instructor. A system that could leverage the benefits of learning communities at the scale of a single class would allow for student collaborations outside lecture times and might reap benefits that are additive to what is already seen when small groups are used in large lecture classes.
All of this determines the constraints for developing more a more effective collaborative class environment. 1) Ideally, groups should be incentivized to work together on discussions and activities in class, while also persisting as learning communities outside lecture times. 2) Participation in groups should be voluntary, so that students who prefer working alone are not penalized. 3) The structure should be flexible and easy to implement so that faculty do not find adoption burdensome (Mulryan-Kyne, 2010). 4) No part of final grades should depend on the performance of the group as a whole. To create an incentive system that would promote student collaboration both in and outside the classroom, we turned to behavioral economics. This field attempts to integrate concepts from psychology, neuroscience, and sociology in order to illuminate how human beings make decisions. In short, behavioral economics posits that cognitive biases in our thinking often lead to us making decisions that are not in our best interest, in contrast to classical economics, which assumes that we always correctly balance short- and long-term gains to make the most logical decisions for ourselves (DellaVigna, 2009). This means that merely pointing out to students that working together is best for them in the long run will likely be insufficient incentive for them to engage in productive collaborations (McClure et al., 2004). Further, providing immediate monetary gains (or in the case of the classroom, grade points) might actually end up disincentivizing the very behavior that we seek to promote (Deci et al., 1999). For example, providing students with an immediate incentive (grades) to perform better in exams could make learning extrinsically motivated and lessen students’ inherent desire to learn. Instead, students begin to only want good grades, rather than wanting to attain mastery (Deci and Ryan, 2010). Therefore, part of the challenge was to develop an incentive system that would provide immediate gains for participating in small groups but that was not obviously tied to the grade that a student would ultimately receive in the class. Finally, while research into effective collaborations highlights the importance of intentionality in forming effective teams, students are rarely taught how to create effective groups and are not instructed in the importance of interdependence, communication, cooperation, or psychological safety in the creation of an inclusive, positive, and constructive learning environment for all members of the group (Billson, 1986; Huerta, 2007; Duhigg, 2016; Google re:Work Team, 2015).
In this paper, we describe a class structure that is easy to implement, in which students are voluntarily placed into academically equal groups and incentivized to work collaboratively on classroom discussions and activities and to continue their collaboration outside the classroom after being provided with instruction on how to be intentional about creating effective groups. We call these small groups “class learning communities” (CLCs), because they incorporate elements of institutional learning communities, combining those elements with small-group activities that are typically implemented in large lecture classes. By providing instruction and a framework for effective collaboration alongside gentle-nudge incentives that are not directly tied to class grades, we maximize the probability of students forming CLCs (as opposed to just working together in class) and working together outside class times. We also describe the changes to our midterms and final grade assignments that together provide the incentive for effective CLC building. Based on this class structure, we address two major questions. First, we examine whether having CLCs provides additional performance gains, compared with only having informal in-class discussion groups. Second, we determine whether this class structure can further close performance gaps for student populations, including women and minoritized students. Our results show that students who participate in CLCs perform better than those who only participate in in-class discussions and activities and that these CLCs can help reduce performance gaps for minoritized students.
METHODS
Students
The study was conducted in two sections of a lower-division biochemistry class in a large R01 university in the western United States in the Winter quarters of 2019 and 2020. The demographic composition of the classes is shown in Table 1 (for the 2020 class).
A | B | ||||||
---|---|---|---|---|---|---|---|
Demographic variable | CLC (n = 541) | Non-CLC (n = 202) | p value | CLC | Non-CLC | p value | |
Sex | Male | 25% | 42% | 0.070 | 3.303 | 3.224 | 0.0181 |
Female | 65% | 58% | |||||
Income | Not low income | 72% | 71% | 0.879 | |||
Low income | 28% | 29% | |||||
First generation status | Not first generation | 56% | 52% | 0.444 | |||
First generation | 44% | 48% | |||||
Transfer status | Not transfer student | 84% | 91% | 0.026 | |||
Transfer student | 16% | 9% | |||||
Minoritized student status | Not minoritized | 63% | 61% | 0.709 | |||
Minoritized | 37% | 39% |
Recruitment for Participation in CLCs
At the beginning of the quarter, all students took an online survey that asked whether they would like to participate in a small group of five to six people. The survey also included questions that made clear the commitment that was required if students chose to participate in small groups. Only students who answered “yes” to all questions were assigned to small groups. The survey used is included in supplementary materials. These small groups are what we refer to hereafter as CLCs (see Implementation of Small Groups).
Implementation of Small Groups
The students who chose to participate in small groups were randomly assigned to groups of five to six students such that the average cumulative GPA of each group was statistically indistinguishable from that of all other groups. For 2019, GPA data were pulled from the registrar by an individual who was not connected to the study. The same individual created the GPA-equal groups, so that the instructor had no knowledge of any individual student’s GPA during the quarter. In 2020, we asked for student GPAs in the recruitment survey, and used an R-Script (available in supplementary material) to create the groups.
Students in these groups (CLC) were encouraged to communicate and meet outside class using whatever methods they saw fit, creating a learning community for themselves. It was not mandatory for CLC students to meet with one another outside class nor did we monitor the frequency of their interactions. Students without groups (non-CLC) interacted randomly with other students in the classroom, and their activities in or outside lecture were not monitored.
Students in CLCs were also assigned seating in the lecture classroom, such that students from the same group were seated near one another and could collaborate on all in-class activities with their group. An example of the seating chart we used is available in the supplementary materials. Students in the class who did not participate in the small groups and chose to work on their own were allowed to sit in any nonassigned seat, and were free to complete in-class activities on their own or to collaborate with anyone in the class.
The first lecture of the class provided students with explicit instructions on how to form effective teams (slides used for this lecture are provided in the supplementary materials). Activities in the class included an icebreaker for students in the groups to introduce themselves and had each group create a plan on how they would incorporate best practices in their interactions so that they could form an effective team. In addition, after their first CLC meeting, students who were participating in CLCs were required to fill in a brief survey reflecting on their first meeting (the questions are available in supplementary materials). No alternate assignments were provided for the non-CLC students.
Course Structure
Figure 1 describes the overall course design for the classes. At the beginning of the quarter, students took a survey in which they decided whether they wanted to be a member of a small group. During lectures, group members (CLC) sat together at assigned tables, while students who had not been assigned a group (non-CLC) students were free to sit in other available seats. The class overall employed a flipped format, with content delivery occurring primarily by required student readings and pre-lecture assignments. In-class activities and lecture were used to apply concepts and help students develop higher-order thinking skills. Learning assistants were employed to promote student interactions during in-class discussions and activities and to normalize the difficulties that students encountered when learning how to analyze data and think critically. During lectures, students in CLCs discussed questions within their groups, whereas non-CLC students interacted with other non-CLC students sitting nearby.

FIGURE 1. Graphic illustrating the overall course structure and implementation of CLCs. Students who chose to participate in CLCs were assigned to small groups that were academically equivalent (see Methods). These groups worked together on activities in class and worked on exam questions within their CLCs (See Figure 2).
Exam Structure
Exams were modified to provide the short-term incentive for students to form meaningful learning communities (illustrated in Figure 2). Data that exam questions would be based on were released to students in the form of a worksheet, approximately 1 week before each midterm (pre-exam). These worksheets only contained descriptions of experiments and data, with no questions or explanations. Students (both CLC and non-CLC) were explicitly encouraged to work together to discuss the experiments, analyze the data, and figure out how to connect their analyses to concepts discussed in class. On the day of each midterm, students were first provided with the same data presented on the worksheets, but this time with the exam questions (phase 1). The questions were open ended, and students were allowed to discuss the data and answers for approximately two-thirds of the total exam time. During this time, students were also allowed the use of their notes and/or the Internet for research. Following this, students were asked to put away everything except the notes they had made during the first two-thirds of the exam time. It is important to note that non-CLC students were also allowed to work with one another during this time, with the only difference being that they had not explicitly formed a learning community during the class. Following this collaborative time, students were then provided with a multiple-choice exam with the same questions they had discussed in the first part of the exam (phase 2). Students worked independently on this part of the exam, and only this multiple-choice section was graded. To assess independent student performance, we also included a few questions in this section that students had not discussed in the first part, so that their performance on these questions would gauge their independent performance in the class without being complicated by help from their group. We refer to performance on these questions as the “independent question score.”

FIGURE 2. Graphic illustrating the structure of exams. Midterm exams were divided into three parts (in the schematic, the actual biological question being asked is irrelevant). Before the exam, worksheets containing only experimental descriptions and data allowed students to analyze the data in the context of concepts covered in class. On the day of the exam, students were first given open-ended questions to work on collaboratively (phase 1) and were then asked to answer these same questions independently in a multiple-choice format (phase 2). Students only saw the multiple-choice options in phase 2. A set of questions that students had not seen in phase 1 (independent questions) allowed us to assess students’ individual performance in the class.
Note that, in 2019, students were not given the questions in phase 2 that they had not previously discussed in phase 1, so we only present overall midterm performance data for 2019.
Final Grade Assignments—Long-Term Incentives for CLCs
To incentivize students to work with one another throughout the quarter in a productive manner, students who participated in CLCs were provided with the opportunity to get a “bonus” credit at the end of the class. At the start of the class, the average incoming cumulative GPA of each CLC was known. This is referred to as the group average university GPA, or GAUG. At the end of the class, students’ grades were calculated according to the posted policies, and then these grades were converted to a GPA (which was only for this class, and independent of a student’s prior GPA). This is referred to as the class GPA. From the class GPAs, we calculated the class average GPA (CAG) for each group.
Based on the GAUG and CAG, each member of every group could get additional “bonus” points added to the total score in the class. This additional score was calculated as follows: [(CAG − GAUG − 0.2) * 20]. Students’ grades were then recalculated with this bonus added to their existing totals, but with no change to the originally determined grade cutoff scores for the class.
To illustrate this, let us assume that the original cutoff for an “A−” was 80 points. In this case, we also assume that a particular student’s total for the class was 79, the student’s CLC GAUG was 3.1, and the student’s CLC CAG was 3.5. In this scenario, each member of this CLC will get an additional [(3.5−3.1−0.2) * 20] points, which comes out to an additional 4 points. The example student’s new total will now be 79 + 4 = 83 points, which will be an “A−”. If a CLC’s CAG was less than the GAUG, no points were taken off. Thus, there was no disincentive for participation in CLCs, and a student’s grade in the class did not depend directly on the overall performance of the CLC. Based on this system, students who built effective CLCs would learn better and get a small bump in their grades, but there was no penalty (except that of a lost opportunity) for students who chose to not participate in CLCs.
Student Survey
At the end of the class in 2019, students completed a survey to assess engagement with their CLCs. We asked students whether they met with their CLCs outside class, how often they met outside lecture times, and what modalities (using apps, in-person, or a combination) they used to meet. We could not repeat the survey in 2020 due to the pandemic.
Variables Analyzed
The dependent variables measured in this study are midterm exam scores and individual question scores (scores on questions that students did not see during phase 1, but answered independently in phase 2 of exams). Midterm exam scores were calculated by taking the average of all three midterm exams and calculating the percentage of the total score. Individual question scores were calculated by taking the average of the individual question scores and calculating the percentage of the total. The demographic variables used in this study include: minoritized status, first-generation college student status, transfer student status, sex, socioeconomic status (low income or not), and GPA. Except for GPA, all other demographic variables were treated as categorical for all analyses.
Data Analysis
All statistical analyses were performed using R in R-Studio. To evaluate whether there were demographic differences in CLC versus non-CLC students, we created contingency tables for each demographic variable and used a chi-square test to evaluate any differences in distribution.
To examine whether there were any differences in cumulative GPA between CLC and non-CLC students, we used a Kruskal-Wallis test to compare median GPAs between these two categories. We estimated effect sizes using the Hedge’s g method (Hedges, 1981).
Students in this study came from heterogeneous backgrounds and had varying levels of GPA. To determine whether group participation works for all students, we used generalized linear regression modeling (GLM) to evaluate the effect of various demographic variables on midterm exam (or independent question) scores. Example formulae are shown in Supplemental Figure S1. This allowed us to examine whether group participation is helpful in improving midterm scores when accounting for student demographics and to check whether there were any interaction effects observed between the demographic variables.
As multiple independent variables were assessed in this study, we examined Akaike information criterion (AIC) values for models that included or excluded a particular variable to test for an increase in accuracy. For all regression models shown, lower AIC values were accepted as the best model of the data. Only models that decreased the AIC value when a variable was included were retained (Wagenmakers and Farrell, 2004). To estimate effect sizes for the independent variables in the GLMs, pseudo-R2 values for the GLMs were first calculated by the method of Nagelkerke (1991)—also called Cragg and Uhler’s method—using the rms package in R. Subsequently, Cohen’s f2 was calculated from the pseudo-R2 values (Selya et al., 2012) to estimate the effect sizes for the significant predictors of the GLMs.
Data from the 2019 and 2020 classes were analyzed separately. For the sake of clarity, all of the data shown in the main paper are from the 2020 class, with equivalent analyses from the 2019 class included in the Supplemental Figures and Tables. Note that the COVID-19 pandemic did not affect our results for 2020, because all data were collected before the shutdown.
RESULTS
Demographic Distribution
As participation in small CLCs was voluntary, we examined the distribution of various demographic variables to determine whether there were any differences for students who chose to participate in the CLCs compared with those who did not (non-CLC). No significant differences were found in any demographic category, except for transfer students (Table 1A), who were more likely to participate in CLCs. CLC students also had a slightly higher average GPA than non-CLC students (Table 1B).
Engagement with CLCs
To assess whether students were actually engaging with their CLCs, we had students complete a survey at the end of the 2019 class (we could not collect these data in 2020 due to the pandemic). Data from the survey indicated that ∼82% of the respondents met with their CLCs at least once outside lecture times. Of the students who met with their CLCs, ∼68% met once a week, and the majority of students (∼54%) used a combination of modalities (in-person and virtually using various online platforms) to meet (complete results are shown in Supplemental Figure S2).
Exam Performance
To examine whether belonging to a CLC positively affects students over and above any benefits accrued from only discussing problems and activities in class, we looked at midterm exam performance for CLC and non-CLC students. Figure 3A shows that CLC students performed significantly better (Hedge’s g = 0.26, small effect) than non-CLC students on midterm exams. However, the difference in scores may be because lower-performing students could be “carried” by their higher-performing group mates during the collaborative portion of midterm exams. To account for this, we added questions that students only saw in phase 2 of the midterms (see Methods), which required students to answer these questions independently, without discussing them with their groups. Performance on these questions is referred to as the “independent question score.” Even on these independently answered questions, CLC students performed significantly better (Hedge’s g = 0.26, small effect) than the non-CLC students (Figure 3B), showing that forming learning communities that worked together in and outside lecture times correlated with additive gains, compared with just having students work together in random groups during class. Further, the results from the independent questions confirm that this is, in fact, due to improved individual student performance, as opposed to students just getting the correct answers from other students in their groups. We saw similar results for the 2019 class (Supplemental Figure S2), demonstrating that these results are reproducible and robust.
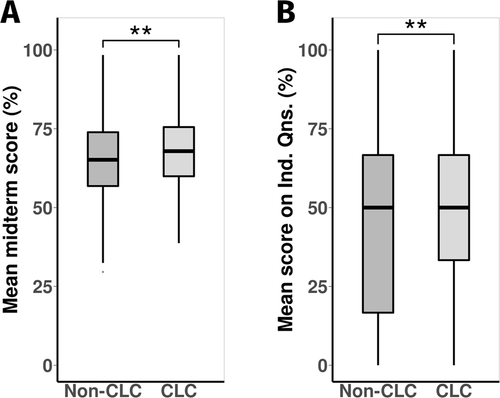
FIGURE 3. Comparison of exam performance between students in a CLC and those who were not in a CLC. (A) Performance on questions that students were allowed to discuss before answering and (B) performance on independent questions, which were questions that students answered with no prior discussions. The independent question score is a measure of true student learning in the class. In both cases, we observe that students in a CLC perform better than their non-CLC peers. **p value < 0.01. The p values were derived from a Tukey honest significant difference post hoc analysis, which followed a one-factor ANOVA to see whether performance differences were significantly affected by students being in a CLC.
To disentangle the effect of forming CLCs from the effect of demographic variables, we constructed GLMs (see Methods) that assessed the effect of various demographic variables on either overall exam performance (Figure 4A) or on independent question performance (Figure 4B). Coefficients for the full models are shown in Supplemental Table S1, and salient coefficients are plotted in Figure 4. Note that GPA is a strong predictor of performance, but is not shown in Figure 4, in order to better display the relative effects of the other demographic variables. We find that being in a CLC significantly improves student performance, independent of student demographics (Cohen’s f2 = 0.08, small to medium effect size), both based on overall performance (Figure 4A) and performance on the independent questions (Cohen’s f2 = 0.06, small to medium effect size; Figure 4B). Similar results were seen in 2019 (Supplemental Table S1), again demonstrating the reproducibility and robustness of our results.
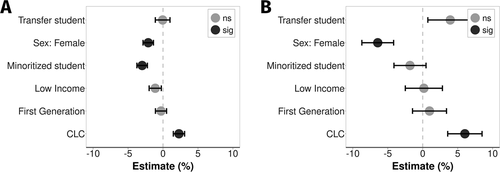
FIGURE 4. Coefficient plots estimating the effect of various demographic factors on overall midterm and independent question performance. Estimates were calculated using generalized linear modeling of (A) midterm exam scores or (B) independent question scores. ns, not significant; sig, p value < 0.05. Complete model coefficients and p values are shown in Supplemental Table S1.
Differential Effects of CLC Participation
To assess whether participating in CLCs disproportionately affects students with different demographic backgrounds, we constructed separate GLMs that included interaction terms between “CLC” (students in a CLC) and each demographic variable. An example of these GLMs is shown in Supplemental Figure S4. We found that only two demographic variables interacted with participation on CLCs: minoritized status and GPA.
First, we found that minoritized students especially benefited from being in CLCs. Equation 1 in Table 2 shows the effect of being in a CLC when students are from a minoritized background (URM = 1) versus when the student is not from a minoritized background (URM = 0). This equation (and all others in Table 2) is derived from the coefficients of the full GLM model (Fox and Weisberg, 2018), and the derivation is illustrated in Supplemental Figure S3. CLC students from minoritized backgrounds have a 4.2% increase in exam scores as opposed to a 0.6% increase when CLC students are not from a minority group. Equation 2 in Table 2 shows the effect of having a minoritized background when students belong to a learning community (CLC = 1) versus when they do not (CLC = 0). Non-CLC minoritized students do 5.8% worse than nonminoritized students on midterm exams compared with CLC minoritized students who do only 2.2% worse. These data indicate that participating in CLCs is especially beneficial to minoritized students (Cohen’s f2 = 0.03, small effect) and can help close the so-called performance gaps observed for this population of students.
Demographic variable or interaction | Equation number | Estimate for variable or interaction | p value | Equation for effect of variable |
---|---|---|---|---|
CLC | 1 | 0.6 | 0.572 | 0.6 + (3.6 × minoritized) |
Minoritized student | 2 | −5.8 | 0.000 | −5.8 + (3.6 × group) |
CLC*Minoritized student | 3.6 | 0.0341 | ||
CLC | 3 | 14.5 | 0.0164 | 14.5 + (−3.9 × GPA) |
GPA | 4 | 14.2 | 0.000 | 14.2 + (−3.9 × group) |
CLC*GPA | −3.9 | 0.0361 |
Second, we found that students with lower cumulative GPAs again benefited disproportionately by being in CLCs. Equation 3 of Table 2 estimates the effect of CLC participation on midterm exam performance when considering the students’ GPAs. Students with a lower GPA benefit more when they are in a CLC, compared with students with higher GPAs. For example, for a one-unit increase in GPA from 1 to 2, students in a CLC perform 6.7% better. However, for a one-unit increase in GPA from 2 to 3, the performance gain from being in a CLC is only 2.8% when other demographic variables are held constant.
Equation 4 in Table 2 estimates the effect of a unit increase in GPA when the student chooses to participate in a group (CLC = 1) or when the student does not belong to a group (CLC = 0). For each unit increase in GPA, belonging to a group (CLC = 1) increases exam score performance by 10.28% as opposed to a 14.2% increase in exam performance when not belonging to a group (CLC = 0). Taken together, these data indicate that, when students participate in CLCs, their performance is more likely to be influenced by what is learned in the class, rather than on prior characteristics that allowed for higher GPAs (Cohen’s f2 = 0.258, medium to large effect). These interactions, with similar trends, were also observed for the class of 2019, again showing the reproducibility of the effects. The complete list of coefficients for all the variables in the model that gave rise to Equations 1–4 and data from 2019 are shown in Supplemental Tables S2 and S3.
Differences between Groups
Although participation in CLCs seems to be beneficial overall, it is possible that the effects were heterogeneous across different groups. To examine this, midterm score distributions for individual groups (Figure 5) were compared against the median midterm score for non-CLC students (represented by the vertical dashed line in Figure 5). Due to the large number of groups, we split the data by class section, and only show data for one section here. Similar results were seen in the other lecture section of the 2020 class (Supplemental Figure S5). While the majority of groups, on average, did better than students who were not in CLCs, we note that there were still several groups that performed worse, showing that there are likely differences in group dynamics that lead to different outcomes and that can be further studied to improve the effect of having CLCs.

FIGURE 5. Overall midterm score distribution for individual groups. Overall midterm score distributions of each group are displayed in box plots. Dashed line shows the median score of non-CLC students for comparison. Light gray boxes indicate groups that had higher medians than the non-CLC students, and dark gray boxes are groups that had lower medians than the non-CLC students. Groups that did significantly better or worse than non-CLC students are indicated by asterisks: *p value < 0.05; **p value < 0.01. The p values were derived from a Wilcox rank-sum test comparing each group to the non-CLC students, and adjusting for multiple comparisons. Data for one section of the class are shown here, data for the second class section are in Supplemental Figure S4.
DISCUSSION
We describe a class structure that goes beyond merely having students discuss activities during class sessions. Using lessons from behavioral economics, we devised an incentive structure comprised of both short- and long-term gains that fosters student collaboration beyond the classroom, allowing for the formation of course-based learning communities. We call such groups class learning communities, or CLCs for short. These CLCs are administratively easy to set up, content independent, and can be adapted to most active-learning classes (including flipped and remote classes) with relatively small changes in the existing pedagogy of the course. Finally, because students are still given the agency to choose whether or not to participate in CLCs, we mitigate some of the negative outcomes seen for some students when they are forced to interact in small groups (Cooper and Brownell, 2016; Cooper et al., 2018; Hood et al., 2021).
Our results are reproducible over multiple years and show that such CLCs allow all students to do better in a large, active-learning class. Further, we show that incorporating CLCs is especially beneficial to minoritized students and allows for better assessment of learning in the class by reducing the effect of prior achievements. By using questions that assessed performance independent of discussions with CLCs, we demonstrated that the improved exam performance is not an indirect effect of “better” students carrying the “worse” students. The improved performance that we observed for students in a CLC (compared with their non-CLC peers) on the independent questions indicates that it was more likely that students learned from their peers throughout the quarter and were able to improve their own performances.
From the perspective of the instructor, there are only five changes that need to be made: 1) at the beginning of the class, the instructor sends out a survey, so that students can sign up to be placed in a CLC (we have included an example of what we used in the Supplemental Material); 2) the instructor must create the CLCs so that the groups are academically equally strong (we have included an R script that we use to accomplish this in the Supplemental Material); 3) there must be explicit instruction on how to engage effectively with small groups (we have included an example of the lecture slides we use in the supplementary materials); 4) there must be some short- and long-term incentivization for engaging with the CLC, but this cannot be directly tied to grades; and 5) there cannot be a perceived negative consequence for students who believe themselves to be in a “bad” group. We believe that these are relatively low-effort investments for most instructors, making this approach easy to adopt. We also refer the reader to the compendium of resources on group work developed by Wilson et al. (2018) that provides a comprehensive starting point for alternate ideas on how to create groups, what size groups to create, and what kinds of activities can be used to motivate true cooperative learning. Further, given the uncertainties of the pandemic, it is also readily adaptable for remote teaching, providing yet another way to increase student engagement and performance when teaching online.
We do note, however, that we do not see the performance gap for minoritized students completely go away in our context, showing that there is still work to be done to make the classroom more equitable and inclusive. Similarly, we observe that females continue to perform worse relative to men, even when they are in a CLC, and we do not see this gap reduce with the use of CLCs, again driving home the idea that there is no magic bullet that can easily solve the issues that we observe in our classrooms. Given the heterogeneous effects of CLCs (Figure 5), there is still much work to be done to understand the limitations of using small groups in education, and how improve them to facilitate optimal outcomes for all students. For example, we did not study the effect of this kind of implementation of small groups on LGBTQ+ students. We also cannot rule out the possibility that we are seeing a bias, perhaps in motivation, in the students who chose to join CLCs compared with those who did not, although demographic comparisons (Table 1) between CLC and non-CLC students makes this rather unlikely. Further, a significant majority of students (∼70%) chose to join CLCs, again arguing against the likelihood of some kind of extraneous bias affecting our results, but because we did not use a randomized control design, we cannot completely rule this out.
A substantial body of literature demonstrates the benefits of increased active learning for students in general and for minoritized populations specifically (Haak et al., 2011; Theobald et al., 2020). We hypothesize that the structured activities included in our intervention, coupled with the increase in self-esteem (Johnson and Johnson, 2009) that is one notable outcome of cooperative learning might lead to the disproportionately beneficial effects we observed for minoritized students. Indeed, a prior work has established that increases in self-efficacy mediated the beneficial effects of active learning for minoritized students (Ballen et al., 2017). Previous studies have also noted that female students tend to have their voices heard less often in the classroom (Eddy et al., 2014), and CLCs might help women (and other minoritized students) feel more comfortable participating more often, thereby also leading to increases in perceived teacher confirmation and/or reduced student apprehension (Ellis, 2004). Further studies that address the question of why some CLCs are more effective than others should also shed light on how these CLCs might be particularly beneficial to minoritized students, and how they can be modified to further narrow achievement gaps.
We posit that this demonstration of the benefit of using CLCs sets the basis for more widespread implementation and study of CLCs. Use of different incentive systems will likely improve student engagement in CLCs, with greater benefits. Being more intentional than merely using GPAs to form groups might also enhance the efficacy of CLCs and make them more equitable and inclusive. Interviewing students with a variety of experiences in their CLCs can help further refine best practices for their use. It is our hope that the relative ease of implementation of CLCs will be an incentive for time-strapped faculty to use this method as another tool in their classrooms to improve equity and inclusion and promote success for all students.
ACKNOWLEDGMENTS
We thank the Undergraduate Research Opportunities Program (UROP) of the University of California Irvine for providing funding for V.H.-I.C.