Understanding Randomness on a Molecular Level: A Diagnostic Tool
Abstract
Undergraduate biology students’ molecular-level understanding of stochastic (also referred to as random or noisy) processes found in biological systems is often limited to those examples discussed in class. Therefore, students frequently display little ability to accurately transfer their knowledge to other contexts. Furthermore, elaborate tools to assess students’ understanding of these stochastic processes are missing, despite the fundamental nature of this concept and the increasing evidence demonstrating its importance in biology. Thus, we developed the Molecular Randomness Concept Inventory (MRCI), an instrument composed of nine multiple-choice questions based on students’ most prevalent misconceptions, to quantify students’ understanding of stochastic processes in biological systems. The MRCI was administered to 67 first-year natural science students in Switzerland. The psychometric properties of the inventory were analyzed using classical test theory and Rasch modeling. Moreover, think-aloud interviews were conducted to ensure response validity. Results indicate that the MRCI yields valid and reliable estimations of students’ conceptual understanding of molecular randomness in the higher educational setting studied. Ultimately, the performance analysis sheds light on the extent and the limitations of students’ understanding of the concept of stochasticity on a molecular level.
INTRODUCTION
Analyzing students’ understanding is essential to gain insights into their conceptual framework in order to understand where they struggle to comprehend the concepts taught. Despite other formative assessment techniques, concept inventories constitute an ecological yet powerful tool to estimate students’ understanding of multiple topics (Klymkowsky and Garvin-Doxas, 2020). Concept inventories generally comprise a set of multiple-choice questions aiming at unveiling the scientifically incorrect conceptions held by students, using distractor items (wrong items) to yield an estimate of the deepness of the understanding of a concept or principle. Since the first publication of an impactful concept inventory in biology on the concepts of diffusion and osmosis (Odom and Barrow, 1995), many more such inventories have been developed (for an overview, see: Gregory; 2009; Furrow and Hsu, 2019). However, even though most of these inventories are designed for high school students, several studies report that even university undergraduate natural science students frequently struggle with fundamental concepts in biology, including randomness, evolution, and energy (Couch et al., 2015; Champagne Queloz et al., 2016; Fiedler et al., 2017; Gauthier et al., 2019).
An early attempt to assess undergraduate students’ understanding of biological concepts was achieved with the Biology Concept Inventory (BCI; Garvin-Doxas and Klymkowsky, 2008), which enabled the authors to identify misconceptions in a broad range of biological topics. Consistent with earlier findings, they discovered that students often misunderstood the role of stochastic (random) processes in biological systems (e.g., Ross et al., 2010). Student replies to questions on the role of randomness often appeared to be learned by rote and lacked deep understanding (Garvin-Doxas and Klymkowsky, 2008).
However, the BCI was intended to survey students’ misconceptions about biological processes in a more general manner rather than mapping out the details of students’ thinking about stochastic processes (Klymkowsky et al., 2003). In fact, only three questions from the BCI specifically tackled stochasticity in molecular systems and can therefore be used as a measure to analyze the understanding of the former (Klymkowsky et al., 2003). When the BCI was implemented in first-year undergraduate biology curricula in various Swiss universities, the results revealed that students frequently struggle with fundamental biological concepts and that the concept of randomness remains challenging in higher education (Champagne Queloz et al., 2016).
A recent study by Gauthier et al. (2019) yielded similar results by assessing students’ comprehension of the random nature of molecular processes using the Molecular Concepts Adaptive Assessment (MCAA). The MCAA constitutes a valuable instrument due to its adaptivity based on students’ answers. However, using the MCAA to identify misconceptions regarding randomness remains limited, as a significant part of the instrument consists of true-false statements and subsequent multiple-choice questions, often without correct answering possibility. Moreover, the fullness of the concept of randomness is only partially acknowledged, and statistical evaluations of the instrument’s validity and reliability are missing. Thus, the MCAA only partially assesses students’ understanding of stochasticity in molecular biology.
At the same time, it is worth noting that the important role of stochastic processes has become more apparent, arising in part from single-cell fluorescence, RNA sequencing, and related studies. These include monoallelic expression in diploid somatic cells (Reinius and Sandberg, 2015) and transcriptional and translational bursting (Ozbudak et al., 2002; Kærn et al., 2005). While the concept of stochasticity has gained importance in biological research and education, adequate tools to assess students’ understanding of this concept are missing. Given that, we set out to develop a set of questions that explicitly focuses on stochastic molecular processes.
Therefore, we developed the Molecular Randomness Concept Inventory (MRCI) and assessed the validity and reliability of undergraduate students’ responses when taking this test, using complementary statistical approaches. As course content often depends on lecturers’ topic preferences, the MRCI focuses on (stochastic) randomness without the requirement of other biological concepts having been covered. The MRCI consists of nine multiple-choice items exploring the boundaries of the students’ understanding of this concept and may help educators in higher education to assess their students’ knowledge and learning processes to design course materials addressing these topics. We administered the MRCI to a cohort of first-year natural science students with the intention of elucidating how well the students understand the concept of stochasticity on a molecular level and whether there are specific contexts in which the concept of randomness is more challenging to students. Furthermore, we tested biology doctoral students to examine the concept inventory’s upper limit and interviewed undergraduate students to analyze the response validity.
METHODS
Development of the MRCI
The MRCI was developed as part of an institutional initiative at ETH Zurich to advance teaching in higher education, namely the Future Learning Initiative (FLI). The development of the MRCI was stepwise and similar to the Randomness and Probability assessment instruments in the contexts of evolution and mathematics (Fiedler et al., 2017). In agreement with the guidelines set out by Treagust (1988), the process comprised 1) building and validating a concept map by collecting propositional knowledge statements and students’ misconceptions, 2) faculty expert review and validation, 3) a pilot study with natural science undergraduate students, 4) item refinement and revision, 5) a second faculty expert review, 6) concept inventory administration, and 7) assessment analysis and student interviews. Test development and administration followed the Standards for Educational and Psychological Testing (American Educational Research Association, American Psychological Association, and National Council on Measurement in Education, 1999). A more extensive elaboration of the individual steps follows in the subsequent paragraphs.
Concept Map and Faculty Review
The findings on students’ thinking from various studies were taken into account to examine the concept (Odom, 1995; Garvin-Doxas and Klymkowsky, 2008; Champagne Queloz et al., 2016). We extracted the naïve and incorrect conceptions that were reported in these publications. The compiled set of misconceptions, grounded in student answers that were either directly collected or indirectly described through test answers, was used to establish a concept map for the concept of “randomness in biological systems on a molecular level” through thematic analysis and subsequent clustering of emerged themes (Braun and Clarke, 2006). The concept map that resulted was used to define the concept inventory’s content (Figure 1). The first and second authors (S.T. and K.K.) of this study worked collaboratively on establishing this concept map, and the residual authors critically analyzed and commented on the map. In line with this, the concept map indicates the propositional knowledge statements, further describing students’ incorrect assumptions and conclusions. Four subconcepts crystallized as central factors influencing students’ understanding. These factors concern 1) the effectiveness of random processes; 2) the stochastic action of molecular processes; 3) the random behavior of molecules; and 4) the molecule-intrinsic thermal motion, which eventually leads to the random movement of molecules and atoms.
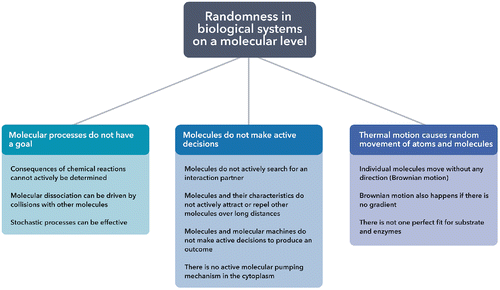
FIGURE 1. Concept map concerning randomness in molecular systems, including knowledge propositions for each subconcept.
Based on this concept map, we formulated four multiple-choice questions in addition to three adapted questions from the BCI (items 17, 18, and 25; Garvin-Doxas and Klymkowsky, 2008), one from the Molecular Biology Capstone Assessment (MBCA: item 12; Couch et al., 2015), and one from the Osmosis and Diffusion Conceptual Assessment (ODCA: item 3; Fisher et al., 2011), which appeared to be of particular importance for measuring the understanding of the concept of randomness. The distractors used in these questions aim to identify commonly held misconceptions and provide evidence for where students’ understanding of stochastic processes in biological systems can be improved and extended. The contexts range from the movement of proteins and RNA across barriers (i.e., the nuclear envelope) and their interactions with the nuclear cytoplasm as well as the membrane macromolecules they encounter, to the movement of neurotransmitters in the synaptic cleft upon synaptic firing. Three biology faculty experts internal to the research team assessed each question’s accuracy, and the items were revised to remove ambiguous formulations or ambiguous answer options.
As the MRCI was validated in German, the questions were developed in this language. For the BCI, the translated version of the questions was taken (Champagne Queloz et al., 2017). The items from the MBCA (Couch et al., 2015) and the ODCA (Fisher et al., 2011) were separately translated from English to German. All MRCI items are designed to preempt the measurement of unintended constructs (e.g., linguistic knowledge) as effectively as possible.
Each MRCI item consists of a question with four possible answers, of which three are distractors, and only one is correct. For the analysis, correct answers were rated with 1 point and wrong answers with no points. Mapping the MRCI items on the concept map highlights that all major subconcepts are directly or indirectly covered. A detailed overview of the, knowledge propositions and the MRCI items is shown in the specification table (Table 1).
Subconcepts and knowledge propositions | Item numbera |
---|---|
Subconcept A: Molecular processes do not have a goal. | |
A1: Consequences of chemical reactions cannot actively be determined. | R2c |
A2: Molecular dissociation can be driven by collisions with other molecules. | R2a, R2b, R2d |
A3: Stochastic processes can be effective. | R2b, R5d, R8a |
Subconcept B: Molecules do not make active decisions. | |
B1: Molecules do not actively search for an interaction partner. | R3a, R7a, R7b, R8b |
B2: Molecules and their characteristics do not actively attract or repel other molecules over long distances. | R3b, R5a, R5b, R6d, R8d, R9c |
B3: Molecules and molecular machines do not make active decisions to produce an outcome. | R1a, R1b, R4a, R4c, R5c, R6a, R6b, R9a, R9b |
B4: There is no active molecular pumping mechanism in the cytoplasm. | R3c, R7c, R8c |
Subconcept C: Thermal motion causes random movement of atoms and molecules. | |
C1: Individual molecules move without any direction (Brownian motion). | R3d, R5d, R6c, R7d, R8a, R9d |
C2: Brownian motion also happens if there is no gradient. | R4b |
C3: There is not one perfect fit for substrate and enzymes. | R1c, R1d |
Pilot Study, Item Refinement, and Second Faculty Review
After the approval of the university’s ethics committee to conduct all studies described herein, the first version of the MRCI was used in a pilot study. The participants were 21 second-semester undergraduate natural science students (52.4% female, 47.6% male, 0% nonbinary; M = 20.1 years, SD = 1.1 years) of a high-ranked Swiss university. Difficulty indices were calculated for the individual items (Blood and Budd, 1972; Padua and Santos, 1997). Additionally, discrimination indices were calculated as the correlation between the item of interest with the whole test score (Revelle, 2021). We found no item to be very easy or very difficult. Discrimination indices revealed that two items had to be revised. After refining these items, they were again discussed with biology experts regarding correctness and clarity. An example of such a revision is given in Table 2.
Before revising | After revising |
---|---|
Although an incorrect amino acid residue is bound to the tRNA molecule, it is attracted to the ribosome, and the incorrect amino acid is incorporated into the protein. | Even if incorrect amino acid residues are bound to the tRNA molecule, such molecules are actively attracted to the ribosome by the cytosol, and the wrong amino acid is incorporated into the protein. |
MRCI Implementation and Analysis
Participants.
The refined (second-generation) concept inventory was administered to 74 first-semester natural science students (71.6% female, 28.4% male, 0% nonbinary; M = 19.7 years, SD = 1.6 years) at the same Swiss university where the pilot study was conducted. The students studied health science and technology (86.5%) or medicine (13.5%). Of the participants, 62.2% had selected a science, technology, engineering, and mathematics (STEM) major in high school. In line with the norms for educational research practice in European contexts, socioeconomic status or diversity of race was not assessed (for recent examples, see Fiedler et al., 2017; Jaimes et al., 2020). The requirement for participation was being enrolled in a particular fundamental biology class. Participation was voluntary, there was no compensation, and the study’s performance did not impact students’ studies.
Materials.
To measure the convergent validity of the MRCI, in addition to the validity measures provided by faculty experts’ reviews, we assessed students’ self-efficacy in this specific topic separately. Self-efficacy regarding performance was previously shown to correlate strongly with actual performance (Richardson et al., 2012). Thus, this correlation measure was taken as an approximation for estimating the convergent validity (Tipton and Worthington, 1984; Weber et al., 2015; Krabbe, 2017). For that, we used an additional four-item questionnaire on a five-point Likert scale (from 1= strongly disagree to 5 = strongly agree, Cronbach’s α = 0.84), which was weakly adapted from Glogger-Frey et al. (2017). An exemplary item from the questionnaire was: “I am confident that I can answer questions about molecular motion.”
Procedure.
The test was administered as part of another study, which investigated the effects of narratives on understanding fundamental biological concepts (Tobler et al., 2022b). The impact of two different intervention materials on students’ understanding was compared using, in part, the MRCI. The study aimed to examine whether undergraduate students learn concepts better when they are presented in an expository text as typically found in textbooks (study group A) or as narratives, in which the same contents are embedded in the historical context of scientists who originally researched this topic (study group B). The participants were randomly assigned to read one of the two text materials and were instructed to answer test questions to assess their performance. The time for answering the questions of the MRCI in this on-site study was unlimited, and students were asked to work alone. They were instructed to select the answer that best fit the solution. Seven participants were excluded due to early submission (n = 1), a lack of effort (n = 1), or insufficient intervention language skills (n = 5; according to Melby-Lervåg and Lervåg, 2014). This procedure resulted in a final data set of 67 participants (70.2% female, 29.8% male, 0% nonbinary; 59.7% STEM background). Of these students, a subset of 24 participants (83.3% female, 16.7% male, 0% nonbinary; 70.1% STEM background) were recruited to retake the test 3 months after the first testing to investigate test–retest reliability.
Statistical Analyses.
All statistical analyses were performed in the R software environment (v. 4.2.1; R Core Team, 2022). The applied R packages are listed in Appendix C in the Supplemental Material. To analyze the subgroup differences (study group and educational background, respectively), we performed analyses of variance. As a proxy for convergent validity (Nunnally, 1967), the relationship between students’ performance and self-reported efficacy was investigated by calculating Pearson’s correlation coefficient (Tipton and Worthington, 1984; Krabbe, 2017). For an in-depth analysis, the reliability of MRCI and its items were analyzed using two complementary approaches: classical test theory and item response theory (IRT; Bechger et al., 2003). Therefore, Cronbach’s α (Cronbach, 1951) values and McDonald’s omega (McDonald, 1999) were calculated, and a Rasch model (Bond and Fox, 2007; Mair and Hatzinger, 2007) was built for the IRT approach. Students’ conceptual understanding in different contexts was investigated by looking at the response patterns of the individual questions of the MRCI. The test–retest reliability was determined by calculating Pearson’s correlation score for the performance results at the two time points (Vilagut, 2014). Additionally, a Bland-Altman plot was drawn (Altman and Bland, 1983) to graphically examine the test–retest reliability of the findings. A latent class analysis (LCA; Linzer and Lewis, 2011) was performed to identify subgroups based on students’ response patterns. The best-fitting model was selected based on a context-dependent fit indices comparison, taking into account the individual measures’ limitations (see Nylund et al., 2007; Yang, 2006) for the specific situation. Eventually, the students’ response patterns in the different latent classes and for the different subconcepts were examined.
MRCI Translation and Translation Validation
The MRCI questions were translated from German to English using the DeepL software environment (v. 3.1.133440). The correctness of the translation was manually examined, and minor issues were adjusted. The translation was validated by five graduate students in various disciplines who were fluent in both languages. These students were given first the English and then the German versions of all the questions, one at a time. After having seen the English version, they were instructed to give feedback on the translation in terms of wording and expressions used. Ignoring the correctness of the answer, each participant selected the same solution in the German and English versions for each question. These results indicate that the German and the English versions of the MRCI might be directly comparable and, thus, might measure the same constructs. Furthermore, comments regarding the translation given by these participants were integrated into the English version of the MRCI. The final products of this concept inventory development process (i.e., the MRCI in English and German) are attached in Appendix A of the Supplemental Material. In all studies described herein, only the German version has been applied.
MRCI Limit Examination
To investigate the upper limits of the assessment, we recruited biology graduate students to participate in the study. The participants were 34 German-speaking doctoral students (41.2% female, 58.8% male, 0% nonbinary; age: M = 28.1, SD = 2.3 years) at the same Swiss university, who arrived from 16 different universities; 97.1% (n = 33) obtained their master’s degrees in Europe, 57.6% of those (n = 19) in Switzerland. The requirement for voluntary participation was fluency in the test language (i.e., German). Three vouchers from a local grocery store valued at 20 Swiss francs were raffled off among all participants.
The doctoral students were invited by email to participate online and were asked work alone to answer the test questions. An outlier analysis was performed before the data analysis. We excluded eight participants due to performance score outliers (n = 1), implausibly short time spent on the test (n = 4), and statistical time outliers (n = 3). The final data set consisted of 26 participants (26.9% female, 73.1% male, 0% nonbinary; age: M = 27.3, SD = 2.3 years).
Student Interviews and Response Validity
To examine the response validity of the MRCI, we invited first-year natural science students to participate in an interview study in which their reasoning when answering the test questions was assessed. The interview process was designed according to the guidelines for cognitive laboratory interviews (Leighton, 2017; Willis, 2005), which aim to investigate whether students understand the test materials as they were intended and prepared.
Participants.
The participants were eight first-year natural science students of the same university (62.5% female, 25.0% male, 12.5% nonbinary; 37.5% STEM major in high school; 25% fluent second language speakers, 75% German as first-language speakers). An a priori informative power analysis indicated a necessary sample size of up to 10 participants (Creswell and Poth, 2016; Omona, 2013). The requirement for eligibility was to be inscribed to a specific first-year introductory biology course. Participation was voluntary and not coupled with passing the course.
Materials.
Whereas the MRCI was used to assess understanding of the concept of molecular randomness, the participants also had to indicate on a four-point Likert scale for each topic of the MRCI whether they had already studied this topic in their prior education.
Procedure.
Before the actual interview, students who indicated an interest in participating were individually contacted and invited to read the information sheet for participants. The meeting was conducted using Zoom. Audio and video files were recorded for subsequent transcription of students’ answers during the interview. For the interview, students were informed about the overall procedure, solved a practice exercise with the interviewer, and then solved the MRCI alone. For each question, they were asked to explain why they selected or deselected a specific answer option. In the end, students indicated their prior experience in education with the covered topics and answered a few questions used for descriptive statistics. Participation was compensated with a voucher for 10 Swiss francs from a local grocery store. The exact wording of the instruction during the interview is presented in Appendix D1 of the Supplemental Material.
Coding and Statistical Analyses.
The registered reports were analyzed grounded in the question feature-coding analysis model (Willis, 2015). This model focuses on the coded rating of verbal data, emphasizing the individual test items. Verbal reports were categorized as 1) comprehension of the question, 2) retrieval from memory, 3) judgment of retrieval, and 4) response (Leighton, 2017; Tourangeau et al., 2000). Subsequently, the data were aggregated following the suggested procedure by Creswell and Creswell (2005). A more detailed overview of the analysis protocol and the coding scheme is available in Appendices D2 and D3 of the Supplemental Material. Performance and questionnaire data were descriptively assessed.
RESULTS
Statistical Analysis of the MRCI
Descriptive Statistics.
The test performance results did not show any significant main effects of the study group A or B, F(1, 63) = 0.009, p = 0.925, or educational background, F(1,63) = 1.784, p = 0.187. Furthermore, descriptive statistics indicate substantial within-group variation (Table 3 and Figure 2). Taking these findings together, we found no evidence favoring the hypothesis that the subgroups are statistically significantly different. However, subsequent equivalence testing did reveal that the observed effects of the comparisons are statistically not different from zero and not equivalent to zero, likely due to the small sample size; study group comparison: t(64.12) = 0.0177, p = 0.986; educational background comparison: t(66.31) = −0.496, p = 0.622 (Lakens et al., 2018). Follow-up equivalence Bayes factor estimation for independent samples (Heck et al., 2019; Kelter, 2021) revealed evidence for equivalence in both comparisons (study group: BF01 = 7.995; educational background: BF01 = 2.985). Thus, these results indicate that the groups are, in fact, similar and can be combined for further analyses to increase the sample size for follow-up statistical analyses. A detailed description of the study groups can be found in the Methods.
Subgroup | Sample size (n) | Mean (M) | SD |
---|---|---|---|
All participants together | 67 | 4.32 | 2.28 |
High school STEM major | 40 | 4.60 | 2.20 |
High school non-STEM major | 27 | 3.93 | 2.34 |
Study group A | 35 | 4.31 | 2.34 |
Study group B | 32 | 4.34 | 2.22 |

FIGURE 2. Participants’ performance distribution in the individual subgroups of interest. Transparently colored dots indicate unique data points, and diamonds indicate the means of the groups. Descriptive statistics are shown in Table 3.
Convergent Validity Examination.
We explored the relationship between the average score of the student-reported self-efficacy in this topic and the individual performance on the MRCI as a proxy for convergent validity. A significant Pearson’s correlation was found between higher self-efficacy scores and better performance on the MRCI (r = 0.33, p = 0.0069, n = 67). This result confirmed that MRCI performance measures the understanding of random processes on a molecular scale and thus further supports the validity of the MRCI.
Item Difficulty, Discrimination, and Reliability Measures.
The item difficulty (P) was calculated by taking the average score per item, as the scoring was dichotomous (1 point = correct answer; 0 points = wrong answer). The difficulty measures ranged from P = 0.27 to P = 0.75 (M = 0.48, SD = 0.17; Table 4). Following Padua and Santos (1997), no question was too difficult or too easy, and the results indicate a diverse range of item difficulty. The discrimination indices (DI), which give an estimate of how well a specific item can discriminate between high- and low-performing students, were found between DI = 0.28 and DI = 0.72 (M = 0.53, SD = 0.17; Table 4). In accordance with Blood and Budd (1972), the results show that no item needs to be discarded (DI < 0.2). Furthermore, the results indicate that most items (six out of nine) show good discrimination indices, thus suggesting the high validity of MRCI items.
R1 | R2 | R3 | R4 | R5 | R6 | R7 | R8 | R9 | |
---|---|---|---|---|---|---|---|---|---|
Item difficulty | 0.75 | 0.31 | 0.69 | 0.52 | 0.42 | 0.63 | 0.27 | 0.42 | 0.33 |
Discrimination | 0.28 | 0.49 | 0.67 | 0.47 | 0.75 | 0.63 | 0.36 | 0.36 | 0.72 |
The internal consistency measure of reliability of the whole concept inventory was calculated as α = 0.68 (95% confidence interval = [0.57, 0.79]). As Cronbach’s α might not always be the preferred method due to measure-inherent assumptions (for an overview, see Zinbarg et al., 2006; McNeish, 2018), McDonald’s ωt value for the MRCI was calculated in a complementary approach (ωt = 0.75). The total omega ωt strives to describe a general factor that explains all individual question items together, thus yielding a more stable reliability estimate (Zinbarg et al., 2006). Taking both measures together, we found evidence for an acceptable level of reliability of the MRCI in the present data set.
Rasch Model Validation.
Using the Rasch model, a person’s ability and the item difficulty are taken as parameters to yield an estimate of the probability of solving a particular item (Rasch, 1960). For instance, higher ability goes along with a higher chance of answering a question correctly. This information can be used to build a person-item map, which allows for analyzing the internal structure of the questionnaire. Taking the empirical results from the MRCI administration into account, such a person-item map was plotted (Figure 3), revealing an even distribution of the estimated item difficulty over different ability ranges. Consequently, item R1 appears to be the easiest item, while item R7 is the most difficult. Items R5 and R8 display a similar level of difficulty. The person-parameter distribution further confirms these results, because the performance of most students (72.6%) was attributable to the normally distributed data around the MRCI items. Only a minor number of students were assigned to the two groups at the minimal and maximal boundary of the scale (minimal boundary: 11.5% of the students; maximal boundary: 15.9% of the students).

FIGURE 3. Person-item map with item difficulty as latent dimension and person parameter distribution indicating participants’ ability. The latter shows a histogram of students’ performance on the MRCI. The location of an MRCI item on the latent dimension (black dot) corresponds to the expected ability at which 50% of the test takers would have solved the item correctly. A higher value on the latent dimension indicates a more challenging item. Ticks below the person parameter distribution indicate the location of the MRCI items on the latent dimension in relation to students’ ability and show the discriminatory power of the MRCI.
For testing the fit of the Rasch model on the MRCI data, a Martin-Löf test was conducted. The results indicate unidimensionality, χ2(19) = 6.62, p = 0.996, and thus a good Rasch model fit. The model fit of each item was analyzed 1) by individual infit and outfit scores, which represent the normalized weighted or unweighted values based on the data’s mean squares statistic (Smith et al., 2008a); and 2) through item-specific χ2-tests, which compare the actual number of correct answers to an item to the expected number of correct answers in the same group of persons (Müller, 2020).
Looking at the fit statistics of the individual items of the MRCI indicates that most items demonstrate a good fit to the model (Pallant and Tennant, 2007; Robinson et al., 2019; Table 5). Reported z-scores of infit and outfit for items R3 and R5 are slightly out of range (i.e., outside the range of ±2.5). However, they do not show a significant deviation from the Rasch model after correcting for multiple testing as indicated by the item-specific χ2-test. Only item R1 shows a significant divergence based on the χ2-test statistics, potentially due to its low difficulty. In conclusion, the Rasch model approach’s findings agree with the item difficulty, discrimination, and reliability measures, indicating overall high reliability and validity of the MRCI’s responses.
R1 | R2 | R3 | R4 | R5 | R6 | R7 | R8 | R9 | |
---|---|---|---|---|---|---|---|---|---|
z-scorea | |||||||||
Infit | 1.327 | 0.264 | −2.773 | 0.947 | −2.882 | −1.866 | 0.968 | 2.109 | −2.290 |
Outfit | 1.881 | 1.183 | −1.868 | 0.156 | −2.537 | −1.512 | 1.767 | 1.556 | −2.093 |
χ2 statistics | |||||||||
χ2 (64) | 114.509 | 86.211 | 34.295 | 66.176 | 35.167 | 42.442 | 105.166 | 87.809 | 34.756 |
p-valueb | <0.001* | 0.034 | 0.999 | 0.402 | 0.999 | 0.983 | 0.001 | 0.026 | 0.999 |
Test–Retest Reliability.
The Pearson’s correlation score indicates a strong and significant correlation between the participants’ performance scores comparing the two time points (r = 0.73, p < 0.0001) and thus suggests high test–retest reliability. The results of the Bland-Altman plot are in line with these findings and indicate that all data points lie between the limits of agreement (Supplemental Figure S1).
Interview Analysis and Response Validity
The average performance of students who participated in the interview was lower than the performance of those in the classroom study (M = 2.25, SD = 1.28). However, when asked how many topics of the MRCI they recognized, the participants indicated that they had already learned ∼89% of the topics on average (SD = 21%). Analyzing the aggregated codes from the interviews and investigating the reasons for selecting or deselecting a specific answer revealed that, in 94% of all cases, students selected either the correct answer with a correct justification (24%) or the wrong answer due to a misconception regarding molecular randomness (76%). Thus, the interview study demonstrated strong response validity for the MRCI, as implied by the students’ thought processes. Descriptive plots of students’ MRCI performance, the indication of recognized topics, and aggregated scores indicating the number of answers related to the randomness concept are displayed in Supplemental Figure S3. The MRCI’s question-wise analysis of topic recognition and response reasoning are shown in Supplemental Figures S4 and S5, respectively.
Performance Analysis of the Study
The analysis of the individual item responses of the MRCI administered to the cohort of first-semester natural science students revealed prevalent difficulties in students’ conceptual understanding of molecular stochasticity in biological systems (Figure 4). Whereas certain items were generally correctly solved (e.g., R1, R3, or R6), other questions were solved correctly by fewer than one out of three students (e.g., R2, R7, or R9). In summary, the results indicate that many students do not fully understand the concept of randomness. Moreover, it appears that understanding the random nature of molecular movements in specific contexts, for example, the movement of the RNA–polymerase complex in prokaryotes (R7), is more challenging than in other contexts, such as diffusion (R6). Taking these observations together, the results indicate individual performance differences.
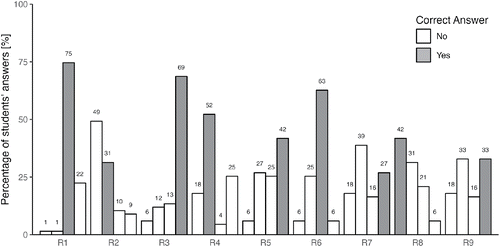
FIGURE 4. Response analysis of the MRCI implementation study results (n = 67). The number above each bar shows the rounded percentage of students who have selected this specific answer. All questions are represented in four grouped bars, whereby each of these bars indicates a different answer possibility in the sequence a to d.
LCA Reveals Three Subclasses in the Student Population.
An LCA was conducted to identify subgroups based on individual performances. The LCA fit measures revealed diverging results (Table 6). Whereas the Akaike information criterion (AIC) and Pearson’s χ2 statistic indicated the highest model fit with three classes, the Bayesian information criterion (BIC) suggested only two classes. Despite the higher vulnerability of the AIC with small sample sizes (Nylund et al., 2007), BIC measures have been reported to underrate the actual number of classes and tend to decrease in reliability with unequal class sizes (Yang, 2006). Hence, we decided to use the three-class model for further analyses.
Latent class analysis | AIC | BIC | χ2 b |
---|---|---|---|
LCA with 2 classes | 727.13 | 769.02 | 350.95 |
LCA with 3 classes | 717.52 | 781.46 | 358.69 |
LCA with 4 classes | 724.05 | 810.04 | 321.02 |
LCA with 5 classes | 731.64 | 839.67 | 223.82 |
In the following and based on the average group performance in the MRCI, these three classes are denoted as “low achievers,” “average achievers,” and “high achievers.” Figure 5 indicates the subgroup-dependent probabilities of answering the different items of the MRCI correctly; Figure 6 splits up the response pattern for the individual classes and questions of the MRCI.

FIGURE 5. LCA of the MRCI. The colored lines in the plot indicate the different classes, and the gray line shows the item difficulty for the nine MRCI questions. Higher values thereby indicate easier questions. The legend's percentage displays the relative number of students assigned to one of the three groups.

FIGURE 6. Performance analysis for the individual identified classes. The numbers on top of the individual bars indicate the relative number of students from a group that selected this answer option. Students are grouped into the three classes: “low achiever” (n = 24), “average achiever” (n = 29), and “high achiever” (n = 14), based on the latent class analysis. Again, all questions are represented in four grouped bars, whereby each of these bars indicates a different answer possibility in the sequence a to d.
The results from the LCA show three very distinct response patterns: While students in the high-achieving group solved most questions correctly, they only seemed to struggle with items R7 and R8. In contrast, the average-achieving students answered only a few questions correctly (i.e., R1, E3, or R6) and struggled with the other questions. Interestingly, there is often no uniform response distribution that would indicate a random answering behavior. Instead, most students actively selected one specific answer containing a distractor, indicating that this group of students holds a particular misconception (e.g., R2 answer a or R7 answer b). In contrast to these two first subgroups, the low-achieving students show very different performance behavior. Similarly, item R1 was solved correctly by most of the students. However, all other items were solved correctly only by a minority or not at all, as in items R5 or R9. Intriguingly, most students in the low-achieving group frequently selected the misconception, which was prominent in the average-achieving group, yet to a lower extent. For instance, for item R2, only 21% of the high-achieving students chose answer option a, whereas more than double as many students in the average-achieving group and around two-thirds of all students in the low-achieving group selected this answer. A similar pattern is detectable when looking at items R8 or R9.
However, analyzing the individual questions in isolation might obscure certain patterns, considering that the items contain distractors concerning various misconceptions (Table 1). Therefore, in a subsequent step, we looked at the response pattern for all questions in relation to those subconcepts that cover misconceptions. This analysis does not consider subconcepts A3, C1, and C2, because these concepts are represented only by correct MRCI answers.
The relative occurrences of a misconception in the subconcepts (Table 1) relative to all wrong answers in a particular class (low, average, and high achievers) are displayed in Figure 7. Although the percentages do not allow us to make statements regarding the frequency of misconceptions within the different classes, as not all subitems from all subconcepts occurred equally often (i.e., concept subitem A1 is only directly assessed by one distractor item in the whole MRCI, whereas concept subitem B1 is covered by four distractor items in the MRCI), the results from this analysis (Figure 7) enable comparisons among the three classes.
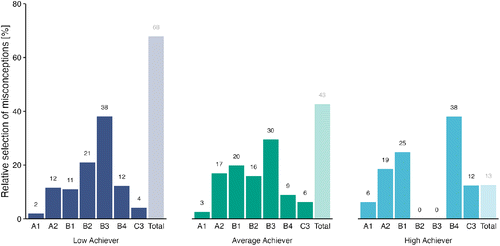
FIGURE 7. Students’ relative choice of misconceptions over the whole MRCI, grouped in the three latent classes. Small numbers above the bars indicate the percentage of students per class who have selected a specific distractor answer that contradicts a particular concept over all possible distractors. The individual bars show the subconcepts from Table 1, subdivided by the individual subitems. Subconcepts A3 and C1 are not shown, as they only contain correct MRCI answer options. The transparent bars indicate the relative number of wrong answers for each latent class.
Subconcept A: Molecular Processes Do Not Have a Goal.
Distractor items concerning subconcept A, which states that molecular processes do not have a goal, were similarly frequently chosen by students in all different classes (in case the question was not answered correctly). For instance, in question R2, 67% of the students in the low-achieving and 48% in the average-achieving class answered that two non-covalently bound molecules could only be separated through distinct processes like chemical reactions (Figure 6). Random collisions with other molecules as mechanisms to separate them remain a less convincing solution than the distractors. Instead, students assume that there needs to be an active process that leads to a specific outcome.
Subconcept B: Molecules Do Not Make Active Decisions.
The analysis of subconcept B shows that students assume various ways molecules make active decisions. Interestingly, the choice of explanation differs in the three latent classes (Figure 7). No student of the high-achieving class selected MRCI distractor items implying that molecular machines make active decisions to produce an outcome or that molecules can actively attract or repel other molecules. However, some students in this group still believed that certain molecules search actively for interaction partners. Looking at the response pattern of question R7, only 57% of the students in the high-achieving class selected the correct answer stating that faulty tRNAs arrive at the ribosome through random motion.
Students in the average-achieving class show a similar, yet more drastic, response pattern. Only 14% of this class’s students selected the correct answer, whereas a majority (76%) assumed that such faulty tRNA molecules are attracted to the ribosome. The response pattern of the low-achieving group also suggests that students believe that some active processes are necessary for these tRNAs to arrive at the ribosome. However, the similarity in the response pattern indicates that students were potentially partly guessing which answer was correct.
Similarly, in question R8, only 57% of the high-achieving students understood that the RNA-polymerase complex arrives at the promotor region on the DNA by random movement and not by active processes. Answer possibilities that include transcription initiation factors that search and recruit the polymerase or active pumping also seem likely to students. Instead, students in the average- and low-achieving classes believed that active processes lead to the polymerase and the promoter region encounter. Yet, in contrast to the high-achieving group, students who did not answer correctly mostly assumed that some molecules actively search for interaction partners. Most students of the high-achieving class who selected the incorrect answer guessed a pumping mechanism. These two questions, R7 and R8, appear to be the most difficult ones for the high-achieving group. In most other questions, they understand that random processes lead to a particular outcome. Nonetheless, they seem to neglect the same underlying processes in more fundamental biological contexts like replication and transcription. These differences might point to a different level of conceptual understanding, which is more naïve in the low- and average-achieving groups and more elaborated in the high-achieving group. However, more than 40% have not reached a complete, correct understanding, even in the high-achieving group.
Students also assume active processes to be involved in bringing ions to membrane-residing ion channels. For question R9, 42% of the students in the low-achieving class and 41% in the average-achieving class chose the distractor that states that energy needs to be made available to recruit these ions. A bit less frequently, students from these two groups answered with a pumping mechanism or the arrival of the ions at the channels due to charge. None of the low-achieving students and only 28% of the average-achieving students understood correctly that ions also move randomly toward the ion channel.
Consequently, in question R3, which asks the student to imagine an ADP molecule in a bacterial cell and how this molecule might get to an ATP synthase to be completed to form an ATP molecule, all students in the high-achieving group selected the correct answer. Similarly, most students in the average-achieving class answered correctly. Only students from the low-achieving group seemed to not understand that ADP can arrive at the ATP synthase without any active processes. Instead, answers including an active pumping of the ADP molecule, an active grabbing by the ATP synthase, or the attraction due to the molecules’ electronegativity appear to be convincing solutions. Even more striking results are obtained in question R5. Asking how a ligand can cross the synaptic cleft, no student in the low-achieving class and only around half of the students in the average-achieving class selected the correct answer, claiming that a neurotransmitter does not always move in the direction of the receptor but can also move away from it. Instead, the students explain this phenomenon by relaying active processes like specific transport proteins or charged regions that attract each other even over the distance of a synaptic cleft. A transport protein is also the most frequently selected answer in the low-achieving group to the question of how proteins that should be imported to the nucleus find the nuclear pore complex.
Whereas low-achieving students consistently choose active processes instead of random events as explanations, this is not the case for average-achieving students. Comparing the response pattern of this class for questions R7 (arrival of tRNA molecules at the ribosome synthase), R8 (access of the RNA polymerase to promotor regions), or R9 (approaching of different ions to transmembrane proteins), with those for questions R3 (arrival of ADP at the ATP synthase) or R6 (protein-nucleus-encounters), for instance, it appears that students are aware of stochastic processes on a molecular level only in distinct contexts (i.e., R3 or R6). However, they were unable to transfer this knowledge to other concepts. The high-achieving students seem to understand the concept of stochasticity in biological systems to a greater extent and were able to transfer the randomness concept to many different contexts or biological processes. Having solved most questions correctly, they only showed partial misconceptions about fundamental processes such as transcription or translation.
Subconcept C: Thermal Motion Causes Random Movement of Atoms and Molecules.
Question R4 indirectly compares diffusion with the thermal motion of molecules. While all students from the high-achieving group understood that random movements of molecules lead to their even distribution, only 34% in the average-achieving class and 46% in the low-achieving class selected this answer. Instead, many students in the latter two groups attributed the overall diffusion mechanism to the individual molecules’ will to move away from those more crowded regions. The idea that the movement of molecules on a macroscopic level is directed away from more crowded areas is not wrong. Yet the reason is that the molecules encounter fewer other molecules with which they would collide and thus move over larger distances without changing their direction. It is not the molecules’ decision to move away but rather the decreased probability of colliding if fewer molecules are around. However, this explanation is only correct in an empty space. The cellular environment is crowded (Brownian diffusion is still applicable; Dix and Verkman, 2008), and the explanation that the chance of repulsion is higher in a region of higher concentration is not correct. Thus, the transfer of a chemical concept (diffusion) in biological systems appears to be difficult for many students. This conclusion was also reflected in the student interviews. For example, one student stated, “[This] answer … sounds too chemical. That is why I exclude it”, implying the challenge of transferring these concepts.
Finally, question R1 asks how it can be ensured that a molecule binds the correct partner and how wrong interactions are avoided. Most students in all latent classes solved this question correctly. However, 22% of all students chose the explanation that correctly bound molecules bind to each other like puzzle pieces. This explanation constitutes a frequently used analogy in natural science education that was already shown to cause remaining misconceptions if not explained properly (Orgill and Bodner, 2007; Tobler et al., 2022a).
MRCI Limit Examination
For determination of the MRCI’s upper limits, the average performance scores of biology doctoral students were examined and calculated as 6.73 (SD = 1.56; min = 3; max = 9), indicating that even some experts in biology do not fully understand the concept of stochasticity in molecular systems. Accordingly, the results indicate that the MRCI might apply at various higher education levels. However, more than 30% (n = 8) had zero or only one mistake, and nearly 60% had no more than two mistakes (n = 15). The summative scores are displayed in Supplemental Figure S2.
DISCUSSION
The growing acknowledgment of stochasticity in biological processes and the lack of a sophisticated diagnostic tool to assess students’ understanding of randomness in molecular biology stimulated the development of a novel concept inventory to assess students’ understanding of this concept. Thus, we developed the MRCI to evaluate students’ understanding of the fundamental concept of stochasticity in molecular processes and examined the validity and reliability of the data gathered for students’ responses using various psychometric analyses. Moreover, implementing the MRCI allowed identification of misconceptions common to undergraduates and pointed to specific biological topics in which the role of randomness is not yet fully understood by students. The materials and findings from this study might help educators reliably assess students’ understanding of the concept of stochasticity, inform teachers and students about their knowledge, and support faculty in adjusting their lectures and curricula.
Validity and Reliability Estimation of the MRCI Administration Study
Our findings suggest that the estimates obtained by administering the MRCI yield reliable and valid estimates of students’ understanding of randomness, also in light of the response validity analysis based on the think-aloud interviews. Moreover, we could show that the MRCI measures one conceptual dimension, as intended, and is a good fit between the Rasch model and the individual items. Only item R1 showed a statistically significant deviation from the model. However, this is potentially due to the relative easiness and, thus the lower discrimination index, of the question. Furthermore, the complementary approaches (i.e., item difficulty and discrimination) supported its retention in the MRCI.
Students’ Conceptions of Randomness and the Role of the MRCI
The results we obtained by administering the MRCI and conducting the interviews aligned with the literature (Garvin-Doxas and Klymkowsky, 2008; Champagne Queloz et al., 2016; Fiedler et al., 2017; Gauthier et al., 2019), in that undergraduate university students do not seem to fully comprehend that stochastic processes underly molecular biological systems. The understanding that random events can be effective in reaching a beneficial outcome seems to be missing for many students. The interviews supported this conjecture. For instance, one participant stated, “It just sounds too random and as if there wouldn’t be any logic behind it.” Similarly, another student independently said, “This [random motion] appears to be very inefficient, and I really hope that it is not inefficient.” In specific examples like diffusion, students know that random processes are involved. However, they did not seem to see the same underlying processes in other situations if trigger words such as “diffusion” are missing. Instead, students may not fully understand the concept but have studied rote answers regarding molecular processes in specific situations. Garvin-Doxas and Klymkowsky (2008) drew a similar conclusion and found that students are aware of the random component in diffusion but cannot transfer this knowledge. However, the implementation of the MRCI allowed for a deeper analysis of students’ conceptions of molecular stochasticity, especially regarding student reasoning, thus yielding insights that earlier published concept inventories (Garvin-Doxas and Klymkowsky, 2008; Fiedler et al., 2017; Gauthier et al., 2019) could not reveal. The nine items, with 36 response options comprising 27 topically diverse distractors, allow a fine-grained analysis of students’ knowledge and a clear elaboration of the related knowledge boundaries. Being able to gather information not only from individual questions but from a multitude of items further allows detection of patterns of students’ conceptions in cases in which certain aspects of a concept are already covered, and thus, the students know the answer to a particular question.
The latent class performance analysis further revealed different patterns of understanding of biological phenomena. Whereas the concept of randomness was clear to almost all students in the high-achieving class for many topics, more than one-third had difficulty applying the concept to the most fundamental processes, namely transcription and translation. Most students from the average achievement class also did not appreciate stochastic processes in these two concepts but additionally failed to understand the same concept in other contexts. The students apparently knew that random motion is essential for the molecules’ movement in specific situations. However, they were unable to transfer this knowledge to other situations. Finally, most students from the low-achieving class struggled with the concept of stochasticity in all contexts presented.
Considering the different incorrect answers given to explain random events through active processes, the response pattern varies drastically among the three groups. Whereas students in the low-achieving group agreed on various wrong explanations (from the active decision making of molecules to active forces acting on molecules), students in the high-achieving group agreed that molecules cannot make decisions or perform specific actions as needed. Instead, if they wrongly assumed an active process, they mainly explained this through mechanisms that work on the molecules as active pumping. In light of the conceptual change framework (Vosniadou et al., 2008), it could be argued that students start with a teleological understanding of the world, in which all processes need to have a causal explanation (Coley and Tanner, 2015). Later in their education, they learn topics in biology such as transcription or translation and neural communication. The new information is thereby integrated into the students’ framework of prior knowledge. So-called synthetic concepts (Vosniadou et al., 2008) emerge, which correspond to a middle way between the naïve understanding and the scientific theory.
A similar development of conceptual understanding could be plausible for the concept of random movement at the molecular level (Figure 8). Students who explain random processes through active actions and decisions of molecules, like the direct grabbing of the ADP molecule by ATP synthase, might later develop an understanding that single molecules cannot perform such actions. However, they continue to assume that a nonrandom process must guide the molecules to the ATP synthase, implying that a stochastic behavior cannot effectively drive this process. Eventually, the students reach a complete, scientifically correct understanding, realizing that the ADP molecules arrive at the ATP synthase by random movements and not through active mechanisms. Therefore, concepts learned earlier might be more strongly anchored in the knowledge framework and are thus more challenging to refute, partly because the knowledge needed to refute these misconceptions is not taught simultaneously when the new concept is introduced. These early synthetic concepts might even be reinforced by attempts to explain these processes, for instance, frequently encountered textbook pictures that show enzymes and their substrates already in the correct position to interact.

FIGURE 8. Conceptual change model for the random movement of molecules in cellular environments. Synthetic models (Vosniadou et al., 2008) describe students’ conceptual explanations that do not entirely agree with the scientific understanding of the same concept. Students’ ideas of how the ADP molecule arrives at the ATP synthase thereby develop from the model in A, the active grabbing of the molecule by the ATP synthase, to the active pumping mechanism that brings the ADP molecule to the synthase without active actions of the molecules themselves (B). Finally, students arrive at acknowledging that molecules move by random motion, which drives ADP toward ATP synthase (C). The latter corresponds to the scientifically accepted model.
In conclusion, the results of this study suggest that students might benefit from timely analysis of putative misconceptions and subsequent targeted support on their learning path toward obtaining a complete conceptual understanding of the role of randomness in biological systems. Instructors at all levels of education, from high school to university, should stress the issue of randomness in biology in much greater detail and point to its role in the various biological processes they present to the students. Potentially, novel teaching methods should be more frequently integrated into higher education settings. For instance, student-centered teaching strategies, including more interactive learning opportunities and formative assessments, increased students’ conceptual understanding in university classrooms (Connell et al., 2016; Smith et al., 2019) and could support advances in tackling the concept of stochasticity in molecular biology.
Administration of the MRCI
As the use of the MRCI is not bound to a specific topic but rather infers conceptual knowledge, its administration is not tied to a specific time point in education or a particular curriculum. Results from the doctoral student examination additionally revealed that the MRCI’s upper limitations are not easily reachable, indicating its applicability on various higher educational levels. Also, the format of the MRCI suggests various economic and straightforward uses to assess students’ understanding. Ways to do so could include 1) using it as a (formative) assessment tool of the current state of students’ knowledge, 2) administering the MRCI in a pre- and posttest design to investigate the impact of a new teaching methodology or curriculum, and 3) taking individual questions from the MRCI to test the understanding of randomness in a specific topic. Moreover, independent of how and when the MRCI is used, educators might be able to specifically detect individual difficulties or ubiquitous incorrect conceptions, which might indicate where or when more detailed or specific instructions could be beneficial for learning. Using this test in classrooms could also help make students aware of potential knowledge gaps and could help them identify critical aspects of randomness in other molecular processes. However, the validity and reliability of the data gathered through the MRCI described here are only applicable if the test is used as a whole. Furthermore, the present results are based on the German version of the MRCI. Additional translation validation might be valuable before conducting a study with the English version.
Based on the test–retest reliability results with delayed testing after 3 months, we recommend using the MRCI as an assessment tool for testing the understanding of random processes in biological systems in higher educational settings, for example, in pre- and posttest designs to assess the development of students’ understanding as encouraged in earlier studies (Smith et al., 2008b; Shi et al., 2010; Fisher et al., 2011).
An important use of the MRCI results could be to raise awareness that explicitly teaching processes on a molecular level could support students in comprehending stochasticity in biological systems, such as the stochastic differences in gene expression levels displayed by cells of the same type as revealed by single-cell methods (Reinius and Sandberg, 2015). Students might benefit from considering why the distractors are wrong to understand why the correct answer is correct. Also, there should be no time restrictions to the MRCI to avoid time pressure as a confounding factor. Further, students should be instructed to work alone and without any aids. The individual questions are not building on top of each other and thus can be displayed randomly. All the MRCI questions can be found in Appendix A of the Supplemental Material.
Limitations and Future Work
A major limitation of the present study is the relatively small sample size, which decreases the findings’ statistical power, making them less robust against variation and more prone to errors. Despite the results of the LCA identifying three subgroups in the present population, it could be worthwhile to administer the MRCI to a larger cohort and investigate whether the current findings could be replicated. Likewise, greater precision regarding the Rasch model’s infit and outfit estimation could be obtained to better judge the fit of individual items. Also, the sample sizes of the pilot study and of the administration study at the delayed time point were relatively small despite great efforts to recruit more students. Hence, these limitations further call for a replication study with more students, potentially even from several different universities at various time points during their studies. Furthermore, the conclusions drawn from the pilot study must be interpreted cautiously regarding the revision of individual items, as they are based solely on a few participants. Thus, it cannot be excluded that conducting the study with more students might reveal the necessity of adjusting the wording of individual items.
Moreover, a general limitation of most concept inventories, including the MRCI, concerns the definition of the concepts in question. Even though an extensive map was established before test development, such frameworks often fail to encapsulate all aspects of a specific concept, and it cannot be excluded that certain wrong conceptions remained obscured. Also, even with an unlimited number of questions in such assessment tools, there still might be undetected differences in the level of understanding. Additionally, perfect test performance does not imply perfect understanding of the topic. However, the results of the implementation study demonstrate that the MRCI yields a reliable and valid estimation of students’ conceptual comprehension of randomness. Furthermore, the insights from think-aloud interviews suggest that the MRCI measures, in most cases, the understanding of the concept of stochasticity on a molecular level, thus supporting the developed concept map and the response validity of the MRCI. A further limitation is the nature of the self-reported self-efficacy values, which can comprise meta-cognitive biases that could lead to under- and overestimations of an individual’s performance (e.g., Nadler et al., 2015).
In future studies, it would be advantageous to assess the psychometric properties of the English version of the MRCI. Even though several people independently validated the translation, having empirical classroom data could help identify items that require fine-tuning. A validation of the translation by a professional might provide further linguistic support for the English version of the assessment. Additionally, as the students in the MRCI implementation study all belonged to the same cohort, replicating the study would allow for assessment of the MRCI’s test reliability in greater detail. As the current study is limited to first-year students, it will be interesting to see how the MRCI can be applied to other student cohorts. Whereas conducting the MRCI with biology doctoral students presents a first step in revealing insights regarding the upper limits of this concept inventory, the investigation of students’ performance in subsequent years of their university studies might give valuable insights regarding students’ ongoing development of a complete conceptual understanding. Eventually, the MRCI could be used to track students’ performance throughout their studies, including investigating the effects of individual courses or curricula on their understanding.
CONCLUSION
The MRCI constitutes the first diagnostic tool that enables a fine-grained analysis of students’ understanding of stochastic processes on a molecular level. It helps to tackle different misconceptions and unveil subconcepts of particular difficulty by covering a broad range of ideas. Furthermore, this tool might help students assess their learning progress, and lecturers or curriculum developers evaluate, plan, and design new courses or learning materials. Eventually, the application of the MRCI in a university classroom could reveal valuable insights into students’ conceptual understanding of the concept of stochasticity on a molecular level.
Accessing Materials
The data supporting this study’s findings, along with all materials and analysis scripts, are openly available (https://osf.io/yztj6/). The German and English versions of the MRCI are also available in the Supplemental Material.
ACKNOWLEDGMENTS
This project is funded by the Future Learning Initiative at ETH Zurich ( www.fli.ethz.ch). We thank the Learning Sciences and Higher Education research group members and Mike Klymkowsky for critically reading an early draft of the article. Further, we thank Professors Jacob Corn, Wolf-Dietrich Hardt, Karsten Weis, and Alex Widmer for supporting the study announcement to the classes, Professor Julia Vorholt-Zambelli and Carmen Fischer-Romero for supporting the recruitment of biology doctoral students, the biology and non-biology graduate students for taking the MRCI assessment and for correcting the MRCI translation, respectively, and the undergraduate students at ETH Zurich for participating in this study. All studies were approved by the Ethics Commission of ETH Zurich, Switzerland (2021-N-12 and 2021-N-142).