Using Students’ Concept-building Tendencies to Better Characterize Average-Performing Student Learning and Problem-Solving Approaches in General Chemistry
Abstract
We previously reported that students’ concept-building approaches, identified a priori using a cognitive psychology laboratory task, extend to learning complex science, technology, engineering, and mathematics topics. This prior study examined student performance in both general and organic chemistry at a select research institution, after accounting for preparation. We found that abstraction learners (defined cognitively as learning the theory underlying related examples) performed higher on course exams than exemplar learners (defined cognitively as learning by memorizing examples). In the present paper, we further examined this initial finding by studying a general chemistry course using a different pedagogical approach (process-oriented guided-inquiry learning) at an institution focused on health science majors, and then extended our studies via think-aloud interviews to probe the effect concept-building approaches have on problem-solving behaviors of average exam performance students. From interviews with students in the average-achieving group, using problems at three transfer levels, we found that: 1) abstraction learners outperformed exemplar learners at all problem levels; 2) abstraction learners relied on understanding and exemplar learners dominantly relied on an algorithm without understanding at all problem levels; and 3) both concept-building-approach students had weaknesses in their metacognitive monitoring accuracy skills, specifically their postperformance confidence level in their solution accuracy.
INTRODUCTION
Extensive science, technology, engineering, and mathematics (STEM) education research has explored the struggles of students in their introductory courses as they transition to college. The reasons students may struggle are complex and are dependent on cognitive factors, academic preparation, and social–psychological factors. Many national reports call for developing and implementing strategies to help all students succeed (Vision and Change, American Association for the Advancement of Science, 2011, 2015, 2018; AAU Undergraduate STEM Education Initiative, Association of American Universities, 2017; Engage to Excel, President’s Council of Advisors on Science and Technology, 2012; HHMI Inclusive Excellence Initiative, Howard Hughes Medical Institute, 2017). In response, the STEM education research community has studied many evidence-based strategies (e.g., Eberlein et al., 2008; Singer et al., 2012; Freeman et al., 2014; Arendale, 2017; Van Dusen et al., 2015). Studies have tested interventions and examined the effects on the performance of an entire class, and more recently the focus has included subgroups of students within a class (e.g., Shields et al., 2012; Hall et al., 2013; Eddy and Hogan, 2014; Batz et al., 2015; Connell et al., 2016; Barral et al., 2018). Other researchers have looked at the effect of affective characteristics and social identity on exam performance and retention in a class (e.g., Trujillo and Tanner, 2014; Jordt et al., 2017; Canning et al., 2018; Fink et al., 2018). Still other studies have examined how students solve problems, and whether students are understanding the concepts behind the problems or just solving the problems algorithmically (e.g., Jiménez-Aleixandre and Erduran, 2007; Hoskinson et al., 2013; Xu and Talanquer, 2012; Knight et al., 2015). Within these studies, when considering different teaching approaches and interventions, the effects are examined across an entire class (or identity-based subgroups), and generally these studies assume that individual differences in student achievement are a consequence of differential aptitude (e.g., math aptitude), prior preparation (e.g., high school AP courses), social identity, or a combination.
In this paper, we examined students’ individual differences in concept building as potentially one key factor in explaining student struggles and differing outcomes of otherwise similar students. We did so with two approaches. First, we investigated how this individual difference in concept building might predict course performance and grade distribution in a process-oriented guided-inquiry learning (POGIL)-based general chemistry course at an institution that focuses on health science majors (study 1). We then extended our understanding of these concept-building differences by sampling from one achievement group in general chemistry (the average-achievement group), and probing their problem-solving behavior through detailed think-aloud interviews (study 2). In the interviews, we also examined their metacognitive-monitoring ability to evaluate their solutions’ accuracy as a function of their concept-building approaches. By doing so, we revealed relationships between individual differences in concept building and the underlying knowledge that these students apply to a range of problems.
The topic we used in the think-aloud interviews (Lewis structures) is a component of structure and bonding, nonquantitative, and requires spatial and symbolic representations. Structure and bonding, as well as spatial and symbolic representations, are important in both biology and chemistry, and thus may allow us to generalize our findings to courses in biology and organic chemistry (e.g., Graulich, 2015; Hoskinson et al., 2013). In addition, focusing on Lewis structure problems allowed us to eliminate the possible contribution of math skills in solving a problem, a factor that could occur in many general chemistry problems (e.g., Frey et al., 2017; Ralph and Lewis, 2018).
Background
The key theoretical underpinning of this study is based on a learning framework from the cognitive science literature, developed by two of the coauthors (M.A.D. and M.J.C.) of the present study (McDaniel et al., 2014). The learning framework assumes that, for a given conceptual task, individual learners extract one of two qualitatively different representations: a representation primarily based on learning the individual training examples or a representation that extracts a more abstract summary of the critical features of the training examples. In a range of laboratory conceptual tasks—category learning (e.g., Craig and Lewandowsky, 2012; Little and McDaniel, 2015), function learning (McDaniel et al., 2014), multiple-cue prediction learning (Juslin et al., 2003; Hoffmann et al., 2014), and skill learning (Bourne et al., 2010)—recent evidence supports this major tenet that an individual learner relies predominantly on either an exemplar or an abstraction approach to learn a particular conceptual task. Generally, laboratory conceptual tasks require participants to learn to predict outcomes (or categorize) from particular combinations or quantities of perceptual features displayed in a set of simple or novel stimuli. For instance, based on a series of observations, participants try learn the relation between how much “Beros” (a fabricated element supposedly found on Mars) a Martian organism releases after absorbing a certain amount of “Zebon” (another fabricated element supposedly found on Mars; McDaniel et al., 2014). Another example of a laboratory conceptual task is where participants try to learn to predict the toxicity of a bug based on a multiplicative combination of perceptual features (leg length, antennae length, wings and the number of spots; Hoffmann et al., 2014). The innovative aspect of the present theoretical framework is the tenet that an individual’s concept-building approach tends to be relatively consistent across conceptual learning tasks, and this has been supported via laboratory-based concept-learning experiments (McDaniel et al., 2014). As developed in this paper, we recently reported a novel extension to the laboratory cognitive science findings, an extension that reveals the application of our concept-building framework to complex STEM learning.
In a series of studies conducted by a cross-disciplinary team of cognitive scientists, a chemistry discipline-based education researcher, and chemistry instructors, we (Frey et al., 2017; McDaniel et al., 2018) showed that students approach learning complex concepts in general chemistry and organic chemistry using two distinct concept-building approaches (exemplar or abstraction learners). These classroom studies expanded upon the initial cognitive science laboratory experiments (McDaniel et al., 2014) in which learners were classified as having one of two distinct concept-building approaches via a concept-building task (described in the Methods section). This task identifies learners as exemplar learners (defined cognitively as learning by memorizing examples), who rely extensively on memorized algorithms or examples to solve new test problems, and abstraction learners (defined cognitively as learning the underlying theory), who apply different levels of understanding of the underlying concept to solve new test problems.
We found that abstraction learners in general chemistry performed equal to exemplar learners on retention problems (course exam problems that were similar to those presented in class or homework), but performed better on far-transfer problems (course exam problems that were not similar to class or homework problems and required generalization) even after controlling for ACT Math (McDaniel et al., 2018). In Frey et al. (2017), we found that, in organic chemistry, abstraction learners performed 13 percentage points higher on exam average than exemplar learners, even after accounting for ACT composite scores and general chemistry performance. We also found that the concept-building approaches seem to be robust across time (at least for 1.5 years); that is, 85% of students who undertook the task using the same function in the Fall semester of General Chemistry I and the Spring semester of Organic Chemistry II consistently adopted the same concept-building approach. In the current study, we wanted to see whether a student’s concept-building approach also affected course performance and grade distribution in a general chemistry course using a different pedagogical approach (i.e., POGIL) at a different type of institution, namely a health professions–focused university.
More importantly, even though we have seen that abstraction learners outperform exemplar learners, we do not know exactly how these different learners solve problems. Therefore, in the current study, we examined via think-aloud interviews how abstraction versus exemplar students might solve retention, near-transfer, and far-transfer problems. We investigated whether students within the same achievement group differ in their concept-building approach, their conceptual understanding, and their approach to problem solving. In addition, because studies have shown that a student’s metacognitive-monitoring accuracy can affect his or her learning (e.g., Dunlosky and Rawson, 2012; Stanton et al., 2015), we probed students’ postperformance confidence in the accuracy of their solutions. We wanted to see whether these students in the same achievement group with different concept-building approaches might also differ in their metacognitive-monitoring accuracy.
In these think-aloud interviews, we focused on the student who is at the average in exam performance. The average-performing student seems the logical place to start in identifying how exemplar and abstraction learners might solve problems, because these students may demonstrate an incomplete, inadequate, or inconsistent approach to problem solving. They are trying, but perhaps they try by using the wrong approach or by not adequately implementing the correct approach to solving problems. In addition, even though abstraction learners outperform exemplar learners on average, are there abstraction learners who are performing at the average exam performance and, if so, why are they not performing at a higher success level? We anticipated that the think-aloud interviews might give us some insight into possible cognitive reasons.
Research Questions
We were interested in examining the generality of the Frey et al. (2017) results to a general chemistry course focused on health science majors and using a different pedagogical approach, specifically the POGIL approach (Moog, 2014; Simonson, 2019). We wanted to determine whether there was a difference in course performance and grade distribution for exemplar versus abstraction learners in this course. For the present purposes, we were most interested in the average-performing achievement group. An initial issue was whether the average-performing group contained both exemplar and abstraction learners, and if so, whether this group was more populated by exemplar than by abstraction learners. Having found both types of learners within the average-performing achievement group (i.e., specifically, students who have average exam performances), our central focus in this project was to illuminate possible differences in the problem-solving approaches between exemplar and abstraction learners who are average-performing students. Specifically, we explored the following research questions:
For study 1, we examined the performance difference between abstraction and exemplar learners in a POGIL-based general chemistry course at an institution focused on health science majors. Hence, the research questions for study 1 are focused on all of the students in the POGIL-based general chemistry course for health science majors.
Do abstraction learners outperform exemplar learners in a POGIL-based general chemistry course for health science majors?
Within the average-exam grade range, do the relative proportions of abstraction and exemplar learners differ?
In study 2, we looked more in depth at the different approaches to problem solving that are used by students who have average exam performances as a function of their concept-building tendency. To do so, we followed established methods in basic and discipline-based education research (DBER) problem-solving research by collecting detailed think-aloud protocols from a small number of participants (N = 11) as they solved three chemistry problems (the think-aloud method and typical sample size are described in a chemistry review by Herrington and Daubenmire, 2014).
We emphasize that the research questions in study 2 are restricted to students who have average exam performances. For retention, near-transfer, and far-transfer problems (we characterize these problem types in the Methods section),
Is there a difference in their problem-solving accuracy as a function of concept-building approach?
Is there a difference in their problem-solving approach as a function of concept-building approach?
Is there a difference in their calibration of postperformance confidence of the accuracy of their solutions, as a function of concept-building approach?
METHODS
General Study Methodology
Institutional Board Approval.
This project has been approved by the institutional review boards at St. Louis College of Pharmacy (IRB ID no. 2017-39) and Washington University in St. Louis (IRB ID no. 201710100).
Study Setting and Course Format.
Our study focused on students enrolled in a first-semester general chemistry course at a small health profession–focused school in the midwestern United States that confers undergraduate and graduate degrees. The course comprised two sections taught by different instructors, with 123 total students enrolled. The sections were managed as a single course with the same content, structure, and assignments. The course followed the POGIL approach (Moog, 2014; Simonson, 2019), with each class session centered on team-based solving of problems designed to promote learning concepts via exploration/interpretation of data and application of new knowledge. After each class, students were required to complete an online quiz on the day’s material before the next class. Additionally, weekly online quizzes were assigned each Friday and were due before the following Monday class. The course had four midterm exams and a cumulative final, all of which comprised multiple-choice questions (73.5%) and free-response problems (26.5%). The classification scheme from the McDaniel et al. (2018) study was modified, as described in the Problem Selection section, and used to characterize the transfer levels of the exam questions in this study. On average (across multiple-choice and free-response questions), 41, 54, and 5% of the exam questions were characterized as retention, near-transfer, and far-transfer questions, respectively.
Concept-Building Task.
To assess students’ concept-building approach (classifying them as either exemplar or abstraction learners), we administered the same concept-building task previously employed in McDaniel et al. (2014), Frey et al. (2017), and McDaniel et al. (2018), and we used the same statistical procedure to classify the students as exemplar or abstraction learners as used in the prior studies. This Web-based task involved a fictional organism and two fictional elements, so students had no prior knowledge about the task. The students were told to imagine that they had been hired by NASA to study a new organism found on Mars that absorbs an element called Zebon and releases an element called Beros. Specifically, students attempted to learn to predict an output variable (quantity of Beros released) based on an input variable (quantity of Zebon absorbed), in which (unknown to the students) these input–output points followed a specific function form (in the current study, an inverted-V function). During a training phase, students made output predictions on training inputs and learned the true outputs via feedback. For each training trial, student viewed an input (a bar representing the quantity of Zebon absorbed), predicted the output (adjusted a bar to predict the quantity of Beros released), and received feedback (a bar showing the correct quantity of Beros and text specifying the prediction error); see Figure 1 for a sample trial. The task was self-paced, and participants were given no instructions on how much time to spend on each trial.

FIGURE 1. Screen shots from the concept-building task. Adapted from Figure 2 in McDaniel, M. A., Cahill, M. J., Robbins, M., & Wiener, C., 2014, “Individual differences in learning and transfer: Stable tendencies for learning exemplars versus abstracting rules,” Journal of Experimental Psychology: General, 143(2), 668–693, publisher: American Psychological Association (adapted with permission).
Training involved repeated exposure to 20 unique input values (all the odd numbers between 61 and 99). Each training block presented each of these input values once, and the order of the inputs varied across blocks. After each block, participants saw their mean prediction error (the mean absolute error, MAE) for that block. Starting with block 2, they also saw their previous MAE and a message depending on whether they had reduced their error (either “Your accuracy IMPROVED. Keep up the good work!” or “Your accuracy DID NOT IMPROVE. Keep working to improve your predictions!”). All participants completed at least 10 training blocks (200 trials), at which point training ended for participants with MAE <10. Those who did not meet this threshold completed up to three additional training blocks, and training ended if MAE fell below 10 in either block 11 or block 12. Training ended after block 13 (trial 260) for all remaining participants, regardless of whether they met the threshold.
After training, all participants completed a 36-trial test phase in which they predicted the outputs for novel (untrained) inputs. The test procedure was identical to the training procedure, except that no feedback was provided. Instead, after making the prediction, participants saw a message that said “Prediction Recorded. Get ready for the next trial.” The test phase included 30 extrapolation trials, in which inputs were odd numbers outside the training domain (all odd numbers between 31 and 59 and between 101 and 129); it also included six interpolation trials, which were even numbers contained within the training domain (94, 80, 64, 88, 100, and 72). The students were allowed to take as long as they needed to finish the task; however, on average, the entire task (training and test trials) took participants approximately 40 minutes to complete.
Following the procedure in the prior studies, the concept-building classification was a two-step process. First, individuals with final training block MAE greater than or equal to 10 were classified as non-learners and were not included in this study. Further classification involved comparing remaining learners’ extrapolation MAEs to the extrapolation MAE from a simple exemplar model. Specifically, a simple exemplar model would predict flat extrapolation extending from the edges of the training domain (represented by the dashed horizontal lines in Figure 2). With the particular function used in this study, a simple exemplar model would make a prediction of 148 for every extrapolation trial, producing an MAE of 34.72. It is worth noting that any set of predictions that average 148 and never overestimate the output value produce the same MAE of 34.72. The extrapolation MAE and surrounding 95% confidence interval were calculated for each learner. If the upper limit of this interval was below 34.72 (i.e., the learner’s predictions were statistically significantly better than a simple exemplar model), the learner was classified as an abstraction learner. The assumption was that, to significantly outperform an exemplar model, learners must have extracted some rule-based information in learning during the training trials that they were able to use during extrapolation. Learners who did not significantly outperform the simple exemplar model were classified as exemplar learners, in which the assumption was that the exemplar learners apparently learned specific input–output associations but did not extract the function rule necessary to make predictions on the novel test inputs. Figure 2 shows the mean prediction on each extrapolation point for abstraction and exemplar learners, descriptively showing the diverging extrapolation patterns of the two groups. Abstraction learners were characterized by steeper extrapolation, closely following the function, whereas exemplar learners exhibited flatter extrapolation, particularly on the right side of the function.
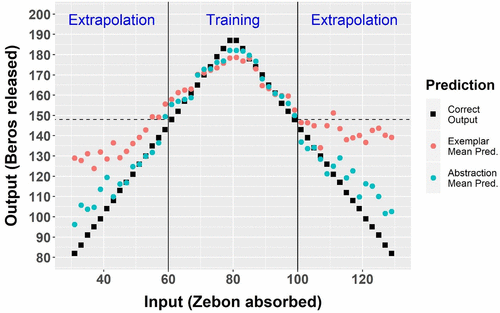
FIGURE 2. Input and mean output values from the final training block and extrapolation trials for the students in this study. The vertical lines represent the boundaries of the training range. The dashed horizontal lines represent theoretical exemplar-model predictions based on learning the outputs at the boundaries of the training range and predicting those same outputs during extrapolation. The black squares represent the correct output values, based on the function. The red circles represent the mean prediction across all exemplar learners for each input value. The blue circles represent the mean prediction across all abstraction learners for each input value.
Study 1 Methodology
Procedure.
During the Fall 2017 semester, all students in the course completed the concept-building task and a short survey during a 1-hour prelab period. The survey included questions for students to self-report their race, gender, and ACT/Scholastic Aptitude Test Math score. Completion of these tasks allowed students to drop their lowest laboratory-experiment grade. Although all students completed these tasks as part of a course activity, students chose whether to provide consent allowing their data to be used as part of this research study. After the semester, one of the course instructors of record (author M.D.P.) shared the course grade book, with non-consenters removed, with the research team so that exam scores could be extracted and combined with concept-building and survey data for analysis.
Sample.
One hundred eight out of 123 (87.8%) students consented to be in the study. Of these consenters, 54 (50.0%) were classified as abstraction learners, 28 (25.9%) were exemplar learners, 25 (23.1%) were non-learners, and 1 student did not complete enough of the task to be classified. Thus, our final sample for analysis included 82 students (54 abstraction learners and 28 exemplar learners). Of the final sample, 44 (55%) were female. In terms of race, 58 (73%) were white, 13 (16%) were Asian, 5 (6%) were African American, and 4 (5%) were from other racial groups.
Analysis Overview.
Primary data analysis involved two analyses of variance (ANOVAs) and a Wilcoxon rank-sum test for the grade distribution. One ANOVA included concept building (abstraction vs. exemplar) as the sole independent variable and final exam score as the dependent variable. The second analysis examined unit exam scores and was a mixed ANOVA with concept building as a between-subjects factor and exam number (1–4) as a within-subjects factor. To examine the difference in the grade distributions, we used the Wilcoxon rank-sum test with concept-building approach as the independent variable. Analyses were conducted with R (v. 3.6.0; R Core Team, 2019), using the aov car function from the afex package (v. 0.25-1; Singmann et al., 2019). As is the default for aov_car (but not aov from base R), all analyses were conducted with orthogonal contrasts (c(“contr.sum”, “contr.poly”)) and type III sums of squares for factorial design.
Study 2 Methodology
Think-Aloud Procedure.
For our research questions in study 2, we used a think-aloud protocol to interview a sample of students having an average exam performance in the class after exam 3. We used a protocol analysis for the interview, in which the students provided verbal reports of their thoughts as they solved a task (Ericsson and Simon, 1993; Ericsson, 2006; Bowen, 1994; Herrington and Daubenmire, 2014).
The think-aloud problems were on Lewis structures, which were covered in exams 2 and 3. The interviews took place approximately 2 weeks after the third exam, approximately mid-November, on two sequential days at the same Midwest institution by M.D.P. or D.M.B. M.D.P.’s students were interviewed by D.M.B. and the other instructor’s students were interviewed by M.D.P. The interviews took approximately 1 hour, and students were paid for their participation.
Student Selection for the Think-Aloud Interviews.
For the think-aloud interviews, we sought to select 10–12 students who were likely to receive an average exam performance in the course. The use of this small number of participants (N = 11) in think-aloud interviews for problem-solving research follows established basic cognitive science and DBER methods (e.g., Herrington and Daubenmire, 2014). Several recent examples underscore the prevalence of such sample sizes in think-aloud DBER research. Petterson et al. (2020) studied 13 college students’ reasoning while solving organic chemistry reaction problems using one of two modalities (paper and pencil; computer app). These 13 students were divided into two groups (six used paper and pencil, and seven used a computer app) to examine whether modality affected reasoning processes when solving two acid/base reaction problems. In Webber and Flynn (2018), 11 students from an organic chemistry course participated in think-aloud interviews to probe the strategies they used in solving familiar and unfamiliar organic chemistry mechanism problems. Xue and Stains (2020) interviewed six students in an organic chemistry course to explore students’ understanding of resonance and how that understanding is related to course instruction.
Using purposeful sampling (Patton, 2002; Creswell, 2007), we selected students based on the fact that their exam average across exams 1–3 fell within a target range. Data from previous semesters of this course revealed that, for students who eventually earned a “C” in the course, the exam average across exams 1–3 was 72%, so we centered the target range around 72%. We had the goal of targeting approximately 20 students, so we expanded the range out in both directions from 72% until this number was met. This process led us to target students whose average on exams 1–3 was between 66% and 78%, with the intent to recruit approximately equal numbers of exemplar and abstraction learners. There were 20 consenting students in our target performance range: 11 abstraction learners, eight exemplar learners, and one non-learner. We recruited all eight exemplar learners and eight of the abstraction learners, with the goal that five or six from each group would participate. For the abstraction learners, we selected the eight recruits by 1) selecting all of the female abstraction learners, a total of three (seven of eight exemplar learners were female), and 2) selecting five of the eight males based on responses to another survey measure (Modified Approaches and Study Skills Inventory [M-ASSIST]), which is a self-report of students’ use of deep- and surface-learning strategies (Bunce et al., 2017). We selected these males to obtain the broadest range of M-ASSIST patterns among abstraction learners. We emailed invitation letters to these eight abstraction learners and all eight exemplar learners, offering $20 for approximately 1 hour of their time, and 12 students (six exemplar and six abstraction) agreed to participate. One student (an exemplar learner) displayed anxiety during the problem-solving session, digressed from the problems, and did not finish the problems. This interview was not transcribed or analyzed, and thus the final sample consisted of six abstraction and five exemplar learners. All interviewed students completed the course. At the end of the course, for their exam score average (as used in the grade distributions in the Results and Discussion section), six received a “C” and five were in the “D”/”F” range. Hence, we interviewed 31% of the available students in the “C” and “D”/”F” ranges.
It is important to note that this selection process was managed entirely by M.J.C. (PhD psychologist, research scientist, and a nonchemist). Not at any time during the interviewing or coding were the interviewers (D.M.B. and M.D.P.) or the coders (R.F.F., D.M.B., and M.D.P.) aware of the concept-building approaches or the course exam scores (beyond that the scores were in the average-performing range) of the interviewed students. In addition, the coders were given de-identified transcripts and therefore were not aware of any demographic information about the students.
Problem Selection.
The think-aloud interviews consisted of three problems on Lewis structure. We selected Lewis structure problems because this topic was covered extensively in this course, being tested on both exams 2 and 3, which allowed us to obtain enough performance data to determine which students were performing at the exam average of the class. In addition, it is a topic that is not mathematically based, which allowed for the possibility of a richer discussion during the think-aloud interviews.
We selected Lewis structure problems (see Table 1) at three different transfer levels: retention, near transfer, and far transfer. We modified the rubric in the McDaniel et al. (2018) study, which was designed to categorize exam questions in general chemistry. The definitions of the three problem levels and the details of the problems used in the current study follow. 1) A retention problem is a problem that has the same structure to previously exposed problems (either in class or homework) and is solved with the same method. For this study, the retention problem was an exact molecule the students had seen in class. 2) A near-transfer problem is a problem that is similar to a problem that the student has previously been exposed to, and the solution is similar to the previous exposure, but it is a new situation. For this study, the molecule was similar to but was not a molecule seen in lecture or homework, and it could be solved using the general algorithm for Lewis structures. This characterization and construction of a near-transfer problem parallels that of DBER work in math, in which near-transfer problems in geometry were based on similar but not identical geometric renderings provided in instruction and could be solved using the previously instructed theorems (Wong et al., 2002). 3) A far-transfer problem is a problem that involves previously exposed concepts but cannot be solved using methods similar to those used to solve prior homework problems. Instead, the student must understand the underlying concept(s) and be able to generate the solution either by applying a concept in a new way or integrating across concepts. This characterization also parallels Wong et al. (2002), whose far-transfer problems required integration of some previously studied theorems and at least one new theorem or involved new constructions. Regarding cognitive theory, this orientation has parallels to the Barnett and Ceci (2002) taxonomy of transfer, in which the content of what is transferred is considered in characterizing the degree of transfer—near versus far. Note, however, that unlike Barnett and Ceci, our and Wong and colleagues’ scheme does not assume that far transfer requires that the knowledge domain change across problems. For the current study, the molecule was a cyclic molecule that was not seen in lecture or homework. Although cyclic molecules were introduced in lecture, Lewis structures for cyclic molecules do not follow an algorithm, and therefore students need to understand the underlying principles for Lewis structures in order to solve the problem correctly.
Directions: Draw the most preferred Lewis structure that obeys the octet rule for the molecule given below (connectivity for each is shown). If there are equivalent resonance structures, please draw all of them. Show all lone pairs and all non-zero formal charges for non-hydrogen atoms. Please circle all structures to be graded. | ||
Retention | Near-transfer | Far-transfer |
CO2; connectivity is [O−C−O] | ClONO2 | C5ON2H4 (Note: Number of valence electrons = 40) |
![]() | ![]() |
Grading of Problems.
Because of their long-time experience teaching Lewis structures in general chemistry, R.F.F. and M.D.P. developed and classified the transfer level of the problems based on the homework given and lecture material in the course during the study year. They also developed the solution key, and M.D.P. assessed the accuracy of student solutions on a 0–3 scale based on the following scheme: 3 = no mistakes (we have termed this as “correct”); 2 = 1 mistake (termed as “partially correct”); 1 = 2 mistakes (termed as “partially incorrect”); and 0 = 3 or more mistakes (termed as “incorrect”).
Structure of Think-Aloud Interviews.
Students completed a think-aloud interview designed specifically for the current study; we followed the standard think-aloud procedure for chemical education research as outlined in Bowen (1994), which describes in detail the interview process and the type of probing questions that are asked to elicit student’s thoughts without leading them in the solution of the problem. In addition to the think-aloud protocol questions, we added additional questions about the student’s level of postperformance confidence in his or her answer. During this interview, each problem was on a separate piece of paper and color coded, and a periodic table was available for the student to use to determine the number of electrons. The prompt was the same on each page (see Table 1). Students were encouraged to draw their structures on the papers as they thought out loud, describing what they were doing to solve the problem. The interviews were audio-recorded, and the completed solutions were saved and scanned.
When the student entered the room, the interviewer used an IRB-approved script (see Supplemental Material) and then started the interview with a warm-up think-aloud exercise, which consisted of assembling s’mores. The problems were given separately in the following order: retention, near-transfer, and far-transfer. The interviewer’s questions were confined to probing questions only; for example, “What are you thinking?” and “Why did you write that?” At the end of each problem (i.e., postperformance), the interviewer asked, “How confident are you that your answer is correct?,” and then asked, “On a scale of 1–5, with 1 being very confident and 5 being very not confident, what number would you give?” For a more complete set of questions, see the Supplemental Material.
Development of the Codebook.
We used a generative process to interpret the think-aloud interviews (Clement, 2000), one in which data are systematically analyzed to identify and construct categories to answer specific research questions. Following the process described in Merriam and Tisdell (2015), the codes were developed using the constant comparative method of qualitative data analysis (Glaser and Strauss, 1967). To ensure the consistency and dependability of the process, we conducted an audit trail of our process (Merriam and Tisdell, 2015; Miles et al., 2020) as described in more detail later.
The interviews were transcribed verbatim, and the transcriptions were coded using the following process. The initial codebook was generated, based on the research questions, by all five researchers (R.F.F., M.D.P., D.M.B., M.A.D., and M.J.C.) as they worked through two transcripts and iteratively developed and applied initial codes and updated the codebook when gaps in the codebook were identified during application. This initial coding was for the purpose of generating the codebook and began with open coding for the following key ideas: 1) the approach the student used to solve the Lewis structure problem and 2) the types of mistakes made by the student. On the metacognitive-monitoring accuracy question (i.e., the level of postperformance confidence the student had in the correctness of his or her answer), the student rated his or her confidence on a scale from 1 [very confident] to 5 [not very confident]). Hence, this answer did not have to be coded; we later binned the scale into low confidence, medium confidence, and high confidence (see end of Methods section). (We then asked and coded for resources the student would use if having difficulty solving the problem and why; this coding is outside the current paper’s scope and is not reported here.) During this initial generation of the codebook, it became obvious that most students did not understand the resonance question component of the prompt. Because this component was not an important element in our research questions, we decided to focus our analysis only on the most-preferred Lewis structure the student chose and not on the resonance structures (although for consistency of the coding process, we continued to code the portions of the transcript related to resonance structures).
After the codebook was generated, all transcripts were coded and discussed by the three chemistry members of the research team (R.F.F., M.D.P., and D.M.B.). To check and refine the codebook, we coded the transcripts in four separate rounds. In each round, M.J.C. selected each set and made it available to the three coders. The transcripts were coded independently by at least one of the authors (R.F.F., M.D.P., and D.M.B., who are PhD chemists and have taught general chemistry) and then group discussions were held in which these three authors deliberated about all coding in every transcript to resolve any discrepancies by group consensus (Saldaña, 2015). When necessary, the codebook was refined. This coding process was repeated for each of the three rounds (2–4) on separate dates, with saturation of coding changes occurring by the third meeting. Coding was conducted via Comments in Microsoft Word.
Coders were blind to the student’s concept-building approach, removing this potentially concerning source of bias. The transcripts did not contain any identifying information, but D.M.B. and M.D.P. likely were not completely blind to students for transcripts of interviews that they conducted. The interviewers’ familiarity with the students they interviewed potentially could have introduced bias into their coding. That is, the interviewers might have remembered things about the students or the interactions themselves that influenced their interpretations of the transcripts. Although potentially influential, the influence of this kind of bias was mitigated by the team-based, group-consensus coding process. Even if an interviewer initially coded one of his or her own interviews, the final codes resulted from discussions of three coders, two of whom had no familiarity with the student or interview beyond what was in the transcript and on the problem-solving sheet.
Subsequently, M.J.C. inputted the documents into NVivo v. 12 (QSR International, 2018) converting the comments into nodes. As he did so, he did a final check for potential uncertainties in the coding. The main uncertainty was that for one problem in two students’ protocols, two approaches were coded; whereas, for every other problem and student, only one approach was evident (and coded). These two problems were reviewed by the three-member coding team, and the team agreed to assign the one approach code that captured the approach leading to the attempted solution.
There were four approaches students used to solve the Lewis structure problems: 1) memory of an answer or memory of a related problem; 2) algorithm without understanding; 3) algorithm with understanding; and 4) reasoning using their understanding of concepts, not tied to an algorithm. These four approaches were valid for all three problem levels; see Table 2 for the descriptions of the four approaches and Supplemental Table S1 for example quotes. For the students’ postperformance confidence level of their solutions accuracy, we binned the student responses as reflecting high (1 to < 2.5), medium (2.5 to < 3.5), or low (3.5 to 5) confidence.
Type of approach | Approach description |
---|---|
Memory of an answer or related problem | The student does not follow any set of steps or cannot explain what he or she is doing; student just starts drawing a “completed” Lewis structure. |
Reasoning, not tied to a specific algorithm | The student tries to use the underlying principles for drawing the most preferred Lewis structure and explain the concepts behind the steps takes, but is not following any specific steps in an algorithm. |
Algorithm without understanding | The student tries to use the general algorithm for drawing the most preferred Lewis structure, but either does not explain the concepts behind the steps taken or explanations are completely incorrect. |
Algorithm with understanding | The student tries to use the general algorithm for drawing the most preferred Lewis structure and tries to explain the concepts behind the steps taken. |
Analysis.
Primary outcomes of interest in study 2 were problem-solving accuracy (determined by scoring the scanned problem sheets), accuracy confidence (binned into three confidence levels), and problem-solving approach (determined via coding of transcripts). Analyses focused on examining the variation of these outcomes across concept-building approach and problem-transfer level. After transcripts and codes were uploaded to NVivo, cases were created linking each transcript to a student. This allowed the coded transcripts to be linked to student-level information, including concept-building approach. Each transcript was also given three overarching nodes, each covering the entirety of one problem (retention, near transfer, or far transfer), allowing the frequency of codes to be separated by problem type. Analyses were descriptive in nature, examining how solution accuracy level, problem-solving approach, and accuracy confidence level vary across both concept-building approach and transfer level.
To study the students’ metacognitive monitoring of the accuracy of their solutions, we analyzed students’ postperformance accuracy confidence as a function of concept-building tendency (abstraction, exemplar) and type of problem. Due to the small sample size, we focused on characterizing the matches or mismatches between confidence ratings and actual solution accuracy, rather than computing correlations between the confidence ratings and solution accuracy scores. Responses for both accuracy confidence and solution accuracy were rescaled with the lowest possible value set to 0 and the highest possible value set to 1 for each measurement type. The binned accuracy confidence values were rescaled to 0 (low), 0.5 (medium), and 1 (high). The solution accuracy values were rescaled to 0 (incorrect), 0.33 (mostly incorrect), 0.67 (mostly correct), and 1 (correct). Using the rescaled values for both measures, we determined the calibration comparisons as follows: 1) if the student’s confidence was higher than his or her solution accuracy by more than 0.25, we denoted the calibration as overconfident; 2) if a student’s confidence was within 0.25 of his or her solution accuracy, we denoted the calibration as accurate; and 3) if a student’s confidence was lower than his or her solution accuracy by more than 0.25, we denoted the calibration as underconfident.
RESULTS AND DISCUSSION
Study 1 Results and Discussion
Having identified students’ concept-building approaches, we examined the association between a student’s concept-building approach and course exam performance for this POGIL course. Abstraction learners (N = 54) tended to perform better than exemplar learners (N = 28) on every exam and on their average exam performance (overall: M = 83.57, SE = 2.16, and M = 78.77, SE = 3.61, respectively); see Supplemental Material for Supplemental Figure S1 and more detail about this analysis. The mixed ANOVA on students’ exam 1–4 scores indicated that the overall advantage for abstraction learners was not significant (F(1, 79) = 2.78, MSE = 612.39, p = 0.10, ηp2 = 0.034). The ANOVA on the cumulative final exam scores showed again that abstraction learners (M = 74.37, SE = 2.17) scored nominally but not significantly better than exemplar learners (M = 72.12, SE = 3.19; F(1,80) = 0.353, p = 0.554).
Though the current performance differences between abstraction learners and exemplar learners for this POGIL health science–oriented general chemistry course are not statistically different, they do show descriptively similar (and even slightly stronger) patterns compared with our previous report (Frey et al., 2017) that examined course performance in a first-semester college-level general chemistry course; the mean difference in exam performance was 4.80 versus 4.32 in the previous report, and effect size was ηp2 = 0.036 versus 0.02 in the previous report. Recently, researchers (Wilson et al., 2020) have made the strong case that effect size is more informative when discussing replication or generalization of a previous study, and on this index, the current study has successfully generalized the previous study.
Two possibilities for the current study not reaching statistical significance are the difference in the exams between this study and the Frey et al. (2017) study, and the difference in the statistical power between the two studies. The exams in the two studies differed in format and in problem-transfer level. Regarding format, the exams in the current study consisted of mostly multiple-choice questions (75%) and some free-response questions (25%), whereas the exams in the previous study were largely free response with short-answer justifications (87%) and just a few multiple-choice (8%) and true-false (4%) questions. In addition, the exams in the current study contained fewer far-transfer questions than the previous study (5% vs. 36%, respectively). (Note: Although we had characterized these exams during the original study, this is the first report of these data concerning the exams in the previous study.) Consequently, because the exams in the current study contained fewer free-response questions, or they contained far fewer far-transfer questions, or due to both these reasons, the differences across the exemplar and abstraction learners may have been attenuated.
Alternatively, the similarity of the effect sizes in the two studies suggests that a more likely reason for the current study not finding statistical differences rests on the much greater power in the Frey et al. (2017) study (N = 470) than in the current sample (N = 82). Using the effect size ηp2 = 0.02, as obtained in the previous study (which is slightly smaller than the current study), achieving 0.8 power to obtain significance would require 387 participants (G*Power 3.1.9.4; Faul et al., 2007).
More aligned with the goals of the current study, in a more fine-grained analysis, we tabulated the average exam grade distributions (i.e., all four exams and the cumulative final weighted appropriately) for abstraction and exemplar learners. Though the distributions of abstraction and exemplar learners were not significantly different from one another, as revealed by Wilcoxon rank-sum test (W = 887, p = 0.18), Figure 3 reveals potentially important descriptive differences in these distributions. Interestingly, the percentages of abstraction and exemplar learners that populated the “A” and “B” grade ranges were similar (59% vs. 50%, respectively), but divergences in concept-building building approaches were manifested in the lower exam grade ranges (“C”–”F”). As seen in Figure 3, of the abstraction learners in the “C”–”F” exam grade range, 73% are in the “C” range; by contrast, of the exemplar learners in the “C”–”F” exam grade range, only 36% of them are in the “C” range (64% are in the “D”–”F” exam grade range). Viewed another way, only 27% of the abstraction learners in the “C”–”F” exam-grade range dropped to the “D”/”F” categories. At these lower achievement levels, the knowledge representations of the abstraction learners confer advantages. Next, we elaborate on the possible advantages of abstraction learners’ knowledge representations and then report a second study to inform that theoretical interpretation.
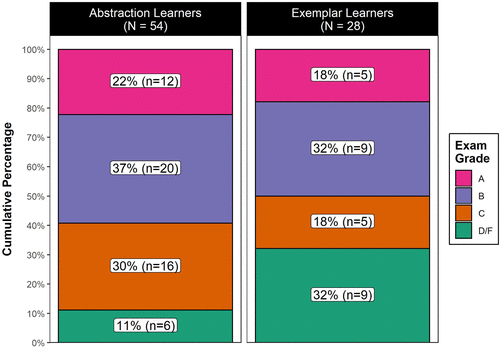
FIGURE 3. The average exam grade distribution for abstraction and exemplar learners. The height of each rectangle represents the proportion of the given concept-building group performing at each exam grade level.
The general interpretation of the performance difference between abstraction learners and exemplar learners offered in Frey et al. (2017) was that science courses focus on complex problem solving. The basic cognitive science work suggests that the more abstract the representations of problem-solving knowledge the learner has (i.e., general principles and concepts that apply to and reflect particular example problems and solutions), the more likely the learner will succeed at solving new problems in that domain (e.g., Gick and Holyoak, 1980; Novick, 1988). Hence, given exemplar learners’ reliance on memorized problems and solutions, it would be expected that exemplar learners would have a more difficult time than abstraction learners. This should especially be the case for assessments (exams) that present problems requiring generalization and transfer from training problems (e.g., homework).
McDaniel et al. (2018) provided initial evidence for this expectation. They reported that for chemistry exam items that relied on retention of homework and in-class problems, abstraction and exemplar learners performed at similar levels. In contrast, for chemistry exam items that required transfer from previous examples, abstraction learners demonstrated higher performance levels than example learners. The implication is that abstraction learners were relying on abstractions and general concepts gleaned from instructed problems, whereas exemplar learners were relying on memorized solutions to instructed problems. Critically, however, this interpretation has not yet been directly evaluated. To do so, techniques to reveal the underlying representations and processes that learners access to solve new problems are needed. Study 2 applied a think-aloud methodology to examine just those aspects of abstraction and exemplar learners’ approaches to solving new chemistry problems.
Study 2 Results and Discussion
In these results, we report the kinds of approaches that students adopted to solve the test problems, as gleaned from the think-aloud protocols, and then examine the accuracy of the students’ solutions to the retention, near-transfer, and far-transfer problems in two ways. First, we focus on both mean accuracy and the distribution of the accuracy scores as a function of the concept-building approach. We also look at the types of mistakes that students made to see whether any patterns could be gleaned from the think-aloud protocols. Finally, we consider students’ postperformance confidence in the accuracy of their solutions and relate their rated accuracy confidence levels to actual performances.
Problem-Solving Approaches.
The basic theoretical and empirical work in cognitive science has established several kinds of approaches that characterize how people solve problems. One prominent approach is to rely on memory for solutions from previously experienced problems (e.g., Gick and Holyoak, 1983; Gick and McGarry, 1992; Ross, 1984; Novick, 1988). Another approach is to apply an algorithm developed from experience and practice with previous problems (e.g., Gick and Holyoak, 1983; McDaniel and Schlager, 1990). A third is to use general strategies or reasoning (e.g., means-ends analysis; Atwood and Polson, 1976). From the think-aloud transcripts, we found evidence for each of these general approaches in solving the target chemistry problems. As expected for the retention problem, some students relied on memory for a previous solution/problem that they had seen (see Figure 4). By contrast, for the near- and far-transfer problems, students did not mention a particular previous problem; instead they mostly applied a learned algorithm relating to Lewis structures. For the far-transfer problem, some use of general reasoning based on understanding the underlying principles was sprinkled in with application of an algorithm.

FIGURE 4. General problem-solving approaches used by students in the think-aloud interviews. Each set of squares and arrows represents a single student’s dominant approach on each problem as the student progresses from retention to near-transfer to far-transfer problems.
A major objective of this study was to inform potential differences in the approaches (and by extension, knowledge) that exemplar learners and abstraction learners brought to bear when solving the target chemistry problems. Two general trends were evident on inspection of Figure 4. First, for the retention problem, all of the abstraction students applied an algorithm, but only three of the five exemplar students did so. This is because exemplar students were nearly as likely to rely on memory for a previously seen problem as they were to rely on an algorithm. Notably, this pattern dovetails with the a priori characterization of these abstraction learners as favoring abstraction versus exemplar learning tendencies for concept building. Moreover, this finding reinforces the theoretical idea motivating this study: Different concept-building approaches (and resulting knowledge representations) may support relatively equivalent performance on retention problems (see Figure 4), despite important differences in the underlying cognitive processes recruited to solve retention problems.
Moving to the transfer problems, the clear distinction was that the abstraction students were more likely than not to show understanding of the algorithm as they attempted to apply it, whereas the exemplar students, while uniformly indicating use of an algorithm, did so without understanding. In other words, the exemplar-oriented students seemed to have memorized an algorithm without the understanding of why it generally applied (i.e., they could not figure out how to map the algorithm to the transfer problems; cf. Novick, 1988, in the basic problem-solving literature).
Problem-Solving Accuracy.
Figure 5 shows the distribution of accuracy scores and the average score (0–3 scale) across the five exemplar and the six abstraction learners for each problem. The first point is that accuracy declines across the retention, near-transfer, and far-transfer problems. This finding reinforces the a priori selection of the problems to reflect tests of retention, near transfer, and far transfer, respectively. With this “continuum” in mind, the patterns across the two concept-building approaches are quite revealing. For the retention problem, abstraction learners demonstrated somewhat higher accuracy in their solutions than did the exemplar learners. This advantage displayed by abstraction learners was augmented in the near-transfer problem and became substantial in the far-transfer problem. For that problem, exemplar learners’ solution accuracy was near zero; by contrast, abstraction learners displayed partially accurate solutions.

FIGURE 5. Accuracy of solutions (0–3 scale) for students in the think-aloud interviews across the different transfer levels and as a function of concept-building approach (exemplar vs. abstraction). The height of each rectangle represents the number of students at each level of correctness (for the given problem and concept-building group). The number above each bar is the mean accuracy computed from the numeric values listed in the legend. For example, the left-most bar has five scores of 3 (termed correct) and one score of 1 (termed partially incorrect). The mean of these six scores comes out to 2.67.
These observations are strengthened and refined by examining the distributions of scores on each problem for each type of learner, as displayed in Figure 5. For the retention problem, nearly all abstraction learners (83%) derived the correct solution, resulting in an average accuracy score (i.e., average number of errors) of 2.67 out of 3, whereas the exemplar learners were more likely to derive a solution that was partially correct, with an average accuracy score of 1.80. For the near-transfer problem, abstraction learners’ solutions generally were partially correct, with an average score of 1.83, but almost all of the exemplar learners’ solutions (80%) were partially incorrect, resulting in an average accuracy score of 0.80. An even greater difference in the solutions emerged for the far-transfer problem. Abstraction learners (67%) were still partially correct, with an average score of 1.67, and now exemplar learners (80%) largely produced completely incorrect solutions, with an average score of 0.20. However, it should be noted that none of these students produced a totally correct solution (i.e., received a score of 3, which denotes zero errors) for the far-transfer problem.
Types of Mistakes.
A second way we characterized solution accuracy was to look at the type of mistakes that the average exam performing students made in their incorrect solutions. In this analysis, we did not find major differences between the abstraction and exemplar learners. From the coding, we discovered three main categories of mistakes: 1) applying an incorrect algorithm, 2) misapplying a correct algorithm, and 3) misunderstanding underlying concepts. Table 3 contains more in-depth descriptions of these three categories. Of the mistakes made by abstraction learners, 11% were made in the “applying an incorrect algorithm” category and 22% were made in the “misapplying a correct algorithm” category; whereas, of the mistakes made by exemplar learners, 18% were made in the “applying an incorrect algorithm” category and 9% were made in the “misapplying a correct algorithm” category. For both learner types, the most mistakes were in the “misunderstanding underlying concepts” category, which included 67% of the mistakes made by abstraction learners and 73% of the mistakes made by exemplar learners.
Type of mistake | Approach description |
---|---|
Applying an incorrect algorithm | The student is attempting to apply a procedure (or algorithm), but either the majority of the steps are not in the correct order or a significant number of steps are missing from the algorithm. |
Misapplying a correct algorithm | The student is using a procedure (or algorithm) and has the majority of steps and is applying them in the correct order, but either missed a step or made a mistake in one or two steps. |
Misunderstanding underlying concepts | The student either 1) is not using an algorithm and is attempting to reason through the process to obtain a correct Lewis structure, or 2) is using a correct algorithm and makes a conceptual error when attempting to explain the reasoning behind the steps taken. During this reasoning process, the student makes a critical error in some underlying concept. |
In addition, in the “misunderstanding underlying concepts” category, we coded the types of conceptual misunderstandings students made. Again, we did not find major differences between abstraction and exemplar learners. There were two key types of conceptual misunderstandings: 1) using the octet rule incorrectly, usually allowing more than an octet (four and six instances for abstraction learners and exemplar learners, respectively), and 2) using formal charges incorrectly, either assuming the formal charges are zero, miscalculating them, or calculating them correctly but not using them correctly to determine the most preferred structure (three and two instances for abstraction and exemplar learners, respectively). The fact that the most mistakes were in the “misunderstanding underlying concepts” category might be the reason all of these students are in the average-performing group. Whether the student is learning by examples and therefore does not know the underlying concept or the student is attempting to learn the underlying concept but is missing principal elements of the concept, both types of students are missing key knowledge about the target concept and thus are not performing at a successful level, especially on transfer problems.
Metacognitive Monitoring for Solution Accuracy.
Figure 6 contains the rescaled values of students’ postperformance accuracy confidence and the corresponding solution accuracy as a function of concept-building tendency (abstraction, exemplar) and type of problem. In Figure 6, we denoted the calibration comparisons between the rescaled values of accuracy confidence and the solution accuracy by using circles of purple, magenta, and green representing overconfident, accurate, and underconfident, respectively. There are three key observations.

FIGURE 6. Student accuracy confidence for their solutions and actual accuracy of their solutions as a function of concept-building tendency (abstraction, exemplar) and type of problem for the students in the think-aloud interviews. Both solution accuracy and accuracy confidence measures are rescaled with the corresponding lowest value set to 0 and highest value set to 1. Possible rescaled binned values for accuracy confidence are low (0.0), medium (0.5), and high (1.0). Possible rescaled values for solution accuracy are incorrect (0.00), partially incorrect (0.33), partially correct (0.67), and correct (1.00). The calibration comparison of solution accuracy and accuracy confidence are denoted by the circles of purple, magenta, and green representing overconfident, accurate, and underconfident, respectively.
The first observation is that, for the majority of the problem solutions (66% of the solutions for both abstraction and exemplar learners), the students’ solution accuracy confidence ratings did not match their corresponding solution accuracy. Of these confidence ratings, the majority were higher than the solution accuracy (75% for abstraction learners, 91% for exemplar learners), with a minority being lower than the solution accuracy (25% for abstraction learners, 9% for exemplar learners). Turning to the 33% of problem solutions for which students’ postperformance confidence rating accurately gauged solution accuracy (i.e., matched solution accuracy), the majority were for the retention problems (6/11 [55%] of the retention problems).
The second key observation is that six of the 11 students (55% of the students) did not modify their postperformance accuracy confidence ratings across the three transfer levels of problems even though their solution accuracies did change (i.e., their solution accuracy confidence was the same for their retention, near-transfer, and far-transfer solutions). One exception was a student (E1) who showed fairly accurate tracking across problems.
The third key observation concerns the misalignment of the postperformance accuracy confidence rating with the solution accuracy for the far-transfer problem. Recall that the far-transfer problem, as one would have expected, produced low solution accuracy, and importantly, no student produced a correct solution. However, eight out of the 11 students (73%) had higher confidence ratings for their solutions than the actual accuracy of their solutions. The three exceptions to this pattern were either students (A4 and E1) with confidence ratings that accurately tracked actual performance for this problem or a student (A6) with lower confidence relative to his/her solution accuracy. (Note that Student A6 was also underconfident in his/her solution accuracy on the retention problem.)
In general, then, this sample of average-achieving students demonstrated low metacognitive-monitoring accuracy on the transfer problems. Despite failing to arrive at correct solutions, students were fairly confident, postperformance, that their solutions were correct. As discussed later, it is perhaps this inaccuracy in metacognitive monitoring that obscures for the average-achieving student the gap in what they know and what they need to know to fare well in the chemistry course. That said, this may be a point for intervention, especially for the abstraction students, who are striving for conceptual understanding but may not be aware that their understanding is incomplete (as shown by their less than accurate solutions on the near- and far-transfer problems).
GENERAL DISCUSSION AND IMPLICATIONS
We examined students’ individual differences in concept building as potentially one key factor in explaining student achievement in STEM courses (in this case, a POGIL-based general chemistry for health science majors) and differing outcomes of students with similar academic preparation. Specifically, we examined the effect of concept-building approach on exam performance and grade distribution, and then extended our understanding of these approaches by probing the problem-solving behavior and metacognitive-monitoring accuracy of average-performing students through think-aloud interviews. We believe these results are of importance and generalizable to both biology and chemistry, because the topic we probed is Lewis structures: a component of structure and bonding, nonquantitative, and requiring spatial and symbolic representations—all constructs important to the learning of both biology and chemistry.
In study 1, we assessed the students’ concept-building approaches using the same laboratory-based concept-building task previously employed in a similar study with general chemistry and organic chemistry courses at a select research-intensive institution (Frey et al., 2017). In investigating the effect of concept-building approach on course performance, both studies showed modest advantages (with small effect sizes) for abstraction learners compared with exemplar learners in exam averages. However, the difference in exam performances between abstraction and exemplar learners was not statistically significant in the present study (unlike in the previous study), possibly due to the differences in exam format or percentage of far-transfer questions. However, the most likely reason is the relatively small sample size in the current study (the previous study’s sample had more than 450 students). The effect sizes were very comparable (in fact, the current study had a slightly higher effect size), and there is recent literature (Wilson et al., 2020) that suggests that effect size is the best index when looking at replication or generalization of previous findings. Hence, the value of our finding is establishing along with Frey et al. (2017), by at least one statistical index, the generality of the result that concept-building approaches derived from basic cognitive science work and assessed with laboratory learning tasks (McDaniel et al., 2014) relate to general chemistry course performances across different institutions with different instructors and different curricula.
The current study also revealed an interesting pattern regarding the grade distributions across the abstraction and exemplar learners, although the group difference in these distributions did not reach statistical significance. The percentages of abstraction and exemplar learners were similar in the “A”/”B” exam grade range, but were very different in the “C”–”F” exam grade range. Within this latter range, the majority of abstraction learners (73%) were in the “C” exam grade range; whereas, the majority of the exemplar learners (64%) were in the “D”/”F” exam grade range. We suggest that, at the lower achievement levels, this advantage of the abstraction learners may at least in part be due to the differences in their problem-solving approaches (relative to the exemplar learners) that were documented in study 2.
In study 2, we examined via think-aloud interviews how abstraction and exemplar learners who have average exam performance solve Lewis structure problems at different levels of transfer. The important contribution of study 2 was the novel application of think-aloud interviews to reveal for the first time how abstraction and exemplar learners (who have average exam performance) might differ in their approaches to solving retention, near-transfer, and far-transfer problems (specifically on Lewis structure problems). The implication from earlier studies (both cognitive laboratory studies; McDaniel et al., 2014; and classroom studies; Frey et al., 2017; McDaniel et al., 2018; and the current study 1) is that abstraction learners were relying on abstractions and general concepts gleaned from instructed problems, whereas exemplar learners were relying on memorized solutions to instructed problems. However, the evidence for this implication was indirect. The three key findings from the think-aloud data included relatively direct evidence regarding the differences in problem-solving approaches across abstraction and exemplar learners.
Finding 1: Abstraction Learners Outperformed Exemplar Learners even for Students at the Average Exam Performance.
For both abstraction and exemplar learners, as one might expect, their accuracy declined as the problems went from retention to near transfer to far transfer. However, we saw that, even at the retention level, the abstraction learners on average performed better than the exemplar learners; 80% of abstraction learners had correct solutions, but 60% of exemplar learners had partially correct solutions. And by the far-transfer level, all of the exemplar learners had incorrect (80%) or partially incorrect (one learner) solutions, whereas 67% of the abstraction learners had solutions that were partially correct. However, it should be noted that, for the far-transfer problem, none of these students had a completely correct solution. Hence, we see that, even with average exam performing students, the abstraction learners have solutions that are more correct than the exemplar learners, but still are not successful at the far-transfer level.
Finding 2: Abstraction Learners Relied on Understanding and Exemplar Learners Dominantly Relied on Algorithm without Understanding at all Problem Levels.
Looking at the problem-solving approaches that the different learners used, we found that the abstraction learners principally used an approach that relied on understanding (predominantly algorithm with understanding for retention [100%] and near-transfer [67%], and algorithm with understanding and reasoning for far transfer [67%]). Thus, even on the retention problem, the abstraction learners were using an understanding of the underlying principles of the Lewis structure topic, rather than attempting to apply a memorized solution. By contrast, the exemplar learners indicated using a memorized solution for the retention problem (40%), and they predominantly relied on algorithm without understanding for the near-transfer (100%) and far-transfer (80%) problems. So, the exemplar learners were generally relying on memorization or using an algorithm without understanding the underlying principles on which the algorithm was developed. Therefore, even though the students in this study were all performing at the exam average, they seemed to be approaching the same problems differently depending on their concept-building approaches. This possibly implies that different interventions are needed for the two types of learners, even though they are in the same achievement group.
Finding 3: Both Concept-Building Learners Have Weaknesses in Their Metacognitive Monitoring Accuracy Skills.
One key area in which these average-achieving students were similar was their inaccuracy in calibrating their postperformance solution accuracy confidence with the actual accuracy of their solutions. In general, for the majority of the problems, students’ confidence ratings for solution accuracy in both concept building–approach subgroups were relatively high no matter how the students actually performed on the problem. In addition, although the abstraction learners did overall perform relatively better than the exemplar learners, their ratings of confidence did not track with their declining problem-solving accuracy from retention to far-transfer problems. Thus, despite not achieving correct solutions, for the majority of the problems, both types of learners were fairly confident in the accuracy of their solutions. This lack of calibration seems to be a weakness in the metacognitive-monitoring skills of both types of learners and may be one key reason these learners are at the average exam performance level despite approaching the problems in different ways.
Implications
Superficially (based just on exam performance), our abstraction and exemplar interviewees would look similar to instructors; however, on deeper inspection, these two groups show very different types of cognitive processing in the problem-solving process. The three findings about students performing at the exam average may suggest that multiple interventions are necessary to help all students in the average-achieving group. This area of which interventions would best help students having different concept-building approaches is a highly interesting one that warrants more research. For example, it seems that the exemplar learners in the average-performing achievement group need help in developing an understanding of the underlying principles; hence pedagogies that include more immediate feedback during problem solving from instructors about how the underlying principles are embedded in problems might spark these exemplar learners to start developing this insight. By contrast, the abstraction learners in the average-performing achievement group are striving to understand the underlying principles. Consequently, having the opportunity during problem solving to gauge their level of understanding compared with others might allow the abstraction learners to see where their understanding of the underlying principles have fallen short. Hence, these students might better benefit from other social constructivist methods such as peer-led team learning (PLTL in biology; Preszler, 2009; Snyder and Wiles, 2015; Kudish et al., 2016) or learning assistant method (LA in biology; Batz et al., 2015; Talbot et al., 2015; Van Dusen et al., 2015; Sellami et al., 2017), in which students are encouraged and prompted to discuss the underlying principles in depth as they solve the problems together.
In addition, both types of learners might benefit from metacognitive-monitoring interventions that would help them learn to calibrate the accuracy rating of their solutions. Although there are studies comparing student’s preperformance confidence accuracy compared with their performance, not much is known about a student’s postperformance confidence accuracy compared with their performance accuracy. This is a rich area for development, because it is known that students’ metacognitive-monitoring accuracy is deficient in learning and memory (e.g., see the recent handbook on metamemory edited by Dunlosky and Tauber, 2016), and here we have shown it is deficient for problem solving.
ACKNOWLEDGMENTS
We want to thank the two anonymous reviewers and the guest editor of this issue for their thoughtful and highly constructive advice given to us during the revision stage; their careful reading and advice greatly improved this article. This study was supported by a grant to J. Mestre and M.A.M. from the National Science Foundation Division of Undergraduate Education, grant no. DUE-1630128, to the University of Illinois, Champaign–Urbana.