Collaborative Teaching plus (CT+): A Timely, Flexible, and Dynamic Course Design Implemented during Emergency Remote Teaching in an Introductory Biology Course
Abstract
Student-centered pedagogies promote student learning in college science, technology, engineering, and mathematics (STEM) classrooms. However, transitioning to active learning from traditional lecturing may be challenging for both students and instructors. This case study presents the development, implementation, and assessment of a modified collaborative teaching (CT) and team-based learning (TBL) approach (CT plus TBL, or CT+) in an introductory biology course at a Minority-Serving Institution. A logic model was formulated depicting the various assessment practices with the culminating goal of improving the student learning experience. We analyzed qualitative and quantitative data based on students and instructors’ behaviors and discourse, and student midsemester and end-of-semester surveys. Our findings revealed that the integration of multiple instructors allowed for knowledge exchange in blending complementary behaviors and discourse practices during class sessions. In addition, the frequent ongoing assessments and incorporation of student feedback informed the CT+ design during both in-person and emergency remote teaching. Furthermore, this course design could be easily adapted to a variety of STEM courses in higher education, including remote instruction.
INTRODUCTION
In the last decade, several national reports have called for universities to move away from teacher-centered, traditional lecturing to student-centered, active, and collaborative learning in college science, technology, engineering, and mathematics (STEM) classes (American Association for the Advancement of Science [AAAS], 2011; President’s Council of Advisors on Science and Technology, 2012; Association of American Universities, 2017; Laursen, 2019). Student-centered pedagogies, like active learning, are more effective than teacher-centered, traditional lecturing for improving the student learning experience (Prince, 2004; Knight and Wood, 2005; Haak et al., 2011; Freeman et al., 2014) and narrowing the achievement gap for underrepresented students in STEM fields (Gavassa et al., 2019; Theobald et al., 2020). Despite these calls and the body of education research, implementation of these student-centered, evidence-based teaching practices (EBTPs) continues to remain low (Henderson et al., 2012), and college STEM classes are still largely being taught using traditional lecturing, not active learning (Stains et al., 2018). Group-work pedagogies (Hodges, 2018) and collaborative teaching (CT; Lochner et al., 2019) are complementary active-learning approaches that promote students’ cognitive, behavioral, and affective engagement and learning (Fredricks et al., 2004). Thus, we are briefly highlighting salient features of these collaborative learning and teaching modalities.
Team-Based Learning: A Formal Group-Work Pedagogy
Group-work pedagogies are conducive to effective learning experiences, as students participate in small-group activities to share their knowledge and expertise (Hmelo-Silver et al., 2013). The teacher acts as a facilitator in these student-driven activities (Kirschner, 2001; Scager et al., 2016). There are four formal group-work pedagogies, including peer instruction (PI; Mazur, 1997; Kirschner et al., 2011; Michaelsen et al., 2014; Hodges, 2018), problem-based learning (Wilkerson and Gijselaers, 1996), team-based learning (TBL; Michaelsen et al., 2004), and process-oriented guided-inquiry learning (POGIL; Moog and Spencer, 2008). Out of these formal pedagogical approaches using groups of students, TBL is the most commonly used in higher education, as it incorporates efficient use of instructor resources and encourages high levels of student participation (Thompson et al., 2007; Sisk, 2011; Haidet et al., 2014).
More specifically, TBL is a versatile collaborative learning pedagogy in which instructor-formed teams of students are held accountable for preparing for class. The students are assessed via individual and team in-class quizzes, and class time is devoted primarily to teams working on application exercises (Michaelsen, 2002). The teams follow a set sequence of activities throughout a unit. In particular, students are organized into instructor-formed, permanent groups of five to seven members for the entire term and use a self- and peer-assessment system to promote the development of self-managed learning teams (Michaelsen, 2002). During a TBL class session, students’ knowledge is tested individually and in teams through a readiness assurance process (RAP), and students engage in PI with highly organized group activities. Additionally, student discourse and immediate feedback during this PI helps students clarify and extend their knowledge (Michaelsen et al., 2011). More specifically, the RAP consists of an initial individual readiness assurance test (iRAT, a quiz structured around pre-lecture material), followed immediately by the same quiz administered to student teams, termed the team readiness assurance test (tRAT; also sometimes referred to as the group readiness assurance test (gRAT; Michaelsen and Sweet, 2008). The two-step formative assessment process serves to provide individual accountability and motivates students to prepare for class (Burgess and Matar, 2020).
TBL engages students in interacting with the content of their course, their peers, and their instructors through active-learning strategies (Freeman et al., 2014). Because the strong focus is on student collaboration, in contrast to traditional teaching methods, instructors often struggle with the transition to TBL (Tharayil et al., 2018). Additionally, the TBL format demands the instructor’s preparation, coordination, and effective implementation of diverse activities during class, which is the focus of CT pedagogies (Parmelee et al., 2012). Therefore, the implementation of TBL could represent a contrasting situation for both instructors and students who favor and expect only traditional, lecture-based instruction (LeClair et al., 2018; Owens et al., 2020). This is an especially prominent issue in large introductory biology courses, where keeping all students engaged in TBL activities during class is a challenge (Kibble et al., 2016). Thus, TBL formats could incorporate modifications to promote instructors and students transitioning to active learning from traditional instruction.
CT: An Interactive Instruction Format
A complementary approach to facilitate the transition into active learning may involve CT pedagogies. CT, or co-teaching, is defined as two or more instructors working concurrently and collaboratively to deliver instruction to a heterogeneous group of students in a shared instructional space (Cook and Friend, 1995). CT has been implemented and studied mostly in the context of K–12 education (Friend et al., 2010; Tzivinikou, 2015; Pratt et al., 2017), but more recently in college STEM courses (Metzger, 2015; Morelock et al., 2017; Thompson and Dow, 2017; Thompson et al., 2019). CT efforts at different educational levels have provided insights to better develop and deliver STEM curricular activities in higher education. Effective implementation of the CT format requires that instructors achieve three overarching components: 1) co-planning, 2) co-instructing, and 3) co-assessing (Dieker and Murawski, 2003; Conderman, 2011; Murawski and Bernhardt, 2015). Briefly, in the co-planning stage, each instructor is expected to contribute instructional methods, materials, assessments, and accommodations adjusting to the students’ diverse learning needs. In co-instructing, the instructors implement instructional approaches agreed upon and designed during the co-planning (Conderman, 2011). There are six co-instructing approaches described in the literature, including 1) one teach, one observe; 2) one teach, one assist; 3) station teaching; 4) parallel teaching; 5) alternative teaching; and 6) team teaching (Cook and Friend, 1995). There are additional variations in courses taught by multiple instructors that may involve team teaching by alternating instructors (Ware et al., 1978) and by simultaneously integrating special education teaching instructors (Rainforth and England, 1997; Friend et al., 2010). Finally, in co-assessing, the team gathers information about student learning from multiple sources to reflect upon the effectiveness of their instructional efforts (Conderman, 2011). Therefore, we propose the integration of a modified TBL and CT (i.e., CT+) to improve active-learning experiences in higher education, especially introductory biology courses.
Assessment to Monitor the Student Learning Experience
A key aspect of creating a student-centered learning environment is using assessment to monitor and drive the course design and develop the student learning experience (Connell et al., 2016; Furtak et al., 2017; Offerdahl et al., 2018; Brooks et al., 2019). Several validated and reliable assessment tools have been developed to document student-centered, EBTPs like TBL and CT, including self-report surveys, interviews, and classroom observation protocols. Self-report surveys and interviews can generate data about specific EBTPs, especially if the sample size is significant. However, the most direct approach to measure an instructor’s EBTPs is through classroom observation protocols, in which trained observers document practices in real-time or via audio or video recordings (AAAS, 2012). For this study, we used both self-report surveys and classroom observation protocols. First, we developed a self-report survey by adapting a previously used set of survey questions hosted by the Center for Engaged Teaching and Learning (CETL) at our home institution. Second, we used both the Classroom Observation Protocol for Undergraduate STEM (COPUS; Smith et al., 2013) and the Classroom Discourse Observation Protocol (CDOP; Kranzfelder et al., 2019). COPUS is commonly used to measure implementation of traditional lecturing versus active learning (Lund et al., 2015; Stains et al., 2018; Meaders et al., 2019; Denaro et al., 2021), while CDOP is used to measure implementation of teacher-initiated discourse moves or the general conversational strategies used by the instructor to improve student understanding of content knowledge (Kranzfelder et al., 2019, 2020; Alkhouri et al., 2021).
A Case Study to Examine Impacts of CT and TBL on Introductory Biology Students
Case studies allow for engaging exploratory detailed examinations of the changes in instruction–learning experiences to improve education (Yin, 2004). This format also permits the incorporation of qualitative and quantitative analyses for an in-depth description of the students’ and instructors’ experiences. Here, we present a case study integrating the collaboration of multiple instructors, using a modified TBL strategy, in a large introductory biology course at a research-intensive, minority-serving institution (MSI). We collected sample self-reported survey and classroom observation data to drive and assess the students’ learning experience and instructors’ behavior and discourse. Our results showed that students reported a positive learning experience with the new modality, and we propose that this novel strategy can be a useful tool that can be extended to other STEM courses in higher education. We termed this modified pedagogical approach CT plus TBL (CT+). For simplicity, we use “CT+” throughout this article, which implies the integration of CT plus modified TBL. The current CT+ approach, as illustrated in Figure 1, evolved from iterative experiences by the two instructors teaching this same course over nine previous academic terms. During that period, we gathered many classroom observations and much feedback from stakeholders (students, graduate teaching assistants, instructors, and campus assessment resources). In addition to integrating critical components of TBL and CT, CT+ relies on a modified instruction timeline (compared with the classic TBL format) and improved instructor coordination or co-planning (compared with previous iterations of the course; Figure 1). Furthermore, CT+ also includes ongoing co-assessment of the course components fueled by student feedback, thus promoting a more student-centered learning environment. The analysis presented in this case study was performed in the Spring semester of 2020, across two sections of the course. This allowed us to consider the transition from in-person to emergency remote teaching (Hodges et al., 2020) due to the SARS-CoV-2 (COVID-19) pandemic and to monitor the use of teamwork through virtual breakout rooms. We found that CT+ is a dynamic and flexible alternative to the classic TBL/single-instructor format for large-enrollment STEM classes, especially biology courses in higher education.
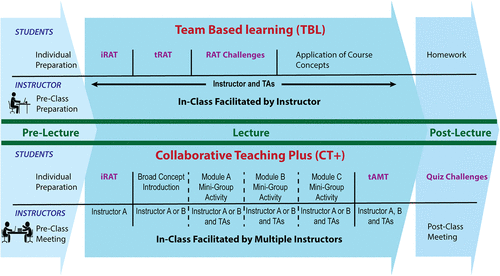
FIGURE 1. Schematic comparison between TBL (top panel) and CT+ (bottom panel). The different components of the two approaches are displayed on the left. The three phases of the instruction format—pre-lecture, lecture, and post-lecture—flow from left to right (arrowheads.) The design content of lectures for both approaches is displayed in the center block. Final block on the right relays any post-lecture components.
The research questions posed were: 1) What instructor behaviors, student behaviors, and instructor discourse occur during a CT+ class session? 2) How do students perceive different aspects of the CT+ course to affect their learning at the middle and end of the semester?
METHODS
Institutional and Course Context
This case study was approved by the Institutional Review Board (UC Merced 2020-3) at the institution of study, and all instructors and students provided informed consent to anonymously participate in the study. We examined the CT+ modality in a large-enrollment undergraduate biology course at a research-intensive, MSI on the West Coast of the United States. The course is part of the introductory biology sequence serving as a gateway to STEM majors with typical enrollments between 200 and 450 students per semester. Each section of this course was delivered as two 75-minute lecture sessions per week for 15 weeks and supplemented by one weekly 50-minute graduate teaching assistant–led discussion session. These discussions reinforced the theme of teamwork by administering worksheet-driven activities in small groups of three to five students. These groups were purposefully different in student composition to those formed in lecture. The graduate student instructors were advised on how to manage group logistics throughout the semester and did not undertake any formal training about the CT+ modality. This study was conducted during Spring 2020, when the course began in the classroom, as a face-to-face offering, but transitioned to synchronous remote instruction via Zoom in week 9 of the 15-week semester.
Students
There were 355 enrolled students in the two sections of this course. The undergraduates in this introductory biology course were predominantly first-generation (73%) and female (70%), more than half identified as Hispanic or Latino ethnicity (53%), 83% were biology majors, and most were freshman (61%). These demographics are reflective of the overall student population at the institution of study (Table 1).
Biology students | ||
---|---|---|
Characteristic | Number (n = 347) | Percent* 4.3% |
First Generation | 252 | 73% |
Gender | ||
Female | 241 | 70% |
Male | 106 | 30% |
IPEDS race/ethnicity | ||
Hispanic or Latino | 185 | 53.3% |
Asian | 84 | 24.2% |
White | 30 | 8.6% |
International | 21 | 6.1% |
Black or African American | 8 | 2.3% |
Multiracial | 14 | 4.0% |
American Indian or Alaska Native | 1 | 0.3% |
Native Hawaiian or Other Pacific Islander | 1 | 0.3% |
Unknown race/ethnicity | 3 | 0.9% |
Major | ||
Biology Sciences | 287 | 83% |
Bioengineering | 31 | 9% |
Other (combined) | 23 | 8% |
Class Standing | ||
Freshman | 211 | 61% |
Sophomore | 102 | 29% |
Other (combined) | 34 | 10% |
To create the semester-long teams for the lectures, students were surveyed on the first day of class by the administration of a nongraded survey. The survey consisted of seven questions adapted from Brickel et al. (1994; Supplemental File S1). Students’ answers were sorted by question type (i.e., gender, year, last science course grade, working in groups, work experience, overseas study, and a critical-thinking question regarding the direction of the Earth’s spin) to create heterogeneous teams of five to six students each. Using a seating chart, teams were allocated seating blocks within which they had to sit for the rest of the semester. These charts, as well as the list of team members, were shared via email with each student before the second class session. Students were first informed of the TBL nature of the course during the first class session. This included a brief introduction to the requirements of the team format. We achieved this by first displaying data from a research study comparing traditional lecturing with active learning, demonstrated active learning by employing an appropriate exercise and then obtaining student buy-in via a survey. We also outlined expectations of every student as suggested by Oakley et al. (2004). During the second class session, time was devoted to discussing team dynamics, defining team roles (based on POGIL; Moog and Spencer, 2008), and answering questions as suggested by Brickel et al. (1994).
Instructors
We observed two biology co-instructors implementing the CT+ modality across two sections of the same course in the same semester. The instructors were senior faculty (one tenure-track research faculty and one non–tenure track continuing lecturer) who regularly teach this course and have complementary disciplinary backgrounds and research expertise in biology. The instructors’ ethnicity aligned with the majority undergraduate demographics, they had training and experience in active learning, and they shared the responsibilities of administering the logistics of the course. Most of the students enrolled in the course identified as female; however, on this occasion, due to department assignment logistics, it was not possible to assign a female instructor.
The interactions and functioning of the co-instructors were critical to a coordinated delivery of the course and unified interactions with the students. To that end, co-instructors met a month before the start of the semester and agreed upon certain course logistics and standards. Throughout the semester, regular weekly meetings between the instructors were used to monitor course progression and manage any challenges. As Table 2 shows, the instructors agreed upon a core set of rules to deliver the course.
Course Content:
|
Course Logistics:
|
Data Collection
The collection of data was primarily carried out by the Students Assessing Teaching and Learning (SATAL) interns, an assessment support team formed by five trained and experienced undergraduates offered by the CETL (Signorini and Pohan, 2019). The data analysis included collaboration with a third biology faculty member, three undergraduates, and two graduate students. In addition, we developed a custom logic model to map all the relevant components and processes driving the student learning experience (Figure 2). A mixed-methods approach using quantitative and qualitative data gathering was employed to analyze data separately and subsequently integrated into the results (Levitt et al., 2018). The survey results were analyzed following the multistep content analysis process in line with Saldaña (2015), as illustrated in Figure 3.
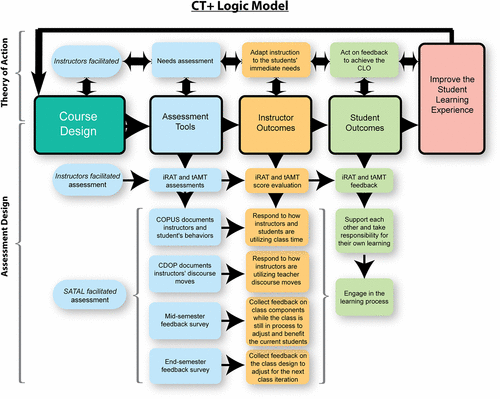
FIGURE 2. CT+ logic model depicts a twofold development process: theory of action and assessment design represented by brackets on the left. The model is read from left to right with the culminating outcome of the improved student learning experience. In broad strokes, the CT+ components are postulated to cause a change in instructor practice that, in turn, influences student behavior and improves the student learning experience. Course design (teal box) is informed by ongoing assessment and evaluation at every level: course (blue), instructor outcomes (orange), and student outcomes (green). The assessment design incorporated collaboration between the course instructors and the SATAL team. The corresponding components are included in the nested brackets and the remaining green boxes. The various arrows represent the flow of information between the respective components. CLO, course learning objective(s).
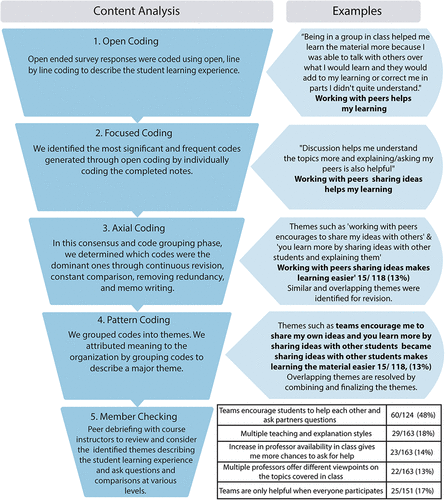
FIGURE 3. Diagram displaying the methodology use for qualitative data analysis. The five steps following content analysis from Saldaña (2015) were used to identify the categories from the open-ended Qualtrics survey (results shown in the left column). Representative examples of each step are listed in the right column.
Data-Collection Instruments and Analysis
Pre-lecture Materials.
Students were provided online access to pre-lecture materials from the onset of the course. Pre-lecture materials consisted of five elements: 1) textbook reading assignments: referencing sections for reading; 2) lecture videos: recorded by the instructors; 3) textbook compendium: a custom set of per-chapter notes composed by one of the instructors (sample chapter included in Supplemental File S5); 4) reading preparedness quizzes (RPQs): a selection of 10–25 nongraded multiple-choice questions centered around interaction with other pre-lecture resources; and 5) past-lecture slides: from a prior non–active learning version of the same course.
iRAT and tAMT.
The change in students’ conceptual understanding was assessed almost every class session by using a pair of in-class quizzes (iRAT and team after module test [tAMT]; examples included in Supplemental File S6). The iRAT consisted of six questions based on the assigned pre-lecture material and was administered at the beginning of that session (as is commonly implemented in TBL; Michaelsen and Sweet, 2008), using Top Hat’s classroom response app (clickers). Eight minutes of class time were allocated to this activity. Students were offered 6 minutes for each iRAT quiz. However, the tRAT was not used but was replaced with an end-of-class team quiz (i.e., tAMT). The tRATs are assessments that 1) consist of the same questions as the iRAT, 2) occur immediately after the iRAT, and 3) provide instant feedback in the form of scratch-off forms or other methods of readily knowing whether the team has chosen the right answer. We replaced the traditional tRAT with tAMTs. The tAMT has a different structure as well as a different function than the classic tRAT. We deferred the administration of tAMT to the end of the class session with the intention of 1) increasing student participation and attendance, 2) collecting feedback about the effectiveness of the in-lecture activities, and 3) promoting heightened engagement within student teams. The tAMT included the same six questions as the iRAT plus four higher-order/critical-thinking questions centered around the main concept delivered in each module of the lesson, for a total of 10 questions. Each tAMT quiz was allocated 10 minutes of classroom time. No student accommodations were implemented, as none of the students requested any this particular semester. Immediately after the end of class, the correct answers and grades for both the iRAT and tAMT were revealed to students, and students had an opportunity to appeal and challenge the answers with the instructors and/or graduate teaching assistants via email or during office hours. Examples of an iRAT and tAMT administered in the course are included in Supplemental File S2.
COPUS
To advance the effective teaching and identify paths to improve their instructional practices, both faculty implementing this CT modality requested documentation of the students’ as well as instructors’ behaviors. These were assessed using the COPUS (Smith et al., 2013). The COPUS report permits the visualization of occurrence and co-occurrence of defined behaviors throughout a class session for one or several instructors. SATAL interns collected COPUS data through live classroom observations. The students and instructors (lead and co-instructor) were observed during an in-person class session in early Spring 2020, before transition to emergency remote teaching. COPUS captures 13 student (e.g., Listening) and 12 instructor (e.g., Lecturing) behaviors that take place during 2-minute time intervals throughout a class session. We followed the code descriptions outlined by Smith et al. (2013), with two modifications: 1) coding for One-on-One Discussions by SATAL interns were based on instructors helping one student or a small group for an extended period of time rather than inclusive of the rest of the class, and 2) Whole-Class Discussion was coded when students were leading a discussion, such as an in-class debate or Socratic seminar (Supplemental Table S1). For a simplified visualization, we combined the 25 COPUS codes and collapsed them into four instructor categories: 1) Presenting, 2) Guiding, 3) Administering, and 4) Other; and four student categories: 1) Receiving, 2) Talking to the Class/Student Working, 3) Assessment, and 4) Other (Supplemental Table S2). This live observation was conducted by three SATAL interns following Smith et al. (2013), and they achieved moderate interrater reliability between coders after training (Fleiss’ kappa = 0.55, 95% confidence interval [CI]: 0.54–0.56). In addition, the SATAL interns met for an additional 30 minutes after the live observation to reach 100% consensus on the coding. Upon completion, the SATAL program forwarded the compiled COPUS results for instructor assessment purposes.
CDOP.
The instructors’ discourse was assessed using the CDOP (Kranzfelder et al., 2019). During the live COPUS observations, SATAL interns collected audio recordings of the instructors for CDOP analysis. CDOP captures 17 teacher discourse moves (i.e., instructors’ disciplinary conversation strategies) that take place during 2-minute time intervals throughout a class session. For a simplified visualization, we combined the 17 CDOP codes and collapsed them into four instructor categories based on Kranzfelder et al. (2020): 1) authoritative, Non-interactive (e.g., providing real-world examples), 2) Authoritative, Interactive (e.g., asking evaluating questions), 2) Dialogic, Interactive (e.g., asking students to challenge each other’s ideas), and 4) Other (e.g., discussing the class agenda; Supplemental Table S3). Three experienced observers (Cohen’s kappa = 0.81, 95% CI: 0.71–0.91) coded the class session recordings using the CDOP training protocol described in Kranzfelder et al. (2019).
Data Analyses for COPUS and CDOP.
To provide an understanding of the sequence of student and instructor behaviors and instructors’ discourse practices in the class period, codes were plotted to create a timeline visualized by the 2-minute observation intervals (Figure 4). For example, each time an instructor or student demonstrated a COPUS code within a 2-minute interval, the code is shown on the timeline by a shaded block. This provides a comprehensive view of which codes are commonly paired together in the class. For example, this timeline shows us that the lead instructor commonly pairs Real-Time Writing with Lecturing. Additionally, the timeline allows us to observe what student behaviors followed instructional practices. For example, we noted that, while the lead instructor is Administering, the students are Taking a Quiz.
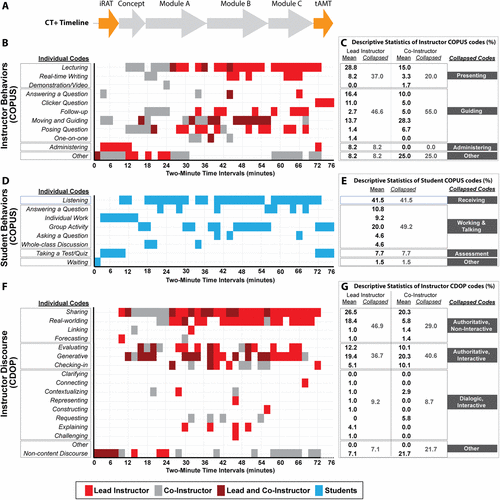
FIGURE 4. Analysis of student and instructor interactions during the CT+ timeline. (A) The CT+ timeline illustrates the components of each element of the session adjusted according to duration (length of the arrows). This timeline correlates directly with the intervals shown in corresponding graphs B, D, and F. (B) Instructor behaviors were measured using COPUS analysis. Individual codes are listed on the left and grouped by collapsed codes. The filled colored boxes (blocks) represent observations of behaviors by the lead instructor (red), co-instructor (gray), and both instructors (magenta). (C) Percentage of time each instructor spent on individual and collapsed COPUS codes during the session. The individual codes are grouped into four collapsed categories (Presenting, Guiding, Administering, Other). (D) Student behavior was measured using COPUS analysis. The individual codes were organized into four categories. The blue boxes correspond to student behaviors observed during those time intervals. (E) Percentage of time students spent with reference to the individual COPUS codes (mean) and total (collapsed) times. The collapsed codes are stated in filled boxes. (F) Instructor discourse was measured using CDOP analysis during the observed session. Individual codes are listed on the left, with grouping. Boxes are color-coded as in A. (G) Percentage of time each instructor spent on individual and collapsed CDOP codes during the session. The individual codes are grouped into four collapsed CDOP codes (Authoritative, Non-interactive; Authoritative, Interactive; Dialogic, Interactive; and Other).
Second, following Kranzfelder et al. (2020), Lewin et al. (2016), and Smith et al. (2014), COPUS and CDOP data were analyzed by the percentage of codes used by both the lead and co-instructor during that class session. To do this, the number of times a code was observed was divided by the sum of all codes recorded for that class session. For example, if Lecturing was marked 20 times, and there was a total of 60 codes, then Lecturing would account for 20/60, or 33% of the total codes. This calculation slightly underestimates the amount of time that an instructor spends on any one behavior, as it counts the behavior relative to all other behaviors.
Qualtrics Surveys.
To obtain student feedback on the CT+ experience, two anonymous surveys were administered online using Qualtrics. Students were asked to complete the midsemester and the end-of-semester surveys through announcements during lectures and via the Canvas site. The surveys consisted of six (midsemester) and 10 (end-of-semester) questions about different class components such as the CT aspect, teamwork, and pre-lecture material (see Supplemental Files S3 and S4). The aim was to gather student perspectives about what is helping with their learning and suggestions that they might have to improve their experience. The midsemester survey (administered in week 7 during in-person instruction) consisted of five Likert-scale questions, each accompanied by a space to explain their responses, and followed by one open-ended question (Supplemental File S3). The end-of-semester survey (administered in week 11 after transition to remote instruction) consisted of six Likert-scale questions, each accompanied by a space to explain their responses, and four open-ended questions (Supplemental File S4). The additional questions were incorporated into the end-of-semester survey to assess the impact that transition to emergency remote teaching had on the teaching practices and to identify students’ needs in the new learning environment. Instructors used the student feedback to both inform the current and next iteration of the course implementing the CT+ experience. The quantitative survey results from the Likert scale questions illustrated in Figures 5 and 6, A and B, were arranged according to students’ level of agreement with the responses and the mean value for each question.
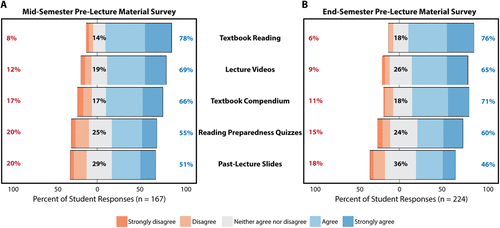
FIGURE 5. Impact of pre-lecture material on students’ learning experience. Midsemester and end-of-semester surveys were used to collect student feedback (A, B). Each row relays levels of student agreement from strongly disagree to strongly agree. Percentages on the left of each box are the combined numbers for students who either strongly disagreed or disagreed (red tones), while those on the right are for the percentage who agreed or strongly agreed (blue tones). The central number corresponds to those who neither agreed nor disagreed (gray boxes).
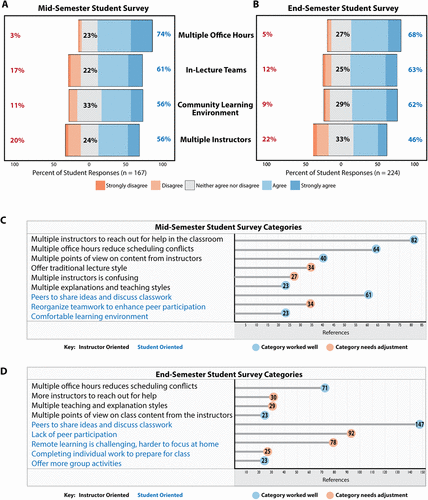
FIGURE 6. Course resources have a positive impact on students’ learning experience. Midsemester and end-of-semester surveys were used to collect student feedback on course resources (A, B). Each row relays levels of student agreement for four resources, from strongly disagree to strongly agree. Percentages on the left of each box are the combined percentages for students who either strongly disagreed or disagreed (red tones), while those on the right are for the percentage who agreed or strongly agreed (blue tones). The central percentage corresponds to those who neither agreed nor disagreed (gray boxes). Open-ended question responses from student feedback were quantified (C, D). The most frequent categories of student comments in the midsemester and end-of-semester feedback was identified, with instructor-oriented comments in black and student-oriented elements in blue. The numbers displayed within each circle refer to the number of times those categories appeared in the survey results (i.e., number of references). The blue circles represent comments with categories that worked well, while orange circles represent categories that needed adjustment.
Data Analysis and Validation of Surveys.
Survey data analysis used a combination of inductive and deductive methods, which allowed categories to emerge as the analysis was ongoing—a strength of the qualitative analysis. To ensure the trustworthiness of the results, multiple criteria were checked as suggested in Shenton (2004). Before working on data coding and analysis from phase 1 (open coding) to phase 4 (pattern coding), the SATAL coordinator and interns initially strategized their roles and research assumptions for this study to ensure consistency of the data analysis in terms of interpreting codes and language. To minimize bias, two trained and experienced SATAL interns independently coded each question and met with the program coordinator to debrief their findings until they arrived at a consensus on the results. In phase 5, course instructors participated in member checking to confirm the emergent categories without knowledge of student identities. The course instructors were shown the interpretation of data to verify the accuracy of interpretations. SATAL interns generated analytic memos throughout the analysis to continue to identify biases and assumptions. Direct participant quotes are presented along with interpretations in the quantitative survey results. Figure 3 represents the “audit trail” used by the SATAL interns to arrive at the results of the experiences and ideas of the students regarding the CT+ modality.
RESULTS
To assess the CT+ modality, course instructors teamed up with colleagues and campus resources to develop, implement, and assess the practices of instructors and students and student perceptions on an ongoing basis in this course. This partnership led to the development of the theory of action (Figure 2). The CT+ logic model was divided into two main components: the theory of action and the assessment design (i.e., instructors and SATAL). We implemented traditional assessment tools, such as midsemester and end-of-semester student feedback surveys, as well as validated and reliable classroom observation protocols, including COPUS (Smith et al., 2013) and CDOP (Kranzfelder et al., 2019). This holistic set of actions allowed instructors to promptly incorporate student feedback in the course design. The instructor outcomes spearheaded the general modifications of the instruction to the students’ immediate needs. The student outcomes reflect the ultimate recipient of the ongoing improvements added by instructor feedback, hence closing the assessment cycle, as have been shown in previous studies, including Bennett (2011) and Brooks et al. (2019). The integration of all these components led to a customized logic model for CT+ that includes an array of assessment tools used to document, understand, and improve course effectiveness.
To assess in-lecture activities in the CT+ modality, we analyzed student and instructor behaviors during a class session over 2-minute time intervals (Figures 3 and 4). Quantitative analysis of student and instructor behaviors was obtained by using the COPUS tool (Smith et al., 2013), with the CDOP tool used to evaluate instructor discourse moves (Kranzfelder et al., 2019). The COPUS and CDOP results showed the student and instructor behaviors and instructor discourse were consistent with the activities occurring during the CT+ format. The most common student and instructor behaviors were plotted over the time of the lesson and aligned with the CT+ timeline (Figure 4). The specific instructor behaviors were recorded and organized based on the collapsed codes during class time (Figure 4, B and C). The results showed the instructor behaviors complemented each other and overlapped minimally as they facilitated CT+ activities (Figure 4B). For instance, evidence of the dynamic roles of the instructors in the class session is shown during module A (Figure 4, A and B), when the two instructors exchanged roles (minutes 26–28, see the transition of top bar from gray to magenta to red). Following the delivery of the concept, the co-instructor continued with facilitating the opening of module A. Thereafter, the lead instructor joined the co-instructor in a collaborative dialogue (magenta) with the students, before assuming the primary role for the remainder of the session (modules B and C). The primary role of the lead instructor was further supported by the descriptive statistics corresponding to the COPUS Presenting collapsed code (i.e., 37% for the lead instructor and 20% for the co-instructor; Figure 4C).
The most prominent activities in the lecture were associated with the Guiding collapsed code, which was equivalently distributed among the instructors (i.e., 46.6% for the leading instructor and 55% for the co-instructor; Figure 4C). This is further illustrated when the lead instructor was Lecturing and the co-instructor was Moving and Guiding students in active-learning practices (minutes 30–32). The co-instructor encouraged students to note pertinent aspects of the lecture, preparing them for upcoming activities. In another instance, both the lead and co-instructor reinforced certain behaviors (Moving and Guiding; minutes 44–56; see the corresponding magenta bar in Figure 4B), which aligned with the delivery of module B. For the final module (module C), the lead instructor conducted an interactive session with the class using Guiding behaviors: Posing and Answering Questions, Following-Up on group activities, One-on-One conversations, and Moving and Guiding, while the co-instructor was observing student behaviors (i.e., Other). Out of all the collapsed codes, the least amount of class time was devoted to Administering the clicker questions and Other activities associated with dealing with technical difficulties and sharing announcements (8.2% and 8.2%, respectively, for the lead instructor, and 0% and 25%, respectively, for the co-instructor; Figure 4C).
The specific student behaviors were recorded and organized based on the collapsed codes during the class session (Figure 4D). The student behavior was distributed heterogeneously across different activities. At the commencement of the lesson (minute 2) students engaged in an iRAT, reflected in the figure as Individual Work and Taking a Test/Quiz. During the delivery of the concept, students are doing Individual Work and Listening to the instructors (minutes 6–18). Throughout modules A–C, the class dynamics encouraged students’ Answering a Question, Asking a Question, and participating in Group Activity (minutes 18–72). The administration of the tAMT at the conclusion of the session showed that students were performing a Group Activity, while Taking a Test/Quiz (minute 72 onward). Indeed, our analysis with the collapsed codes strongly supports that the student behaviors were mostly dedicated to Working and Talking activities (49.2%; Figure 4E). This was followed by behaviors related to Receiving (41.5%; Figure 4E). The perceived extended level of Listening may be overestimated due to the 2-minute time intervals employed to code these behaviors. For instance, if an instructor interjected clarifications about content to the students during any time interval, these would have been coded as Listening, together with other behaviors. The remainder of the class time was devoted to assessments (7.7%) and Other (i.e., 1.5% waiting).
Lead and co-instructor discourse practices were plotted over class time, aligned with the CT+ timeline, and organized based on the collapsed codes (Figure 4, A, F, and G). A prominent feature of the analysis was the overlap in discourse moves between the lead and co-instructor as they facilitated CT+ activities (i.e., modules A–C). For instance, during the delivery of the concept (minutes 8–16), both lead and co-instructors were using Sharing, Generative, and Evaluating discourse moves, but as they moved into module A, they switched to distinctive discourse approaches. In module A, the lead instructor was reviewing core knowledge before administering the group assignment (i.e., Sharing, Real-Worlding, and Evaluating), and the co-instructor was interacting with the students and assessing their understanding through questioning (i.e., Contextualizing). Out of the three modules (A–C), module B was the most evolved with respect to instructional practices, such as well-structured and timed activities for team discussions (influenced by feedback from prior semesters), use of the overhead projector to draw out relatable examples, and posing many questions, which involved a wide variety of discourse moves from Real-Worlding to Explaining. As the lesson transitioned to module C, the instructors moved back to mostly using Sharing, Real-Worlding, Evaluating, Generative, and Connecting, making moderate use of prepared slides to convey facts, as well as use of a simple but time-extensive classroom skit. From highest to lowest, the most individual CDOP codes implemented were Sharing (27%), Generative (19%), and Real-Worlding (18%) for the lead instructor and Non-content Discourse (22%), Sharing (20%), and Generative (20%) for the co-instructor.
CDOP results showed that both instructors were using more Authoritative (i.e., Sharing, Real-Worlding, Evaluating, and Generative) versus Dialogic discourse approaches (i.e., Explaining; Figure 4G). While the lead instructor mostly used an Authoritative, Non-interactive discourse approach, the co-instructor mostly used a more Authoritative, Interactive discourse approach (i.e., 46.9% and 40.6%, respectively). Additionally, the lead instructor was using less Other than the co-instructor (7.1% and 21.7%, respectively). Both instructors used Dialogic, Interactive moves less frequently than other discourse approaches (9.2% and 8.7%, respectively).
To assess course components employed in the CT+ modality, we first focused on the pre-lecture material, which consisted of different resources to prepare the students for in-class learning activities. The information was collected from the midsemester and end-of-semester surveys. The results suggested that students mostly agreed with the usefulness of the pre-lecture material offered (Figure 5). Students identified the textbook reading (78% for midsemester and 76% for end of semester) as the most helpful pre-lecture material in preparation for the in-class learning activities. One student described the value of reading the textbook as follows: “The textbook by far is the most useful element in helping me comprehend the material as the information it contains is so in-depth that it covers the same, if not more, material [than] we go through in class” (Supplemental Figures S1 and S2). Students also agreed that the video lectures (69% and 65%, respectively), reading material from the textbook compendium (66% and 71%, respectively), and the readiness quizzes (55% and 60%, respectively) helped them prepare for the in-class activities. In addition, many students found that the RPQs were a good motivator to read the textbook (55% and 60%, respectively). Overall, the data indicated that the students perceived the material to be effective in helping them prepare for the in-class activities (Figure 5).
To assess the overall student learning experience in the introductory biology course, we administered surveys in the middle (midsemester) and at the end of the course (end of semester). For the end-of-semester survey, besides the pre-lecture material questions previously described, students responded to four additional questions consisting of Likert-scale and open-ended exploratory questions about relevant course aspects, including: 1) multiple office hours, 2) in-lecture teams, 3) community learning environment, and 4) multiple instructors.
The midsemester student survey generated a total of 167 responses from the 355 registered students (47% participation rate; one student dropped the course at this stage), suggesting that completion bias could have informed results due to the low response rate (Figure 6A). From the highest to lowest level of consensus, students agreed and strongly agreed that: 1) the multiple office hours options increased their likelihood of attending them (74% of respondents); 2) being part of a learning team in lecture encouraged them to participate in class (61%); 3) the simultaneous presence of multiple instructors during in-class time helped them to achieve the learning outcomes for the course (56%); and 4) the classroom learning environment gave them a sense of belonging to a team-learning community (56%). We further analyzed the exploratory open-ended questions in the survey by applying the content analysis steps as previously described in Saldaña (2015). This additional analysis gathered the student perspectives on the in-person and remote learning experiences, highlighted the most common categories, and recorded them as references.
The most frequent student-referenced categories revealed the benefit of having multiple instructors in the classroom and the collaborative aspects of CT+, as described in Figure 6, C and D. The categories are listed according to instructor-oriented and student-oriented responses. Blue circles indicate categories with positive outcomes, while orange circles reference categories identified by students as needing improvement. Of the instructor-oriented categories, the possibility of reaching out for help (82 references) was the most prominently mentioned in survey responses. Common student comments are relayed in Supplemental Figures S3 and S4. One student described it as follows: “There were enough people to go around, answer questions and help a large number of students.”
The next most common category referred the students’ ability to attend multiple office hours (64 references), in particular because of their conflicting schedules. Students also highlighted the benefits of having multiple instructor points of view on content (40 references) and multiple explanations and teaching styles (23 references). In addition, the student cluster showed that students appreciated the collaborative learning environment by frequently stating the value of having peers with whom to share ideas and discuss classwork (61 references) in a comfortable learning environment (23 references). Furthermore, the content analysis (orange circles) also provided important suggestions about future modifications to the course that may include adjusting the teamwork activities to promote better peer participation (34 references) and incorporating the traditional lecture style (34 references). Some students identified the presence of multiple instructors as confusing (27 references).
The end-of-semester survey was administered following the transition to emergency remote teaching due to the COVID-19 pandemic. This survey had a higher response rate, with a total of 224 out of the 335 students responding (i.e., 63% compared with 47% for the midsemester survey). The reason for an increased response rate for this survey was probably due to multiple factors. One of these could have been that students wanted to share the need for peers to understand ideas, discuss classwork, and student participation at a critical time. According to the end-of-semester feedback received, students agreed that moving to remote instruction had impacted their learning experience (80%; Supplemental Figure S5). Also, students’ appreciation for being part of a learning team increased (63% from 61%), as well as the sense of belonging provided by the learning environment (62% from 56%) compared with the midsemester feedback results. However, the presence of multiple instructors (46%, down from 56%) and having multiple office hours (68%, down from 74%) were rated less favorably by the end of the semester (Figure 6, A and B).
The qualitative data analysis from the end-of-semester survey revealed that the students had a different pattern of references from the midsemester results (Figure 6, C and D). The references are displayed in Figure 6D. The transition to remote learning increased most students’ appreciation for the in-person collaborative learning environment. This was reflected by the increase in references (147) related to the modified peer interactions to share ideas and discuss classwork during remote instruction. The 147 references referred to the students’ newfound appreciation and an increased need to share ideas and discuss classwork with peers. A student described the experience as follows: “Being in a group in class helped me learn the material more because I was able to talk with others over what I would learn, and they would add to my learning or correct me in parts I didn’t quite understand.” Another student described the transition in the following way: “Remote learning is an extreme challenge for me because there are many distractions at home. So, it is a bit difficult being able to fully concentrate on my coursework like readings and studying.” Other students commented as follows: “It is much easier to learn inside of the classroom than it is inside of your actual room of your home” and “There is less motivation when working online and it makes it harder for those of us that are visual learners.” As some students identified the difficulty with in-person interactions (92), others preferred online instruction. For example, one student comment was: “Communicating with others was easier online than in person.” Another wrote: “Attending office hours or supplemental instruction sessions were easier online because I was shy to attend in person.” There were also references to the challenges associated with the transition to remote instruction (78) as issues needing to be addressed in future course design and delivery if taught remotely. Nonetheless, the option to attend multiple office hours with different instructors remained a highly referenced comment (71) in the remote learning environment (Figure 6D).
DISCUSSION
In this case study, we developed, implemented, and assessed the impact of the CT+ modality on instructor and student behaviors and instructor discourse practices and student perceptions of the learning experience in a large-enrollment introductory biology course. This pedagogical approach integrated a modified CT and TBL and relied on frequent, ongoing assessment. The collaborative learning and teaching experience we presented in this study could be adapted for all undergraduate course levels (e.g., lower and upper division) and across STEM disciplines (e.g., chemistry and physics) with diverse institutional, student, and instructor demographics.
The CT+ logic model mapped the relevant assessment tools to integrate the qualitative and quantitative data driving the student learning experience (Hattie and Timperley, 2007; Bennett, 2011; Brooks et al., 2019; Figure 2). This holistic representation allowed instructors to assess and incorporate changes to the course design based on both observational data and student feedback. Furthermore, the logic model facilitated coordination and collaboration of instructional experts (e.g., research, teaching professors, and contingent faculty) and other campus resources (e.g., SATAL) with the collection and analysis of the data in an unbiased manner. The embedded logic model proved useful in adjusting and rearranging the course components in every iteration, offering a dynamic and flexible course design. In addition, the logic model is an integrative framework for a multiple assessment tool impact analysis, which could serve as complementary evidence for the teaching evaluation dossier of each instructor of record and could be used for tenure and promotion, programmatic, and institutional assessment (Cooksy et al., 2001; WK Kellogg Foundation, 2004; Knowlton and Phillips, 2013).
The CT+ modality made a diverse array of pre-lecture materials available to students. This comprehensive set of resources provided choice and helped prepare them for the in-class learning activities, including the RATs and modular group activities. The value-added items included in the pre-lecture materials, other than commonly used textbook readings, lecture videos, and past-lecture slides, were the textbook compendium and the RPQs. The compendium is an evolving tailored synopsis of common and core concepts pertinent to the course and relayed the information in easy-to-understand language (sample chapter included in Supplemental File S5). Additionally, the students had access to the optional, nongraded RPQs, which offered an added opportunity for students to check their knowledge before taking the graded iRAT (example included in Supplemental File S6). Access and guidance to these pre-lecture materials were centralized in a supplied course timetable for students. The usefulness of the pre-lecture material, as reported by the survey results, was consistent in the in-person and remote format of instruction. Although students mentioned that transitioning to remote instruction impacted their learning, the top three most helpful pre-lecture material to complete the class-activities remained unchanged. Students perceived that the pre-lecture material prepared them for in-class activities throughout the semester. Additionally, our findings suggested that the pre-lecture material could be easily adapted to different modes of instruction, including online instruction. Engaging with the pre-lecture material shifts the responsibility for learning to the students, who engage actively in a range of activities on their own that is relevant to their learning in higher education during the COVID-19 pandemic and beyond (Nerantzi, 2020).
One of the modifications we introduced in CT+ was the separation of the two quizzes—the iRAT was administered at the beginning of each lesson, while the tAMT was taken at the end of the class session. A previous study in an upper-division physiology course found that students performed better on the classic tRAT when they took the iRAT and the tRAT versus the tRAT alone (Gopalan et al., 2013). The changes in administration of these quizzes resulted from students’ suggestions in previous iterations of the course. Before this modification, some students would depart the class session soon after the classic tRAT was concluded and the answers shared. Several students shared that the reason they would not remain for the duration of the lesson, and leave after the tRAT, was that they were familiar with the topics and could better use that time elsewhere. An important consequence of the separation of the iRAT and tAMT was that students remained present and engaged in the learning process with their peers. Thus, the separation of the two in-class quizzes provides an example of the modifications resulting from the students’ feedback and a demonstration of the flexibility of the CT+ modality.
Our classroom observation data allowed us to examine how the CT+ modality impacted our teaching and discourse practices. Based on our sample COPUS data, it appears both instructors spent about 50% of the class time using student-centered Guiding behaviors, such as Moving and Guiding students through TBL activities. This outcome can be compared with other introductory undergraduate STEM classrooms where the instructors spend about 75% (Stains et al., 2018) to 80% of the time (Akiha et al., 2018) on teacher-centered, traditional lecturing. The CT+ modality allowed the lead and co-instructor to switch between active-learning activities and lecturing depending on the phase of the CT+ timeline. In particular, the CT+ timeline followed the basic elements of the 5E model as described by Tanner (2010): 1) engagement (i.e., engage interest and activate students’ prior knowledge with a question, task, or problem), 2) exploration (i.e., student-driven information acquisition by grappling with a problem on their own or in groups), 3) explanation (i.e., the introduction of terms and concepts by the instructor), 4) elaboration (i.e., application of the newly acquired knowledge to a novel situation), and 5) evaluation (i.e., opportunities for students to reflect on and demonstrate their understanding or mastery of concepts and skills; Atkin and Karplus, 1962; Tanner, 2010; Withers, 2016). Thus, before class and at the start of class during the iRAT, students are experiencing engagement; however, during the broad concept introduction, one of the two instructors is Explaining terms and concepts. During modules A through C, students elaborate and apply their newly acquired knowledge in a new situation in small teams. And finally, during the tAMT, students have opportunities to work in their small teams to reflect on, discuss, and demonstrate their understanding of the new concepts and skills.
In addition, our COPUS results suggest different degrees of student-centered teaching practices throughout the CT+ timeline. Not only is the CT+ a flexible course design, but it is also dynamic in terms of the instructors’ co-teaching roles in the classroom. In this format, students had access to alternating instructor roles and viewpoints to clarify material being presented and led to students being engaged and participating in class. This dynamic exchange of roles is commonly observed in K–12 classrooms during CT or co-teaching (Austin, 2001; Dieker and Murawski, 2003; Scruggs et al., 2007; Saloviita and Takala, 2010; Graziano and Navarrete, 2012; Roehrig et al., 2012; Moorehead and Grillo, 2013), but is less frequently implemented in college classrooms (Metzger, 2015; Morelock et al., 2017; Thompson and Dow, 2017; Thompson et al., 2019). In the current case study, the distribution of roles was based on areas of expertise and a somewhat similar amount of material to be covered by each instructor throughout the semester. It is important to emphasize that these instructor interactions in class are discussed during the preclass meetings (i.e., co-planning) to make sure the transition of roles comes up naturally and spontaneously during co-teaching of the lectures.
The expanded analysis with sample CDOP data was intended to complement the sample COPUS data and obtain a deeper perspective on instructor–student and student–student interactions in the classroom. This complementary approach of using multiple observation protocols to analyze one class session can provide a more accurate representation of the student-centered pedagogies in undergraduate STEM classrooms (Lund et al., 2015; Kranzfelder et al., 2020; McConnell et al., 2021). In our case, integrating CDOP into the analysis revealed another piece of evidence that was not readily evident from the COPUS analysis alone. For example, the CDOP was able to identify that the lead instructor used more Authoritative, Non-interactive approaches, while the co-instructor used more Authoritative, Interactive discourse approaches. Also, the simultaneous presence of multiple instructors, as in the CT+ modality, allowed for dynamic interchange between both teacher-centered, Authoritative moves and student-centered, Dialogic moves.
Further, additional supportive assessment measures of the CT+ modality included gathering student feedback on the learning experience through surveys, quizzes, and homework assignments as well as from conversations with students and the teaching assistants throughout the semester. The student surveys were collected at two time points, midsemester and end of semester, which coincided with in-person and remote instruction, respectively. These surveys helped the instructors check the pulse of the student learning experience in the course. The midsemester survey data revealed several supportive outcomes of our use of CT+. Students described the benefits of the presence of multiple instructors who explained disciplinary concepts in different ways and addressed student queries from diverse points of view. However, a few students also described the presence of multiple instructors as unusual, confusing, and distracting. There are two equally probable explanations of this student confusion. First, students could prefer and/or expect traditional lecturing and resist active-learning strategies, like collaborative learning (Lake, 2001; Yadav et al., 2010; Tharayil et al., 2018). If this is the case, then our results indicate the need for more student buy-in (Smith, 2008; Cavanagh et al., 2016) at various points in the semester. This would provide opportunities to emphasize the benefits of active-learning activities, especially for those students who see the instructor as the source from which knowledge is extracted and seem unprepared to negotiate that expectation (Tharayil et al., 2018). And second, the multiple instructors’ roles and expectations could have been unclear and confusing for the students (i.e., instructor deficit). In a systematic review of co-teaching in medical sciences, Dehnad et al. (2021) described how a lack of coordination between two instructors could lead to disjoined or distracting class sessions for students. Our findings suggest a need to clearly identify the active and passive instructional roles of the co-instructors and to share that with the students at various points throughout the term. Future plans will include the preparation of specific materials to assist instructors on how to manage different roles in class (e.g., effective timeline to exchange leading and supporting instructor roles in lecture).
Our survey results suggest that teamwork via small-group discussions encouraged students to participate in class. These findings are consistent with TBL research showing the effectiveness of working in small-groups (Chi, 2009; Chi and Wylie, 2014). Small groups prompt students to think about and explain the course material to one another in ways that benefit both the students giving and the students receiving the explanations (Chi, 2009; Chi and Wylie, 2014). However, students also identified that teamwork needed more organization and everyone’s participation to be most effective, which can be a greater challenge in larger classes due to lack of instructor time and resources. CT+ may benefit by introducing precise parameters for student accountability and peer feedback as part of TBL. Thus, future iterations of the course will include individual and group assessments for self-reflection and to incentivize student participation in the team activities (Jacobs et al., 2013; van Lierop et al., 2018; Carrasco et al., 2019).
The emergency transition to remote instruction prompted by the COVID-19 pandemic impacted the CT+ design and the student learning environment. Compared with midsemester, end-of-semester survey results revealed a clear and surprising shift in favor of teamwork and peer collaboration. Perhaps, the use of virtual breakout rooms was sufficient to enhance the perceived value of the teams. Likewise, the availability of multiple office hours and the presence of multiple instructors was another commonly referenced component of the CT+ modality. The transition to remote teaching prompted students to request more team activities, the need for instructors’ flexibility with assignments, and understanding of the way the current pandemic was affecting student lives, as documented by Reinholz et al. (2020), Jung et al. (2021), and Walsh et al. (2021).
Students’ perceptions across the four general course components remained consistent from midsemester to end of semester, even with the emergency transition to remote learning. However, the small downward shifts in having multiple office hours, the community learning environment, and the presence of multiple instructors were expected with the transition to remote learning. Even when instructor office hours were maintained at equivalent times, the use of the electronic interface was perceived to not be as conducive to interaction as face-to-face meetings. These findings are supported by previous work showing that there was a drop-off in such interactions as a result of the transition to the emergency remote teaching (Meeter et al., 2020). Our survey findings showed that one of the students’ most referenced aspects was the multiple offerings to attend office hours with different instructors. Recent research suggests that student–faculty interaction outside the classroom can improve student learning and retention of all students, including first-generation and racial/ethnic minority students (Lundberg and Schreiner, 2004; Schelbe et al., 2019). The low attendance at office hours by students from minority groups is a pervasive concern in STEM fields that has been associated with increased chances of dropping out of college (Smith et al., 2017). Therefore, the increased student participation in office hours offered additional opportunities for interactions with the instructors.
The forced remote instruction was inherently more difficult for multiple instructors to interact at the same level as during in-person instruction. In the in-person classroom setting, the multiple instructors were always visible, and their presence was apparent at all times. This aspect of the CT+ modality did not initially transfer well to the emergency remote format, even though instructors could be seen and heard online. The instructor pre-lecture meetings permitted appropriate planning and adjustment to adopt new instructors’ dynamics. Furthermore, in subsequent iterations of the course, CT+ instructors were able to use these assessment results to rethink their in-class collaboration. Instructors started to support the class sessions in new ways by using the Chat function in Zoom to respond to students’ questions and for concept clarification, actively moving and guiding teamwork by visiting student teams during the breakout room activities, and promptly responding to technological issues and maximizing support and connections with students.
Limitations and Future Directions
There are several factors that limit our study and provide opportunities for studies in the future. First, we conducted a convenience sample in our large-enrollment introductory biology class at one MSI; therefore, our results have limited generalizability. The instructors of this biology class had been collaborating with the SATAL program on formative feedback for many years and wanted to share their own and their students’ experiences with the greater biology education community. The case analysis we present does not allow us to make conclusive recommendations about the suitability and impact of CT+ in contexts beyond our own. Nonetheless, we believe that the collaborative learning and teaching experience described in this study could be easily adapted at all course levels (e.g., lower and upper division) and across disciplines (e.g., chemistry and physics). Second, we characterized instructor and student behaviors and instructor discourse based on one classroom observation; however, at least two classroom observations are ideal for characterizing teaching and learning practices, especially for COPUS data (Lund et al., 2015). As a result, we recommend that this biology classroom, and other similar classrooms, collect data at multiple classroom observations each semester to get a better understanding of their classroom dynamics for assessment purposes. Third, we have not established the optimal threshold for the use of specific teacher discourse moves (i.e., do certain moves lead to more student learning?). In the future, we could provide teaching professional development, including video-based professional development (Tekkumru-Kisa and Stein, 2017; van Es et al., 2019) or transcript-based lesson analysis (Janah et al., 2019), to try and help instructors implement more Dialogic discourse moves. Long-term studies could engage quasi-experimental designs to document the most effective sequence and combination of discourse moves for particular modules and activities of the course (O’Connor et al., 2015, 2017). Ultimately, incorporating a variety of teacher discourse moves could lead to students developing their higher-order thinking skills (Styers et al., 2018) and long-term retention and transfer of information and knowledge in upper-division coursework (Pérez-Sabater et al., 2011; Schwartz et al., 2011; Vanags et al., 2013). Fourth, the CT+ modality has shown many teaching and learning benefits to both the instructors and students. Nonetheless, CT is more expensive than solo teaching. We envision new alternatives for CT+ that include team teaching involving faculty and teaching assistants, which could reduce institutional costs while maintaining instructional quality. The perceived flexibility of the CT+ model is open to modifications of the teaching team. Therefore, future studies will be needed to address the cost-efficiency of the implementation of the CT+ modality. Nevertheless, it is likely that the additional institutional investment in multiple instructors for a single course could lead to a lasting impact on students’ early interest in research and reducing attrition in STEM disciplines. And finally, our student perception data were limited to asking them about course design and structure, but future studies could be strengthened by measuring students’ beliefs and attitudes, especially self-efficacy, in the CT+ modality (Bandura, 2008). According to Camfield et al. (2021), through mindset coaching, active-learning techniques, and positive relationship building, instructors can increase students’ capacity to persist through setbacks and succeed in the biology class. Furthermore, Trujillo and Tanner (2014) listed some factors that may support self-efficacy, such as social persuasion, supportive comments from instructors or peers, or vicarious experiences, which derive from observing others. Meanwhile, Dou et al. (2018) concluded that both the number of interactions that students have, as well as the kinds of people students interact with, matter for self-efficacy development in the subject area.
ACKNOWLEDGMENTS
We thank Dr. Chris Amemiya for his contributions to the initial versions of the course design. We would also like to thank all the SATAL interns for their contributions to COPUS data collection and survey data analysis: Sandy Dorantes, Hoa Nguyen, Leslie Bautista, Guadalupe Covarrubias-Oregel, Jesus Lopez, Sara Patino, Andrew Perez, Matias Lopez, Abrian Villalobos, Monica Ramos, Ayo Babalola, and Gurpinder Bahia. Special thanks to members of the Kranzfelder lab, especially Jourjina Alkhouri and Cristie Donham, for their contributions to CDOP data collection. We want to extend our gratitude to the Advanced Studies faculty: Drs. Debra Bukko, Virginia Montero Hernandez, and Anysia Mayer at California State University, Stanislaus, for their valuable input on aspects of the qualitative data analysis and graphs layout. Finally, we want to thank Drs. Jennifer Manilay and Eileen Camfield for their critical review of and suggestions for improving this article. This study was funded by the Howard Hughes Medical Institute Inclusive Excellence (HHMI IE) award no. GT11066 and startup funding from the Department of Molecular & Cell Biology at the University of California Merced to P.K. The National Institutes of Health (NIH), National Institute of General Medical Sciences (NIGMS) award R01GM132753 supports N.J.O.